- 1Department of Psychiatry and Psychotherapy, Central Institute of Mental Health, Medical Faculty Mannheim - University of Heidelberg, Mannheim, Germany
- 2Research Group Stress-Related Disorders, Department of Psychiatry and Psychotherapy, Central Institute of Mental Health, Medical Faculty Mannheim - University of Heidelberg, Mannheim, Germany
- 3German Centre for Mental Health (Deutsches Zentrum für Psychische Gesundheit, DZPG), Partner Site Heidelberg/Mannheim/Ulm, Mannheim, Germany
- 4Department of Molecular Neuroimaging, Central Institute of Mental Health, Medical Faculty Mannheim - University of Heidelberg, Mannheim, Germany
- 5Research Group Translational Imaging, Department of Psychiatry and Psychotherapy, Central Institute of Mental Health, Medical Faculty Mannheim - University of Heidelberg, Mannheim, Germany
- 6Research Group Systems Neuroscience and Mental Health, Department of Psychiatry and Psychotherapy, University Medical Center Mainz, Johannes Gutenberg University, Mainz, Germany
Background: Neuroinflammatory processes in depression are associated with treatment resistance to conventional antidepressants. Ketamine is an effective new therapeutic option for treatment-resistant depression (TRD). Its well-established immunomodulatory properties are hypothesized to mediate its antidepressant effect. In this context, higher levels of inflammation may predict a better treatment response. However, conclusive evidence for this hypothesis is lacking. We thus investigated whether standard peripheral inflammatory cell markers and C-reactive protein (CRP) levels could predict symptom improvement during intravenous ketamine therapy in TRD patients.
Methods: 27 participants with TRD were treated with six weight-adjusted intravenous ketamine infusions (0.5 mg/kg bodyweight) over three weeks. Baseline assessments included CRP, absolute monocyte count (AMC), and absolute neutrophil count (ANC). Depression severity was measured using the Montgomery-Åsberg Depression Rating Scale (MADRS) at baseline (D1), after the first (D3) and before the last ketamine infusion (D18). Raters were blinded for the baseline laboratory assessments.
Results: 13 participants responded to ketamine treatment, and 8 participants partially responded. Baseline AMC showed a strong negative correlation with MADRS change at D3 (r=-0.57, p=0.002) and at D18 (r =-0.48, p=0.010), indicating that a high baseline AMC was associated with greater symptom improvement. A generalized linear model confirmed the association of baseline AMC with symptom improvement during ketamine treatment when additionally accounting for age, sex, and body mass index. Specifically, baseline AMC demonstrated predictive value to discriminate responders and partial responders from non-responders, but lacked discriminative ability between partial responders and responders. Baseline ANC correlated with the MADRS changes at D3 (r=-0.39, p=0.046), while CRP values did not correlate at all.
Conclusions: Our prospective single-arm open-label observational study demonstrated that baseline AMC reliably predicted symptom improvement during intravenous ketamine treatment in TRD patients. AMC could therefore serve as a simple and easily accessible marker for symptom improvement during ketamine therapy in daily clinical practice. Future studies with larger sample sizes and a more detailed longitudinal assessment of AMC subtypes are needed to better understand the specific relationship between monocytes and the neuromodulatory effects of ketamine.
Introduction
Depression is one of the most common and complex diseases worldwide. It affects about 300 million people, representing 4.4% of the global population (1). Treatment-resistant depression (TRD) is a particularly challenging form of the disease that is associated with substantial burden and high economic costs (2). TRD is usually characterized by the failure of at least two pharmacological antidepressant interventions, although definitions vary (3). Different analyses of the STAR*D study data showed markedly heterogeneous results regarding its prevalence, estimating the percentage of depressive patients affected by treatment resistance from 10 to 70% (4–7).
Even though TRD might not reflect a separate neurobiological entity compared to major depressive disorder (MDD), emerging evidence suggests that TRD has distinct neurobiological features (8). One such feature could be elevated inflammation levels (9). Although the influence of the immune system and its role in psychopathology have not yet been sufficiently understood, multiple reviews have reliably demonstrated elevated inflammatory markers in TRD (10, 11). The majority of immune biomarker research has thereby focused on cytokines (12). In this context, some studies suggested that the extent of inflammatory processes could influence or even predict the response to alternative treatment options in TRD (13). The other way round, treatment of TRD might also modulate inflammatory processes and thereby improve psychopathology (14, 15).
One of the most promising treatment approaches for TRD is subanesthetic intravenous infusion therapy with ketamine (16). In various administration forms, accumulating evidence has revealed robust fast-acting antidepressant effects of ketamine and its enantiomer esketamine in patients with TRD (17–19). However, considering that clinical response to ketamine can only be observed in around 50% of TRD patients (20, 21), treatment decisions lack reliable predictors of individual patient benefit. Thus, it would be advantageous to have markers which indicate who will respond to ketamine early in the treatment.
Glutamate levels in the brain are influenced by inflammatory processes (22). Inter alia, ketamine acts as a N-methyl-D-aspartate receptor (NMDAR) antagonist, thereby modulating glutamate via the mTOR pathway, so that anti-inflammatory effects have been investigated even before its use in psychiatry (23). Similarly, neuroplasticity, which has been postulated as one of the main mechanisms underlying ketamine’s antidepressant effects (24), seems to have a bidirectional link with inflammation (25). In addition, there is evidence that ketamine has an effect on the gut microbiota, which could be explained by antibacterial and anti-inflammatory processes in the gut-brain axis (26). This raises the question whether the antidepressant effect of ketamine might potentially be mediated by a reduction of inflammatory processes. In this context, higher levels of inflammation could potentially predict a better treatment response in TRD patients. However, evidence for these hypotheses remain inconclusive.
The elevation of the pro-inflammatory cytokine interleukin 6 (IL-6) is one of the most reliable findings in TRD patients (27). Conversely, there have been contradictory results concerning the levels of IL-6 in patients who responded to ketamine. One small study showed a quick decrease of IL-6 after ketamine infusion and that baseline levels of IL-6 predicted antidepressant treatment response (28). This study, however, was criticized for its methodological flaws and could not be replicated in a broader sample (11). Changes in other acute phase proteins, such as C-reactive protein (CRP) or the cytokine tumor necrosis factor-alpha (TNF-α), were also not associated with the response to ketamine (29, 30). However, immune reactions are much more complex, and cytokines reflect only a part of the multi-step processes involved in the immune cascade. Potentially, alterations in the cellular components of the immune system at baseline, which are easily accessible and routinely estimated in patients, could be associated with the therapeutic outcome of ketamine treatment.
Specifically, we hypothesized that higher baseline inflammatory cell counts correlate with symptom improvement during a three-week intravenous ketamine therapy in TRD patients. We focused on absolute monocyte and neutrophil counts at baseline and additionally examined the acute phase protein CRP as a non-cellular marker for acute inflammation. In a next step, we probed whether these baseline inflammatory cell counts provide predictive value for discriminating different therapeutic outcomes during ketamine therapy. Such a marker could be a first step towards a more personalized treatment selection for TRD patients.
Methods
Study design
This prospective single-arm open-label observational study was conducted at the Central Institute of Mental Health (CIMH), Mannheim, Germany, from August 2022 to March 2024. It aimed to assess the predictive value of routine clinical inflammatory markers for the treatment response to a three-week-long therapy with intravenous ketamine in patients with TRD. The study was conducted in accordance with ethical principles based on the Declaration of Helsinki and consistent with Good Clinical Practice. The Ethics Committee of the Medical Faculty Mannheim at Heidelberg University approved the protocol (Registration number: 2021–902).
Participants
All patients received comprehensive information about the purpose and procedure of the study and provided written informed consent to participate in the study. Eligible candidates were screened for inclusion and exclusion criteria and underwent physical and mental examinations. Treatment resistance was required and defined as a lack of clinical response (< 50% improvement in MADRS) to a minimum of two different classes of antidepressants over a period of at least six weeks for each medication in sufficient dosage during the current depressive episode (2). Patients had to be able to prove how long they had been taking the medication and that they had been classified as non-responsive by a healthcare professional during this time. Participants were included in the study if they were at least 18 years old, had a total Montgomery–Åsberg Depression Rating Scale (MADRS) score of ≥ 20 points at the time of the screening, had a current moderate or severe depressive episode according to the 10th version of the International Classification of Diseases (ICD-10), and signed the written informed consent after the examination and verbal explanation of the purposes and procedures of the study. Exclusion criteria comprised bipolar disorder, ketamine treatment during current or past depressive episodes, previous psychotic symptoms, refusal of clinical or laboratory tests or informed consent, and severe medical conditions contraindicating the administration of ketamine. These included, in particular, acute or chronic inflammatory diseases, heart failure, severe arterial hypertension, unstable angina, myocardial or cerebral infarct within the last 12 months, elevated intracranial pressure, severe or treatment-resistant hyperthyroidism, glaucoma, liver cirrhosis or severe hepatic dysfunction, and current (during the last six months before study inclusion) substance use disorder (all except tobacco-related disorders/caffeine-related disorders; alcohol consumption was limited to ≤ 40 gram for men and ≤ 20 gram for women per day, e.g. ≤ two/one large beer (0.5 L) for men/women).
Patients who met all criteria were registered for treatment in the CIMH’s inpatient unit for affective disorders. Here, an experienced psychiatrist re-examined the participant and re-evaluated the criteria for treatment resistant depression and ketamine treatment. Patients were excluded from the study if they decided to discontinue ketamine treatment or withdraw their consent before the last day of treatment. 52 patients were screened for the study and 27 participants were enrolled. All participants completed the study until the last ketamine administration on D18.
Ketamine application
Ketamine was administered intravenously twice a week over a period of three weeks. The first two administrations took place in the inpatient unit for affective disorders. The following four administrations were administered on an outpatient basis in the Early Clinical Trials Unit of the CIMH, if the patient’s condition permitted. Prior to ketamine administration, patients underwent a thorough physical examination including an ECG. No abnormalities in the pre-ketamine examinations were observed. The first ketamine administration was supervised by an anesthetist. No severe side effects were observed. A few patients experienced changes in perception, dissociation, nausea, vomiting, an increase in blood pressure, or headaches, of which none necessitated the intervention of the anesthetist. Dimenhydrinate (i.v.) was administered in cases of nausea or vomiting, and increased blood pressure with hypertensive symptoms (> 160/100 mmHg) was managed with urapidil (i.v.). Ketamine was administered intravenously at a dose of 0.5 mg/kg bodyweight solved in 50 mL of sodium chloride solution using a syringe pump (BRAUN Perfusor - compact plus, Braun Industries, Hesse, Germany) at a rate of 75 mL/h. Each session lasted approximately 45 minutes. All infusions were administered in a low-stimulus environment. Blood pressure and heart rate were monitored regularly during and up to two hours after the ketamine infusion.
Baseline laboratory assessments
Venous peripheral fasting blood samples were collected at D1 between 8 and 10 am for baseline laboratory assessments. For the collection of AMC and ANC, 3 mL EDTA tubes (S-Monovette® EDTA K3E, Sarstedt, Northern Rhine-Westphalia, Germany) and for CRP 7.5 mL serum tubes (Serum-Gel®, Sarstedt, Northern Rhine-Westphalia, Germany) were used. After blood sampling, the tubes were transported at 4 degrees Celsius to a clinical laboratory in Mannheim for further analysis. AMC and ANC were determined using the XN 9000/1000 with TS-10/SP-10 Celladivision DI-60, RPU 2100R, and XS-800i (Sysmex Hematology Analyzer, Schleswig-Holstein, Germany). Count procedures were performed automatically for AMC and ANC. For AMC, the laboratory reference ranges varied between 0.19 x 109 and 0.77 x 109 cells/L (men) and 0.29 x 109 and 0.71 x 109 cells/L (women). The laboratory reference ranges for ANC varied between 1.82 x 109 and 7.42 x 109 cells/L (men) and 2.00 x 109 and 7.15 x 109 cells/L (women). Quantitative estimation of CRP was performed using the Cobas c701 analyzer (Roche Industries, Basel, Switzerland) based on an enzymatic particle-enhanced immunological turbidity test. The measuring range was between 0.6 and 350 mg/L, and the dilution limit was 350 mg/L using NaCl 0.9% as a dilution medium with a dilution factor of 2. Reference values were defined as < 5 mg/L. In cases of CRP concentrations under 0.6 mg/L (low detection level), these were presented automatically as < 0.6 after analysis. Regarding the statistical analysis, participants with these findings were included dividing 0.6 by 2, as recommended in the literature (31).
Psychometric assessments
The Montgomery–Åsberg Depression Rating Scale (MADRS) is an external assessment tool designed as an interview to evaluate psychopathological symptoms of depression. The MADRS is validated in German (32), has a high internal consistency (Cronbach’s alpha = 0.86) (33), and is a sensitive instrument for the changes in psychopathology associated with antidepressant drug treatment (34). Severity grades are typically defined as follows: mild (7 to 19 points), moderate (20 to 34 points), and severe (≥ 35 points) (35). Clinical response was defined by a reduction of the total MADRS scores of ≥50% (36), and remission was defined as a score of 10 or lower after the ketamine treatment (37). The raters of the MADRS were blinded for the results of the baseline laboratory assessments. The Beck Depression Inventory (BDI-II) is a self-report questionnaire for depression, which is also validated in German and has a high internal consistency (Cronbach’s alpha = 0.86) (38). It was used to assess patients’ subjective perception of depressive symptomatology. Higher BDI-II scores represent higher level of depression, with the following classification for depression severity: mild (14 to 19 points), moderate (20 to 28 points), and severe (≥29 points) (33). MADRS and BDI-II were assessed on D1, D3, and D18.
Statistical analysis
All statistical analysis were performed using the R-based software jamovi 2.5.2 (39) together with the GAMLj toolbox (40). Sample characteristics were described using mean and standard deviation. Shapiro-Wilk test was used to assess normal distribution. All baseline laboratory values were logarithmically transformed due to non-normal distribution. Categorical and count data were presented as numbers or fractions. Bivariate differences between groups were tested using either Fisher’s exact test for categorical variables or Mann-Whitney U-test or Student’s t-test for quantitative variables.
To analyze general differences in depressive symptoms over time, we employed a repeated measures analysis of variance (rmANOVA) for the MADRS and BDI-II scores including D1, D3, and D18. We examined differences in MADRS and BDI-II scores between measurement time points (D1, D3, and D18) and assessed the interaction between response and measurement time points. Effect sizes were quantified using partial eta-square (η²p), with interpretations categorized as very small (η²p < 0.01), small (0.01 ≤ η²p < 0.06), moderate (0.06 ≤ η²p < 0.14), and large (η²p ≥ 0.14) (41, 42). When significant differences were detected, Tukey-adjusted post hoc comparisons were performed, and a significance threshold of ptukey < 0.05 was defined.
Pearson’s correlation analysis was applied to examine associations between absolute and relative changes in depressive symptoms (MADRS and BDI-II score differences for [D3-D1] and [D18-D1]) and baseline clinical laboratory parameters (logCRP, logAMC, and logANC). To estimate an appropriate sample size, a power analysis with G*Power (43) and the appropriate statistical test for a bivariate linear correlation (Pearson’s r) between two non-dichotomous variables (A priori: “Correlation: Bivariate Normal Model”) was performed prior to study initiation. Assuming a relatively strong effect with a correlation coefficient of r = 0.5, an α error probability of 0.05, and a power (1-β) of 0.80, we obtained a sample size of 23 participants. Absolute symptom improvement was calculated as the difference between the MADRS scores (e.g. D3-D1), while relative symptom improvement was calculated as the percentage change compared to the baseline at D1 (e.g. ([D3-D1]/D1)*100). P-values were Bonferroni-corrected for multiple comparison (alpha = 0.05, number of investigated parameters = 3, adjusted p-value = 0.0167).
Further, generalized linear models were used to assess the predictive value of baseline logAMC and logANC when accounting for additional factors. Specifically, in addition to logAMC or logANC, age, BMI, and sex were included as independent variables, with absolute and relative changes in MADRS/BDI-II scores serving as the dependent variables. The parameter estimates and their corresponding 95% confidence intervals and p-values were presented in tables. In this context, significance was determined at p < 0.05, considering that the generalized linear models allowed corrections for the abovementioned sample characteristics.
To assess the discriminative ability of logAMC, we plotted receiver operating characteristic (ROC) curves and calculated the area under the ROC curve (AUC) as well as specificity, sensitivity, positive and negative predictive values, and likelihood ratios using the PsychoPDA package (44). The optimal threshold values were determined using Youden’s J statistic (45). For this approach, we used the trichotomized classification of symptom improvement during ketamine treatment at D3 and D18 into non-responders (<25% MADRS improvement), partial responders (25% to 50% MADRS improvement), and responders (>50% MADRS improvement) and compared the three groups with each other. The ROC curves were illustrated with GraphPad Prism version 8.0 (GraphPad Software Inc.; San Diego, CA, USA).
Results
Sample characteristics
The sample characteristics are illustrated in Table 1. A total of n = 27 patients with TRD (14 females and 13 males) were enrolled in the study. Patients were, on average, 45.44 ± 11.83 years old, had a mean BMI of 27.14 ± 5.94 kg/m2, and 6.70 ± 2.30 previous psychiatric medications. The patients’ mean MADRS scores at baseline (28.59 ± 4.34) indicated an overall moderate depression severity, which was confirmed by the BDI-II self-ratings (33.50 ± 11.09). None of the patients had a relevant concomitant physical illness. In particular, there was no evidence of acute or chronic inflammatory diseases. The most common concomitant somatic diseases were arterial hypertension, obstructive sleep aponeurosis syndrome and chronic migraine, in that order. In addition, no patient was taking immunomodulatory medication (e.g., cytostatics, antibiotics, etc.). Baseline ANC was at 0.52 ± 0.14 x 109 cells/L, logAMC at 0.52 ± 0.14 x 109 cells/L, and CRP at 2.33 ± 2.45 mg/dL. One male participant had an AMC value that was above the normal range. This participant was 38 years old, had no previous chronic inflammatory diseases, no infection at the beginning of the study (at the time of blood sampling) and his physical examination was without pathological findings.
Change of depressive symptoms during ketamine treatment
Over the three weeks, 13 TRD patients (4 females, 9 males) responded to the treatment with intravenous ketamine, as defined by a reduction in MADRS of more than 50% at D18. 8 participants showed a partial response, as indicated by a 25–50% reduction in MADRS scores. 6 participants (5 females and 1 male) did not respond. We observed no significant differences between the three groups with regard to gender (Fisher’s exact test, p = 0.073), the number of psychiatric comorbidities (Fisher’s exact test, p = 1.000) or previous medications (Spearman’s rho = -0.32, p = 0.106).
Although not the main objective of the study, we could observe a significant decrease of the mean MADRS score over the three-week treatment period (rmANOVA, Mauchly’s W = 0.98, p = 0.766, F(2, 52) = 34.78, p < 0.001, η²p = 0.57; Figure 1A). Specifically, the MADRS scores at D3 (Tukey-adjusted post-hoc comparison, MDiff = 9.89, SE = 1.52, p < 0.0001) and D18 (Tukey-adjusted post-hoc comparison, MDiff = 12.78, SE = 1.71, p < 0.0001) were significantly lower than on the pre-treatment screening at D1. We could further observe a similar decrease in mean BDI-II scores (Mauchly’s W = 1.00, p = 0.999, F(2, 48) = 27.06, p < 0.001, η²p = 0.53; Figure 1B) over the treatment period. Again, Tukey-adjusted post hoc comparisons indicated significantly lower BDI-II scores at D3 (MDiff = 8.76, SE = 1.67, p < 0.0001) and D18 (MDiff = 11.84, SE = 1.66, p < 0.0001) in comparison to D1. Finally, we could confirm that the differences between responders, partial responders, and non-responders in absolute and relative MADRS scores were significant for [D3-D1] (Fisher’s one-way ANOVA for absolute MADRS: F(2,24) = 4.87, p = 0.017, Fisher’s one-way ANOVA for relative MADRS: F(2,24) = 4.98, p = 0.016) and for [D18-D1] (Fisher’s one-way ANOVA for absolute MADRS: F(2,24) = 63.55, p < 0.001, Fisher’s one-way ANOVA for relative MADRS: F(2,24) = 62.70, p < 0.001). Post-hoc comparisons showed significant differences between non-responders and responders in absolute and relative MADRS improvement at D3, as well as significant differences between all three groups for absolute and relative MADRS improvement at D18 (Figure 1C; Supplementary Figure S1). For BDI-II scores, only absolute and relative differences at D18 reached significance (Fisher’s one-way ANOVA for absolute BDI-II: F(2,23) = 3.710, p = 0.040, Fisher’s one-way ANOVA for relative BDI-II: F(2,23) = 4.55, p = 0.022). Post-hoc comparisons demonstrated only significant differences between responders and non-responders at D18 for both absolute and relative BDI-II scores (Supplementary Figures S2, S3).
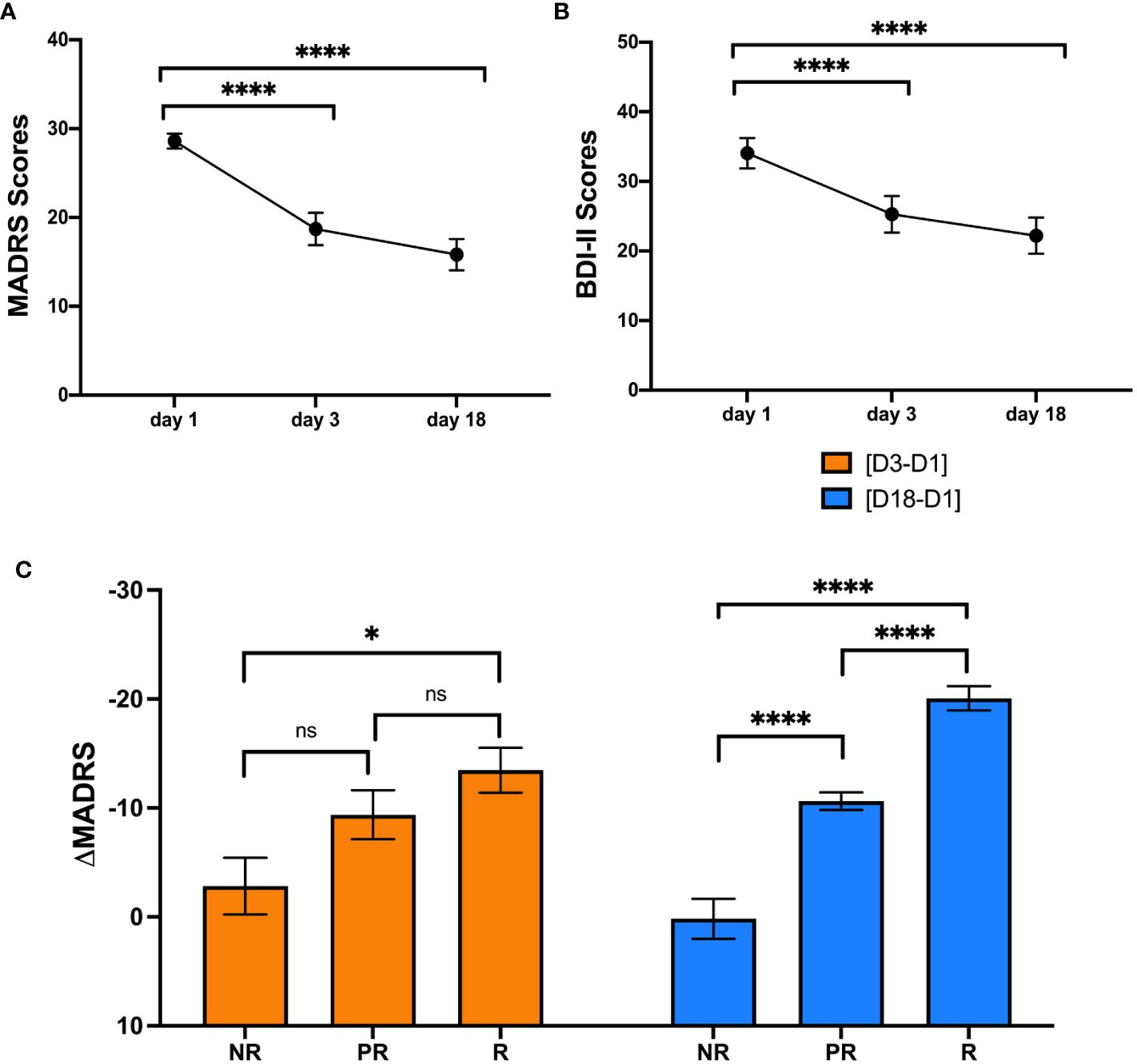
Figure 1 Repeated measures analysis of variance for MADRS and BDI-II scores and comparison of MADRS change between responders, partial responders and non-responders.The MADRS (A) and BDI-II scores (B) are illustrated as mean ± standard error of the mean at D1, D3 and D18. The bar plots in (C) illustrate the change in MADRS score (mean ± standard error of the mean) compared to baseline for D3 (orange, left) and D18 (blue, right) in non-responders, partial responders and responders.BDI-II = Beck Depression Inventory, MADRS = Montgomery-Åsberg Depression Rating Scale, D1 = baseline or day 1, D3 = day 3, D18 = day 18, NR = non-responders, ns = not significant, PR = partial responders, R = responders. * = p < 0.05, **** = p < 0.0001.
Baseline AMC is associated with the improvement of depressive symptoms during ketamine treatment
Our study primarily aimed to investigate the relationship between peripheral cellular inflammatory markers and CRP at baseline and improvement of depressive symptoms during intravenous ketamine therapy. Thus, we first performed bivariate correlation analyses between changes in depressive symptoms (i.e., [D3-D1] and [D18-D1]) and three standard baseline inflammatory markers, namely logANC, logAMC, and logCRP. The results of the correlation analyses are presented in Table 2.
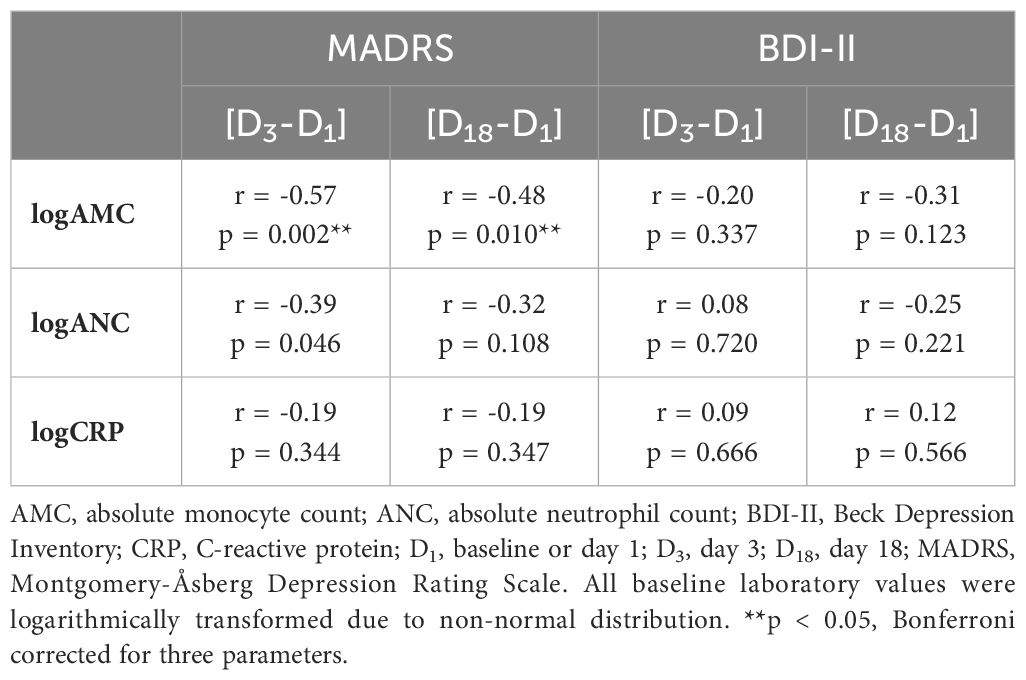
Table 2 Correlation (Pearson’s r) between change of depressive symptoms and baseline laboratory parameters (logAMC, logANC, and logCRP).
Most importantly, baseline logAMC negatively correlated to the absolute MADRS change at D3 (Figure 2, r=-0.57, p=0.002, surviving Bonferroni correction) and at D18 (Figure 2, r =-0.48, p=0.010, surviving Bonferroni correction). Thus, higher monocytes at baseline were associated with greater treatment response to ketamine, as measured with the MADRS. While baseline logANC correlated with absolute MADRS changes only at D3 (r = -0.39, p=0.046, not surviving Bonferroni correction), logCRP did not exhibit any significant association with the absolute MADRS changes. No significant correlations could be observed between the absolute BDI-II changes and the inflammatory markers (Table 2), although the correlation with logAMC at D18 almost reached trend level.
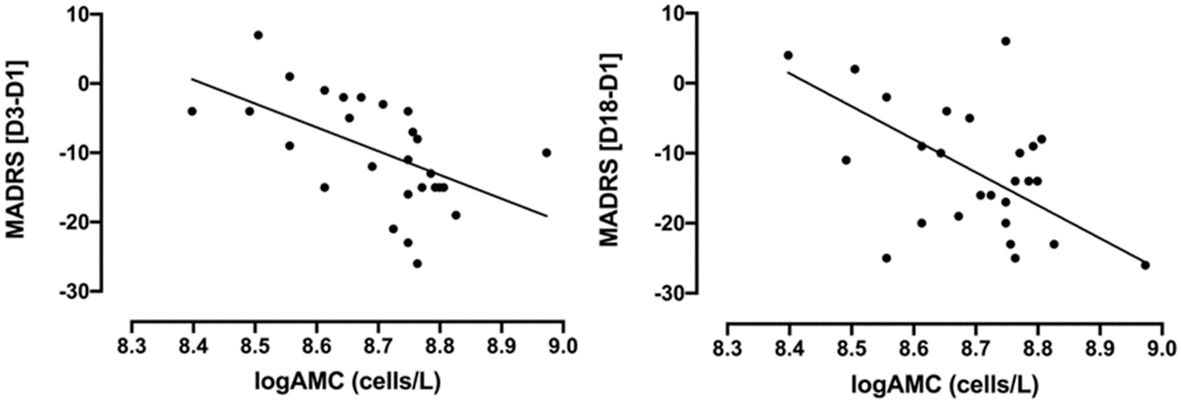
Figure 2 Correlations between absolute changes in MADRS scores (D3-D1 and D18-D1) and baseline logAMC (cells/L) in TRD patients treated with intravenous ketamine for three weeks. MADRS [D3-D1]: Pearson’s r=-0.57, p=0.002; MADRS [D18-D1]: Pearson’s r =-0.48, p=0.010. AMC, absolute monocyte count; D1, baseline or day 1; D3, day 3; D18, day 18; MADRS, Montgomery-Åsberg Depression Rating Scale; TRD, treatment-resistant depression. AMC was logarithmically transformed due to non-normal distribution.
We further investigated relative MADRS changes, as measured by the relative improvement in relation to the baseline at D1, finding similar results (Supplementary Table S1). Specifically, logAMC also correlated to the relative MADRS improvement at D3 and D18, surviving Bonferroni correction at both time points (Supplementary Figure S4; Supplementary Table S1).
Baseline AMC predicts symptom improvement during ketamine therapy in a generalized linear model
A generalized linear model (GLM) was performed to evaluate the predictive value of baseline AMC for treatment response when additionally accounting for other factors. In addition to baseline logAMC, age, sex, and BMI were included in the model as explanatory variables. The outcome was quantified using the absolute and relative changes in MADRS scores between D18 or D3 and D1.
The models explained 42% and 34% of the variance in treatment response as measured by the absolute reduction of MADRS scores at D3 (MADRS [D3-D1], R2 = 0.42) and D18 (MADRS [D18-D1], R2 = 0.34). Baseline logAMC significantly predicted absolute changes of MADRS scores between D3 and D1 (estimate = -38.43, 95%CI [-59.87; -16.99], p = 0.002; Table 3) and between D18 and D1 (estimate = -31.39, 95%CI [-57.16; -5.63], p = 0.026; Table 4). Here, negative beta estimates indicated that higher baseline logAMC were associated with a stronger response to intravenous ketamine treatment. In contrast, no significant effects were observed for age, sex, or BMI (Tables 3, 4). However, baseline logAMC did not predict absolute changes in BDI-II scores between D3 and D1 (Supplementary Table S2) or between D18 and D1 (Supplementary Table S3). Similar results were found for the GLM with the relative MADRS improvement, in which the models explained a variance of 40% and 34%. Here, baseline logAMC also significantly predicted the relative MADRS improvement at D3 and D18 (Supplementary Tables S4, 5), but not the relative changes in BDI-II scores at the two time points (Supplementary Tables S6, 7).
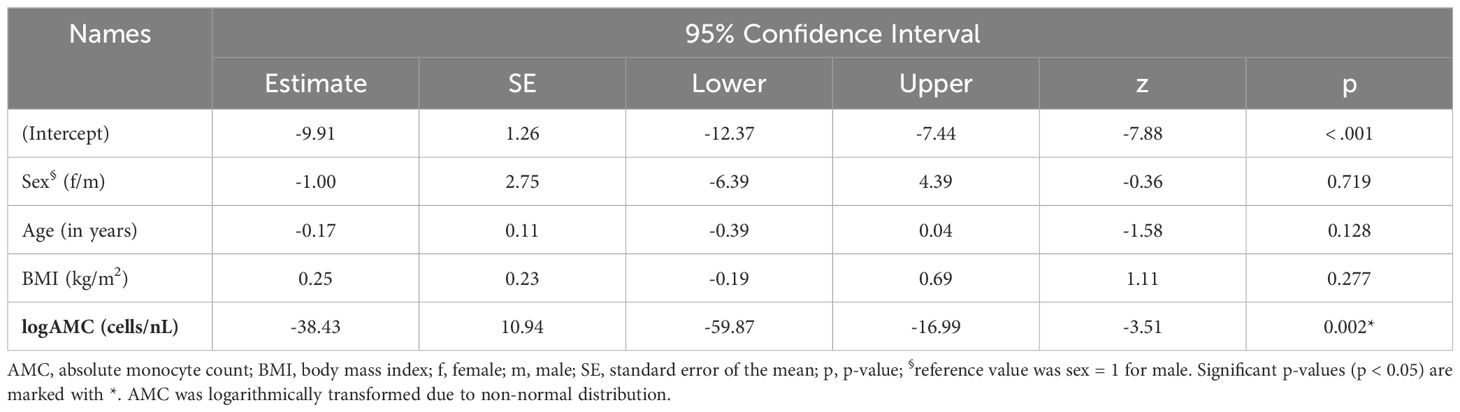
Table 3 Generalized linear model for absolute changes in MADRS score [D3-D1] in TRD patients treated with intravenous ketamine for three weeks.
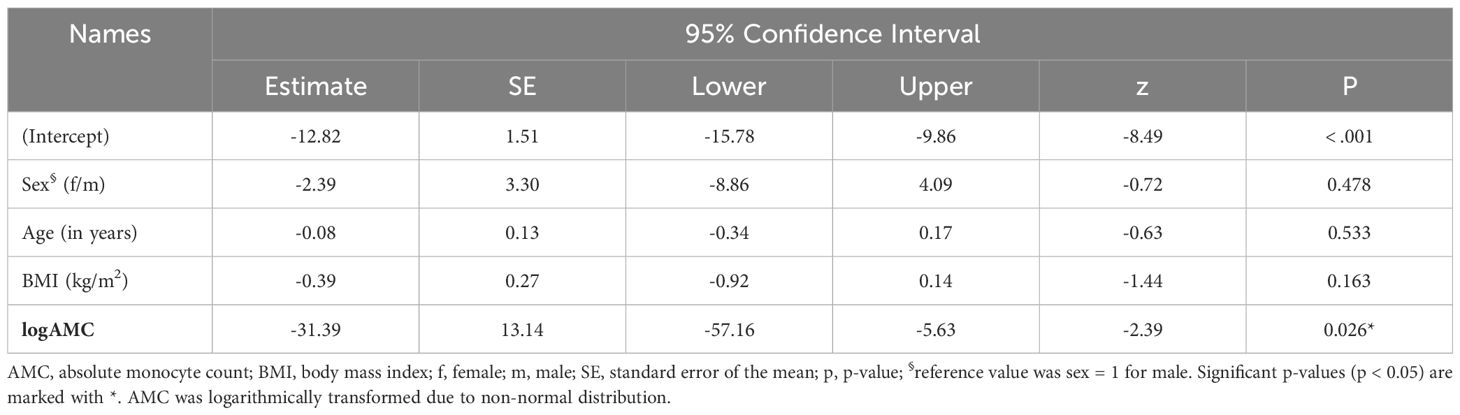
Table 4 Generalized linear model for absolute changes in MADRS score [D18-D1] in TRD patients treated with intravenous ketamine for three weeks.
We also analyzed the predictive value of baseline ANC for response to intravenous ketamine treatment in TRD patients with a GLM. Again, we included the additional variables age, sex, and BMI and assessed the absolute MADRS improvement at D3 and D18 (MADRS [D3-D1], R2 = 0.28; MADRS [D18-D1], R2 = 0.29). Baseline logANC was identified as a significant predictor for absolute changes in MADRS scores at D3 (MADRS [D3-D1]: estimate = -22.37, 95%CI [-41.09; -3.66], p = 0.029; Supplementary Table S8), but only on a trend-level at D18 (MADRS [D18-D1]: estimate = -20.13, 95%CI [-41.08; 0.82], p = 0.073; Supplementary Table S9). In contrast, no significant predictive effects were observed for logANC on relative MADRS improvement at both time points (Supplementary Tables S10, 11). Similarly, baseline logANC did neither predict the absolute nor relative change in BDI-II scores at D3 (Supplementary Tables S12, S14) and D18 (Supplementary Tables S13, S15).
Predictive power of baseline AMC is specifically important for distinguishing non-responders from partial responders and responders
To evaluate the predictive performance of baseline AMC, symptom improvement at D3 and D18 were first divided into the three categories “non-responders” (< 25% MADRS improvement), “partial responders” (25% to 50% MADRS improvement) and “responders” (> 50% MADRS improvement) and then compared with each other using ROC curves (Figure 3). The six ROC curves illustrate the trade-off between sensitivity and specificity across different threshold values of log-transformed monocyte concentrations for the comparisons between the three categories at D3 and D18. Note that the model performed better than random models for the discrimination between non-responders and responders at D3 (AUC = 0.884, p = 0.004) and D18 (AUC = 0.846, p = 0.018) and between non-responders and partial responders at D3 (AUC = 0.844, p = 0.016), while reaching trend-level at D18 (AUC = 0.771, p = 0.093). In contrast, the discriminative ability between partial responders and responders was neither significant at D3 nor at D18. The corresponding sensitivities, specificities, positive and negative predictive values and likelihood-ratios are summarized in Tables 5, 6.
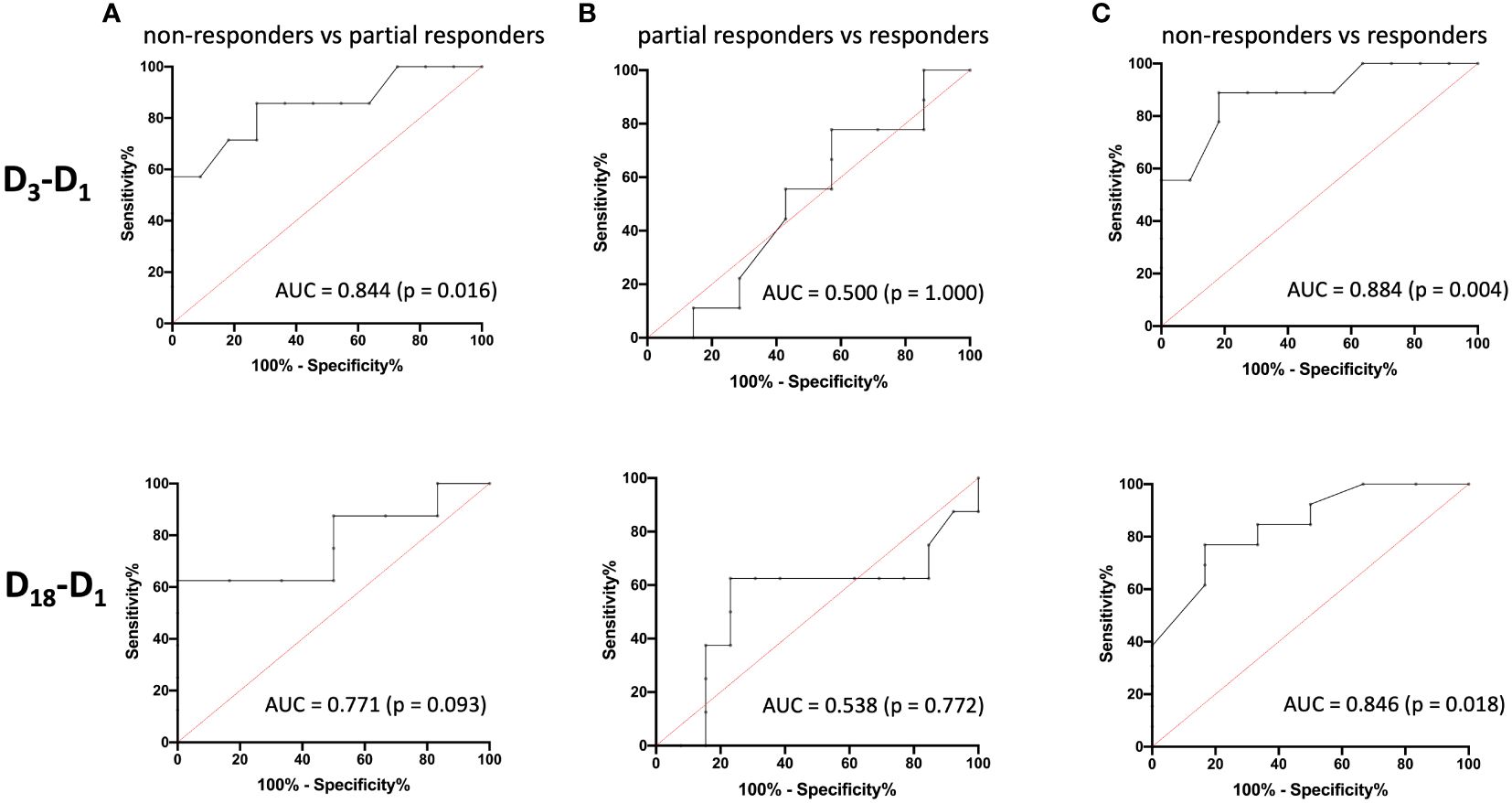
Figure 3 Receiver operating characteristic (ROC) curves and area under the ROC curve (AUC) values for the prediction of treatment response. The ROC curves illustrate the ability of logAMC to discriminate between non-responders and partial responders (A), partial responders and responders (B), and non-responders and responders (C) for D3 (top) and D18 (bottom), respectively. logAMC, log-transformed absolute monocyte count.
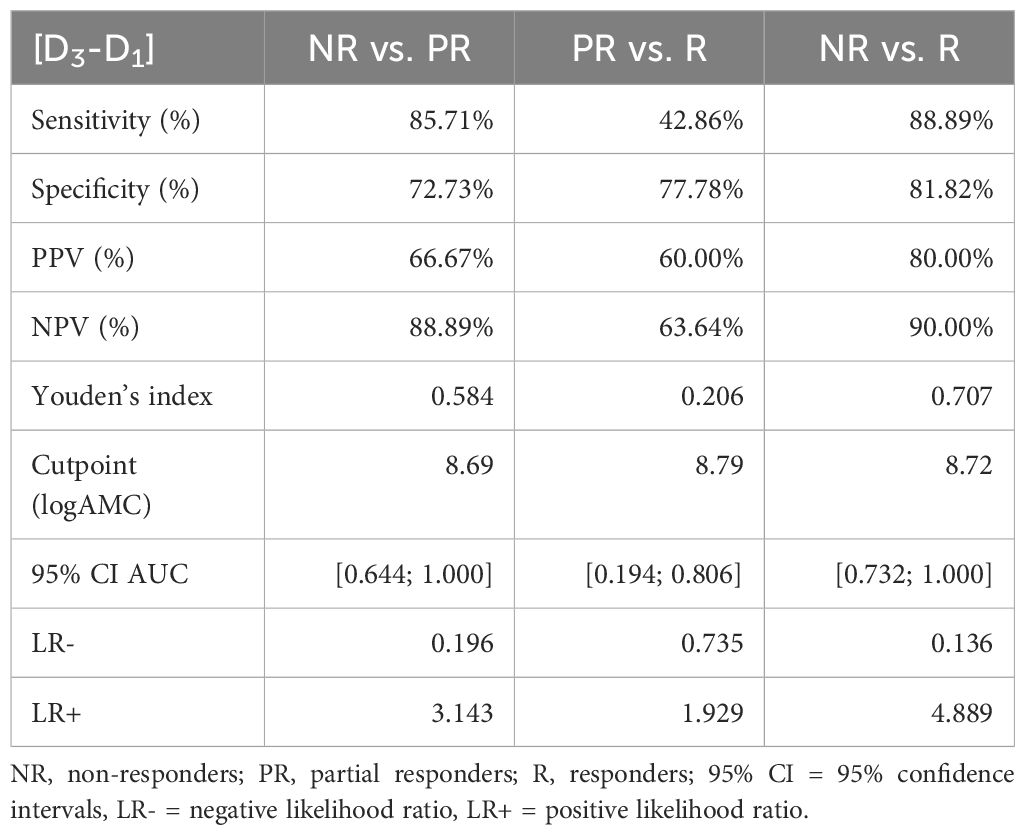
Table 5 Sensitivity, specificity, positive predictive value (PPV), negative predictive value (NPV), and likelihood ratios of the MADRS changes D3-D1 and logAMC.
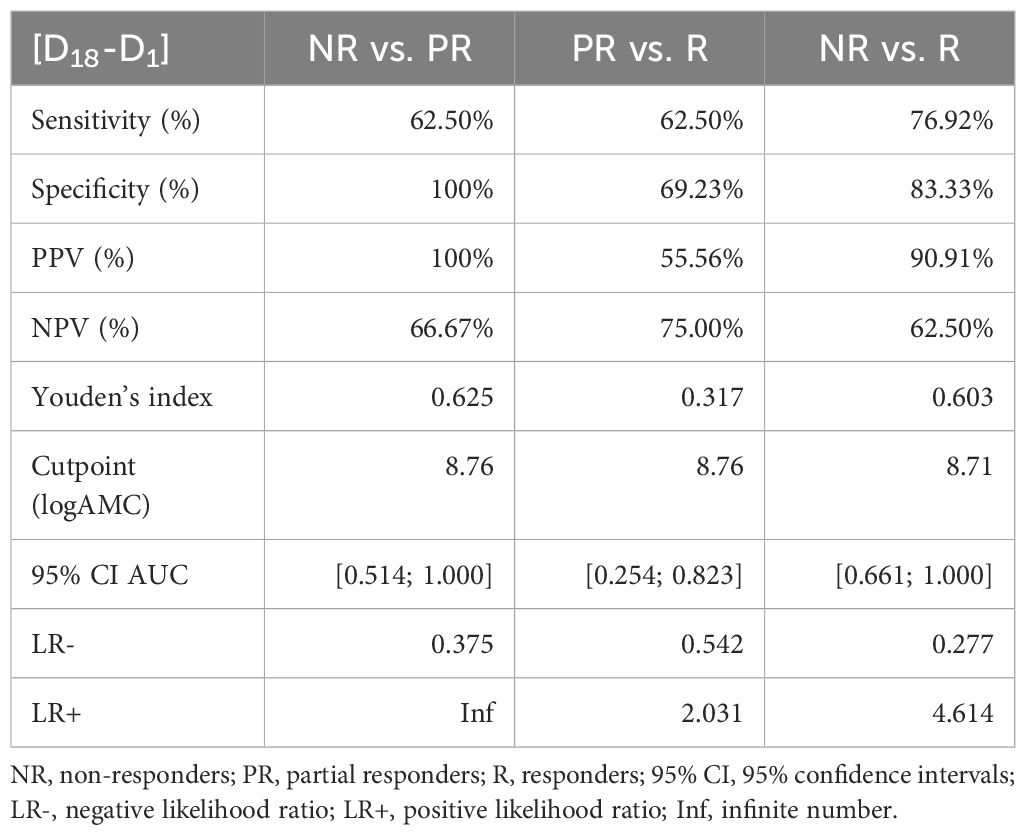
Table 6 Sensitivity, specificity, positive predictive value (PPV), negative predictive value (NPV), and likelihood ratios of the MADRS changes D18-D1 and logAMC.
Discussion
Our prospective single-arm open-label observational study investigated whether baseline inflammatory cellular markers or CRP can predict symptom improvement during intravenous ketamine treatment in TRD patients. Most importantly, baseline absolute monocyte count (AMC) was strongly associated with the improvement of depressive symptoms in the MADRS. This effect was already significant 24 hours after the first treatment, suggesting a link to the fast-acting antidepressant properties of ketamine, and remained stable over the three-week treatment period. Further, this association was robust when accounting for other factors, such as age, sex, or BMI, but could not be observed for CRP and only on a trend level for absolute neutrophil count (ANC).
Although our study was not primarily aimed at investigating the treatment effect of intravenous ketamine treatment in TRD patients, we could replicate its well-described antidepressant efficacy (17, 20) as well as its fast-acting properties (46). Specifically, we observed a response to intravenous ketamine treatment after 18 days in approximately 48% of the patients, together with a significant improvement of the MADRS scores already after the first ketamine session. Both numbers are well in line with data from the literature, describing that approximately 50% of TRD patients respond to ketamine treatment (20), with most treatment effects being observed in early stages (47). Thus, despite its relatively small sample size, our study appears to be reasonably representative. In addition, approximately 29% of the patients demonstrated a partial response, as indicated by a MADRS improvement between 25% and 50% at D18.
Importantly, AMC demonstrated the most significant association to the therapeutic outcome of all three inflammatory markers. The correlation coefficients after the first (r = -0.57) and before the last (r = -0.48) ketamine infusion indicate a moderate effect (48), which was stable even when additional factors (age, gender, BMI) were taken into account in the GLM. In summary, TRD patients with a high monocyte count showed a more pronounced improvement of depressive symptoms in the MADRS score during ketamine treatment, while patients with a low AMC showed weaker responses. Baseline AMC could therefore serve as a simple and easily accessible marker for predicting improvement of depressive symptoms during ketamine treatment in clinical practice. Of note, the predictive performance of AMC was particularly effective in distinguishing responders – and to a less strong extent partial responders – from non-responders, but lacked the ability to discriminate between partial responders and responders. Therefore, in everyday daily clinical practice, baseline AMC appear to be more appropriate for determining whether a patient is likely to benefit from ketamine treatment in general, rather than for measuring the extent of the treatment response.
A broad meta-analysis (44 studies (49) suggests that high inflammatory status is associated with non-response to classical antidepressants and thus a hallmark of TRD patients. While most studies have focused on cytokines, some indicate that TRD also leads to changes in immune cells such as monocytes and other macrophages, which are partly responsible for the production of cytokines such as IL-6. Notably, the gene expression of monocyte chemoattractant protein-1 (MCP-1) was significantly increased in white matter samples from depressed suicide completers (50). Kiraly et al. (11) hypothesized that patients under chronic stress experience an increase in chemotactic factors that attracted monocytes to the brain and led to increased mobilization of monocytes from the bone marrow. Specifically, the non-classical fraction of monocytes is proposed to be elevated in chronic inflammatory and autoimmune conditions (51–53). Indeed, patients with MDD showed high levels of the pro-inflammatory cytokines IL-12 and IL-6, increased numbers of non-classical monocytes, and increased activation of classical monocytes in the periphery (54). Further, two studies have demonstrated that pro-inflammatory compounds are associated with an M1-like pro-inflammatory state of monocytes/macrophages (55), and that the presence of ‘inflammatory’ monocytes are correlated with a poor response to antidepressant therapy with serotonergic reuptake inhibitors (56), total sleep deprivation or light therapy (55).
More importantly for our study, Nowak et al. (54) found that a subanesthetic dose of ketamine can significantly reduce the percentage of circulating pro-inflammatory monocytes in mice. Interestingly, the same study showed that subanesthetic ketamine specifically promotes the conversion of monocytes into M2c-like macrophages, thus reducing circulating classical pro-inflammatory monocytes and increasing alternative M2 macrophage subtypes (54). Circulating monocytes and monocytes that traffic to the brain showed increased expression of matrix metalloproteinase 8 (MMP8) both in patients with depression and in chronic stress models in mice (57). These monocytes specifically infiltrated the extracellular space in the CNS and thereby impaired brain function (57). Potentially, via this pathway, ketamine’s effect on monocytes might exert its neuromodulatory properties (Supplementary Figure S5).
Branchi et al. (58) proposed that anti-inflammatory treatment might exert a positive effect specifically in people with depression and high baseline inflammatory levels, increasing the efficacy of the treatment on depressive symptoms as well as normalizing immune activation. Ketamine treatment had an immunomodulatory effect via the stimulation of mTOR-associated gene expression receptors, as well as via the programing of human monocytes into M2c-like anti-inflammatory macrophages by inducing high levels of cluster of differentiation 163 (CD163) and Mer tyrosine kinase (MERTK) (54). Such immunomodulatory mechanisms of ketamine potentially explain its positive effect on depressive symptoms especially in patients with higher baseline inflammatory activity and thereby provide a potential neuronal background for the predictive value of the baseline AMC observed in our data.
While we observed a very prominent association between treatment response and baseline AMC, other effects appeared to be more subtle. Only at D3, baseline ANC weakly correlated to ketamine treatment response and showed a significant effect in the GLM. Notably, the sample size calculation for our study was designed to detect relatively strong associations, resulting in insufficient power to identify weaker correlations. Indeed, post-hoc power analyses (Supplementary Table S16) confirmed adequate power for logAMC but indicated insufficient power for detecting effects with logANC and logCRP. Further, the more subtle association of baseline ANC and symptom improvement could possibly be explained by the diverse functions of monocytes and neutrophils during inflammation (59, 60). Chronic inflammatory processes, which are mainly discussed in TRD, usually have a stronger effect on monocyte levels than on neutrophil levels, while the latter tend to reflect predominantly acute inflammatory reactions (61). A more detailed breakdown of the ANC in future studies could help to clarify the specific role of neutrophil subtypes in depression and their potential benefits as a biomarker for ketamine treatment. In line with previous studies, peripheral baseline CRP did not correlate with treatment response (30).
In contrast to the prominent association between AMC and treatment response measured with the MADRS score, the association with changes in the BDI-II scores was not significant. The same applies to ANC. Interestingly, BDI-II scores often change with a temporal delay in comparison to MADRS scores during ketamine treatment (62), suggesting that TRD patients need more time to assess subjective improvement in at least some psychopathological dimensions. This is consistent with our data, in which correlations between BDI-II scores at D18 showed a stronger association with AMC compared to D3 (r = -0.30 vs. r = -0.17). It is possible that the power of our study was insufficient to unravel such more subtle association.
Limitations
In general, the small sample size of the study limits the generalizability of the results. However, it is worth noting that the effect size of the current sample, calculated using partial eta squared, and the correlation coefficients suggest a medium-sized to large effect. Our study is further limited by the lack of longitudinal laboratory measurements of inflammation markers during the three-week long treatment period. Thus, we cannot estimate whether the antidepressant effects of ketamine treatment also correlate with a modulation of monocytes or other inflammatory markers during the treatment. This aspect would be crucial to disentangle the immunomodulatory effect of ketamine over time and could provide additional neuromechanistic insights on its action. Discrimination between pro- and anti-inflammatory monocytes has not been performed, and additional inflammatory markers related to monocyte or neutrophil activity, such as the cytokines IL-6 and TNF-α, were neither measured during the ketamine infusion nor at baseline. Finally, no follow-up was performed to evaluate whether the described effects also correlate with a long-lasting antidepressant response.
Conclusions
Our study proposes baseline AMC as a reliable predictor for response to intravenous ketamine treatment in TRD patients. Its simple accessibility as a routinely examined laboratory marker facilitates seamless integration into daily clinical practices and makes it particularly attractive. In this context, there is an opportunity not only to personalize ketamine as a treatment option, but also to improve the treatment of patients with TRD in general. Nevertheless, further studies are needed to replicate the predictive value of AMC in larger samples and to unravel the neuronal mechanisms underlying the relationship between neuroinflammatory markers in TRD and response to ketamine longitudinally.
Data availability statement
The datasets presented in this article are not readily available because the datasets generated and analyzed in this study are currently not publicly available but are available from the corresponding author on reasonable request. Requests to access the datasets should be directed to bruno.pedraz@zi-mannheim.de.
Ethics statement
This study involving humans was approved by the ethics committee of the Medical Faculty Mannheim at Heidelberg University (Registration number: 2021-902). The study was conducted in accordance with the local legislation and institutional requirements. The participants provided their written informed consent to participate in this study.
Author contributions
BPP: Conceptualization, Data curation, Formal analysis, Investigation, Methodology, Software, Validation, Visualization, Writing – original draft, Writing – review & editing. MS: Conceptualization, Data curation, Investigation, Methodology, Software, Validation, Visualization, Writing – original draft, Writing – review & editing. AD: Data curation, Investigation, Writing – review & editing. LD: Data curation, Investigation, Writing – review & editing. JD: Data curation, Investigation, Writing – review & editing. AYSC: Data curation, Investigation, Writing – review & editing. PW: Data curation, Investigation, Writing – review & editing. ER: Data curation, Investigation, Writing – review & editing. GG: Investigation, Project administration, Resources, Supervision, Writing – review & editing. MG: Conceptualization, Data curation, Investigation, Methodology, Supervision, Writing – review & editing. AS: Conceptualization, Data curation, Funding acquisition, Investigation, Methodology, Project administration, Resources, Supervision, Writing – review & editing. JRR: Conceptualization, Data curation, Formal analysis, Investigation, Methodology, resources, Software, Supervision, Validation, Visualization, Writing – original draft, Writing – review & editing.
Funding
The author(s) declare financial support was received for the research, authorship, and/or publication of this article. This work was supported by the German Federal Ministry of Education and Research (BMBF 01EW2010B NeuroMarKet) in the frame of EU ERA-Net NEURON to JRR and AS.
Conflict of interest
Gerhard Gründer has served as a consultant for Boehringer Ingelheim, Institute for Quality and Efficiency in Health Care (IQWiG), Janssen-Cilag, Lundbeck, MindMed, Otsuka, Recordati, Roche, ROVI, Sage, and Takeda. He has served on the speakers’ bureau of Gedeon Richter, Janssen Cilag, Lundbeck, Otsuka, Recordati, and ROVI. He has received grant support from Beckley Psytech and Boehringer Ingelheim. He is co-founder and/or shareholder of Mind and Brain Institute GmbH, OVID Health Systems GmbH and MIND Foundation gGmbH. Jonathan Reinwald has received speaker fees from Egetis Therapeutics. Moritz Spangemacher has received speaker fees from MIND Foundation gGmbH.
The remaining authors declare that the research was conducted in the absence of any commercial or financial relationships that could be construed as potential conflict of interest.
Publisher’s note
All claims expressed in this article are solely those of the authors and do not necessarily represent those of their affiliated organizations, or those of the publisher, the editors and the reviewers. Any product that may be evaluated in this article, or claim that may be made by its manufacturer, is not guaranteed or endorsed by the publisher.
Supplementary material
The Supplementary Material for this article can be found online at: https://www.frontiersin.org/articles/10.3389/fpsyt.2024.1415505/full#supplementary-material
References
1. World Health Organization. Depression and other common mental disorders: global health estimates (No. WHO/MSD/MER/2017.2). Geneva: World Health Organization (2017).
2. McIntyre RS, Alsuwaidan M, Baune BT, Berk M, Demyttenaere K, Goldberg JF, et al. Treatment-resistant depression: definition, prevalence, detection, management, and investigational interventions. World Psychiatry Off J World Psychiatr Assoc WPA. (2023) 22:394–412. doi: 10.1002/wps.21120
3. Sforzini L. Lost in translation. The quest for definitions of treatment-resistant depression with a focus on inflammation-related gene expression. Brain Behav Immun - Health. (2021) 16:100331. doi: 10.1016/j.bbih.2021.100331
4. Rush AJ, Trivedi MH, Wisniewski SR, Nierenberg AA, Stewart JW, Warden D, et al. Acute and longer-term outcomes in depressed outpatients requiring one or several treatment steps: A STAR*D report. Am J Psychiatry. (2006) 163:1905–17. doi: 10.1176/appi.ajp.163.11.1905
5. Rush AJ, Kraemer HC, Sackeim HA, Fava M, Trivedi MH, Frank E, et al. Report by the ACNP task force on response and remission in major depressive disorder. Neuropsychopharmacology. (2006) 31:1841–53. doi: 10.1038/sj.npp.1301131
6. Pigott HE, Kim T, Xu C, Kirsch I, Amsterdam J. What are the treatment remission, response and extent of improvement rates after up to four trials of antidepressant therapies in real-world depressed patients? A reanalysis of the STAR*D study’s patient-level data with fidelity to the original research protocol. BMJ Open. (2023) 13:e063095. doi: 10.1136/bmjopen-2022–063095
7. Sakurai H, Noma H, Watanabe K, Uchida H, Furukawa TA. Cumulative remission rate after sequential treatments in depression: reappraisal of the STAR*D trial data. World Psychiatry. (2024) 23:156–7. doi: 10.1002/wps.21169
8. Runia N, Yücel DE, Lok A, de Jong K, Denys DAJP, van Wingen GA, et al. The neurobiology of treatment-resistant depression: A systematic review of neuroimaging studies. Neurosci Biobehav Rev. (2022) 132:433–48. doi: 10.1016/j.neubiorev.2021.12.008
9. Yang C, Wardenaar KJ, Bosker FJ, Li J, Schoevers RA. Inflammatory markers and treatment outcome in treatment resistant depression: A systematic review. J Affect Disord. (2019) 257:640–9. doi: 10.1016/j.jad.2019.07.045
10. Strawbridge R, Arnone D, Danese A, Papadopoulos A, Herane Vives A, Cleare AJ. Inflammation and clinical response to treatment in depression: A meta-analysis. Eur Neuropsychopharmacol. (2015) 25:1532–43. doi: 10.1016/j.euroneuro.2015.06.007
11. Kiraly DD, Horn SR, Van Dam NT, Costi S, Schwartz J, Kim-Schulze S, et al. Altered peripheral immune profiles in treatment-resistant depression: response to ketamine and prediction of treatment outcome. Transl Psychiatry. (2017) 7:e1065–5. doi: 10.1038/tp.2017.31
12. Foley ÉM, Parkinson JT, Mitchell RE, Turner L, Khandaker GM, et al. Peripheral blood cellular immunophenotype in depression: a systematic review and meta-analysis. Mol Psychiatry. (2023) 28:1004–19. doi: 10.1038/s41380–022-01919–7
13. Strawbridge R, Izurieta E, Day E, Tee H, Young K, Tong CC, et al. Peripheral inflammatory effects of different interventions for treatment-resistant depression: A systematic review. Neurosci Appl. (2023) 2:101014. doi: 10.1016/j.nsa.2022.101014
14. Bennabi D, Haffen E. Immunomodulation of resistant depression. In: Berk M, Leboyer M, Sommer IE, editors. Immuno-psychiatry: facts and prospects. Springer International Publishing, Cham (2021). p. 389–400.
15. Almutabagani LF, Almanqour RA, Alsabhan JF, Alhossan AM, Alamin MA, Alrajeh HM, et al. Inflammation and treatment-resistant depression from clinical to animal study: A possible link? Neurol Int. (2023) 15:100–20. doi: 10.3390/neurolint15010009
16. Anand A, Mathew SJ, Sanacora G, Murrough JW, Goes FS, Altinay M, et al. Ketamine versus ECT for nonpsychotic treatment-resistant major depression. N Engl J Med. (2023) 388:2315–25. doi: 10.1056/NEJMoa2302399
17. Alnefeesi Y, Chen-Li D, Krane E, Jawad MY, Rodrigues NB, Ceban F, et al. Real-world effectiveness of ketamine in treatment-resistant depression: A systematic review & meta-analysis. J Psychiatr Res. (2022) 151:693–709. doi: 10.1016/j.jpsychires.2022.04.037
18. Loo C, Glozier N, Barton D, Baune BT, Mills NT, Fitzgerald P, et al. Efficacy and safety of a 4-week course of repeated subcutaneous ketamine injections for treatment-resistant depression (KADS study): randomised double-blind active-controlled trial. Br J Psychiatry J Ment Sci. (2023) 223:533–41. doi: 10.1192/bjp.2023.79
19. Reif A, Bitter I, Buyze J, Cebulla K, Frey R, Fu D-J, et al. Esketamine nasal spray versus quetiapine for treatment-resistant depression. N Engl J Med. (2023) 389:1298–309. doi: 10.1056/NEJMoa2304145
20. Schwartz J, Murrough JW, Iosifescu DV. Ketamine for treatment-resistant depression: recent developments and clinical applications. Evid Based Ment Health. (2016) 19:35–8. doi: 10.1136/eb-2016–102355
21. Ekstrand J, Fattah C, Persson M, Cheng T, Nordanskog P, Åkeson J, et al. Racemic ketamine as an alternative to electroconvulsive therapy for unipolar depression: A randomized, open-label, non-inferiority trial (KetECT). Int J Neuropsychopharmacol. (2022) 25:339–49. doi: 10.1093/ijnp/pyab088
22. Cui W, Ning Y, Hong W, Wang J, Liu Z, Li MD. Crosstalk between inflammation and glutamate system in depression: signaling pathway and molecular biomarkers for ketamine’s antidepressant effect. Mol Neurobiol. (2019) 56:3484–500. doi: 10.1007/s12035–018-1306–3
23. Zanos P, Moaddel R, Morris PJ, Riggs LM, Highland JN, Georgiou P, et al. Ketamine and ketamine metabolite pharmacology: insights into therapeutic mechanisms. Pharmacol Rev. (2018) 70:621–60. doi: 10.1124/pr.117.015198
24. Kopelman J, Keller TA, Panny B, Griffo A, Degutis M, Spotts C, et al. Rapid neuroplasticity changes and response to intravenous ketamine: a randomized controlled trial in treatment-resistant depression. Transl Psychiatry. (2023) 13:159. doi: 10.1038/s41398–023-02451–0
25. Calabrese F, Rossetti AC, Racagni G, Gass P, Riva MA, Molteni R. Brain-derived neurotrophic factor: a bridge between inflammation and neuroplasticity. Front Cell Neurosci. (2014) 8. doi: 10.3389/fncel.2014.00430
26. Wilkowska A, Szałach ŁP, Cubała WJ. Gut microbiota in depression: A focus on ketamine. Front Behav Neurosci. (2021) 15:693362. doi: 10.3389/fnbeh.2021.693362
27. Maes M, Vandoolaeghe E, Van Hunsel F, Bril T, Demedts P, Wauters A, et al. Immune disturbances in treatment-resistant depression: modulation by antidepressive treatments. Hum Psychopharmacol Clin Exp. (1997) 12:153–62. doi: 10.1002/(ISSN)1099-1077
28. Yang J, Wang N, Yang C, Shi J, Yu H, Hashimoto K. Serum interleukin-6 is a predictive biomarker for ketamine’s antidepressant effect in treatment-resistant patients with major depression. Biol Psychiatry. (2015) 77:e19–20. doi: 10.1016/j.biopsych.2014.06.021
29. Park M, Newman LE, Gold PW, Luckenbaugh DA, Yuan P, Machado-Vieira R, et al. Change in cytokine levels is not associated with rapid antidepressant response to ketamine in treatment-resistant depression. J Psychiatr Res. (2017) 84:113–8. doi: 10.1016/j.jpsychires.2016.09.025
30. Mkrtchian A, Evans JW, Kraus C, Yuan P, Kadriu B, Nugent AC, et al. Ketamine modulates fronto-striatal circuitry in depressed and healthy individuals. Mol Psychiatry. (2021) 26:3292–301. doi: 10.1038/s41380–020-00878–1
31. Vexler A, Tao G, Chen X. A toolkit for clinical statisticians to fix problems based on biomarker measurements subject to instrumental limitations: from repeated measurement techniques to a hybrid pooled-unpooled design. Methods Mol Biol Clifton NJ. (2015) 1208:439–60. doi: 10.1007/978–1-4939–1441-8_31
32. Schmidtke A, Fleckenstein P, Moises W, Beckmann H. [Studies of the reliability and validity of the German version of the Montgomery-Asberg Depression Rating Scale (MADRS)]. Schweiz Arch Neurol Psychiatr Zurich Switz 1985. (1988) 139:51–65.
33. Riedel M, Möller H-J, Obermeier M, Schennach-Wolff R, Bauer M, Adli M, et al. Response and remission criteria in major depression – A validation of current practice. J Psychiatr Res. (2010) 44:1063–8. doi: 10.1016/j.jpsychires.2010.03.006
34. Montgomery SA, Åsberg M. A new depression scale designed to be sensitive to change. Br J Psychiatry. (1979) 134:382–9. doi: 10.1192/bjp.134.4.382
35. Herrmann N, Black SE, Lawrence J, Szekely C, Szalai J. The sunnybrook stroke study. Stroke. (1998) 29:618–24. doi: 10.1161/01.STR.29.3.618
36. Trivedi MH, Corey-Lisle PK, Guo Z, Lennox RD, Pikalov A, Kim E. Remission, response without remission, and nonresponse in major depressive disorder: impact on functioning. Int Clin Psychopharmacol. (2009) 24:133–8. doi: 10.1097/YIC.0b013e3283277614
37. Hawley CJ, Gale TM, Sivakumaran T, Hertfordshire Neuroscience Research group. Defining remission by cut off score on the MADRS: selecting the optimal value. J Affect Disord. (2002) 72:177–84. doi: 10.1016/s0165–0327(01)00451–7
38. Keller F, Hautzinger M, Kühner C. Zur faktoriellen Struktur des deutschsprachigen BDI-II. Z Für Klin Psychol Psychother. (2008) 37:245–54. doi: 10.1026/1616–3443.37.4.245
39. jamovi - open statistical software for the desktop and cloud. Available at: https://www.jamovi.org/ (Accessed 29 Oct 2022).
40. jamovi library. Available at: https://www.jamovi.org/library.html (Accessed 10 Apr 2024).
41. Richardson JTE. Eta squared and partial eta squared as measures of effect size in educational research. Educ Res Rev. (2011) 6:135–47. doi: 10.1016/j.edurev.2010.12.001
42. Morris PE, Fritz CO. Effect sizes in memory research. Mem Hove Engl. (2013) 21:832–42. doi: 10.1080/09658211.2013.763984
43. Faul F, Erdfelder E, Lang A-G, Buchner A. G*Power 3: A flexible statistical power analysis program for the social, behavioral, and biomedical sciences. Behav Res Methods. (2007) 39:175–91. doi: 10.3758/BF03193146
44. Friesen L, Kroc E, Zumbo B. Psychometrics & Post-data analysis: test ROC [jamovi module]. (2019).
45. Ruopp MD, Perkins NJ, Whitcomb BW, Schisterman EF. Youden index and optimal cut-point estimated from observations affected by a lower limit of detection. Biom J Biom Z. (2008) 50:419–30. doi: 10.1002/bimj.200710415
46. Zarate CA, Singh JB, Carlson PJ, Brutsche NE, Ameli R, Luckenbaugh DA, et al. A randomized trial of an N-methyl-D-aspartate antagonist in treatment-resistant major depression. Arch Gen Psychiatry. (2006) 63:856–64. doi: 10.1001/archpsyc.63.8.856
47. Wilkinson ST, Katz RB, Toprak M, Webler R, Ostroff RB, Sanacora G. Acute and longer-term outcomes using ketamine as a clinical treatment at the yale psychiatric hospital. J Clin Psychiatry. (2018) 79:17m11731. doi: 10.4088/JCP.17m11731
49. Liu JJ, Wei YB, Strawbridge R, Bao Y, Chang S, Shi L, et al. Peripheral cytokine levels and response to antidepressant treatment in depression: a systematic review and meta-analysis. Mol Psychiatry. (2020) 25:339–50. doi: 10.1038/s41380–019-0474–5
50. Torres-Platas SG, Cruceanu C, Chen GG, Turecki G, Mechawar N. Evidence for increased microglial priming and macrophage recruitment in the dorsal anterior cingulate white matter of depressed suicides. Brain Behav Immun. (2014) 42:50–9. doi: 10.1016/j.bbi.2014.05.007
51. Smiljanovic B, Radzikowska A, Kuca-Warnawin E, Kurowska W, Grün JR, Stuhlmüller B, et al. Monocyte alterations in rheumatoid arthritis are dominated by preterm release from bone marrow and prominent triggering in the joint. Ann Rheum Dis. (2018) 77:300–8. doi: 10.1136/annrheumdis-2017–211649
52. Teng MWL, Bowman EP, McElwee JJ, Smyth MJ, Casanova J-L, Cooper AM, et al. IL-12 and IL-23 cytokines: from discovery to targeted therapies for immune-mediated inflammatory diseases. Nat Med. (2015) 21:719–29. doi: 10.1038/nm.3895
53. Chuluundorj D, Harding SA, Abernethy D, La Flamme AC. Expansion and preferential activation of the CD14(+)CD16(+) monocyte subset during multiple sclerosis. Immunol Cell Biol. (2014) 92:509–17. doi: 10.1038/icb.2014.15
54. Nowak W, Grendas LN, Sanmarco LM, Estecho IG, Arena ÁR, Eberhardt N, et al. Pro-inflammatory monocyte profile in patients with major depressive disorder and suicide behaviour and how ketamine induces anti-inflammatory M2 macrophages by NMDAR and mTOR. EBioMedicine. (2019) 50:290–305. doi: 10.1016/j.ebiom.2019.10.063
55. Benedetti F, Poletti S, Hoogenboezem TA, Locatelli C, Wit H, Wijkhuijs AJM, et al. Higher baseline proinflammatory cytokines mark poor antidepressant response in bipolar disorder. J Clin Psychiatry. (2017) 78:4015. doi: 10.4088/JCP.16m11310
56. Arteaga-Henríquez G, Simon MS, Burger B, Weidinger E, Wijkhuijs A, Arolt V, et al. Low-grade inflammation as a predictor of antidepressant and anti-inflammatory therapy response in MDD patients: A systematic review of the literature in combination with an analysis of experimental data collected in the EU-MOODINFLAME consortium. Front Psychiatry. (2019) 10:458. doi: 10.3389/fpsyt.2019.00458
57. Cathomas F, Lin H-Y, Chan KL, Li L, Parise LF, Alvarez J, et al. Circulating myeloid-derived MMP8 in stress susceptibility and depression. Nature. (2024) 626:1108–15. doi: 10.1038/s41586–023-07015–2
58. Branchi I, Viglione A, Vai B, Cirulli F, Benedetti F, Poggini S. Breaking free from the inflammatory trap of depression: Regulating the interplay between immune activation and plasticity to foster mental health. Neurosci Appl. (2024) 3:103923. doi: 10.1016/j.nsa.2023.103923
59. Austermann J, Roth J, Barczyk-Kahlert K. The good and the bad: monocytes’ and macrophages’ Diverse functions in inflammation. Cells. (2022) 11:1979. doi: 10.3390/cells11121979
60. Fujiwara N, Kobayashi K. Macrophages in inflammation. Curr Drug Targets Inflammation Allergy. (2005) 4:281–6. doi: 10.2174/1568010054022024
61. Ley K, Laudanna C, Cybulsky MI, Nourshargh S. Getting to the site of inflammation: the leukocyte adhesion cascade updated. Nat Rev Immunol. (2007) 7:678–89. doi: 10.1038/nri2156
Keywords: ketamine, prediction, treatment response, treatment-resistant depression, inflammation, monocyte, neutrophil, immunity
Citation: Pedraz-Petrozzi B, Spangemacher M, Deicher A, Drews L, Defert J, Silva-Colmenero AY, Wein P, Riedinger E, Gründer G, Gilles M, Sartorius A and Reinwald JR (2024) Baseline monocyte count predicts symptom improvement during intravenous ketamine therapy in treatment-resistant depression: a single-arm open-label observational study. Front. Psychiatry 15:1415505. doi: 10.3389/fpsyt.2024.1415505
Received: 10 April 2024; Accepted: 05 June 2024;
Published: 24 June 2024.
Edited by:
Maria Grazia Morgese, University of Foggia, ItalyReviewed by:
Federico Manuel Daray, University of Buenos Aires, ArgentinaAlina Wilkowska, Medical University of Gdansk, Poland
Copyright © 2024 Pedraz-Petrozzi, Spangemacher, Deicher, Drews, Defert, Silva-Colmenero, Wein, Riedinger, Gründer, Gilles, Sartorius and Reinwald. This is an open-access article distributed under the terms of the Creative Commons Attribution License (CC BY). The use, distribution or reproduction in other forums is permitted, provided the original author(s) and the copyright owner(s) are credited and that the original publication in this journal is cited, in accordance with accepted academic practice. No use, distribution or reproduction is permitted which does not comply with these terms.
*Correspondence: Bruno Pedraz-Petrozzi, bruno.pedraz@zi-mannheim.de
†These authors share first authorship
‡ORCID: Bruno Pedraz-Petrozzi, orcid.org/0000-0002-4119-971X
Moritz Spangemacher, orcid.org/0009-0007-1268-4808
Gerhard Gründer, orcid.org/0000-0001-7868-3903
Maria Gilles, orcid.org/0000-0002-9604-4459
Alexander Sartorius, orcid.org/0000-0002-1243-3693
Jonathan R. Reinwald, orcid.org/0000-0001-9508-3942