- 1Center for External Treatment of Traditional Chinese Medicine, Affiliated Hospital of Shandong University of Traditional Chinese Medicine, Jinan, Shandong, China
- 2College of Acupuncture and Massage, Shandong University of Traditional Chinese Medicine, Jinan, Shandong, China
- 3College of First Clinical Medicine, Shandong University of Traditional Chinese Medicine, Jinan, China
- 4Department of Neurology, Affiliated Hospital of Shandong University of Traditional Chinese Medicine, Jinan, Shandong, China
Background and objectives: Major Depressive Disorder (MDD) is one of the most prevalent and debilitating health conditions worldwide. Previous studies have reported a link between metabolic dysregulation and MDD. However, evidence for a causal relationship between blood metabolites and MDD is lacking.
Methods: Using a two-sample bidirectional Mendelian randomization analysis (MR), we assessed the causal relationship between 1,400 serum metabolites and Major Depressive Disorder (MDD). The Inverse Variance Weighted method (IVW) was employed to estimate the causal association between exposures and outcomes. Additionally, MR-Egger regression, weighted median, simple mode, and weighted mode methods were used as supplementary approaches for a comprehensive appraisal of the causality between blood metabolites and MDD. Pleiotropy and heterogeneity tests were also conducted. Lastly, the relevant metabolites were subjected to metabolite function analysis, and a reverse MR was implemented to explore the potential influence of MDD on these metabolites.
Results: After rigorous screening, we identified 34 known metabolites, 13 unknown metabolites, and 18 metabolite ratios associated with Major Depressive Disorder (MDD). Among all metabolites, 33 were found to have positive associations, and 32 had negative associations. The top five metabolites that increased the risk of MDD were the Arachidonate (20:4n6) to linoleate (18:2n6) ratio, LysoPE(18:0/0:0), N-acetyl-beta-alanine levels, Arachidonate (20:4n6) to oleate to vaccenate (18:1) ratio, Glutaminylglutamine, and Threonine to pyruvate ratio. Conversely, the top five metabolites that decreased the risk of MDD were N6-Acetyl-L-lysine, Oleoyl-linoleoyl-glycerol (18:1 to 18:2) [2] to linoleoyl-arachidonoyl-glycerol (18:2 to 20:4) [2] ratio, Methionine to phosphate ratio, Pregnanediol 3-O-glucuronide, and 6-Oxopiperidine-2-carboxylic acid. Metabolite function enrichment was primarily concentrated in pathways such as Bile Acid Biosynthesis (FDR=0.177), Glutathione Metabolism (FDR=0.177), Threonine, and 2-Oxobutanoate Degradation (FDR=0.177). In addition, enrichment was noted in pathways like Valine, Leucine, and Isoleucine Biosynthesis (p=0.04), as well as Ascorbate and Aldarate Metabolism (p=0.04).
Discussion: Within a pool of 1,400 blood metabolites, we identified 34 known metabolites and 13 unknown metabolites, as well as 18 metabolite ratios associated with Major Depressive Disorder (MDD). Additionally, three functionally enriched groups and two metabolic pathways were selected. The integration of genomics and metabolomics has provided significant insights for the screening and prevention of MDD.
1 Introduction
Globally, Major Depressive Disorder (MDD) stands as one of the most common and incapacitating health concerns. In the past three decades, the worldwide incidence of MDD has surged by nearly 50%, impacting over 264 million individuals across various age groups (1). According to the World Health Organization, depression ranks as the foremost cause of mental and physical disability on a global scale and stands as a significant contributor to the burden of health issues faced worldwide (2). Regarding mental health challenges, Major Depressive Disorder (MDD) is regarded as one of the most critical conditions, necessitating immediate and proactive intervention (3).
Metabolites, which are the substrates and products of metabolism, not only drive essential cellular activities but also serve as functional intermediates that can predict or influence the onset and progression of diseases. In recent years, an increasing number of studies have been exploring the relationship between Major Depressive Disorder (MDD) and metabolomics, signaling a burgeoning interest in this area of research (4). These investigations have substantiated the effectiveness of metabolomics in unraveling the complex characteristics of depression, along with the molecular underpinnings associated with the clinical facets of Major Depressive Disorder (MDD). Significantly, metabolomics has illuminated the association between MDD and the gut microbiome and has further delineated the significance of lipids in the composition and operation of cellular membranes. The metabolic profile of Major Depressive Disorder (MDD) encompasses 124 metabolites, spanning across pathways of energy and lipid metabolism. The research identified 49 metabolites, including metabolites involved in the tricarboxylic acid (TCA) cycle, such as citrate and pyruvate. Notably, levels of citrate were significantly reduced, whereas pyruvate was markedly increased in patients with severe depression (5).
It is important to note that there is a paucity of cohort-based causality studies linking metabolites with Major Depressive Disorder (MDD). Understanding whether disparate abundance metabolites act as risk factors or protective agents for MDD would be meaningful for predicting the disease and aiding diagnosis through specific targeted approaches. Mendelian randomization (MR) analysis employs single nucleotide polymorphisms (SNPs), which occur randomly within human genes, as instrumental variables. This method is akin to the design of a randomized controlled trial (RCT), which enhances the randomization of the sample selection. By establishing a connection between exposure factors and outcome variables through instrumental variables, this method provides a more robust demonstration of the causal relationship between them. Moreover, since metabolic products can be either substances that influence the onset of a disease or substances that are produced as a result of the disease, bidirectional MR analysis can more effectively elucidate the causal direction between metabolic products and disease.
Therefore, this study collected a relatively complete set of serum metabolomics data and introduced a Mendelian Randomization (MR) analysis akin to the design of a randomized controlled trial (RCT). Through bidirectional MR validation, we elucidated the causal relationship between Major Depressive Disorder (MDD) and related metabolites. In this research, the MR approach utilized metabolite-associated SNPs as instrumental variables (IVs) to assess the causal effects of genetic proxy markers for metabolites and their ratios on MDD. Additionally, we evaluated integrated metabolic pathways to shed light on their biological mechanisms.
2 Methods
2.1 Study design
The dataset containing all the data from this study is made available to the public on the database website. Summarized statistical data from published genome-wide association studies (GWAS) have been included. Written informed consent for all subjects was obtained in separate studies, authorized by the Institutional Review Boards’ ethics committees. No additional ethical approval or informed consent is required.
We performed a two-sample Mendelian analysis using metabolites as exposure and MDD as outcome. Then the 65 metabolites selected were used as outcome, and MDD was used as exposure for reverse MR Analysis. Finally, the function analysis of the related metabolites was carried out.
In the current study, we conducted a comprehensive assessment of the relationship between Major Depressive Disorder (MDD) and metabolism based on a rigorous Mendelian Randomization (MR) design, evaluating a total of 1,091 metabolites and 309 metabolite ratios, encompassing 1,400 metabolic correlates. The clinical diagnosis of Major Depressive Disorder (MDD) relies on the use of the Patient Health Questionnaire-9 (PHQ-9). Kroenke et al (6) outlined the initial development of the PHQ-9 and proposed cutoff scores indicative of varying levels of depression severity, which were validated through the observation of disparities in health-related quality of life across adjacent severity categories. Consequently, the researchers employed 5-point intervals to delineate score ranges associated with varying levels of depression severity, with scores falling within the range of 20–27 indicative of severe depression (7).
Scientific MR studies must scrutinize three critical assumptions: A) The genetic instrumental variables must be strongly associated with the exposure of interest; B) The genetic instrumental variables should be independent of the outcome and not related to any known or unknown confounding factors; C) The effect of the instrumental variables on the outcome should be exclusively mediated through the exposure of interest. In summary, a bidirectional analytic strategy was employed to select genetic single nucleotide polymorphisms (SNPs) with significance to both 1,400 human serum metabolites and Major Depressive Disorder (MDD). To prevent sample overlap, the metabolite and MDD genetic information selected in this study was derived from separate GWAS datasets.The schematic illustration of this bidirectional MR study is shown in Figure 1.
2.2 Data sources
We conducted a series of large-scale Genome-Wide Association Studies (GWAS), which included metabolomic data of 1,091 metabolites and 309 metabolite ratios from a cohort of 8,299 individuals belonging to the Canadian Longitudinal Study on Aging (CLSA).
Chen et al. have obtained a comprehensive genome-wide association summary dataset for 1,091 metabolites and 309 metabolite ratios included in this study. This dataset is publicly accessible from the GWAS server (http://metabolomics.helmholtz-muenchen.de/gwas/). The service platform has gathered a relatively comprehensive set of human serum metabolomic data. The GWAS analysis included 1,091 metabolites and 309 metabolite ratios from 8,299 individuals, which are part of the Canadian Longitudinal Study on Aging (CLSA) cohort. Among the 1,091 plasma metabolites analyzed, 850 have known characteristics associated with eight super-pathways, namely lipids, amino acids, xenobiotics, nucleotides, cofactors and vitamins, carbohydrates, peptides, and energy. The remaining 241 were categorized as unknown or ‘partially’ characterized molecules. The current study features 96 metabolites that had not been tested in previous representative large-scale metabolomics GWASs. Detailed cohort characteristics and metabolite information can be found in Supplementary Table 1.
The summary data for Major Depressive Disorder come from the Integrated Epidemiology Unit (IEU) (https://gwas.mrcieu.ac.uk/) Open GWAS project. GWAS ID is ieu-b-102 (8). In this GWAS meta-analysis, the aggregate data comprise of 170,756 cases of Major Depressive Disorder (MDD) and 329,443 control subjects, yielding a total of 8,447,813 SNPs. We extracted the SNP information by analyzing the VCF format files shared through the platform.
2.3 Instrumental variable selection
In this MR (Mendelian Randomization) analysis, the selection of IVs (Instrumental Variables) was based on three core assumptions. Firstly, for each metabolite, we set a genome-wide significance threshold p < 1 × 10^−5 for the selection of strongly associated SNPs. Following the extraction of significant SNPs for each metabolite, we performed linkage disequilibrium analysis. Linkage disequilibrium was deemed present if the linkage disequilibrium coefficient (r^2) was less than 0.1 and the distance between SNPs fell within a 500-kilobase (kb) radius (9). Moreover, to mitigate the potential bias due to weak instrumentality, the F-statistic for each SNP was computed. SNPs exhibiting an F-statistic lower than 10 were deemed weak instruments and were subjected to further scrutiny (10).
2.4 Sensitivity analysis
The standard Inverse Variance Weighted (IVW) method (a random-effects model) (11) is the primary evaluative approach for investigating the causal relationship between metabolites and Major Depressive Disorder (MDD) in this analysis, encompassing both forward and reverse MR analyses. MR-Egger and the Weighted Median (WM) provide secondary methods of evaluation. When the instrumental variables satisfy all three core assumptions, the IVW method can estimate the causal effects of exposure with greater precision and is considered the most robust method for Mendelian Randomization. However, the analysis may yield inaccurate results if some of the instrumental variables do not adhere to the instrumental variable assumptions. Consequently, we conducted the following sensitivity analyses: 1) We performed Cochran’s Q test via the IVW and MR-Egger methods to detect potential violations of assumptions through heterogeneity in the correlations between individual IVs; 2) We implemented the MR-Egger intercept to estimate pleiotropy and ensure that genetic variations are independently associated with metabolites and MDD; 3) Additional analyses such as Weighted Median and Mode-based Estimation were used to enhance the reliability and stability of our hypothesis testing; 4) Individual SNP analyses and leave-one-out diagnostics were carried out to evaluate the likelihood of the observed associations for individual SNPs. MR analysis may breach causality assumptions under the premise of genetic correlation between exposure and study outcomes. While SNPs associated with MDD were excluded during IV selection, SNPs without known associations might also influence the incidence of MDD. Linkage Disequilibrium Score (LDSC) regression can assess pleiotropy by utilizing SNP-based chi-square statistics. Therefore, to ensure that the causal relationship is not confounded by coherence between exposure and outcome, we implemented LDSC to corroborate the genetic correlation between differential abundance of serum metabolites and MDD.
2.5 Statistical analysis
All MR analyses were carried out using the “TwoSampleMR” package within R (version 4.2.1). LDSC was conducted via the “ldsc” package, with a p-value of less than 0.05 considered to be statistically significant. Odds ratios (OR) were employed to estimate the magnitude and direction of the metabolic impact alongside their corresponding 95% confidence intervals (CIs).
2.6 Verification of genetic correlation and direction
Under the assumption of genetic correlation between exposures and study outcomes, MR analysis could violate the principles of causation. Although SNPs associated with MDD were excluded when selecting instrumental variables (IVs), non-associated SNPs might also influence the occurrence of MDD. Linkage disequilibrium score (LDSC) regression can calculate pleiotropy by invoking SNP-based chi-square statistics. Therefore, to ensure the causal inference is not confounded by the coherence between exposure and outcome, LDSC was employed to verify the genetic correlation between the differential abundance of serum metabolites and MDD.
2.7 Metabolic pathway analysis
Metabolic pathways were estimated using the web-based MetaboAnalyst 6.0 (https://www.Metaboanalyst.ca/). The Pathway Analysis and Enrichment Analysis modules were utilized to identify clusters of metabolites or super-pathways potentially associated with metabolic processes and their potential links to MDD. The human metabolome database (HMDB) and the Kyoto Encyclopedia of Genes and Genomes (KEGG) were used as reference databases. The significance level for pathways was set at 0.05.
3 Result
3.1 Step 1: The impact of 1400 serum metabolites on MDD
Due to the genome-wide significance threshold set at p < 1×10^-5 for selecting SNPs with strong associations, a total of 645 serum metabolites and metabolite ratios were chosen. All featured F-statistics values greater than 10, suggesting that a weak instrument bias is unlikely to be significant. All metabolic analyses employed the Inverse Variance Weighted (IVW) approach as the main method, with no evidence of heterogeneity or weak instruments. From the primary outcomes, 96 metabolites were significantly associated (with IVW p < 0.05). After screening for pleiotropy, 65 metabolites remained significantly associated (with IVW p < 0.05 and Pleiotropy p > 0.05), including 35 metabolites and 17 metabolite ratios.
Of the 13 metabolites, the chemical properties are unknown, while the remaining 35 identified metabolites fall into several categories including: Lipids, Carboxylic acids, Organooxygen compounds, amino acids, Keto acids, Naphthalenes, Purine nucleotides, Organic acids, Glycerophospholipids, Quinic acid, Fatty acids, Steroids, and Naphthalenes, among others. Notably, lipids are the most frequently occurring category, accounting for 60% of these identified substances. Within the group of 65 metabolites and metabolite ratios, 32 are positively associated with MDD, while 33 show a negative association with the condition. The IVW forest plot for the 65 significantly associated metabolites is presented in Figure 2. Alternate MR analyses, Q-tests, and sensitivity analysis results for the 65 known metabolites can be found in Table 1. All instrumental variables (IVs) passed the sensitivity tests (p > 0.05).
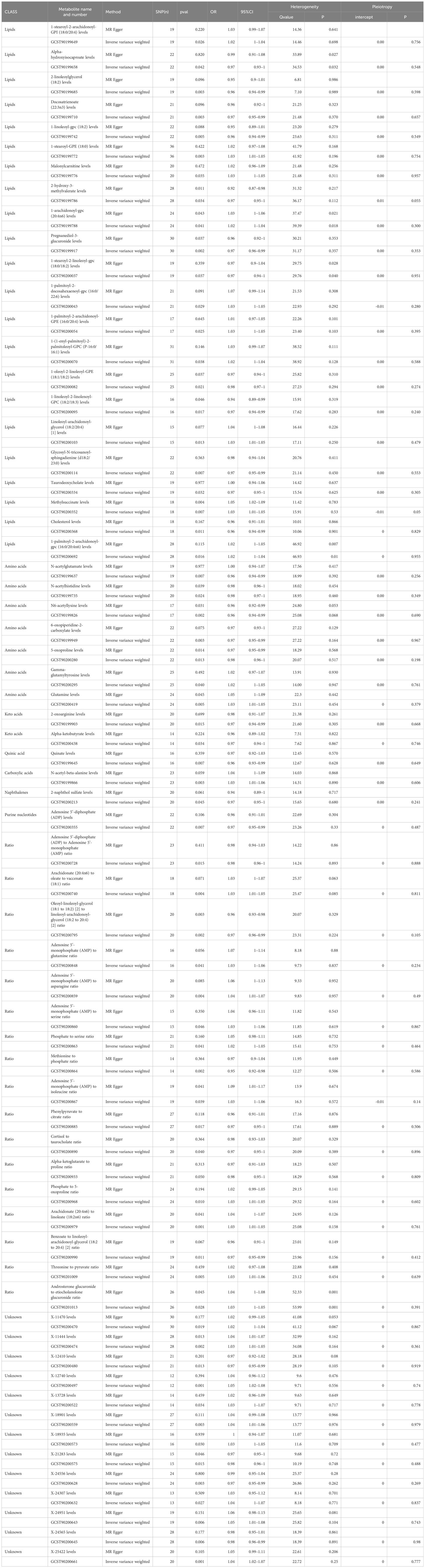
Table 1 Two MR Models estimated causality between 65 metabolites and their ratio and major depressive disorder, and tested for heterogeneity and level pleiotropy.
Among the 34 identified metabolites, we found that N6-acetyllysine levels have the most significant negative correlation with MDD (IVW OR = 0.96; 95% CI = 0.92–0.99; P = 0.0015), followed by Pregnanediol-3-glucuronide levels (IVW OR = 0.97; 95% CI = 0.96–0.99; P = 0.002); 6-oxopiperidine-2-carboxylate levels (IVW OR = 0.97; 95% CI = 0.95–0.99; P = 0.003); 2-linoleoylglycerol (18:2) levels (IVW OR = 0.96; 95% CI = 0.94–0.99; P = 0.003); Docosatrienoate (22:3n3) levels (IVW OR = 0.97; 95% CI = 0.95–0.99; P = 0.003); 1-linoleoyl-gpc (18:2) levels (IVW OR = 0.96; 95% CI = 0.94–0.99; P = 0.005); Quinate levels (IVW OR = 0.96; 95% CI = 0.93–0.99; P = 0.007); Adenosine 5’-diphosphate (ADP) levels (IVW OR = 0.97; 95% CI = 0.95–0.99; P = 0.007); and Taurodeoxycholate levels (IVW OR = 0.97; 95% CI = 0.95–1; P = 0.032).
The most significantly positive correlation with MDD was observed in the levels of 1-stearoyl-GPE (18:0) (IVW OR = 1.03; 95% CI = 1.01–1.05; P = 0.003); followed by N-acetyl-beta-alanine levels (IVW OR = 1.03; 95% CI = 1.01–1.06; P = 0.003); Glutamine levels (IVW OR = 1.03; 95% CI = 1.01–1.05; P = 0.005); Methylsuccinate levels (IVW OR = 1.03; 95% CI = 1.01–1.05; P = 0.007); Linoleoyl-arachidonoyl-glycerol (18:2/20:4) [1] levels (IVW OR = 1.03; 95% CI = 1.01–1.05; P = 0.013); and 1-palmitoyl-2-arachidonoyl-gpc (16:0/20:4n6) levels (IVW OR = 1.02; 95% CI = 1–1.04; P = 0.016).
In terms of metabolite ratios, the most significant positive correlation with MDD was observed in the ratio of Arachidonate (20:4n6) to Linoleate (18:2n6) (IVW OR = 1.03; 95% CI = 1.01–1.05; P = 0.001); the most significant negative correlation with MDD was found in the ratio of Oleoyl-linoleoyl-glycerol (18:1 to 18:2) [2] to Linoleoyl-arachidonoyl-glycerol (18:2 to 20:4) [2] (IVW OR = 0.97; 95% CI = 0.96–0.99; P = 0.002).
In summary, MR estimates from IVW, WM, and MR-Egger regression for 34 metabolites and 18 metabolite ratios showed consistent direction and magnitude, supporting robustness in the causal inference, except for Alpha-hydroxyisocaproate levels (IVW OR = 0.97; 95% CI = 0.93–1; P = 0.042; heterogeneity Q value = 34.53; P = 0.032); 1-arachidonoyl-gpc (20:4n6) levels (IVW OR = 1.02; 95% CI = 1–1.04; P = 0.041; heterogeneity Q value = 39.39; P = 0.018); 1-stearoyl-2-linoleoyl-gpc (18:0/18:2) levels (IVW OR = 0.97; 95% CI = 0.94–1; P = 0.037; heterogeneity Q value = 29.76; P = 0.040); 1-palmitoyl-2-arachidonoyl-gpc (16:0/20:4n6) levels (IVW OR = 1.02; 95% CI = 1–1.04; P = 0.016; heterogeneity Q value = 46.93; P = 0.01); and the Androsterone glucuronide to etiocholanolone glucuronide ratio (IVW OR = 1.03; 95% CI = 1–1.05; P = 0.028; heterogeneity Q value = 53.99; P = 0.001). Heterogeneity was not detected in the p-values of the Cochran Q test for the remaining metabolites and metabolite ratios (Table 1; Supplementary Tables 1, 2). The MR-Egger intercept did not indicate the presence of pleiotropy (Table 1; Supplementary Tables 1, 2). Furthermore, a Leave-One-Out (LOO) analysis did not identify any highly influential SNPs that could bias the combined effect estimates (Supplementary Table 3). Therefore, these 34 metabolites and 18 metabolite ratios have been identified as potential candidate metabolomic markers involved in the pathogenesis of major depressive disorder (MDD) and warrant further analysis, with particular focus on the levels of N6-acetyllysine and 1-stearoyl-GPE (18:0).
3.2 Step 2: The impact of MDD on the inverse MR Of 65 metabolites and metabolite ratios
Given a genome-wide significance threshold of p < 1 × 10^−5, fifty significant SNPs were extracted to serve as instrumental variables (IVs) for major depressive disorder (MDD) (Supplementary Table 4). The analysis utilized 65 serum metabolites and metabolite ratios as outcomes. Moreover, the F-statistics were all well above 10, suggesting that a bias from weak instruments is unlikely to be significant. The Inverse Variance Weighted (IVW) method was employed as the primary estimation approach for MDD. Of the principal findings, only one metabolite, X-12740 levels, was identified as significantly associated (IVW OR = 1.21; 95% CI = 1.00–1.47; P = 0.045; heterogeneity Q value = 27.79; P = 0.980); however, since this metabolite is unidentified, it lacks research significance. Consequently, it is evident that the influence of the 34 metabolites and 18 metabolite ratios on MDD is singular.
3.3 Step 3: Metabolic pathway analysis
The 34 metabolites significantly associated with MDD were inputted into the MetaboAnalyst 6.0 platform to identify various potential metabolic pathways involved in the pathogenesis and immunology of MDD. Among them, Bile Acid Biosynthesis (FDR = 0.177), Glutathione Metabolism (FDR = 0.177), and Threonine and 2-Oxobutanoate Degradation (FDR = 0.177) demonstrated notable functional enrichment (Figure 3). Additionally, the pathways of Valine, Leucine and Isoleucine Biosynthesis (p = 0.04), and Ascorbate and Aldarate Metabolism (p = 0.04) were even more significant (Table 2, Figure 4). The metabolic mechanisms formed by these metabolites may be related to the pathogenesis affected by MDD. Figure 3 illustrates the Enrichment Overview(top 25). Table 2 and Figure 4 show the top ten enrichment pathways.
Figure 4 is a figure in Table 2, with Impact as the horizontal coordinate and -LOG10(p) as the vertical coordinate. The pathway marked in the figure above is the significant pathway (Raw p< 0.05).
4 Discussion
Our research findings confirm a causal relationship between 34 metabolites and 18 metabolite ratios with Major Depressive Disorder (MDD). Specifically, increased levels of 13 metabolites including 1-stearoyl-2-arachidonoyl-GPI (18:0/20:4), 1-stearoyl-GPE (18:0), Malonylcarnitine, 1-arachidonoyl-gpc (20:4n6), 1-palmitoyl-2-docosahexaenoyl-gpc (16:0/22:6), 1-palmitoyl-2-arachidonoyl-GPE (16:0/20:4), 1-(1-enyl-palmitoyl)-2-palmitoleoyl-GPC (P-16:0/16:1), Linoleoyl-arachidonoyl-glycerol (18:2/20:4), Methylsuccinate, 1-palmitoyl-2-arachidonoyl-gpc (16:0/20:4n6), Gamma-glutamyltyrosine, Glutamine, N-acetyl-beta-alanine and an increase in 7 metabolite ratios including Arachidonate (20:4n6) to oleate to vaccenate (18:1) ratio, Adenosine 5’-monophosphate (AMP) to glutamine ratio, AMP to asparagine ratio, AMP to serine ratio, Phosphate to serine ratio, AMP to isoleucine ratio, Phosphate to 5-oxoproline ratio, Arachidonate (20:4n6) to linoleate (18:2n6) ratio, and Threonine to pyruvate ratio, Androsterone glucuronide to etiocholanolone glucuronide ratio, are associated with adverse effects on MDD.
Conversely, the elevation of 22 other metabolites including Alpha-hydroxyisocaproate, 2-linoleoylglycerol (18:2), Docosatrienoate (22:3n3), 1-linoleoyl-gpc (18:2), 2-hydroxy-3-methylvalerate, Pregnanediol-3-glucuronide, 1-stearoyl-2-linoleoyl-gpc (18:0/18:2), 1-oleoyl-2-linoleoyl-GPE (18:1/18:2), 1-linoleoyl-2-linolenoyl-GPC (18:2/18:3), Glycosyl-N-tricosanoyl-sphingadienine (d18:2/23:0), Taurodeoxycholate, Cholesterol, N-acetylglutamate, N-acetylhistidine, N6-acetyllysine, 6-oxopiperidine-2-carboxylate, 5-oxoproline, 2-oxoarginine, Alpha-ketobutyrate, Quinate, 2-naphthol sulfate, Adenosine 5’-diphosphate (ADP), along with an increase in 7 additional metabolite ratios, including ADP to AMP ratio, Oleoyl-linoleoyl-glycerol (18:1 to 18:2) [2] to linoleoyl-arachidonoyl-glycerol (18:2 to 20:4) [2] ratio, Methionine to phosphate ratio, Phenylpyruvate to citrate ratio, Cortisol to taurocholate ratio, Alpha-ketoglutarate to proline ratio, Benzoate to linoleoyl-arachidonoyl-glycerol (18:2 to 20:4) [2] ratio, serve a protective role in the onset of MDD. Among the findings, the blood metabolite N6-acetyllysine displayed the most significant correlation with Major Depressive Disorder (MDD). Additionally, our research identified that three functionally enriched pathways: Bile Acid Biosynthesis (FDR = 0.177), Glutathione Metabolism (FDR = 0.177), and Threonine and 2-Oxobutanoate Degradation (FDR = 0.177), along with two metabolic pathways: Valine, Leucine and Isoleucine Biosynthesis (p = 0.04), and Ascorbate and Aldarate Metabolism (p = 0.04), play a crucial role in the progression of MDD.
Major Depressive Disorder (MDD) is a significant factor impacting people’s health and affects the entire lifespan of individuals. It is associated with a plethora of debilitating symptoms beyond mood dysregulation, ranging from cognitive and motor dysfunctions to autonomic nervous symptoms, as well as an increased risk of inflammation, immune system disruption, cardiovascular diseases, and heightened mortality. While most antidepressant medications target the monoaminergic pathways, mounting evidence suggests a more intricate interplay involving multiple pathways, reflecting extensive metabolic alterations, including those in energy and lipid metabolism. Changes in lipids, such as triglycerides, low and very-low-density lipoproteins (LDLs and VLDLs), high-density lipoproteins (HDLs), phosphatidylcholine, lysophosphatidylcholine, and sphingomyelins, have been observed. A recent study involving 5,283 MDD patients and 10,145 controls has indicated that individuals with MDD have reduced levels of HDLs and elevated levels of VLDLs and triglycerides. This indicates that metabolites, particularly lipids, play a crucial role in the onset and progression of MDD (5). To our knowledge, this is the first study utilizing a Mendelian Randomization (MR) approach in order to evaluate the causal relationship between 1,400 blood metabolites and metabolite ratios and the risk of Major Depressive Disorder (MDD).
4.1 Adverse reactions of metabolites to MDD
After excluding unknown metabolites and metabolite ratios, 13 metabolites were found to have adverse effects on Major Depressive Disorder (MDD), including: 1-stearoyl-GPE (18:0), N-acetyl-beta-alanine, Glutamine, Methylsuccinate, Linoleoyl-arachidonoyl-glycerol (18:2/20:4), 1-palmitoyl-2-arachidonoyl-gpc (16:0/20:4n6), 1-palmitoyl-2-arachidonoyl-GPE (16:0/20:4), 1-stearoyl-2-arachidonoyl-GPI (18:0/20:4), 1-palmitoyl-2-docosahexaenoyl-gpc (16:0/22:6), Malonylcarnitine, 1-(1-enyl-palmitoyl)-2-palmitoleoyl-GPC (P-16:0/16:1), Gamma-glutamyltyrosine, and 1-arachidonoyl-gpc (20:4n6). 1-stearoyl-GPE (18:0), also known as LysoPE(18:0/0:0), is a type of lysophospholipid. Lysophosphatidylcholines (lysoPCs) and lysophosphatidylethanolamines (lysoPEs) are categorized as glycerophospholipids, which are the metabolic products of cell membranes. Research indicates that lysoPCs and lysoPEs can be interconverted by the action of phospholipase A2 and phosphatidylethanolamine N-methyltransferase. Furthermore, LysoPC(0:0/18:0) may serve as a sensitive potential biomarker for hepatocyte death induced by chronic exposure to chlorpyrifos in Wistar rats (12, 13). Strikingly, the findings of Zhang et al. revealed a negative correlation between lysoPE (0:0/18:0) and the increased expression of genes related to Hmgcs1, Acat2, and Apoa4. This interaction suggests that lysoPE (0:0/18:0) may disrupt metabolic pathways, including the synthesis and degradation of ketone bodies, fat digestion and absorption, butanoate metabolism, and the degradation of the branched-chain amino acids valine, leucine, and isoleucine, following exposure to PFOS. Consequently, they postulated that reduced liver levels of lysoPE (0:0/18:0) in offspring exposed to PFOS could potentially elevate hepatotoxicity (14). Linoleoyl-arachidonoyl-glycerol (18:2/20:4), also known as PC(18:2(9Z,12Z)/20:4(5Z,8Z,11Z,14Z)), and 1-palmitoyl-2-docosahexaenoyl-gpc (16:0/22:6), referred to as PC(38:6), are types of phosphatidylcholines that are closely associated with breast cancer, cervical cancer, ulcerative colitis, and atherosclerosis. Studies have uncovered a relationship between the gut microbiota-dependent metabolism of dietary phosphatidylcholine and the pathogenesis of cardiovascular diseases (15). 1-palmitoyl-2-arachidonoyl-gpc (16:0/20:4n6), also known as PC(16:0/20:4(5Z,8Z,11Z,14Z)), along with 1-arachidonoyl-gpc (20:4n6), are currently under-researched. 1-palmitoyl-2-arachidonoyl-GPE (16:0/20:4)(PE(16:0/20:4(5Z,8Z,11Z,14Z))) is a phosphatidylethanolamine that has recently been recognized for its significant role in mammalian health following its association with diseases such as Alzheimer’s, Parkinson’s, non-alcoholic liver disease, and the virulence of certain pathogens through the discovery of its metabolic importance (16). 1-stearoyl-2-arachidonoyl-GPI (18:0/20:4), also known as PI(18:0/20:4(5Z,8Z,11Z,14Z)), is a phosphatidylinositol, which is an essential lipid playing dual roles as a crucial membrane component and as a participant in fundamental metabolic processes. Phosphoinositides (PI) comprise only a small fraction of the total cellular phospholipid content, yet they have a significant role in the development and progression of cancer. In various types of cancer, specific phosphoinositides such as phosphatidylinositol 3,4,5-trisphosphate [PtdIns(3,4,5)P3] and phosphatidylinositol 4,5-bisphosphate [PtdIns(4,5)P2] are critically involved in the regulation of survival, proliferation, invasion, and growth of cancer cells (17). Furthermore, they are closely associated with conditions such as Alzheimer’s disease, epileptic seizures, and Parkinson’s disease (18).
N-Acetyl-beta-alanine, also known as 3-(acetylamino)propionic acid, falls into the category of organic compounds known as carboxylic acids. It is an endogenous β amino acid that is metabolized into acetate and β alanine by the enzyme N-acetyl-β alanine dehydrogenase. β-alanine serves as an intermediate molecule between GABA (gamma-aminobutyric acid) and glycine, exhibiting a mechanism of action that is very similar to these neurotransmitters. It is considered to be an inhibitory neurotransmitter. Studies have shown that metabolomic analysis of the prefrontal cortex in mice indicates an upregulation of N-acetyl-beta-alanine during wakefulness compared to sleep, suggesting a potential link with sleep regulation (19). It is well-established that there is a close correlation between sleep and major depressive disorder. Studies support the notion that an early bedtime is associated with a protective effect against the risk of developing major depressive disorder (20, 21).
Therefore, whether N-acetyl-beta-alanine could be a metabolic product associated with the link between sleep and major depressive disorder (MDD) merits further investigation. Glutaminylglutamine, which belongs to the class of organic compounds known as dipeptides, as well as Methylsuccinic, Malonylcarnitine, and gamma-Glutamyltyrosine, have been minimally studied in relation to MDD.
4.2 Positive effects of metabolites on MDD
After excluding unknown metabolites and their ratios, there are 22 metabolites identified that have an adverse impact on Major Depressive Disorder (MDD), including: N6-Acetyl-L-lysine, Pregnanediol 3-O-glucuronide, 6-Oxopiperidine-2-carboxylic acid, MG(0:0/18:2(9Z,12Z)/0:0), Docosatrienoic acid, LysoPC(18:2/0:0), Quinic acid, ADP, SM(d18:2(4E,14Z)/23:0), N-Acetyl-L-glutamic acid, Cholesterol, Pyroglutamic acid, 2-Oxoarginine, PC(18:2(9Z,12Z)/18:3(6Z,9Z,12Z)), PE(18:1(9Z)/18:2(9Z,12Z)), N-Acetylhistidine, Taurodeoxycholic acid, 2-Ketobutyric acid, (+/-)-Ethyl 2-hydroxy-3-methylvalerate, PC(18:0/18:2(9Z,12Z)), Hydroxyisocaproic acid, and 2-Naphthol sulfate.
Existing research has discovered that in overweight and obese COVID-19 patients, levels of N6-acetyl-L-lysine and p-cresol are elevated. Lysine acetylation is an emerging post-translational pathway primarily induced by obesity, which has been proven to modulate the enzymatic activity involved in fatty acid and glucose metabolism. This mechanism involves the transfer of an acetyl group from acetyl coenzyme A (acetyl-CoA), which is a key mediator and metabolic regulator of protein acetylation, targeting the amino groups of lysine (22). Existing research has discovered that disruption in lysine degradation may play a role in the development of early cardiac hypertrophy. Metabolites such as N6-acetyl-L-lysine might serve as potential predictive and therapeutic targets for subclinical myocardial cell hypertrophy (23). The primary metabolic product of progesterone is pregnanediol. The excretion of pregnanediol in urine can be used as an indicator to evaluate luteal function. Pregnanediol 3-O-glucuronide is a natural metabolite of pregnanediol, produced by the action of UDP-glucuronyltransferase in the liver. A metabolomic study has indicated that the levels of pregnanediol 3-O-glucuronide are significantly reduced in the placental metabolome of women with spontaneous preterm birth (24). 2-Linoleoylglycerol (18:2) has been identified as being associated with colorectal cancer (25). LysoPC (18:2/0:0) and 1-linoleoyl-gpc (18:2) are considered to be candidate diagnostic biomarkers for Hunner’s type interstitial cystitis (26). Additionally, they may represent potential therapeutic targets for patients hospitalized with mild traumatic brain injury (27). Studies have found that dicaffeoylquinic acids (diCQAs) reduce depression-like behaviors in mice treated with corticosterone (CORT), including memory loss. The potential mechanism of diCQAs’ antidepressant effects may involve the inhibition of monoamine oxidase types A and B (MAO-A and MAO-B) activities in neurons and astrocytes, leading to a decrease in the production of reactive oxygen species (ROS) in the brain (28). This is consistent with our findings that quinic acid acts as a protective factor against Major Depressive Disorder (MDD). Adenosine 5’-diphosphate (ADP) is a vital organic compound in metabolism and is crucial for the flow of energy within living cells. Adenosine 5’-(α,β methylene)diphosphate (APCP), an ecto-5’-nucleotidase (e5NT) inhibitor, was administered through intraventricular injection to explore the regulatory effects of e5NT on nucleoside levels and the behavioral changes induced by acute restraint stress in mice.
Liu and colleagues have posited that inhibiting ecto-5’-nucleotidase (e5NT) could potentially alleviate anxious behaviors in mice. Consequently, targeting e5NT might represent a promising therapeutic strategy for the management of anxiety in murine models (29). Research indicates that the ratio between non-high-density lipoprotein cholesterol and high-density lipoprotein cholesterol is significantly correlated with an increased risk of depression among adults in the United States (30). The Reverse Cholesterol Transport (RCT) system constitutes a vital detoxification pathway that facilitates the clearance of free cholesterol from the body, thereby preventing the development of atherosclerosis. It also protects against lipid peroxidation, the oxidation of low-density lipoprotein (LDL) and high-density lipoprotein (HDL) cholesterol, as well as inflammatory responses. Current evidence suggests that dysfunction within the HDL-PON1-ApoA-LCAT complex is closely associated with the pathogenesis of affective disorders, the recurrence of Major Depressive Disorder (MDD) and Bipolar Disorder (BD), suicidal behaviors, and the severity of depression. Interestingly, in approximately 30% of patients experiencing severe Major Depressive Episodes (MDE), higher Hamilton Depression Rating Scale (HAMD) scores have also been linked with elevated levels of triglycerides, total cholesterol, and LDL cholesterol (31).
4.3 Metabolic pathway analysis
Bile acids, synthesized predominantly in the liver through the enzymatic oxidation of cholesterol, are essential for the digestion and absorption of lipids and fat-soluble vitamins. Emerging research has uncovered further physiological roles for bile acids that extend beyond their digestive function. These include the modulation of energy homeostasis, regulation of glucose metabolism, and influence on immune system responses. The mechanism underlying these diverse effects involves the activation of bile acid receptors, particularly the Farnesoid X receptor (FXR) and the G protein-coupled bile acid receptor 5 (TGR5). In recent years, studies have begun to examine the potential link between bile acid biosynthesis and mood regulation, particularly in relation to major depression. Some research suggests that bile acids may indirectly impact mood and cognitive functions by affecting the composition of the gut microbiota, communication along the gut-brain axis, and the release of hormones and metabolites. The gut microbiome is considered a potential key factor influencing brain function and emotional regulation, with bile acids being one of the elements that regulate the balance of gut flora (32, 33).
The antioxidant glutathione (GSH), tripeptidic in nature, plays a pivotal role in numerous biological processes. As the most abundant non-protein thiol antioxidant within cells, it primarily functions to neutralize reactive oxygen species (ROS) and peroxides, thereby shielding cells from the damaging effects of oxidative stress. Oxidative stress is thought to potentially play a role in mental illnesses, especially in major depression. Some studies have indicated that there may be an increase in oxidative stress among individuals with depression, and GSH, as a key antioxidant, could have its levels and metabolism affected in this context. Low levels of GSH may lead to a reduced capacity for antioxidant defense in the brain, potentially damaging neurons, disrupting the balance of neurotransmitters, and consequently, might be associated with the development of depressive symptoms (34). Furthermore, some studies have shown that a decline in glutathione levels may be connected with functional impairments in specific brain regions, such as the prefrontal cortex, which is a key area associated with emotion regulation and cognitive functions. In addition, inflammation has been linked to depression, and GSH plays a role in regulating inflammatory responses (35).
However, further research is needed to elucidate the precise mechanisms of GSH metabolism and how it may directly or indirectly affect the onset, progression, and treatment of depression. This could include investigating whether supplementing GSH or enhancing the activity of its metabolic pathways could serve as potential therapeutic approaches for depression. This aligns with the findings of our current research. To date, there is no direct evidence or widely recognized studies linking the metabolism of threonine and 2-oxobutanoate directly with major depression. However, the connection between metabolic pathways and mental health conditions is an area continuously explored in psychopathology and neurobiology, which includes the influence of amino acid metabolic pathways on emotion regulation and brain function.
Threonine is an essential amino acid that plays a role in protein synthesis as a building block and also participates in key metabolic pathways such as the threonine-methionine cycle and the glycine-serine cycle. Additionally, threonine can be transformed into glycine and serine and is associated with one-carbon metabolism, which involves neurotransmitter synthesis and methylation reactions (36).
2-Oxobutanoate is an intermediate product in the degradation pathways of certain amino acids such as isoleucine, lysine, methionine, and threonine. Through metabolic reactions, it can ultimately be converted into succinyl-CoA, which then enters the tricarboxylic acid cycle (TCA cycle), a critical pathway for cellular energy production (37).
Valine, leucine, and isoleucine are three branched-chain amino acids (BCAAs) which have been implicated in the pathogenesis of various mental disorders, including major depressive disorder (MDD), according to some studies. Research suggests that patients with depression might exhibit alterations in BCAAs levels in their blood compared to the healthy population, and these changes could reflect on their mood, cognitive function, and overall mental state. BCAAs serve as precursors for crucial neurotransmitters in the brain, can compete with tryptophan and tyrosine for the same transport system across the blood-brain barrier, and are also involved in energy metabolism, potentially exerting a significant impact on brain function, particularly in response to stress and during the regulation of emotions (38, 39).
Ascorbate metabolism and aldarate metabolism involve the biochemical pathways and respective metabolites of vitamin C (ascorbate) within the human body (40). Ascorbic acid, commonly known as vitamin C, is a potent antioxidant that helps neutralize free radicals and reduce oxidative stress. Oxidative stress is believed to play a critical role in the onset and progression of depression. Vitamin C is involved in the synthesis of various neurotransmitters, such as dopamine and serotonin, which are directly linked to mood regulation, depression, and other psychological disorders. The connection between inflammation and depression is increasingly recognized, and vitamin C possesses anti-inflammatory properties. Vitamin C has demonstrated neuroprotective effects in some studies, potentially aiding in the prevention of neurodegenerative diseases, which may be associated with maintaining cognitive function and emotional health (41). However, at present, there is limited research on its association with Major Depressive Disorder (MDD), which warrants further investigation.
Depression may be linked to a variety of factors, including genetics, environment, psychosocial elements, and the neurobiology of the brain. Abnormalities in certain metabolic pathways may lead to or be associated with changes in neurotransmitter balance, which in turn affect mood. For instance, disruptions in methylation reactions can impact gene expression and neurotransmitter synthesis, while aberrations in the energy metabolism implicated in the tricarboxylic acid (TCA) cycle may affect the function of the nervous system (42). Therefore, these metabolic pathways are closely related to Major Depressive Disorder (MDD) and warrant further in-depth research.
4.4 Advantages and limitations
This study boasts several advantages. Firstly, using GWAS data, our Mendelian Randomization (MR) analysis provides new insights into potential causal mediators for 1,400 metabolites and Major Depressive Disorder (MDD). Secondly, the multiple cohort setup based on original GWAS data enables us to make effective causal inferences within a large population scale, yielding high statistical power. Lastly, by integrating the relevant metabolites into MetaboAnalyst 6.0 for analysis, we are able to comprehensively evaluate the functional enrichment and metabolic pathways of these metabolites. This can further aid in informing the prioritization of drug targets and the development of clinical trials.
However, our study is not without its limitations. First, our MR study is based on summary data from GWAS, while psychiatric disorders are primarily caused by brain pathologies. Further research is required to analyze changes in metabolites within the cerebrospinal fluid to identify other promising biomarkers and drug targets for psychiatric conditions. Second, our study was predominantly conducted in individuals of European descent, reducing population stratification bias yet limiting the extrapolation of our findings across different ethnicities. Additional research in non-European populations is necessary to confirm our findings. Lastly, there may be participant overlap between GWAS cohorts, potentially leading to weak instrument bias. Although F-statistics suggest that there is no instrument bias in our MR study, further MR research based on independent cohorts with no participant overlap is needed to better understand the role of blood metabolites in the etiology of psychiatric diseases.
5 Conclusion
In summary, this MR study has determined that out of 1,400 blood metabolites analyzed, we have identified 34 known metabolites and 13 unknown metabolites, as well as 18 metabolite ratios, that are associated with Major Depressive Disorder (MDD). Additionally, we have highlighted 3 functionally enriched groups and 2 metabolic pathways. These findings provide preliminary evidence on the impact of blood metabolite dysregulation on the risk of MDD. The integration of genomics and metabolomics offers significant insights for the screening and prevention of MDD.
This study identified specific metabolites associated with increased or decreased risk of major depression. For example, N6-acetyllysine levels and the levels of 1-stearoyl-GPE (18: 0). This is crucial because these metabolites can be used as biomarkers for early detection or development risk of major depression. In clinical practice, having reliable biomarkers can significantly improve the screening process and carry out early intervention, which is of great significance for the treatment of this disease. The rich data of metabolic pathways (such as bile acid biosynthesis, glutathione metabolism) enhance the understanding of the pathophysiological mechanism behind MDD. This deeper understanding may lead to the development of new therapeutic targets. For example, interventions aimed at altering specific metabolic pathways may help prevent or alleviate symptoms of major depression. In addition, this study also paves the way for more personalized treatment interventions. Understanding individual metabolic characteristics can enable clinicians to tailor treatment plans based on the patient ‘s specific metabolic disorders, thereby potentially improving the therapeutic effect and minimizing unnecessary side effects.
Data availability statement
The datasets presented in this study can be found in online repositories. The names of the repository/repositories and accession number(s) can be found in the article/Supplementary Material.
Ethics statement
Ethical review and approval was not required for the study of human participants in accordance with the local legislation and institutional requirements.
Author contributions
TD: Conceptualization, Methodology, Supervision, Writing – original draft, Writing – review & editing. XW: Data curation, Investigation, Software, Writing – original draft. ZJ: Formal analysis, Methodology, Project administration, Writing – original draft. YL: Formal analysis, Project administration, Resources, Writing – review & editing. JY: Funding acquisition, Project administration, Visualization, Writing – review & editing.
Funding
The author(s) declare financial support was received for the research, authorship, and/or publication of this article. National Natural Science Foundation of China (No. 82174491) supports this research.
Acknowledgments
The authors thank the participants and investigators of each GWAS study included in this project.
Conflict of interest
The authors declare that the research was conducted in the absence of any commercial or financial relationships that could be construed as a potential conflict of interest.
Publisher’s note
All claims expressed in this article are solely those of the authors and do not necessarily represent those of their affiliated organizations, or those of the publisher, the editors and the reviewers. Any product that may be evaluated in this article, or claim that may be made by its manufacturer, is not guaranteed or endorsed by the publisher.
Supplementary material
The Supplementary Material for this article can be found online at: https://www.frontiersin.org/articles/10.3389/fpsyt.2024.1391535/full#supplementary-material
References
1. Liu Q, He H, Yang J, Feng X, Zhao F, Lyu J. Changes in the global burden of depression from 1990 to 2017: Findings from the Global Burden of Disease study. J Psychiatr Res. (2020) 126:134–40. doi: 10.1016/j.jpsychires.2019.08.002
2. WHO (World Health Organ). Depression. Fact Sheet. Geneva: WHO (2021). Available at: http://www.who.int/news-room/fact-sheets/detail/depression.
3. Monroe SM, Harkness KL. Major depression and its recurrences: life course matters. Annu Rev Clin Psychol. (2022) 18:329–57. doi: 10.1146/annurev-clinpsy-072220–021440
4. Dwyer JB, Aftab A, Radhakrishnan R, Widge A, Rodriguez CI, Carpenter LL, et al. Hormonal treatments for major depressive disorder: state of the art. Am J Psychiatry. (2020) 177:686–705. doi: 10.1176/appi.ajp.2020.19080848
5. Amin N, Liu J, Bonnechere B, MahmoudianDehkordi S, Arnold M, Batra R, et al. Interplay of metabolome and gut microbiome in individuals with major depressive disorder vs control individuals. JAMA Psychiatry. (2023) 80:597–609. doi: 10.1001/jamapsychiatry.2023.0685
6. Kroenke K, Spitzer RL, Williams JB. The PHQ-9: validity of a brief depression severity measure. J Gen Intern Med. (2001) 16:606–13. doi: 10.1046/j.1525-1497.2001.016009606.x
7. Zimmerman M. Symptom severity and guideline-based treatment recommendations for depressed patients: implications of DSM-5’s potential recommendation of the PHQ-9 as the measure of choice for depression severity. Psychother Psychosom. (2012) 81:329–32. doi: 10.1159/000342262
8. Zheng X, Zhou X, Tong L, Gu W, Wang S, Yuang W, et al. Mendelian randomization study of gastroesophageal reflux disease and major depression. PloS One. (2023) 18:e0291086. doi: 10.1371/journal.pone.0291086
9. Yang J, Yan B, Zhao B, Fan Y, He X, Yang L, et al. Assessing the causal effects of human serum metabolites on 5 major psychiatric disorders. Schizophr Bull. (2020) 46:804–13. doi: 10.1093/schbul/sbz138
10. Choi KW, Chen C, Stein MB, Klimentidis YC, Wang MJ, Koenen KC, et al. Assessment of bidirectional relationships between physical activity and depression among adults: A 2-sample mendelian randomization study. JAMA Psychiatry. (2019) 76:399–408. doi: 10.1001/jamapsychiatry.2018.4175
11. Zhao X, Liu L. Mendelian randomization analyses for the causal relationship between early age at first sexual intercourse, early age at first live birth, and postpartum depression in pregnant women. Front Psychiatry. (2024) 15:1287934. doi: 10.3389/fpsyt.2024.1287934
12. Ren C, Liu J, Zhou J, Liang H, Wang Y, Sun Y, Ma B, Yin Y. Lipidomic analysis of serum samples from migraine patients. Lipids Health Dis. (2018) 17(1):22. doi: 10.1186/s12944–018-0665–0
13. Yang J, Wang H, Xu W, Hao D, Du L, Zhao X, Sun C. Metabolomic analysis of rat plasma following chronic low-dose exposure to dichlorvos. Hum Exp Toxicol. (2013) 32(2):196–205. doi: 10.1177/0960327112459533
14. Zhang R, Yu G, Luo T, Zeng X, Sun Y, Huang B, et al. Transcriptomic and metabolomic profile changes in the liver of Sprague Dawley rat offspring after maternal PFOS exposure during gestation and lactation. Ecotoxicol Environ Saf. (2024) 270:115862. doi: 10.1016/j.ecoenv.2023.115862
15. Wang Z, Klipfell E, Bennett B, Koeth R, Levison BS, Dugar B, et al. Gut flora metabolism of phosphatidylcholine promotes cardiovascular disease. Nature. (2011) 472:57–63. doi: 10.1038/nature09922
16. Calzada E, Onguka O, Claypool SM. Phosphatidylethanolamine metabolism in health and disease. Int Rev Cell Mol Biol. (2016) 321:29–88. doi: 10.1016/bs.ircmb.2015.10.001
17. Owusu Obeng E, Rusciano I, Marvi MV, Fazio A, Ratti S, Follo MY, et al. Phosphoinositide-dependent signaling in cancer: A focus on phospholipase C isozymes. Int J Mol Sci. (2020) 21:2581. doi: 10.3390/ijms21072581
18. Dickson EJ. Recent advances in understanding phosphoinositide signaling in the nervous system. F1000Res. (2019) 8:F1000. doi: 10.12688/f1000research.16679.1
19. Bourdon AK, Spano GM, Marshall W, Bellesi M, Tononi G, Serra PA, et al. Metabolomic analysis of mouse prefrontal cortex reveals upregulated analytes during wakefulness compared to sleep. Sci Rep. (2018) 8:11225. doi: 10.1038/s41598–018-29511–6
20. Daghlas I, Lane JM, Saxena R, Vetter C. Genetically proxied diurnal preference, sleep timing, and risk of major depressive disorder. JAMA Psychiatry. (2021) 78:903–10. doi: 10.1001/jamapsychiatry.2021.0959
21. Cunningham JEA, Stamp JA, Shapiro CM. Sleep and major depressive disorder: a review of non-pharmacological chronotherapeutic treatments for unipolar depression. Sleep Med. (2019) 61:6–18. doi: 10.1016/j.sleep.2019.04.012
22. Jalaleddine N, Hachim M, Al-Hroub H, Saheb Sharif-Askari N, Senok A, Elmoselhi A, et al. N6-acetyl-L-lysine and p-cresol as key metabolites in the pathogenesis of COVID-19 in obese patients. Front Immunol. (2022) 13:827603. doi: 10.3389/fimmu.2022.827603
23. Liu J, Hu J, Tan L, Zhou Q, Wu X. Abnormalities in lysine degradation are involved in early cardiomyocyte hypertrophy development in pressure-overloaded rats. BMC Cardiovasc Disord. (2021) 21:403. doi: 10.1186/s12872-021-02209-w
24. Zhang Y, Li W, Chen TT, Yang Y, Wu MY, Luo JY, et al. Chemical fingerprint analysis and ultra-performance liquid chromatography quadrupole time-of-flight mass spectrometry-based metabolomics study of the protective effect of buxue yimu granule in medical-induced incomplete abortion rats. Front Pharmacol. (2020) 11:578217. doi: 10.3389/fphar.2020.578217
25. Gao R, Wu C, Zhu Y, Kong C, Zhu Y, Gao Y, et al. Integrated analysis of colorectal cancer reveals cross-cohort gut microbial signatures and associated serum metabolites. Gastroenterology. (2022) 163:1024–1037.e9. doi: 10.1053/j.gastro.2022.06.069
26. Torimoto K, Ueda T, Kasahara M, Hirayama A, Matsushita C, Matsumoto Y, et al. Identification of diagnostic serum biomarkers for Hunner-type interstitial cystitis. Low Urin Tract Symptoms. (2022) 14:334–40. doi: 10.1111/luts.12439
27. Gusdon AM, Savarraj JP, Redell JB, Paz A, Hinds S, Burkett A, et al. Lysophospholipids are associated with outcomes in hospitalized patients with mild traumatic brain injury. J Neurotrauma. (2024) 41:59–72. doi: 10.1089/neu.2023.0046
28. Lim DW, Park J, Jung J, Kim SH, Um MY, Yoon M, et al. Dicaffeoylquinic acids alleviate memory loss via reduction of oxidative stress in stress-hormone-induced depressive mice. Pharmacol Res. (2020) 161:105252. doi: 10.1016/j.phrs.2020.105252
29. Liu P, Zhu L, Zhou L, Bai X. e5NT inhibitor protects acute restraint stress-induced depression by regulating nucleoside release in mice. J Pharm Pharmacol. (2020) 72:1556–63. doi: 10.1111/jphp.13341
30. Qi X, Wang S, Huang Q, Chen X, Qiu L, Ouyang K, et al. The association between non-high-density lipoprotein cholesterol to high-density lipoprotein cholesterol ratio (NHHR) and risk of depression among US adults: A cross-sectional NHANES study. J Affect Disord. (2024) 344:451–7. doi: 10.1016/j.jad.2023.10.064
31. Wagner CJ, Musenbichler C, Böhm L, Färber K, Fischer AI, von Nippold F, et al. LDL cholesterol relates to depression, its severity, and the prospective course. Prog Neuropsychopharmacol Biol Psychiatry. (2019) 92:405–11. doi: 10.1016/j.pnpbp.2019.01.010
32. Sun N, Zhang J, Wang J, Liu Z, Wang X, Kang P, et al. Abnormal gut microbiota and bile acids in patients with first-episode major depressive disorder and correlation analysis. Psychiatry Clin Neurosci. (2022) 76:321–8. doi: 10.1111/pcn.13368
33. Tung TH, Chen YC, Lin YT, Huang SY. N-3 PUFA ameliorates the gut microbiota, bile acid profiles, and neuropsychiatric behaviours in a rat model of geriatric depression. Biomedicines. (2022) 10:1594. doi: 10.3390/biomedicines10071594
34. Mandal PK, Gaur S, Roy RG, Samkaria A, Ingole R, Goel A. Schizophrenia, bipolar and major depressive disorders: overview of clinical features, neurotransmitter alterations, pharmacological interventions, and impact of oxidative stress in the disease process. ACS Chem Neurosci. (2022) 13:2784–802. doi: 10.1021/acschemneuro.2c00420
35. Todorović N, Filipović D. Prefrontal cortical glutathione-dependent defense and proinflammatory mediators in chronically isolated rats: Modulation by fluoxetine or clozapine. Neuroscience. (2017) 355:49–60. doi: 10.1016/j.neuroscience.2017.04.044
36. Malinovsky AV. Why threonine is an essential amino acid in mammals and birds: studies at the enzyme level. Biochem (Mosc). (2018) 83:795–9. doi: 10.1134/S0006297918070039
37. Wu N, Ma YC, Gong XQ, Zhao PJ, Jia YJ, Zhao Q, et al. The metabolite alpha-ketobutyrate extends lifespan by promoting peroxisomal function in C. elegans. Nat Commun. (2023) 14:240. doi: 10.1038/s41467–023-35899–1
38. Zandonadi FS, Silva AAR, Melo AAS, Ignarro RS, Matos TS, Santos EAF, et al. Understanding ayahuasca effects in major depressive disorder treatment through in vitro metabolomics and bioinformatics. Anal Bioanal Chem. (2023) 415:4367–84. doi: 10.1007/s00216–023-04556–3
39. Zakaria F, Akhtar MT, Wan Norhamidah WI, Noraini AB, Muhamad A, Shohaimi S, et al. Centella asiatica (L.) Urb. Extract ameliorates branched-chain amino acid (BCAA) metabolism in acute reserpine-induced stress zebrafish model via 1H Nuclear Magnetic Resonance (NMR)-based metabolomics approach. Comp Biochem Physiol C Toxicol Pharmacol. (2023) 264:109501. doi: 10.1016/j.cbpc.2022.109501
40. Hondal RJ. Selenium vitaminology: The connection between selenium, vitamin C, vitamin E, and ergothioneine. Curr Opin Chem Biol. (2023) 75:102328. doi: 10.1016/j.cbpa.2023.102328
41. Moritz B, Schmitz AE, Rodrigues ALS, Dafre AL, Cunha MP. The role of vitamin C in stress-related disorders. J Nutr Biochem. (2020) 85:108459. doi: 10.1016/j.jnutbio.2020.108459
Keywords: metabolites, major depressive disorder (MDD), Mendelian randomization study, pathways, metabolomics
Citation: Dong T, Wang X, Jia Z, Yang J and Liu Y (2024) Assessing the associations of 1,400 blood metabolites with major depressive disorder: a Mendelian randomization study. Front. Psychiatry 15:1391535. doi: 10.3389/fpsyt.2024.1391535
Received: 28 February 2024; Accepted: 13 May 2024;
Published: 06 June 2024.
Edited by:
Alina Wilkowska, Medical University of Gdansk, PolandCopyright © 2024 Dong, Wang, Jia, Yang and Liu. This is an open-access article distributed under the terms of the Creative Commons Attribution License (CC BY). The use, distribution or reproduction in other forums is permitted, provided the original author(s) and the copyright owner(s) are credited and that the original publication in this journal is cited, in accordance with accepted academic practice. No use, distribution or reproduction is permitted which does not comply with these terms.
*Correspondence: Jiguo Yang, c2R5YW5namlndW9AMTI2LmNvbQ==; Yuanxiang Liu, bHl4bHd0Z0AxMjYuY29t