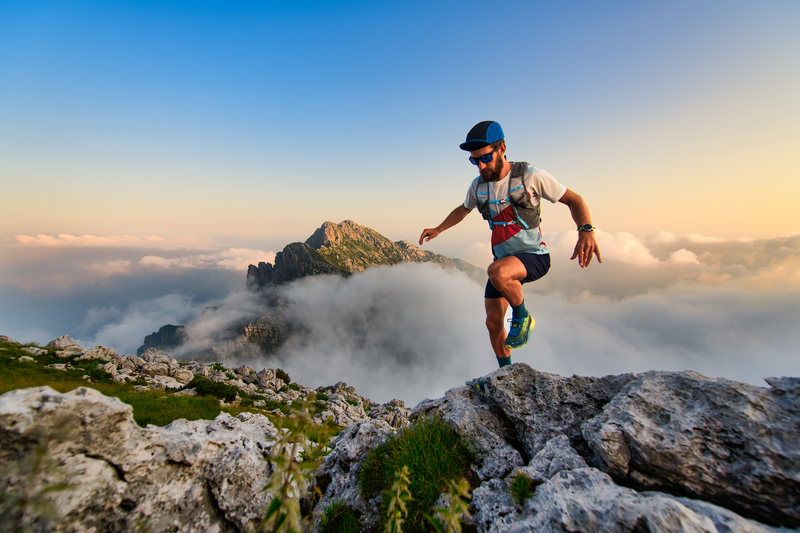
94% of researchers rate our articles as excellent or good
Learn more about the work of our research integrity team to safeguard the quality of each article we publish.
Find out more
ORIGINAL RESEARCH article
Front. Psychiatry , 29 April 2024
Sec. Neuroimaging
Volume 15 - 2024 | https://doi.org/10.3389/fpsyt.2024.1349989
Objective: Although extensive structural and functional abnormalities have been reported in schizophrenia, the gray matter volume (GMV) covariance of the amygdala remain unknown. The amygdala contains several subregions with different connection patterns and functions, but it is unclear whether the GMV covariance of these subregions are selectively affected in schizophrenia.
Methods: To address this issue, we compared the GMV covariance of each amygdala subregion between 807 schizophrenia patients and 845 healthy controls from 11 centers. The amygdala was segmented into nine subregions using FreeSurfer (v7.1.1), including the lateral (La), basal (Ba), accessory-basal (AB), anterior-amygdaloid-area (AAA), central (Ce), medial (Me), cortical (Co), corticoamygdaloid-transition (CAT), and paralaminar (PL) nucleus. We developed an operational combat harmonization model for 11 centers, subsequently employing a voxel-wise general linear model to investigate the differences in GMV covariance between schizophrenia patients and healthy controls across these subregions and the entire brain, while adjusting for age, sex and TIV.
Results: Our findings revealed that five amygdala subregions of schizophrenia patients, including bilateral AAA, CAT, and right Ba, demonstrated significantly increased GMV covariance with the hippocampus, striatum, orbitofrontal cortex, and so on (permutation test, P< 0.05, corrected). These findings could be replicated in most centers. Rigorous correlation analysis failed to identify relationships between the altered GMV covariance with positive and negative symptom scale, duration of illness, and antipsychotic medication measure.
Conclusion: Our research is the first to discover selectively impaired GMV covariance patterns of amygdala subregion in a large multicenter sample size of patients with schizophrenia.
Schizophrenia (SCZ), as a mental disorder with a high rate of disability, has a serious influence on the normal life of patients and their families, and imposes a significant burden on society (1). The main clinical symptoms of schizophrenia patients include hallucinations, disorganized thinking, impaired executive ability, and reduced emotional expression (2). Numerous neuroimaging studies of SCZ have demonstrated the abnormalities in multiple brain regions, especially the prefrontal cortex (PFC) (3) and hippocampus (4), and proposed a hypothesis that SCZ is a widespread dysconnection disorder (5). Recently, the amygdala has been a research focus in human mental health (6), and shows a close relationship with the pathophysiology of schizophrenia (7).
As a connecting hub, the amygdala has extensive connections to cortical and subcortical areas such as the frontal lobe, temporal lobe, and striatum (8), and plays important roles in emotional processing, memory encoding, and executive control functions (7). Research has highlighted that the medial prefrontal lobe, which exhibits significant connectivity with the amygdala, plays a crucial role in modulating stress, facilitating social cognition, making decisions in events, and regulating emotions (9–11). Furthermore, evidence suggests that negative psychological states, such as stress or anxiety, can impair the regulatory functions of the medial prefrontal-amygdala circuit (12, 13). In parallel, the amygdala’s direct and robust connection with the hippocampus is essential for processing contextual memories and behaviors (14–16). Investigations using structural MRI have revealed a reduction in amygdala volume in schizophrenia patients and their first-degree relatives compared to healthy subjects (17–19). Studies utilizing resting state functional MRI studies have identified disturbances in the amygdala’s functional connectivity with other cerebral regions (20–23). Such structural and functional anomalies contribute to cognitive deficits in schizophrenia (24, 25). Therefore, the disrupted amygdala connectivity may serve as a potential trigger for schizophrenia, and its intensive study contributes to our understanding of the pathophysiological mechanisms of schizophrenia (26).
The amygdala can be defined as a more detailed heterogeneous complex of nuclei due to its different cytoarchitecture, neurotransmitters, and connectivity patterns (27). It has been demonstrated that different heterogeneous nuclei are embedded in various connectivity pathways and perform roles in processing different cognitions. For instance, the cortical nucleus is the main projection nucleus of the olfactory cortex (27); the lateral nucleus has a significant role in the fear conditioning reflex (28); the central nucleus receives information input from the hippocampus (29). Reductions in the volume of refined amygdala nuclei were apparent in schizophrenia: all nuclei except the medial nucleus, suggesting a more widespread change in amygdala morphology than previously thought (30).
According to the dysconnection hypothesis, schizophrenia is characterized by a reduced ability to integrate information between distinct brain regions (5, 31, 32). Structural covariance is often used to analyze the topology of the brain as a method capable of testing for connectivity deficits in brain regions. Differences in internal covariance patterns between schizophrenia and healthy controls were revealed by calculating correlations in morphological indicators such as volume, cortical thickness, or surface area of brain regions (33). Recently, based on the more refined segmentation approach, researchers found that the amygdala subregions exhibit selective regional structural damages in schizophrenia patients (30, 34). Thus, we have developed a hypothesis that the gray matter volume (GMV) covariance of amygdala subregions would be selectively impaired in schizophrenia. To test this hypothesis, we investigated whether GMV covariance of amygdala subregions selectively differs between schizophrenia and healthy controls; moreover, we tried to validate if the impairment patterns could be replicated by multicenter datasets from multiple first-episode versus non-first-episode schizophrenia centers.
A total of 11 datasets was enrolled in the study as follows: 2 first-episode schizophrenia local datasets (FE_Guangzhou and FE_Harbin) and 9 non-first-episode schizophrenia local and public datasets with 5 local (NFE_Tianjin1, NFE_Tianjin2, NFE_Shanghai, NFE_Harbin and NFE_Wuhan) and 4 public datasets (NFE_BrainGluSchi [www.schizconnect.org], NFE_COBRE [http://fcon_1000projects.nitrc.org/indi/retro/cobre.html], NFE-NMorphCH [www.schizconnect.org] and NFE_UCLA [https://openneuro.org/datasets/ds000030/versions/00016]). The patients with schizophrenia were diagnosed by the structured clinical interview in DSM-IV. Exclusive criteria were contraindications to MRI; intracerebral lesions or structural abnormalities; no history of mental illness; no history of alcohol, drug, or substance abuse; female subjects were not pregnant or lactating. Other exclusive criteria for healthy controls were a history of any Axis I or II disorder and psychiatric disorders and first-degree relatives with psychiatric disorders. Our definition of a patient with first-episode schizophrenia was that the patient was not on antipsychotic medication or had not been on medication for more than two weeks at the time of inclusion in the trial. To eliminate possible effects of covariates on the results, we matched the age and sex of healthy controls (HC) to patients with schizophrenia in each center separately. Eventually, we included 831 schizophrenia patients and 851 healthy controls. The studies involving human participants were reviewed and approved by the Ethics Committee of Tianjin Medical University General Hospital, Tianjin, China. The patients/participants provided their written informed consent to participate in this study. Relevant Institutional Review Boards also approved the four public test-retest datasets, and detailed recruitment information was provided on the website.
During the acquisition, subjects were told to keep their bodies still, to immobilize their heads using comfortable and tight foam pads, and to use earplugs to reduce scanning noise. Structural MRI data were all acquired by 3.0T MRI scanners, including two GE MR750 scanners, four Siemens Trio Tim scanners, one Siemens Prisma, one Siemens Prisma_fit scanner, one Philips Ingenia, and one Philips Achiva scanner. A 3D fast spoided gradient echo sequence was used to acquire the high-resolution T1-weighted structural MRI images. The scanner information, sequence, and acquisition parameters are shown in Table 1. To ensure the image quality of the dataset, each MRI image was scrutinized by two experienced MRI experts (W.Q and H.L). We removed 30 subjects with obvious artifacts (head motion artifacts, wrap-around artifacts, metal artifacts, etc.), including 24 patients with schizophrenia and 6 healthy controls. Finally, the T1 structural MRIs of 1652 participants, including 807 schizophrenia patients and 845 healthy controls, were included in the following analyses.
Previous neuroimaging approaches divided the amygdala into 2-4 nuclei (35–38). However, most of these amygdala atlases are group-based and ignore the inter-subject variability of the subfields’ boundaries, which may introduce bias in calculating the covariance between these amygdala subfields and other brain regions. Thus, in this study, we turn to apply an individual-level segmentation atlas to define the amygdala subfields, which has been incorporated into the FreeSurfer software package v7.1.1 (http://surfer.nmr.mgh.harvard.edu/). This automatic atlas segments the amygdala subfields based on each person’s high-resolution MRI data and Bayesian inference algorithm with postmortem specimens at high resolution at 7T field strength as reference (39). The amygdala of each subject was automatically segmented into 9 subregions per hemisphere: lateral (La), basal (Ba), accessory-basal (AB), anterior-amygdaloid-area (AAA), central (Ce), medial (Me), cortical (Co), corticoamygdaloid-transition (CAT), paralaminar (PL) nucleus (39). Finally, we extracted the volume of each amygdala subregion and the total volume for each side of the amygdala.
Other cerebral areas’ absolute GMV and total intracranial volume (TIV) were obtained by the new segment pipeline using SPM12 (SPM12; http://www.fil.ion.ucl.ac.uk) with steps including bias correction, segmentation, spatial normalization using Diffeomorphic Anatomical Registration Through Exponentiated Lie Algebra (DARTEL) algorithms, Jacobian modulation, and smoothing with full width at half maximum (FWHM) kernel of 8×8×8mm3.
In order to eliminate systematic deviations in the eleven center GMVs, combat harmonization model was carried out before statistics, in which the center IDs were defined as the batch variable, and group, age, sex and total intracranial volume (TIV) were considered as biological covariates (40, 41). Then, we employed a general linear model (GLM) to construct an interaction model between the group (schizophrenia vs. healthy controls) and the volume of the amygdala subregions. This model has been frequently used to estimate the structural covariance coefficient map of a seed region across subjects, and to compare intergroup differences in their covariance coefficient at the voxel level (42, 43). Specifically, the basic idea of the GLM for structural covariance comparison in the present study is to estimate the covariate coefficients between each amygdala subregion and each other brain voxels for schizophrenia patients and healthy control groups, respectively. Then, a two-sample t-test was employed to compare whether the covariate coefficients between the two groups differed, with age, sex, and total intracranial volume (TIV) as nuisance confounders [equation (1)]:
where GMV denotes the gray matter volume per voxel, SCZ and HC represent schizophrenia and healthy controls, respectively. VolS denotes the volume of the amygdala subregion, and Covs refers to covariates that need to be controlled for. β3 and β4 signify the covariate coefficients for schizophrenia and healthy controls. The effect is the difference between these covariate coefficients, expressed as β3-β4. The assessment of whether this covariate difference is significant was performed using a non-parametric permutation-based two-sample t-test (with 5,000 permutations) and further employing threshold-free cluster enhancement (TFCE) combined with family-wise error (FWE) correction to control for false positives due to multiple comparisons at the voxel level. To further correct the multiple comparisons false positives derived by multiple subregions, we evaluated the effective numbers of independent tests Meff (6.17 times) (44), and at last, using a strict threshold for the statistics of all amygdala subregions (P< 0.05 [TFCE-FWE threshold]/6.17 = 0.0081). To clarify whether there is an advantage by sub-regional analysis, we conducted the same GLM to compare the intergroup differences in GMV covariance of the whole left and right amygdala with the same significant threshold as the subregions. Finally, to validate the stability of the covariance pattern alterations in each of the 11 datasets, we extracted the average GMV of the brain with changed covariance in each amygdala subregion and carried out ROI-wise GLM described above for each site.
Furthermore, to better observe the alteration of subregional structure, we employed a GLM model to investigate whether there were GMV differences in whole amygdala and each of its subregions between schizophrenia patients and healthy controls within both male and female subgroups, taking age and TIV as confounding confounders (P< 0.0081). Additionally, we compared whether there were inter-sex differences between the observed differences in the male group and those observed in the female group.
To reveal the amygdala subregion-specific covariance disruption patterns of schizophrenia, we extract the average GMV of brain region-of-interest (ROI) with changed covariance in at least one amygdala subregion. This procedure was performed with the following steps: (1) for each subregion’s statistic map, we generated a binary mask where intergroup differences of the GMV covariance between this subregion and voxel within the mask survived; (2) we merged these binary masks of all amygdala subregion into a union mask; (3) we identified the intersection voxels between the union mask and each AAL3 region (45); (4) we calculated the overlapping ratio between the intersection voxels and each ALL region; (5) we selected the target ROIs with the criteria: the overlapping ratio is greater than 15% the volume of overlapping region is greater than 1mm3; (6) finally, the average GMV of each target ROIs was extracted for each subject.
We then carried out ROI-wise GLM to compare the intergroup differences in GMV covariance between each amygdala subregion and target ROI. We generated fingerprints plot to demonstrate the unique structural covariance disruption patterns between amygdala subregions in schizophrenia. This plot had been applied to represent the unique spatial connectivity patterns of a specific brain subregion that differentiated it from other subregions (46, 47). Besides, we conducted a sex-specific analysis, which could provide valuable insights into whether the observed GMV covariance disruptions are differed between the females or males. We further explored whether these abnormal GMV covariance patterns were replicable across different data centers.
The clinical features included the Positive and Negative Syndrome Scale (PANSS), duration of illness, and dose of antipsychotic medication (chlorpromazine equivalent doses) (48). A Spearman correlation analysis was performed between the GMV covariance alterations and the clinical features (P< 0.05).
We finally included 807 schizophrenia patients (32.44 ± 10.34 years, 484 males) and 845 healthy controls (32.57 ± 10.47 years, 491 males). The detailed demographic information of the subjects is displayed in Table 2. There were no statistical group differences in age (F = 1.09, P = 0.79) or sex (χ2 = 0.60, P = 0.44). There were no significant differences in either sex or age between schizophrenia patients and healthy controls in each center (P > 0.05).
We found significantly increased GMV covariance patterns in five amygdala subregions, L_AAA, R_AAA, L_CAT, R_CAT and R_Ba (Figure 1). Among them, changed GMV covariance was found in both hemispheres of anterior-amygdaloid-area and corticoamygdaloid-transition with a relatively stable altered GMV covariance patterns. Both bisymmetric AAA subregions exhibited increased GMV covariance with the bilateral putamen. Additionally, the R_AAA subregion also showed increased GMV covariance with the bilateral caudate nucleus, hippocampus, and parahippocampal gyrus. The bilateral CAT subregions demonstrated the most extensive significant GMV covariance alterations, including the bilateral putamen, caudate nucleus, hippocampus, parahippocampal gyrus, olfactory cortex, anterior and middle cingulate cortex, fusiform gyrus, lingual gyrus, and superior frontal gyrus. Increased GMV covariance of R_Ba with bilateral accumbens nucleus and the left putamen.
Figure 1 Altered GMV covariance patterns of the five amygdala subregions in patients with schizophrenia and the validation across centers. The color bar indicates the intensity of altered GMV covariance in schizophrenia (t-value). The forest plots represent the effect size (also known as Hedges’ adjusted g*) for inter-group GMV covariance differences of the five amygdala subregions in each centers. L_AAA, Anterior-amygdaloid-area of left hemisphere; R_AAA, Anterior-amygdaloid-area of right hemisphere; L_CAT, Corticoamygdaloid-transitio of left hemisphere; R_CAT, Corticoamygdaloid-transitio of right hemisphere; R_Ba, Basal nucleus of right hemisphere. L, left, R, right. SCZ, Schizophrenia, HC, Healthy control.
Moreover, the trend of the altered GMV covariance patterns can be replicated in most centers, such as FE_Harbin, NFE_Tianjin1, NFE_Tianjin2, NFE_Shanghai, NFE_Wuhan, NFE_Harbin, NFE_BrainGluSchi, NFE_COBRE. Specifically, we validated the increased GMV covariance pattern of R_AAA except for the NFE_NMorphCH center and the pattern of R_CAT except for the NFE_UCLA center. However, the FE_Guangzhou and NFE_NMorphCH obtained opposite results in the subregions of L_AAA, L_CAT, and R_Ba.
In Figure 2A, we present a visualization of the amygdala subregions. In Figure 2B, we demonstrated two binary masks for amygdala subregions versus whole amygdala, allowing us to compare significant findings between amygdala subregions and the whole amygdala. We observed that the whole amygdala only showed increased GMV covariance with the bilateral thalamus and basal ganglia. In contrast, the amygdala subregion analyses revealed more distributed brain regions with increased covariance, including the frontal, occipital, and temporal lobes. Our results demonstrated that the subregional analysis could uncover more GMV covariance abnormalities and may improve our understanding of the different roles of amygdala subregions in the pathological process of schizophrenia.
Figure 2 Comparison of the altered GMV covariance between the amygdala subregions and the whole amygdala. (A) Visualization of amygdala subregions in the brain. (B) The different mask of the significant GMV covariance results for the amygdala subregions (red color) and whole amygdala (blue color). axi, axial, cor, coronal, sag, sagittal. AAA, anterior-amygdaloid-area; AB, accessory-basal nucleus; Ba, basal nucleus; CAT, corticoamygdaloid-transition; Ce, central nucleus; Co, cortical nucleus; La, lateral nucleus; Me, medial nucleus; PL, paralaminar nucleus; L, left; R, right.
At the same time, our analysis revealed that the GMV was significantly reduced in the whole amygdala across both hemispheres for both male and female subgroups of schizophrenia patients. In the male subgroup, GMV was significantly lower in all subregions except for bilateral Ce, Me and right Co. Conversely, in the female subgroup, all subregions exhibited significantly reduced GMV except for the bilateral Ce, Me, PL. There were no inter-sex differences in the observed differences between the male and female groups (P > 0.05) (Figure 3).
Figure 3 GMV alteration of the amygdala and its subregions in schizophrenia patients between the male and female subgroups. * indicates corrected by multiple comparisons. AAA, anterior-amygdaloid-area; AB, accessory-basal nucleus; Ba, basal nucleus; CAT, corticoamygdaloid-transition; Ce, central nucleus; Co, cortical nucleus; La, lateral nucleus; Me, medial nucleus; PL, paralaminar nucleus; L, left; R, right; SCZ, Schizophrenia; HC, Healthy control.
According to the target ROIs defined in the methods, we selected 28 target ROIs, including pregenual part of anterior cingulate cortex (ACCpre), subgenual part of anterior cingulate cortex (ACCsub), supra callosal part of anterior cingulate cortex (ACCsup), caudate nucleus (CAU), hippocampus (HIP), accumbens nucleus (Nacc), olfactory cortex (OLF), parahippocampal gyrus (PHG), putamen (PUT), pallidum (PAL), thalamus (THA) in the bilateral cerebral hemispheres, medial orbital part of superior frontal gyrus (PFCmed), and fusiform gyrus (FFG) in the right cerebral hemisphere, and calcarine fissure and surrounding cortex (CAL), cuneus (CUN), lingual gyrus (LING), and rectus gyrus (REC) in the left cerebral hemisphere.
To visualize the specific differential GMV covariance patterns of each amygdala subregion, we calculated the differential GMV covariance fingerprints between each amygdala subregion and the 28 target ROIs (Figure 4). Relative to the HC, the L_AAA of schizophrenia exhibited mostly strengthened covariance with bilateral PUT; R_AAA had stronger covariance with bilateral HIP in schizophrenia; L_CAT mainly showed greater covariance with the OLF_L in schizophrenia; R_CAT had higher covariance with the LING_L and Nacc_L in schizophrenia; R_Ba showed stronger covariance with the bilateral Nacc in schizophrenia. In the validation of subregional GMV covariance specificity between different centers, we found that our results were consistent in most centers to an extent (Figure 4). Specifically, the covariance pattern of L_AAA was not stable in FE_Guangzhou, and the non-first-episode centers could be verified except for Shanghai and NMorphCH. The covariance pattern of R_AAA could be validated except for NFE_BrainGluSchi and NFE_NMorphCH. The findings of L_CAT could not be validated in the FE_Guangzhou, NFE_Shanghai, and NFE_NMorphCH centers. The GMV covariance patterns of R_CAT and R_Ba were not replicated in the NFE_NMorphCH and NFE_UCLA centers.
Figure 4 GMV covariance fingerprints of the five amygdala subregions and the heat map exhibiting validation across centers. The scale value of the fingerprint graph represents the t-value of inter-group differences in GMV covariance. The colorbar of the heat map represents the Hedges’ adjusted g* of the inter-group GMV covariance fingerprints of the five amygdala subregions in each centers. ACCpre, pregenual part of anterior cingulate cortex; ACCsub, subgenual part of anterior cingulate cortex; ACCsup, supra callosal part of anterior cingulate cortex; CAL, calcarine fissure and surrounding cortex; CAU, caudate nucleus; CUN, cuneus; FFG, fusiform gyrus; HIP, hippocampus; LING, lingual gyrus; Nacc, accumbens nucleus; OFCmed, medial orbital gyrus; OLF, olfactory cortex; PAL, pallidum; PHG, parahippocampal gyrus; PUT, putamen; REC, rectus gyrus; THA, thalamus. L_AAA, Anterior-amygdaloid-area of left hemisphere; R_AAA, Anterior-amygdaloid-area of right hemisphere; L_CAT, Corticoamygdaloid-transitio of left hemisphere; R_CAT, Corticoamygdaloid-transitio of right hemisphere; R_Ba, Basal nucleus of right hemisphere.
In our sex-specific analysis experiment, we found that GMV covariance fingerprints exhibited distinct patterns across sexes, with these patterns being more pronounced in women. Specifically, in R_AAA, there was an observed trend towards sex differences in HIP_L, PHG_R, HIP_R, PFCmed_R and OLF_R (P< 0.05, uncorrected). In L_CAT, we identified sex-differentiated trends in bilateral HIP and CAU (P< 0.05, uncorrected). Similarly, in R_CAT, there was a trend towards sex differences in bilateral HIP and left THA (P< 0.05, uncorrected) (Figure 5).
Figure 5 The different GMV covariance fingerprints for five amygdala subregions in male and female subjects. * indicates trends in sex differences, uncorrected. ACCpre, pregenual part of anterior cingulate cortex; ACCsub, subgenual part of anterior cingulate cortex; ACCsup, supra callosal part of anterior cingulate cortex; CAL, calcarine fissure and surrounding cortex; CAU, caudate nucleus; CUN, cuneus; FFG, fusiform gyrus; HIP, hippocampus; LING, lingual gyrus; Nacc, accumbens nucleus; OFCmed, medial orbital gyrus; OLF, olfactory cortex; PAL, pallidum; PHG, parahippocampal gyrus; PUT, putamen; REC, rectus gyrus; THA, thalamus. L_AAA, Anterior-amygdaloid-area of left hemisphere; R_AAA, Anterior-amygdaloid-area of right hemisphere; L_CAT, Corticoamygdaloid-transitio of left hemisphere; R_CAT, Corticoamygdaloid-transitio of right hemisphere; R_Ba, Basal nucleus of right hemisphere. M, male, F, female.
A Spearman correlation analysis was performed between the GMV covariance alteration and the clinical information in schizophrenia patients. There was a weak positive association between the positive syndrome scale total score and the GMV covariance of R_AAA & CUN_L (P = 0.034, uncorrected), R_AAA & PAL_L (P = 0.026, uncorrected), R_AAA & Nacc_L (P = 0.021, uncorrected). The total score of negative syndrome scale showed a negative association with the GMV covariance of L_CAT & CAU_L (P = 0.047), L_CAT & PAL_R (P = 0.038), L_CAT & CAU_R (P = 0.023), R_CAT & ACCsup_R (P = 0.046), R_Ba & CAU_L (P = 0.016), R_Ba & CAU_R (P = 0.017). There was a weak positive association between general psychopathology scale total score with the GMV covariance of L_AAA & OLF_L (P = 0.022, uncorrected), L_AAA & REC_L (P = 0.047, uncorrected), L_AAA & Nacc_L (P = 0.041, uncorrected), L_AAA & PFCmed_R (P = 0.049, uncorrected), L_CAT & Nacc_L (P = 0.049, uncorrected), R_AAA & OLF_L (P = 0.020, uncorrected), R_AAA & CUN_L (P = 0.014, uncorrected), R_AAA & PHG_R (P = 0.046, uncorrected), R_AAA & OLF_R (P = 0.032, uncorrected), R_CAT & Nacc_L (P = 0.037, uncorrected), R_CAT & Nacc_R (P = 0.048, uncorrected), R_CAT & FFG_R (P = 0.036, uncorrected), R_Ba & CUN_L (P = 0.034, uncorrected), R_Ba & Nacc_L (P = 0.048, uncorrected). We identified no association between PANSS total score and amygdala GMV covariance patterns. We revealed a weak negative correlation between disease course information and GMV covariance of L_AAA & ACCsup_R (P = 0.39, uncorrected), L_CAT & ACCsup_R (P = 0.045, uncorrected), R_AAA & THA_L (P = 0.038, uncorrected), R_CAT & ACCsup_R (P = 0.036, uncorrected), R_Ba & ACCsup_R (P = 0.018, uncorrected). Finally, we revealed an uncorrected negative association between the CPZ with the GMV covariance of L_AAA & CUN_L (P = 0.030), L_AAA & ACCsub_L (P = 0.006), L_AAA & ACCsub_R (P = 0.007), L_CAT & ACCsub_L (P = 0.031), L_CAT & ACCpre_R (P = 0.037), L_CAT & ACCsub_R (P = 0.011), R_AAA & PAL_L (P = 0.006), R_AAA & ACCsub_R (P = 0.018), R_AAA & PAL_R (P = 0.005), R_AAA & FFG_R (P = 0.019), R_CAT & CUN_L (P = 0.008), R_CAT & PHG_R (P = 0.048), R_Ba & HIP_L (P = 0.043), R_Ba & PHG_L (P = 0.011), R_Ba & PHG_R (P = 0.014), R_Ba & HIP_R (P = 0.016).
To our knowledge, this is the first study to investigate the GMV covariance changes of the amygdala in schizophrenia at the subregion level. We used extensive data from multicenters and verified that only 5/18 amygdala subregions in schizophrenia patients showed replicable increased GMV covariance compared to healthy controls. Moreover, strengthened GMV covariance patterns are relatively unique for each of the five amygdala subregions in individuals with schizophrenia. These findings support our hypothesis that the GMV covariances of amygdala subregions are selectively impaired in schizophrenia.
Combining structural brain MRI data from 807 patients with schizophrenia and 845 healthy controls from 11 centers, we calculated the robustness of effect sizes and assessed variability across centers. Most remarkably, our study found extensive GMV covariance enhancement between five subregions of the amygdala and the frontal, occipital, and temporal cortex and in SCZ patients, which is consistent with the hypothesis that SCZ is a widespread dysconnection disorder (8, 49, 50). The enhanced GMV covariance in SCZ patients may indicate coordinated GMV loss throughout neurodevelopment (51, 52). In fact, schizophrenia patients also exhibits widespread atrophy in amygdala, frontal, occipital, temporal cortex, and basal ganglia, which may be caused by excessive synaptic pruning during adolescence (53, 54). We speculated that the GMV covariance perturbations between amygdala subregions and other brain areas may result from shared common causal factors. Future studies are expected to focus on the effects of genetic and environmental factors on the impairment of amygdala subregions’ structure and their covariance, which may provide new perspectives to unravel the etiology and mechanisms of amygdala damage in schizophrenia (55, 56).
Previous postmortem investigations in schizophrenia patients reported reduced mean total neuron number in the lateral nucleus of the amygdala (31), as well as alterations in the nuclear area, nucleolar volume (29), and oligodendrocyte density (57) in the basolateral complex. Recent in vivo studies have shown that all amygdala nuclei have reduced in size (30) except the medial nucleus, and primarily impaired amygdala nuclei are the basal and lateral nuclei (22) or the right basolateral complex (58), supporting our findings with regional atrophy of amygdala subfields.
Moreover, we found significantly increased GMV covariance patterns in five amygdala subregions in schizophrenia patients, including the right basal nucleus, bilateral anterior-amygdaloid-area, and bilateral bilateral corticoamygdaloid-transition. The basal nucleus, a part of the basolateral complex, undergoes marked atrophy in schizophrenia (59). The basal nucleus receives input from the lateral nucleus and transmits signals to the central nucleus, which is involved in the expression and extinction of fear (60). And has extensive connections with ventral striatal areas and the orbital prefrontal cortex (61), which are important in integrating, coordinating, and processing of external sensory input (27, 62). It has been demonstrated that the basal amygdala has strong functional connectivity with the regions of the ventral caudal, medial frontal, and caudal orbitofrontal cortex. Functional connectivity between the accumbens nucleus (Nacc) and the basal amygdala is positively associated with negative emotions (6, 63). Simultaneously, we observed a significant enhanced GMV covariance pattern between the right basal nucleus and Nacc/OLF. Thus, the GMV covariance abnormalities in the basolateral complex (consisting of the basal, lateral, and accessory basal nuclei) may be linked to dysfunctional emotional regulation and ensuing deficiencies in adaptive behavior in schizophrenia.
We also found the corticoamygdaloid-transition area (CAT) had increased GMV covariance with the hippocampus, parahippocampal gyrus, olfactory cortex, and anterior cingulate cortex. The hippocampus, as one of the prominently affected brain areas in the pathogenesis of schizophrenia, is a core region for learning and episodic memory (64). There is evidence that CAT receives inputs from the hippocampus and outputs the emotional context of memories to inferior neurons (63, 65). Hence, disrupting connections between the amygdala and hippocampus may affect memories of emotionally relevant events in schizophrenia (29, 66). Additional studies have demonstrated a negative correlation between CAT volume and salivary cortisol (67). Stress is one of the main factors contributing to the production of cortisol, and this stress exposure greatly increases the risk of schizophrenia (68, 69). Preliminary evidence suggests that the CAT is involved in assessing negative emotions (70). Deficits in facial emotion interpretation and social skills in schizophrenic patients may stem from a reduction in the volume of the CAT (30). Our finding provides additional evidence for its involvement in emotional processing. Finally, athough there is evidence of a significant reduction in GMV of AAA in schizophrenia patients (30), it is difficult to draw conclusions regarding the involvement of AAA in schizophrenia since little is known about its connections and functions.
We further conducted a sex-specific analysis and found that there were sex differences in GMV covariance fingerprints, the female was more pronounced. However, the sample size of the male and female groups was different. The sex differences in GMV covariance in schizophrenia patients can be attributed to several biological, genetic, and hormonal factors that interact with the disease’s pathophysiology (71–73). Understanding these differences was crucial for developing sex-specific approaches to treatment and management, which needs further exploration.
A large number of previous studies have shown varying degrees of correlation between reduced GMV in brain regions and negative or positive symptoms in patients with schizophrenia (57, 74). However, we did not obtain significant results in our study on the correlation between the altered GMV covariance and clinical symptoms, consistent with the findings of Spreng et al (75). Investigating the lack of correlation between GMV covariance alterations and the clinical features in schizophrenia patients offers a nuanced view into the complexities of the disease. This phenomenon can be attributed to several factors: 1) Schizophrenia is highly heterogeneous (2), with significant variability in pathology and progression among individuals. This heterogeneity might obscure any consistent correlation between GMV covariance alterations and clinical features across a broad sample of patients; 2) Different stages of schizophrenia (e.g., early vs. late) may impact brain structure in varying ways, and the progression rate can differ among individuals. Clinical features capture symptoms at a specific point in time, whereas GMV covariance alterations might reflect the cumulative effects of the disease, making synchronous correlation unlikely (20, 76). We propose that our findings, while preliminary, have the potential to be an effective imaging marker for differentiating schizophrenia from healthy populations and open avenues for future research. However, further studies are still needed to validate its differentiation value. We validated the GMV covariance model separately for the data between each center, and consistent results were obtained at most centers. Validation was not obtained from all centers, and we argued that the possible reasons included inconsistency in sample size between centers, differences in MRI models and scanning parameters between centers, and diversity in the causes of patient morbidity (77–79).
There are some limitations in our study. First, due to a lack of information on the PANSS, illness history, and antipsychotic medication usage, it was not feasible to determine if the altered GMV covariance patterns were correlated with the existence of clinical measures. Second, the etiology and biological pathways of GMV covariation in the amygdala subregion of schizophrenia are still unknown.
In conclusion, our study discovered a selective disruption of GMV covariance of amygdala subregions in a large sample of schizophrenia patients for the first time. We validated the reproducibility of our findings in 11 centers containing first-episode and non-first-episode schizophrenia patients. Further study is needed to disentangle the biological mechanisms and clinical significance of perturbed GMV covariance of amygdala subregions.
The original contributions presented in the study are included in the article/Supplementary Material, further inquiries can be directed to the corresponding author.
The studies involving humans were approved by the Ethics Committee of Tianjin Medical University General Hospital, Tianjin, China. The patients/participants provided their written informed consent to participate in this study. Relevant Institutional Review Boards also approved the four public test-retest datasets, and detailed recruitment information was provided on the website. The studies were conducted in accordance with the local legislation and institutional requirements. Written informed consent for participation was not required from the participants or the participants’ legal guardians/next of kin in accordance with the national legislation and institutional requirements.
ZC: Formal analysis, Visualization, Writing – original draft, Writing – review & editing. LL: Data curation, Writing – original draft. LL: Data curation, Writing – original draft. GW: Data curation, Writing – original draft. CZ: Data curation, Writing – original draft. HT: Data curation, Writing – original draft. WL: Data curation, Writing – original draft. LW: Data curation, Writing – original draft. BZ: Data curation, Writing – original draft. JR: Data curation, Writing – original draft. YZ: Formal analysis, Data curation, Writing – original draft. YX: Data curation, Writing – original draft. XD: Data curation, Writing – original draft. XW: Data curation, Writing – original draft. LW: Data curation, Writing – original draft. YL: Data curation, Writing – original draft. HD: Data curation, Writing – original draft. XL: Data curation, Writing – original draft. ZZ: Data curation, Writing – original draft. ML: Funding acquisition, Writing – original draft. CZ: Data curation, Writing – original draft. XW: Data curation, Writing – review & editing. CY: Funding acquisition, Writing – review & editing. WQ: Methodology, Funding acquisition, Writing – review & editing. HL: Methodology, Funding acquisition, Writing – review & editing.
The author(s) declare financial support was received for the research, authorship, and/or publication of this article. This work was supported by the National Natural Science Foundation of China (81971599, 81771818, 82030053, and 81971694), National Key Research and Development Program of China (2018YFC1314300), Tianjin Natural Science Foundation (19JCYBJC25100, 21JCYBJC01370), and Tianjin Key Medical Discipline (Specialty) Construction Project (TJYXZDXK-001A).
The authors would like to acknowledge the staff of the Tianjin Key Laboratory of Functional Imaging and Tianjin Medical University General Hospital for technical and administrative support, commentary, and advice.
The authors declare that the research was conducted without any commercial or financial relationships that could be construed as a potential conflict of interest.
All claims expressed in this article are solely those of the authors and do not necessarily represent those of their affiliated organizations, or those of the publisher, the editors and the reviewers. Any product that may be evaluated in this article, or claim that may be made by its manufacturer, is not guaranteed or endorsed by the publisher.
The Supplementary Material for this article can be found online at: https://www.frontiersin.org/articles/10.3389/fpsyt.2024.1349989/full#supplementary-material
1. Ferrari AJ, Santomauro DF, Mantilla Herrera AM, Shadid J, Ashbaugh C, Erskine HE, et al. Global, regional, and national burden of 12 mental disorders in 204 countries and territories, 1990-2019: a systematic analysis for the Global Burden of Disease Study 2019. Lancet Psychiatry. (2022) 9:137–50. doi: 10.1016/s2215-0366(21)00395-3
2. Jauhar S, Johnstone M, Mckenna PJ. Schizophrenia. Lancet. (2022) 399:473–86. doi: 10.1016/S0140-6736(21)01730-X
3. Zhou Y, Fan L, Qiu C, Jiang T. Prefrontal cortex and the dysconnectivity hypothesis of schizophrenia. Neurosci Bull. (2015) 31:207–19. doi: 10.1007/s12264-014-1502-8
4. Heckers S. Neuroimaging studies of the hippocampus in schizophrenia. Hippocampus. (2001) 11:520–8. doi: 10.1002/hipo.1068
5. Friston KJ, Frith CD. Schizophrenia: a disconnection syndrome? Clin Neurosci (New York NY). (1995) 3:89–97.
6. Klein-Flugge MC, Jensen DEA, Takagi Y, Priestley L, Verhagen L, Smith SM, et al. Relationship between nuclei-specific amygdala connectivity and mental health dimensions in humans. Nat Hum Behav. (2022) 6:1705–22. doi: 10.1038/s41562-022-01434-3
7. Ho NF, Li Hui Chong P, Lee DR, Chew QH, Chen G, Sim K. The amygdala in schizophrenia and bipolar disorder: A synthesis of structural MRI, diffusion tensor imaging, and resting-state functional connectivity findings. Harvard Rev Psychiatry. (2019) 27:150–64. doi: 10.1097/HRP.0000000000000207
8. Amaral DG, Price JL. Amygdalo-cortical projections in the monkey (Macaca fascicularis). J Comp Neurol. (1984) 230:465–96. doi: 10.1002/cne.902300402
9. Mcklveen JM, Myers B, Herman JP. The medial prefrontal cortex: coordinator of autonomic, neuroendocrine and behavioural responses to stress. J Neuroendocrinol. (2015) 27:446–56. doi: 10.1111/jne.12272
10. Sotres-Bayon F, Sierra-Mercado D, Pardilla-Delgado E, Quirk GJ. Gating of fear in prelimbic cortex by hippocampal and amygdala inputs. Neuron. (2012) 76:804–12. doi: 10.1016/j.neuron.2012.09.028
11. Laviolette SR, Lipski WJ, Grace AA. A subpopulation of neurons in the medial prefrontal cortex encodes emotional learning with burst and frequency codes through a dopamine D4 receptor-dependent basolateral amygdala input. J neuroscience: Off J Soc Neurosci. (2005) 25:6066–75. doi: 10.1523/jneurosci.1168-05.2005
12. Correll CM, Rosenkranz JA, Grace AA. Chronic cold stress alters prefrontal cortical modulation of amygdala neuronal activity in rats. Biol Psychiatry. (2005) 58:382–91. doi: 10.1016/j.biopsych.2005.04.009
13. Shin LM, Orr SP, Carson MA, Rauch SL, Macklin ML, Lasko NB, et al. Regional cerebral blood flow in the amygdala and medial prefrontal cortex during traumatic imagery in male and female Vietnam veterans with PTSD. Arch Gen Psychiatry. (2004) 61:168–76. doi: 10.1001/archpsyc.61.2.168
14. Pitkänen A, Pikkarainen M, Nurminen N, Ylinen A. Reciprocal connections between the amygdala and the hippocampal formation, perirhinal cortex, and postrhinal cortex in rat. A review. Ann New York Acad Sci. (2000) 911:369–91. doi: 10.1111/j.1749-6632.2000.tb06738.x
15. Okuyama T, Kitamura T, Roy DS, Itohara S, Tonegawa S. Ventral CA1 neurons store social memory. Sci (New York NY). (2016) 353:1536–41. doi: 10.1126/science.aaf7003
16. Ortiz S, Latsko MS, Fouty JL, Dutta S, Adkins JM, Jasnow AM. Anterior cingulate cortex and ventral hippocampal inputs to the basolateral amygdala selectively control generalized fear. J neuroscience: Off J Soc Neurosci. (2019) 39:6526–39. doi: 10.1523/jneurosci.0810-19.2019
17. Seidman LJ, Faraone SV, Goldstein JM, Goodman JM, Kremen WS, Toomey R, et al. Thalamic and amygdala-hippocampal volume reductions in first-degree relatives of patients with schizophrenia: an MRI-based morphometric analysis. Biol Psychiatry. (1999) 46:941–54. doi: 10.1016/S0006-3223(99)00075-X
18. De Zwarte SMC, Brouwer RM, Tsouli A, Cahn W, Hillegers MHJ, Hulshoff Pol HE, et al. Running in the family? Structural brain abnormalities and IQ in offspring, siblings, parents, and co-twins of patients with schizophrenia. Schizophr Bull. (2019) 45:1209–17. doi: 10.1093/schbul/sby182
19. Kim JY, Jeon H, Kwon A, Jin MJ, Lee SH, Chung YC. Self-awareness of psychopathology and brain volume in patients with first episode psychosis. Front Psychiatry. (2019) 10:839. doi: 10.3389/fpsyt.2019.00839
20. Wang X, Yin Z, Sun Q, Jiang X, Chao L, Dai X, et al. Comparative study on the functional connectivity of amygdala and hippocampal neural circuits in patients with first-episode schizophrenia and other high-risk populations. Front Psychiatry. (2021) 12:627198. doi: 10.3389/fpsyt.2021.627198
21. Hoptman MJ, D’angelo D, Catalano D, Mauro CJ, Shehzad ZE, Kelly AM, et al. Amygdalofrontal functional disconnectivity and aggression in schizophrenia. Schizophr Bull. (2010) 36:1020–8. doi: 10.1093/schbul/sbp012
22. Jalbrzikowski M, Murty VP, Tervo-Clemmens B, Foran W, Luna B. Age-associated deviations of amygdala functional connectivity in youths with psychosis spectrum disorders: relevance to psychotic symptoms. Am J Psychiatry. (2019) 176:196–207. doi: 10.1176/appi.ajp.2018.18040443
23. Stegmayer K, Bohlhalter S, Vanbellingen T, Federspiel A, Wiest R, Muri RM, et al. Limbic interference during social action planning in schizophrenia. Schizophr Bull. (2018) 44:359–68. doi: 10.1093/schbul/sbx059
24. Guo H, Ye H, Li Z, Li X, Huang W, Yang Y, et al. Amygdala signal abnormality and cognitive impairment in drug-naive schizophrenia. BMC Psychiatry. (2023) 23:231. doi: 10.1186/s12888-023-04728-6
25. Wen D, Wang J, Yao G, Liu S, Li X, Li J, et al. Abnormality of subcortical volume and resting functional connectivity in adolescents with early-onset and prodromal schizophrenia. J Psychiatr Res. (2021) 140:282–8. doi: 10.1016/j.jpsychires.2021.05.052
26. Zhang M, Yang F, Fan F, Wang Z, Hong X, Tan Y, et al. Abnormal amygdala subregional-sensorimotor connectivity correlates with positive symptom in schizophrenia. NeuroImage Clin. (2020) 26:102218. doi: 10.1016/j.nicl.2020.102218
27. Sah P, Faber ES, Lopez De Armentia M, Power J. The amygdaloid complex: anatomy and physiology. Physiol Rev. (2003) 83:803–34. doi: 10.1152/physrev.00002.2003
28. Johansen JP, Cain CK, Ostroff LE, Ledoux JE. Molecular mechanisms of fear learning and memory. Cell. (2011) 147:509–24. doi: 10.1016/j.cell.2011.10.009
29. Phelps EA, Delgado MR, Nearing KI, Ledoux JE. Extinction learning in humans: role of the amygdala and vmPFC. Neuron. (2004) 43:897–905. doi: 10.1016/j.neuron.2004.08.042
30. Barth C, Nerland S, De Lange AG, Wortinger LA, Hilland E, Andreassen OA, et al. In vivo amygdala nuclei volumes in schizophrenia and bipolar disorders. Schizophr Bull. (2021) 47:1431–41. doi: 10.1093/schbul/sbaa192
31. Prasad K, Rubin J, Mitra A, Lewis M, Theis N, Muldoon B, et al. Structural covariance networks in schizophrenia: A systematic review Part I. Schizophr Res. (2022) 240:1–21. doi: 10.1016/j.schres.2021.11.035
32. Van Den Heuvel MP, Fornito A. Brain networks in schizophrenia. Neuropsychol Rev. (2014) 24:32–48. doi: 10.1007/s11065-014-9248-7
33. Prasad K, Rubin J, Mitra A, Lewis M, Theis N, Muldoon B, et al. Structural covariance networks in schizophrenia: A systematic review Part II. Schizophr Res. (2022) 239:176–91. doi: 10.1016/j.schres.2021.11.036
34. Tesli N, van der Meer D, Rokicki J, Storvestre G, Røsæg C, Jensen A, et al. Hippocampal subfield and amygdala nuclei volumes in schizophrenia patients with a history of violence. Eur Arch Psychiatry Clin Neurosci. (2020) 270:771–82. doi: 10.1007/s00406-020-01098-y
35. Solano-Castiella E, Anwander A, Lohmann G, Weiss M, Docherty C, Geyer S, et al. Diffusion tensor imaging segments the human amygdala in vivo. Neuroimage. (2010) 49:2958–65. doi: 10.1016/j.neuroimage.2009.11.027
36. Amunts K, Kedo O, Kindler M, Pieperhoff P, Mohlberg H, Shah NJ, et al. Cytoarchitectonic mapping of the human amygdala, hippocampal region and entorhinal cortex: intersubject variability and probability maps. Anat Embryol (Berl). (2005) 210:343–52. doi: 10.1007/s00429-005-0025-5
37. Saygin ZM, Osher DE, Augustinack J, Fischl B, Gabrieli JD. Connectivity-based segmentation of human amygdala nuclei using probabilistic tractography. Neuroimage. (2011) 56:1353–61. doi: 10.1016/j.neuroimage.2011.03.006
38. Saygin ZM, Osher DE, Koldewyn K, Martin RE, Finn A, Saxe R, et al. Structural connectivity of the developing human amygdala. PloS One. (2015) 10:e0125170. doi: 10.1371/journal.pone.0125170
39. Saygin ZM, Kliemann D, Iglesias JE, van der Kouwe AJW, Boyd E, Reuter M, et al. High-resolution magnetic resonance imaging reveals nuclei of the human amygdala: manual segmentation to automatic atlas. Neuroimage. (2017) 155:370–82. doi: 10.1016/j.neuroimage.2017.04.046
40. Reynolds M, Chaudhary T, Eshaghzadeh Torbati M, Tudorascu DL, Batmanghelich K. ComBat Harmonization: Empirical Bayes versus fully Bayes approaches. NeuroImage Clin. (2023) 39:103472. doi: 10.1016/j.nicl.2023.103472
41. Wang YW, Chen X, Yan CG. Comprehensive evaluation of harmonization on functional brain imaging for multisite data-fusion. NeuroImage. (2023) 274:120089. doi: 10.1016/j.neuroimage.2023.120089
42. Seeley WW, Crawford RK, Zhou J, Miller BL, Greicius MD. Neurodegenerative diseases target large-scale human brain networks. Neuron. (2009) 62:42–52. doi: 10.1016/j.neuron.2009.03.024
43. Mechelli A, Friston KJ, Frackowiak RS, Price CJ. Structural covariance in the human cortex. J Neurosci. (2005) 25:8303–10. doi: 10.1523/JNEUROSCI.0357-05.2005
44. Li MX, Yeung JM, Cherny SS, Sham PC. Evaluating the effective numbers of independent tests and significant p-value thresholds in commercial genotyping arrays and public imputation reference datasets. Hum Genet. (2012) 131:747–56. doi: 10.1007/s00439-011-1118-2
45. Rolls ET, Huang CC, Lin CP, Feng J, Joliot M. Automated anatomical labelling atlas 3. Neuroimage. (2020) 206:116189. doi: 10.1016/j.neuroimage.2019.116189
46. Fan L, Wang J, Zhang Y, Han W, Yu C, Jiang T. Connectivity-based parcellation of the human temporal pole using diffusion tensor imaging. Cereb Cortex. (2014) 24:3365–78. doi: 10.1093/cercor/bht196
47. Kim JH, Lee JM, Jo HJ, Kim SH, Lee JH, Kim ST, et al. Defining functional SMA and pre-SMA subregions in human MFC using resting state fMRI: functional connectivity-based parcellation method. Neuroimage. (2010) 49:2375–86. doi: 10.1016/j.neuroimage.2009.10.016
48. Gardner DM, Murphy AL, O’donnell H, Centorrino F, Baldessarini RJ. International consensus study of antipsychotic dosing. Am J Psychiatry. (2010) 167:686–93. doi: 10.1176/appi.ajp.2009.09060802
49. Minzenberg MJ, Laird AR, Thelen S, Carter CS, Glahn DC. Meta-analysis of 41 functional neuroimaging studies of executive function in schizophrenia. Arch Gen Psychiatry. (2009) 66:811–22. doi: 10.1001/archgenpsychiatry.2009.91
50. Ragland JD, Laird AR, Ranganath C, Blumenfeld RS, Gonzales SM, Glahn DC. Prefrontal activation deficits during episodic memory in schizophrenia. Am J Psychiatry. (2009) 166:863–74. doi: 10.1176/appi.ajp.2009.08091307
51. Gogtay N, Giedd JN, Lusk L, Hayashi KM, Greenstein D, Vaituzis AC, et al. Dynamic mapping of human cortical development during childhood through early adulthood. Proc Natl Acad Sci USA. (2004) 101:8174–9. doi: 10.1073/pnas.0402680101
52. Alexander-Bloch A, Giedd JN, Bullmore E. Imaging structural co-variance between human brain regions. Nat Rev Neurosci. (2013) 14:322–36. doi: 10.1038/nrn3465
53. Gogtay N, Vyas NS, Testa R, Wood SJ, Pantelis C. Age of onset of schizophrenia: perspectives from structural neuroimaging studies. Schizophr Bull. (2011) 37:504–13. doi: 10.1093/schbul/sbr030
54. Mcglashan TH, Hoffman RE. Schizophrenia as a disorder of developmentally reduced synaptic connectivity. Arch Gen Psychiatry. (2000) 57:637–48. doi: 10.1001/archpsyc.57.7.637
55. Wright IC, Sham P, Murray RM, Weinberger DR, Bullmore ET. Genetic contributions to regional variability in human brain structure: methods and preliminary results. Neuroimage. (2002) 17:256–71. doi: 10.1006/nimg.2002.1163
56. Pezawas L, Meyer-Lindenberg A, Drabant EM, Verchinski BA, Munoz KE, Kolachana BS, et al. 5-HTTLPR polymorphism impacts human cingulate-amygdala interactions: a genetic susceptibility mechanism for depression. Nat Neurosci. (2005) 8:828–34. doi: 10.1038/nn1463
57. Zhao C, Zhu J, Liu X, Pu C, Lai Y, Chen L, et al. Structural and functional brain abnormalities in schizophrenia: A cross-sectional study at different stages of the disease. Prog Neuropsychopharmacol Biol Psychiatry. (2018) 83:27–32. doi: 10.1016/j.pnpbp.2017.12.017
58. Zheng F, Li C, Zhang D, Cui D, Wang Z, Qiu J. Study on the sub-regions volume of hippocampus and amygdala in schizophrenia. Quant Imaging Med Surg. (2019) 9:1025–36. doi: 10.21037/qims.2019.05.21
59. Mahon PB, Lee DS, Trinh H, Tward D, Miller MI, Younes L, et al. Morphometry of the amygdala in schizophrenia and psychotic bipolar disorder. Schizophr Res. (2015) 164:199–202. doi: 10.1016/j.schres.2015.02.011
60. Amano T, Duvarci S, Popa D, Paré D. The fear circuit revisited: contributions of the basal amygdala nuclei to conditioned fear. J neuroscience: Off J Soc Neurosci. (2011) 31:15481–9. doi: 10.1523/jneurosci.3410-11.2011
61. Saddoris MP, Gallagher M, Schoenbaum G. Rapid associative encoding in basolateral amygdala depends on connections with orbitofrontal cortex. Neuron. (2005) 46:321–31. doi: 10.1016/j.neuron.2005.02.018
62. Bzdok D, Laird AR, Zilles K, Fox PT, Eickhoff SB. An investigation of the structural, connectional, and functional subspecialization in the human amygdala. Hum Brain Mapp. (2013) 34:3247–66. doi: 10.1002/hbm.22138
63. Kirstein CF, Güntürkün O, Ocklenburg S. Ultra-high field imaging of the amygdala - A narrative review. Neurosci Biobehav Rev. (2023) 152:105245. doi: 10.1016/j.neubiorev.2023.105245
64. Lieberman JA, Girgis RR, Brucato G, Moore H, Provenzano F, Kegeles L, et al. Hippocampal dysfunction in the pathophysiology of schizophrenia: a selective review and hypothesis for early detection and intervention. Mol Psychiatry. (2018) 23:1764–72. doi: 10.1038/mp.2017.249
65. Fudge JL, Tucker T. Amygdala projections to central amygdaloid nucleus subdivisions and transition zones in the primate. Neuroscience. (2009) 159:819–41. doi: 10.1016/j.neuroscience.2009.01.013
66. Vuilleumier P, Armony JL, Driver J, Dolan RJ. Effects of attention and emotion on face processing in the human brain: an event-related fMRI study. Neuron. (2001) 30:829–41. doi: 10.1016/s0896-6273(01)00328-2
67. Roddy D, Kelly JR, Farrell C, Doolin K, Roman E, Nasa A, et al. Amygdala substructure volumes in Major Depressive Disorder. NeuroImage Clin. (2021) 31:102781. doi: 10.1016/j.nicl.2021.102781
68. Walter EE, Fernandez F, Snelling M, Barkus E. Stress induced cortisol release and schizotypy. Psychoneuroendocrinology. (2018) 89:209–15. doi: 10.1016/j.psyneuen.2018.01.012
69. Shah JL, Malla AK. Much ado about much: stress, dynamic biomarkers and HPA axis dysregulation along the trajectory to psychosis. Schizophr Res. (2015) 162:253–60. doi: 10.1016/j.schres.2015.01.010
70. Kilts CD, Egan G, Gideon DA, Ely TD, Hoffman JM. Dissociable neural pathways are involved in the recognition of emotion in static and dynamic facial expressions. NeuroImage. (2003) 18:156–68. doi: 10.1006/nimg.2002.1323
71. Stauffer EM, Bethlehem R, Dorfschmidt L, Won H, Warrier V, Bullmore ET. The genetic relationships between brain structure and schizophrenia. Nat Commun. (2023) 14:7820. doi: 10.1038/s41467-023-43567-7
72. Brand BA, De Boer JN, Sommer IEC. Estrogens in schizophrenia: progress, current challenges and opportunities. Curr Opin Psychiatry. (2021) 34:228–37. doi: 10.1097/yco.0000000000000699
73. Mendrek A, Mancini-Marïe A. Sex/gender differences in the brain and cognition in schizophrenia. Neurosci Biobehav Rev. (2016) 67:57–78. doi: 10.1016/j.neubiorev.2015.10.013
74. Lui S, Deng W, Huang X, Jiang L, Ma X, Chen H, et al. Association of cerebral deficits with clinical symptoms in antipsychotic-naive first-episode schizophrenia: an optimized voxel-based morphometry and resting state functional connectivity study. Am J Psychiatry. (2009) 166:196–205. doi: 10.1176/appi.ajp.2008.08020183
75. Spreng RN, Dupre E, Ji JL, Yang G, Diehl C, Murray JD, et al. Structural covariance reveals alterations in control and salience network integrity in chronic schizophrenia. Cereb Cortex (New York NY: 1991). (2019) 29:5269–84. doi: 10.1093/cercor/bhz064
76. Chen Z, Deng W, Gong Q, Huang C, Jiang L, Li M, et al. Extensive brain structural network abnormality in first-episode treatment-naive patients with schizophrenia: morphometrical and covariation study. psychol Med. (2014) 44:2489–501. doi: 10.1017/S003329171300319X
77. Garcia-Dias R, Scarpazza C, Baecker L, Vieira S, Pinaya WHL, Corvin A, et al. Neuroharmony: A new tool for harmonizing volumetric MRI data from unseen scanners. Neuroimage. (2020) 220:117127. doi: 10.1016/j.neuroimage.2020.117127
78. Fortin JP, Parker D, Tunc B, Watanabe T, Elliott MA, Ruparel K, et al. Harmonization of multi-site diffusion tensor imaging data. Neuroimage. (2017) 161:149–70. doi: 10.1016/j.neuroimage.2017.08.047
Keywords: schizophrenia, amygdala subregions, gray matter volume, structural covariance, magnetic resonance imaging
Citation: Chang Z, Liu L, Lin L, Wang G, Zhang C, Tian H, Liu W, Wang L, Zhang B, Ren J, Zhang Y, Xie Y, Du X, Wei X, Wei L, Luo Y, Dong H, Li X, Zhao Z, Liang M, Zhang C, Wang X, Yu C, Qin W and Liu H (2024) Selective disrupted gray matter volume covariance of amygdala subregions in schizophrenia. Front. Psychiatry 15:1349989. doi: 10.3389/fpsyt.2024.1349989
Received: 05 December 2023; Accepted: 11 April 2024;
Published: 29 April 2024.
Edited by:
Ali Saffet Gonul, Ege University, TürkiyeReviewed by:
Hao Yan, Peking University Institute of Mental Health, ChinaCopyright © 2024 Chang, Liu, Lin, Wang, Zhang, Tian, Liu, Wang, Zhang, Ren, Zhang, Xie, Du, Wei, Wei, Luo, Dong, Li, Zhao, Liang, Zhang, Wang, Yu, Qin and Liu. This is an open-access article distributed under the terms of the Creative Commons Attribution License (CC BY). The use, distribution or reproduction in other forums is permitted, provided the original author(s) and the copyright owner(s) are credited and that the original publication in this journal is cited, in accordance with accepted academic practice. No use, distribution or reproduction is permitted which does not comply with these terms.
*Correspondence: Huaigui Liu, aHVhaWd1aTA5MTNAMTYzLmNvbQ==; Wen Qin, cWlud2VuQHRtdS5lZHUuY24=; Chunshui Yu, Y2h1bnNodWl5dUB0bXUuZWR1LmNu; Xijin Wang, aGlnZ2luc3dAMTYzLmNvbQ==
†These authors have contributed equally to this work
Disclaimer: All claims expressed in this article are solely those of the authors and do not necessarily represent those of their affiliated organizations, or those of the publisher, the editors and the reviewers. Any product that may be evaluated in this article or claim that may be made by its manufacturer is not guaranteed or endorsed by the publisher.
Research integrity at Frontiers
Learn more about the work of our research integrity team to safeguard the quality of each article we publish.