- 1School of Psychology, Murdoch University, Murdoch, WA, Australia
- 2Centre for Molecular Medicine and Innovative Therapeutics, Health Futures Institute, Murdoch University, Murdoch, WA, Australia
- 3War Related Illness and Injury Study Center (WRIISC), Veterans Affairs Palo Alto Health Care System, Palo Alto, CA, United States
- 4Department of Psychiatry and Behavioral Sciences, Stanford University School of Medicine, Stanford, CA, United States
- 5Mental Illness Research, Education and Clinical Center (MIRECC), Veterans Affairs Palo Alto Health Care System, Palo Alto, CA, United States
Introduction: High variability in response and retention rates for posttraumatic stress disorder (PTSD) treatment highlights the need to identify "personalized" or "precision" medicine factors that can inform optimal intervention selection before an individual commences treatment. In secondary analyses from a non-inferiority randomized controlled trial, behavioral and physiological emotion regulation were examined as non-specific predictors (that identify which individuals are more likely to respond to treatment, regardless of treatment type) and treatment moderators (that identify which treatment works best for whom) of PTSD outcome.
Methods: There were 85 US Veterans with clinically significant PTSD symptoms randomized to 6 weeks of either cognitive processing therapy (CPT; n = 44) or a breathing-based yoga practice (Sudarshan kriya yoga; SKY; n = 41). Baseline self-reported emotion regulation (Difficulties in Emotion Regulation Scale) and heart rate variability (HRV) were assessed prior to treatment, and self-reported PTSD symptoms were assessed at baseline, end-of-treatment, 1-month follow-up, and 1-year follow-up.
Results: Greater baseline deficit in self-reported emotional awareness (similar to alexithymia) predicted better overall PTSD improvement in both the short- and long-term, following either CPT or SKY. High self-reported levels of emotional response non-acceptance were associated with better PTSD treatment response with CPT than with SKY. However, all significant HRV indices were stronger moderators than all self-reported emotion regulation scales, both in the short- and long-term. Veterans with lower baseline HRV had better PTSD treatment response with SKY, whereas Veterans with higher or average-to-high baseline HRV had better PTSD treatment response with CPT.
Conclusions: To our knowledge, this is the first study to examine both self-reported emotion regulation and HRV, within the same study, as both non-specific predictors and moderators of PTSD treatment outcome. Veterans with poorer autonomic regulation prior to treatment had better PTSD outcome with a yoga-based intervention, whereas those with better autonomic regulation did better with a trauma-focused psychological therapy. Findings show potential for the use of HRV in clinical practice to personalize PTSD treatment.
Clinical trial registration: ClinicalTrials.gov identifier, NCT02366403
Introduction
Posttraumatic stress disorder (PTSD) is a debilitating mental health condition that can develop following exposure to a traumatic event (1). The efficacy of several trauma-focused therapies for PTSD is well-established (2), across cognitive processing therapy (CPT), prolonged exposure therapy, imaginal exposure, eye-movement desensitization and reprocessing, and trauma-focused cognitive behavioral therapy (CBT) (3–8). Yet, there is significant variability in PTSD treatment response (as well as what denotes response; 9): systematic reviews and meta-analyses of randomized controlled trials (RCTs) suggest that clinically meaningful symptom reduction is achieved in 49%–70% of individuals whereas 60%–72% still retain a PTSD diagnosis posttreatment (10) and anywhere from 0% to 50% are classified as non-responders (11). Furthermore, treatment dropout can be as high as 50% (11) or 60% (2) and is significantly higher among military/defense personnel who have experienced military trauma, especially for trauma-focused therapy (12).
Variability in PTSD treatment response and retention highlights the need to identify “personalized” or “precision” medicine factors that can identify the most optimal intervention before an individual commences treatment. These factors can be considered treatment moderators, which are pre-intervention (baseline) characteristics that alter the treatment effect and identify for whom an intervention works (or does not) (13, 14). Emotion regulation (ER)—the awareness and understanding of, and adaptive responding to, emotional experiences (15–18)—is a promising candidate as a treatment moderator. ER is a transdiagnostic process underlying several emotional-based mental health disorders like PTSD (16, 19) and improves significantly with CBT for these disorders (18). While PTSD is sometimes underrepresented in these systematic reviews and meta-analyses, we recently confirmed that self-reported ER improves with CPT for PTSD (20). Yet, few studies have examined if ER acts as a treatment moderator. One study failed to confirm that ER predicts CBT outcome for anxiety disorders (21). Another recent study of treatment-seeking adults with transdiagnostic emotional symptoms (diagnostic breakdown unavailable) found that higher self-reported use of maladaptive ER strategies at baseline significantly predicted greater improvement in symptoms of anxiety and depression following CBT (22). Yet, these effects are more akin to those of a non-specific predictor: a baseline factor that determines which individuals are more likely to respond to treatment, regardless of treatment type (13). In contrast, true treatment moderators more precisely identify which treatment works best for whom (13, 14). To our knowledge, only one study has demonstrated ER acting as a true treatment moderator: for individuals with co-occurring PTSD and substance use disorder (SUD), those who had more overall self-reported ER difficulties at baseline had better PTSD outcome with a combined CBT for PTSD plus CBT for SUD, whereas those who had fewer baseline ER difficulties had better SUD outcome with CBT for SUD only (23). Further research is clearly needed.
As self-report measures are associated with measurement bias (24, 25), there is value in supplementing self-reported ER with objective biomarkers of ER. One biomarker that is relatively quick, easy, and inexpensive—and could therefore be translatable to clinical practice—is heart rate variability (HRV). HRV is a measure of the differences in the time interval between adjacent heart beats (26, 27) and is considered a well-validated biomarker of ER in both healthy (28, 29) and clinical populations (30), including PTSD (31, 32). Generally, lower HRV is associated with difficulties in ER and poorer mental and physical health while higher HRV is associated with more adaptive regulation and healthier functioning overall (28–30, 33, 34). Two adult studies found that baseline HRV was a significant non-specific predictor of CBT outcome, although the direction of effects diverged. Davies et al. (35) found that low-to-average baseline HRV at rest predicted better anxiety symptom outcome with either CBT or acceptance and commitment therapy for a mixed sample of individuals with DSM-IV anxiety disorders (predominantly panic with or without agoraphobia; only 3.3% PTSD). In contrast, for individuals with primary SUD plus co-occurring PTSD, Soder et al. (36) found that high baseline HRV at rest predicted better PTSD symptom outcome with either CBT for SUD or combined CBT for SUD plus CPT for PTSD. Crucially, neither study found that HRV was able to differentially predict outcome between the two active treatments (i.e., no true treatment moderator effect for HRV), possibly because they were too similar (all CBT-like interventions). In contrast, in a study comparing CBT with yoga for pain, we found that Veterans with Gulf War illness who had higher HRV at baseline experienced greater reductions in pain with yoga, whereas those who had lower baseline HRV experienced greater pain reductions with CBT (37). Thus, HRV may be a treatment moderator, depending on the diagnostic profile of individuals or the nature of the two treatments.
Identifying treatment moderators is particularly useful when comparing two or more efficacious interventions, as it could help guide treatment allocation or selection. Yoga is a novel intervention that is garnering an increasing evidence base for its efficacy for mental health disorders, including PTSD (38). In a recently completed non-inferiority RCT, we demonstrated that a breathing-based yoga practice (Sudarshan kriya yoga; SKY) was not clinically inferior to evidence-based CPT at end-of-treatment for symptoms of PTSD, depression, and negative affect among US Veterans (39). In secondary analyses of this RCT, we demonstrated that both self-reported ER and HRV improved with SKY, but only self-reported ER reliably improved with CPT (20).
Here, we aimed to explore if ER (the self-reported Difficulties in Emotion Regulation Scale; DERS) and HRV (5-min during sleep) were non-specific predictors or treatment moderators of PTSD treatment outcome (measured by the Posttraumatic Stress Disorder Checklist-Civilian Version; PCL-C) among Veterans who received either SKY or CPT. For Hypothesis A (non-specific predictors), based on extant findings, we expected that poorer overall ER at baseline would predict better PTSD outcome overall, regardless of whether Veterans received SKY or CPT. We similarly expected that HRV would be a non-specific predictor of outcome, although due to inconsistencies in extant literature, we refrained from hypothesizing a direction. For Hypothesis B (treatment moderators), extant findings hint that poorer overall ER at baseline and lower baseline HRV may predict better PTSD symptom outcome with CPT, whereas higher HRV at baseline may predict better PTSD outcome with SKY. However, these hypothesized directions are tentative due to divergent findings and a paucity of ER/HRV treatment moderator findings in PTSD populations and/or with yoga-based interventions.
Materials and methods
Participants
Participants were US Veterans recruited from the San Francisco Bay Area via advertisements, flyers, social media, etc. All participants had clinically significant PTSD symptoms (≥38 on the PTSD Checklist for DSM-5; PCL-5; 40) and were randomized into the preregistered non-inferiority RCT “Breathing Meditation Intervention for Post-Traumatic Stress Disorder” (ClinicalTrials.gov NCT02366403; 39, 41). Here, we report secondary intent-to-treat (ITT; N = 85) analyses on the DERS and HRV data collected at baseline as non-specific predictors and treatment moderators of PTSD symptom outcome at end-of-treatment and across follow-up (1-month, 1-year). Approximately 25% of baseline cardiac data were excluded due to either equipment failure, missing (i.e., participant did not complete), or poor data quality (20). There were no significant differences between treatment groups in percentage of excluded data or demographics (all p >.05) and no significant differences in DERS scores for those with or without cardiac data (all p >.29). Groups did not differ on basic demographics (all p >.05; Table 1) nor on DERS/HRV at baseline (20).
Procedure
The protocol was approved by the Stanford University Institutional Review Board and conducted in accordance with the Declaration of Helsinki. The full procedure and primary outcomes of the RCT are described elsewhere (39, 41). Briefly, participants were randomized to receive either CPT or SKY across a 6-week period. Each intervention was delivered according to recommendations and manualized protocols (including home practice on non-class days): CPT was provided as individual, twice-weekly sessions, and SKY was provided as a 5-day group workshop followed by 10, twice-weekly group sessions (39, 41). Multiple clinician-administered, self-reported, and physiological measures were administered at multiple timepoints. Here, we report on the DERS and HRV collected at baseline and analyzed as moderators of treatment outcome for the primary outcome measure of self-reported PTSD symptoms (PCL-C), collected at baseline, end-of-treatment, 1-month follow-up, and 1-year follow-up.
Measures
Posttraumatic Stress Disorder Checklist-Civilian Version
The PCL-C (42) is a 17-item self-report measure that assesses current PTSD symptom severity corresponding to the DSM-IV diagnostic criteria for PTSD (43). Items are rated on a 5-point Likert scale according to how much the symptom bothered the respondent over the past month (1 = “not at all” to 5 = “extremely”), with scores of 38 or higher denoting clinically significant severity levels. The PCL-C has high internal consistency (α = .91–.94), test–retest reliability (r = .66–.68), and convergent validity (r = .93) (44–47). At the time of commencing the primary outcomes study, the psychometric properties of the newer PCL-5 (corresponding to the DSM-5 diagnostic criteria for PTSD (1)) were unknown and no established margin of clinically meaningful change or non-inferiority margin existed. For those reasons, the now replaced PCL-C was the primary outcome measure for the non-inferiority RCT (39, 41) and was our treatment outcome measure for these secondary moderator analyses.
The Difficulties in Emotion Regulation Scale
The DERS (15) is a 36-item self-report measure that assesses difficulties in emotional awareness, acceptance, comprehension, and adaptive reactions to emotional experiences. Items are rated on a 5-point Likert scale (0 = “almost never” to 5 = “almost always”), where higher scores reflect more difficulties with ER. The DERS can be scored as a measure of overall difficulties in ER (DERS-Total), as well as six separate subscales (DERS-Non-Acceptance, DERS-Goals, DERS-Impulse, DERS-Awareness, DERS-Strategies, and DERS-Clarity), all of which demonstrate high internal consistency (Cronbach’s α = .80–.93) and good test–retest reliability (ρI = .57–.89). As some researchers suggest ER should be measured as specific strategy use alongside overall difficulties (e.g., see 48, 49)—and to maintain consistency with our study exploring ER as an outcome of CPT/SKY for PTSD (20)—here, we assessed the DERS-Total and all DERS subscales for the non-specific predictor and treatment moderator analyses.
Heart Rate Variability
Ambulatory cardiac data were collected continuously over a 24-h period using Actiwave Cardio monitors (CamNtech Ltd): lightweight, waterproof, chest-worn devices that record heart rate (bpm) and inter-beat-interval (IBI). Data were visually inspected for artifacts (26) and preprocessed and extracted (blind to treatment group) via Kubios HRV Premium 3.1.1 (Kubios, 2019; 50–53), which can calculate HRV from Actiwave data without the need to concurrently measure respiratory rate. We extracted HRV indices from a 5-min epoch of clean, artifact-free data during the first hour of sleep; based on suggestions, this timeframe has the greatest discriminatory power across different mental health disorders (54, 55) and between Veterans and non-Veterans (56, 57). Sleep onset was defined using concurrently recorded triaxial accelerometer (actigraphy) data (Motionlogger, Ambulatory Monitoring, Ardsley NY) and validated algorithms (58) embedded in manufacturer-provided software (ActionW, Ambulatory Monitoring, Ardsley NY).
Per recommendations (26), we extracted two indices each of time-domain HRV (square root of the mean squared differences between successive R–R intervals [RMSSD (ms)], standard deviation of the IBI of normal sinus beats [SDNN (ms)]) and frequency-domain HRV (high-frequency power [HF-HFV (normalized [FFT n.u.], absolute [FFT ms²])]). These are also the most commonly examined indices in PTSD (20, 59, 60) and non-specific predictor/treatment moderator studies (35–37). RMSSD and SDNN are proposed to reflect parasympathetic activation and general autonomic function, with higher values denoting healthier functioning (26, 27, 61). While both normalized and absolute HF-HRV are also proposed to reflect parasympathetic activation (56, 57), normalized HF-HRV can also reflect general autonomic balance (26) and does not always align with absolute HF-HRV (37).
Analyses
All analyses were ITT, blind to treatment group, and conducted using IBM SPSS Statistics 29.0.0.0. We report all significant (p <.05) and trend-level (p = .05–1.0) effects alongside model fit comparisons to highlight overarching patterns that warrant further research and replication. The smallest values Akaike’s Information Criterion (AIC), Schwarz’s Bayesian Criterion (BIC), and –2 Log Likelihood (–2LL) identify the model of best fit (62). Examination of residual plots confirmed that linear mixed model assumptions were met (62, 63).
We estimated separate linear mixed models for each of the hypothesized predictors/moderators, with the primary outcome measure (PCL-C total) as the dependent variable. We included intercept for participants as random effects and by-participant random slopes for the effect of time. Fixed effects were baseline PTSD (to better equate groups at baseline; 35, 64), group (CPT, SKY; coded −0.5, +0.5 per recommendations; 65, 66), time (baseline, end-of-treatment, 1-month follow-up, 1-year follow-up; coded to reflect actual time [i.e., 0, 2, 3, 14 months] 67), the predictor/moderator (DERS-Total, DERS-Subscales, RMSSD, SDNN, normalized HF-HRV, absolute HF-HRV), and all interactions except those with baseline PTSD (covariate only). Per recommendations, baseline PTSD, time, and the predictors/moderators were mean-centered (65, 66, 68, 69) and outliers (≥ ± 3 SD) were Winsorized and replaced with the next highest value (70). Note that each of the HRV indices had two outliers.
To account for non-linear associations, we also included quadratic (squared) variables of time, the predictors/moderators, and their interactions. Factors can have different moderating effects across the short- versus long-term, for example, due to the passage of time and interference from extraneous factors that may weaken moderation effects. Therefore, consistent with other studies (e.g., 71–73), we estimated separate models for short-term predictors/moderators (i.e., from baseline to end-of-treatment) and long-term predictors/moderators (from baseline to 1-year follow-up, including end-of-treatment and 1-month follow-up).
Interaction effects and figures were probed and plotted using online Excel templates (http://www.jeremydawson.co.uk/slopes.htm; 68, 74). These figures more accurately represent the statistical model of the moderator interactions rather than arbitrary splits by low/medium/high or one-to-one data point plots of interactions (74). As the primary outcomes paper (75) reported the main effects of group and time and the group × time interactions (which also do not correspond to the current hypotheses), those effects are not reported here. Hypothesis A (non-specific predictors) was tested by the time × moderator interaction effects and Hypothesis B (treatment moderators) was tested by the group × time × moderator interaction effects, including all possible quadratic time/moderator interactions.
Results
Hypothesis A (non-specific predictors): baseline DERS/HRV will predict PTSD response to treatment, regardless of group
We found partial support for Hypothesis A: across all DERS and HRV indices, only DERS-Awareness was a non-specific predictor of either short-term (time: b = −.12, t = −2.52, p = .013) or long-term (time: b = −.20, t = −3.73, p <.001; time²: b = .02, t = 2.28, p = .024) PTSD treatment outcome. Consistent with the primary outcomes paper, Veterans showed significant treatment-related reductions in PTSD symptoms following either CPT or SKY; however, the slope of short-term (baseline to end-of-treatment) improvement was steeper for Veterans who had high baseline DERS-Awareness (p <.001; i.e., poorer self-reported ER) than for those with low baseline DERS-Awareness (p = .008; Figure 1A). Across long-term, this effect strengthened, whereby only Veterans who had high DERS-Awareness at baseline (p <.001; i.e., poorer self-reported ER) maintained treatment-related reductions in PTSD across follow-up, whereas effects were non-significant (p = .301) for those with low DERS-Awareness at baseline (Figure 1B).
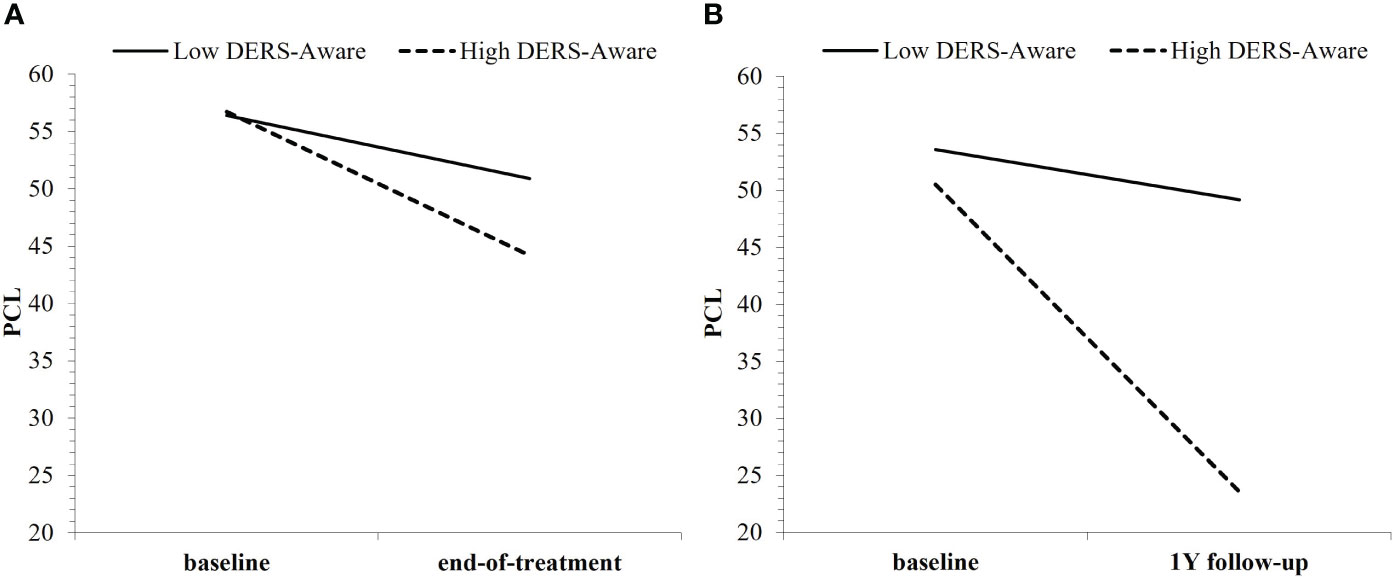
Figure 1 Baseline DERS-Awareness (where “low”/”high” refer to ±1SD from the mean) as a non-specific predictor of both (A) short-term and (B) long-term PTSD treatment outcome, regardless of group.
Hypothesis B (treatment moderators): baseline DERS/HRV will identify which Veterans respond best to SKY versus CPT for PTSD
The Difficulties in Emotion Regulation Scale
The general pattern of findings was for Veterans with higher baseline DERS to have greater reductions in and lower PTSD severity at end-of-treatment with CPT than with SKY (Figures 2A–F). This was true for DERS-Total, as well as the subscales DERS-Non-Acceptance, DERS-Impulse, DERS-Awareness, DERS-Strategies, and DERS-Clarity, but not for DERS-Goals, at end-of-treatment (i.e., support for Hypothesis B across short-term). Overall, the strongest effects were for the DERS-Non-Acceptance subscale, which was also the only DERS index to consistently moderate outcome across long-term (Table 2).
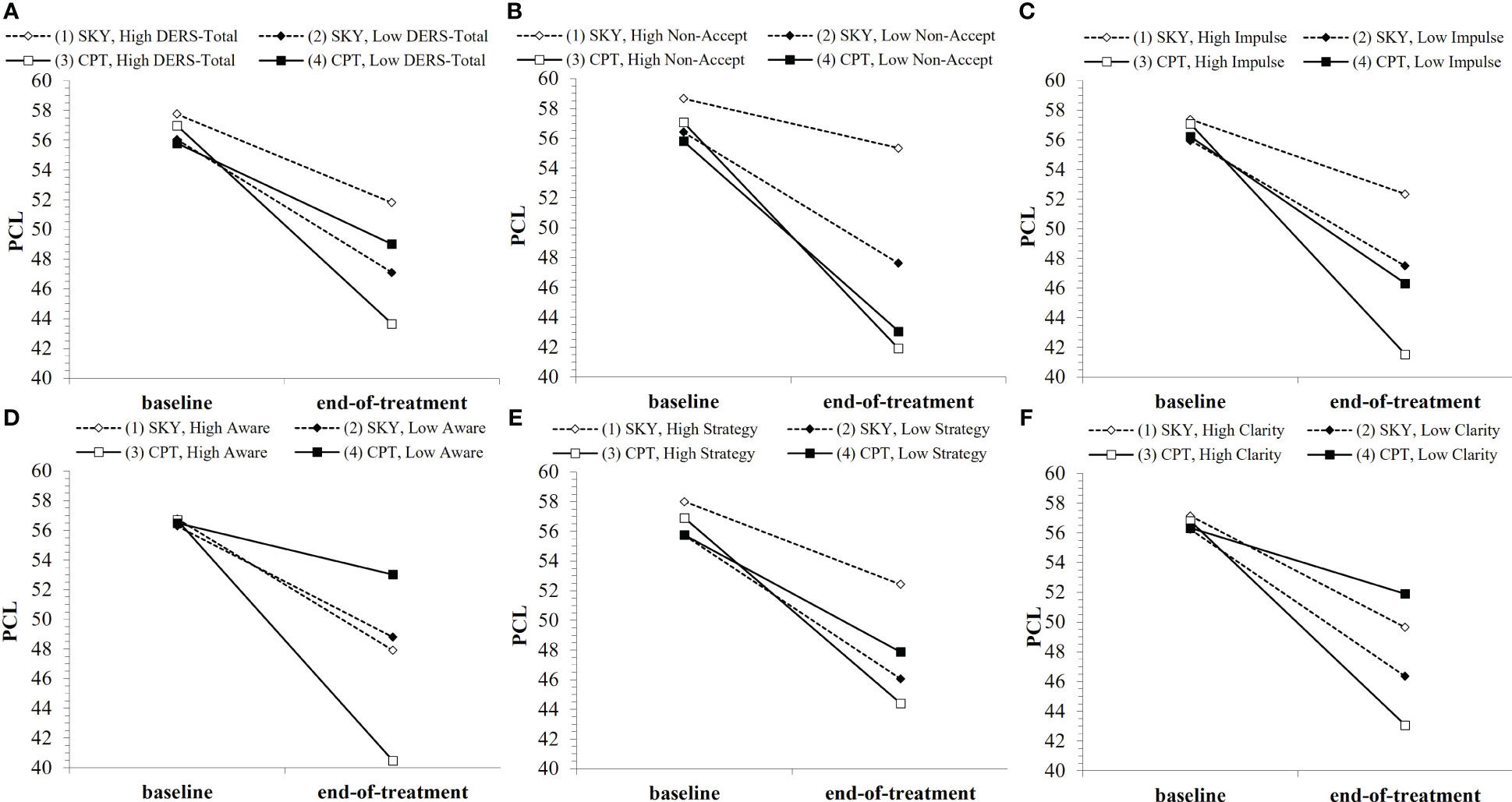
Figure 2 Baseline DERS indices (where “low”/”high” refer to ±1SD from the mean) as moderators of PTSD treatment outcome over the short-term: (A) DERS-Total, (B) DERS-Non-Acceptance, (C) DERS-Impulse, (D) DERS-Awareness, (E) DERS-Strategy, (F) DERS-Clarity. Note that points are used to differentiate lines rather than to represent data plotting. Open points (1 and 3) represent high baseline DERS, and enclosed points (2 and 4) represent low baseline DERS. Solid lines/square points represent CPT, and dotted lines/diamond points represent SKY.
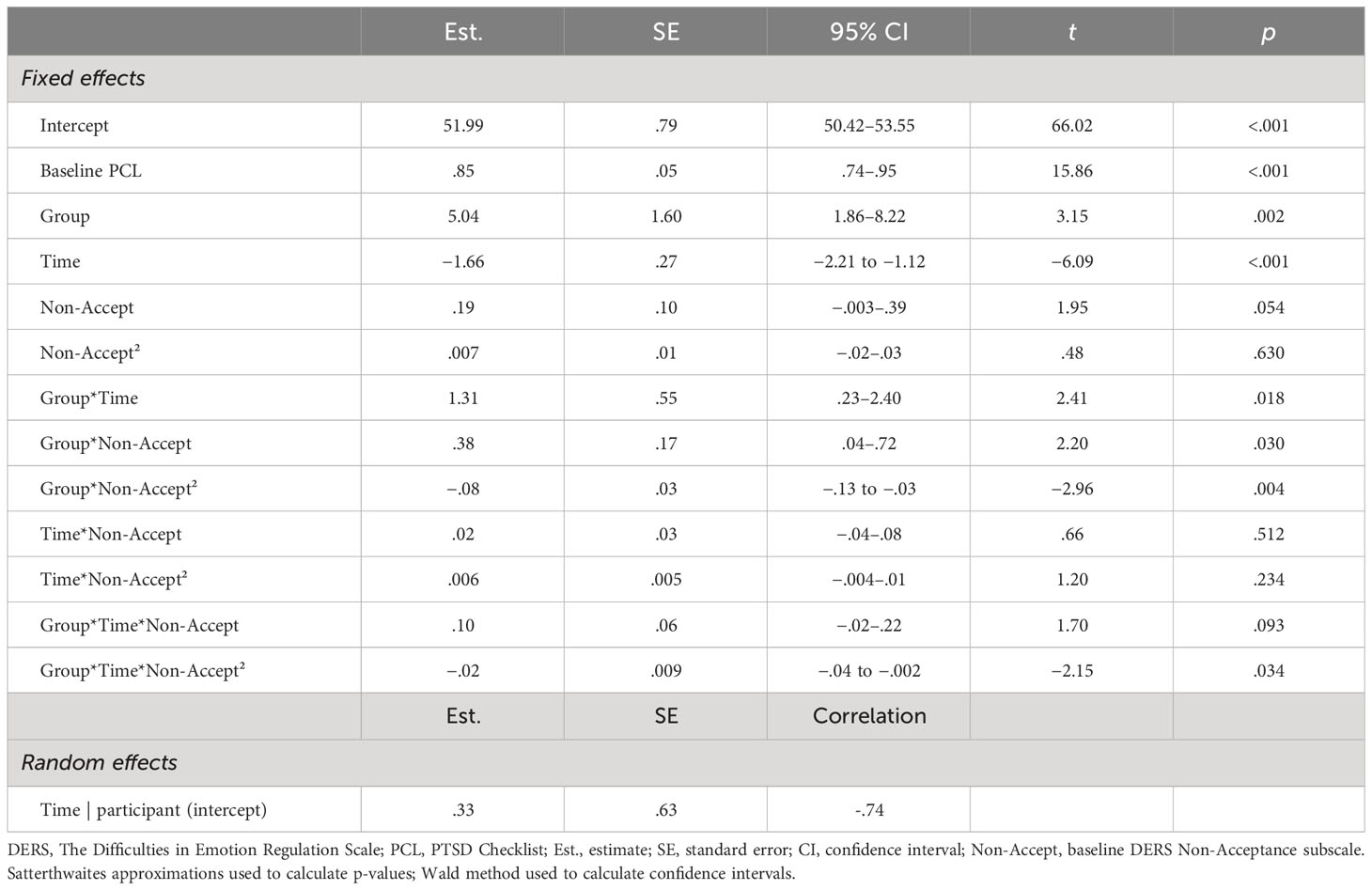
Table 2 Final full model for DERS-Non-Acceptance, the DERS index with best fit as moderator of PCL outcome across short-term.
For short-term treatment outcome, there was a significant interaction effect for group × time × DERS-Total (b = .03, t = 2.00, p = .049) and trend-level-to-significant effects for group × time × DERS for all subscales except DERS-Goals (DERS-Non-Acceptance: b = .10, t = 1.70, p = .093; DERS-Impulse: b = .12, t = 1.82, p = .072; DERS-Awareness: b = .19, t = 2.03, p = .045; DERS-Strategy: b = .10, t = 1.76, p = .079; DERS-Clarity: b = .24, t = 2.14, p = .035; Supplementary Table 1). There was also a significant interaction effect for group × time × DERS-Non-Acceptance² (b = −.02, t = −2.15, p = .034). While all group and baseline DERS-Total combinations showed significant reductions in PTSD with treatment (all p <.032), when Veterans had high DERS-Total (i.e., poorer self-reported ER) at baseline, they tended to have greater treatment-related reductions from baseline to end-of-treatment (p = .055) and significantly lower PTSD severity at end-of-treatment (p =.005) with CPT than with SKY (Figure 2A). Except for DERS-Goals, the DERS-subscales tended to show this similar pattern of greater treatment-related reductions from baseline to end-of-treatment (Non-Acceptance: p = .005; Impulse: p = .026; Awareness: p = .076) and significantly lower PTSD severity at end-of-treatment (Non-Acceptance: p <.001; Impulse: p =.002; Awareness: p = .018; Strategy: p = .011; Clarity: p = .029) for Veterans who had high baseline DERS and received CPT versus SKY (Figures 2B–F).
However, these DERS moderator effects were less consistent across long-term follow-up: there were interaction effects for group × time × DERS-Impulse (b = .14, t = 1.73, p = .085), group × time × DERS² (Non-Acceptance: b = −.03, t = −2.73, p = .007; Impulse: b = −.02, t = −1.84, p = .067), and group × time² × DERS-Non-Acceptance² (b = .005, t = 2.76, p = .006; Supplementary Table 2). While effects tapered off over long-term follow-up, Veterans who had high or average-to-high baseline DERS[-Non-Acceptance] had greater treatment-related reductions in PTSD severity across follow-up with CPT (Supplementary Figure 1A) versus SKY (Supplementary Figure 1B).
Heart Rate Variability
We found support for Hypothesis B for SDNN, RMSSD, and absolute HF-HRV, but not for normalized HF-HRV. The general pattern of findings was for Veterans with lower baseline HRV to have greater treatment-related reductions in PTSD severity with SKY and for Veterans with higher baseline HRV to have greater treatment-related reductions in PTSD severity with CPT (Figures 3A–C). Overall, the best model fit was for absolute HF-HRV (Table 3), with SDNN and RMSSD almost equivalent to each other. All HRV models were stronger fits than all DERS models (Supplementary Tables 1–4).
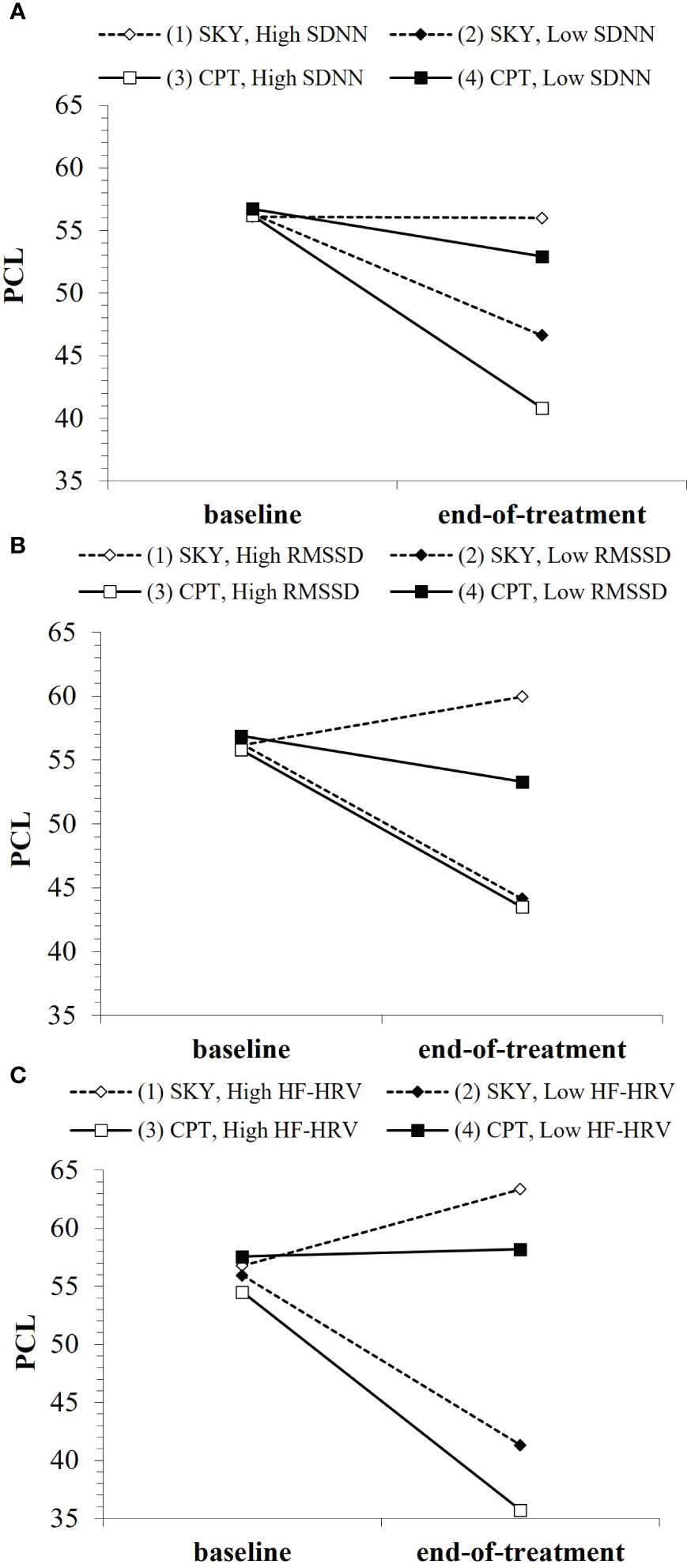
Figure 3 Baseline HRV indices (where “low”/”high” refer to ±1SD from the mean) as moderators of PTSD treatment outcome over the short-term: (A) SDNN, (B) RMSSD, (C) HF-HRV. Note that points are used to differentiate lines rather than to represent data plotting. Open points (1 & 3) represent high baseline HRV, enclosed points (2 & 4) represent low baseline HRV. Solid lines/square points represent CPT, dotted lines/diamond points represent SKY.
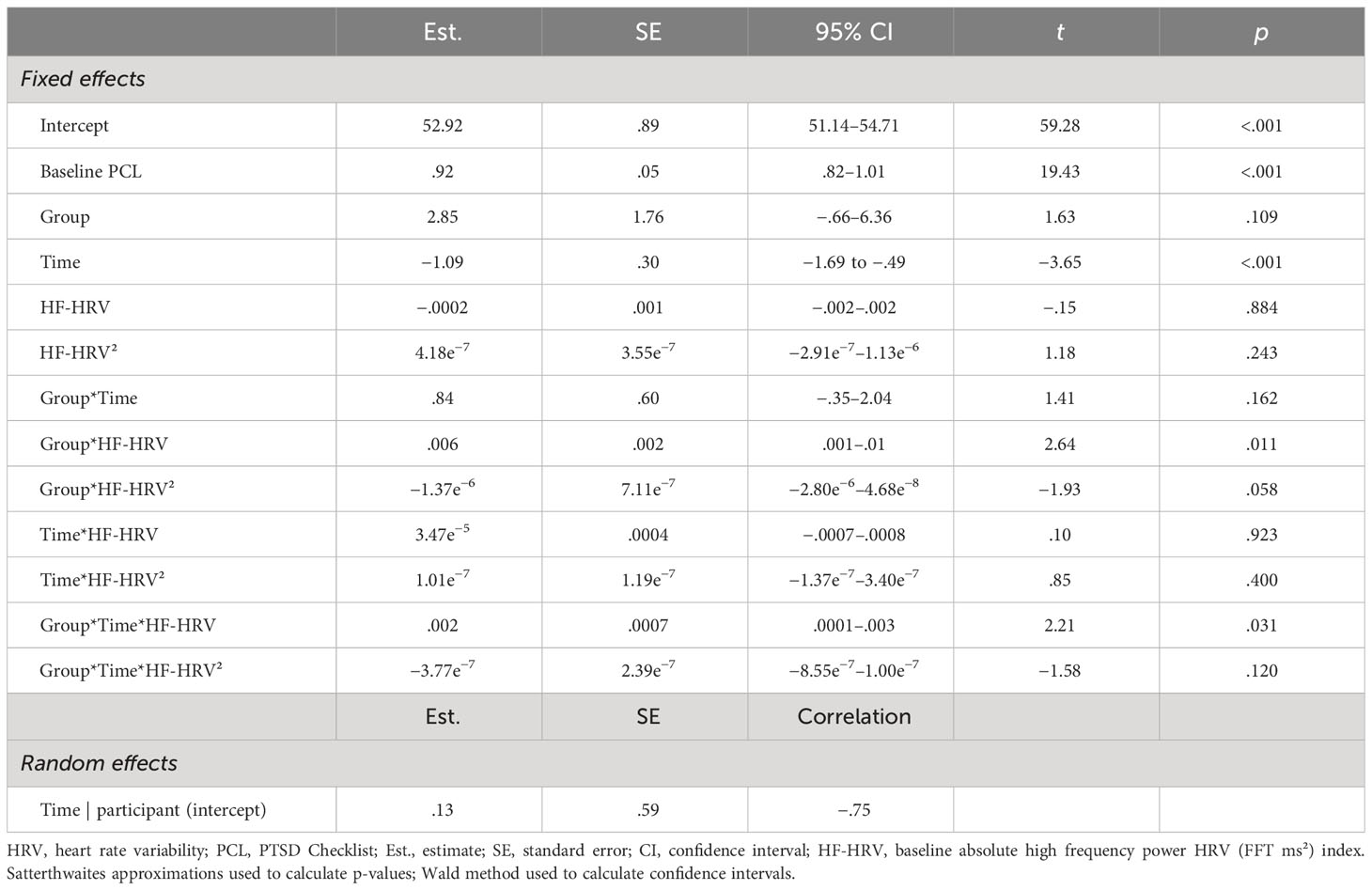
Table 3 Final full model for absolute HF-HRV (ms²), the HRV index with best fit as moderator of PCL outcome across short-term.
For short-term treatment outcome, there were interaction effects for group × time × HRV (SDNN: b = .05, t = 2.11, p = .039; RMSSD: b = .05, t = 1.92, p = .059; HF-HRV [ms²]: b = .002, t = 2.21, p = .031) and group × time × HRV² (SDNN²: b = −.0009, t = −2.32, p = .023; RMSSD²: b = −.0004, t = −1.88, p = .064; Supplementary Table 3). When Veterans had low HRV at baseline, their PTSD only significantly improved (i.e., symptoms only significantly reduced) with SKY (SDNN: p = .002; RMSSD: p = .002; HF-HRV [ms²]: p <.001) and they had significantly lower PTSD severity at end-of-treatment with SKY than with CPT (SDNN: p =.046; RMSSD: p = .020; HF-HRV [ms²]: p =.002; Figures 3A–C). In contrast, when Veterans had high HRV at baseline, their PTSD only consistently improved with CPT (SDNN: p = .005; RMSSD: p = .058; HF-HRV [ms²]: p =.097) and they had significantly lower PTSD severity at end-of-treatment with CPT than with SKY (SDNN: p =.010; RMSSD: p = .016; HF-HRV [ms²]: p =.002; Figures 3A–C).
Similarly, for long-term follow-up, there were interaction effects for group × time² × HRV (SDNN: b = −.010, t = −2.02, p = .045; HF-HRV [ms²]: b = −.0003, t = −2.03, p = .044) and group × time² × HRV² (SDNN²: b = .0002, t = 2.14, p = .034; RMSSD²: b = 8.40e−5, t = 1.77, p = .079; HF-HRV² [ms²]: b = 8.54e−8, t = 1.70, p = .092; Supplementary Table 4). While effects tapered off over long-term follow-up, Veterans who had low baseline HRV had greater treatment-related reductions in PTSD severity across follow-up with SKY (Supplementary Figures 2B, D, F), whereas Veterans who had high or average-to-high baseline HRV had greater treatment-related reductions in PTSD severity across follow-up with CPT (Supplementary Figures 2A, C, E).
Discussion
To our knowledge, this is the first study to examine both self-reported ER and HRV, within the same study, as both non-specific predictors and moderators of PTSD treatment outcome. We found that self-reported ER acted as a non-specific predictor, such that a greater baseline deficit in emotional awareness predicted better overall PTSD improvement across both short- and long-term, regardless of treatment group. Self-reported ER was also a significant treatment moderator: more overall difficulties with ER at baseline—and most strongly for high levels of emotional response non-acceptance—were associated with better PTSD treatment response with CPT than with SKY, across both short- and long-term. However, all significant HRV indices were stronger moderators than all DERS scales. Across SDNN, RMSSD, and absolute (but not normalized) HF-HRV, Veterans with lower baseline HRV (poorer autonomic function) had better PTSD treatment response with SKY, whereas Veterans with higher or average-to-high baseline HRV (better autonomic function) had better PTSD treatment response with CPT, across both short- and long-term. Absolute HF-HRV was the strongest moderator overall.
Non-specific predictor studies employing self-reported ER are rare and only recently emerging. However, our findings are consistent with the handful of extant studies demonstrating that poorer ER at baseline predicts better overall treatment response among individuals with emotional disorders. This effect occurs for self-reported expressive suppression (22) as well as experimentally induced negative emotion reactivity (76) and behavioral avoidance (35) in CBT-like therapies for transdiagnostic anxiety and/or emotional disorders. Here, we extend these findings to confirm self-reported emotional awareness as a non-specific predictor of PTSD outcome with either a CBT-like therapy (CPT) or yoga-based intervention (SKY). Specifically, impairment in emotional awareness—difficulty acknowledging or attending to feelings, also known as alexithymia—was associated with better overall PTSD treatment response. This is perhaps unsurprising given the high prevalence of alexithymia and correlation with symptom severity among PTSD populations (77, 78). Furthermore, to our knowledge, only one study has demonstrated self-reported ER acting as a true treatment moderator: among individuals with co-occurring PTSD and SUD, those who had more overall difficulties with ER at baseline had better PTSD outcome with combined CBT for PTSD/SUD, whereas those who had fewer baseline ER difficulties had better SUD outcome with CBT for SUD only (23). Here, we also confirmed that Veterans who had more overall difficulties with ER at baseline (especially greater difficulties accepting distress and a greater tendency for distress to elicit secondary emotions such as guilt or shame) had better PTSD outcome with CPT. Together, these findings suggest that for individuals with PTSD, if they also have poor ER, they are more likely to benefit from a trauma-focused psychological therapy than a non-trauma-focused psychological therapy (23) or yoga-based intervention (SKY; this study). While CBT-based therapies generally target maladaptive ER by promoting emotional awareness and acceptance and increasing adaptive ER strategies (18, 79, 80)—and we have previously demonstrated that both CPT and SKY improve self-reported ER in individuals with PTSD (20)—it is possible that trauma-focused therapies more directly target PTSD-related ER difficulties and therefore were more effective for individuals with poorer ER, whereas individuals with better ER were able to benefit from either CPT or SKY.
There are some important caveats to these conclusions. First, while self-reported ER had a statistical treatment moderator effect, this effect only resulted in a steeper slope for CPT than SKY; it did not suggest that those receiving SKY were treatment non-responders. Second, PTSD treatment dropout is more likely to occur among people with more overall self-reported ER difficulties at baseline (81) and especially for trauma-focused therapy (12). This highlights the importance of identifying predictors of dropout as well as treatment response. Furthermore, there is high heterogeneity in PTSD presentation: not only are there over 600,000 possible symptom combinations to receive a PTSD diagnosis (82), but different trauma types are associated with different symptom cluster patterns (83) that may require different treatment approaches. At the same time, there is much debate over whether ER should be measured as overall difficulties or competence or more specific ER strategy use (e.g., see 48, 49). Add to this the inherent bias in self-report measures (24, 25) and there is a need to expand our measurement of ER, particularly to objective biomarkers like HRV (28–32).
We demonstrated that lower baseline HRV was associated with better PTSD treatment response with SKY, whereas higher or average-to-high baseline HRV was associated with better PTSD treatment response with CPT. These moderator effects were strongest for absolute HF-HRV though were consistent across SDNN and RMSSD and across both short- and long-term. Importantly, all HRV moderator effects were stronger than the self-reported ER moderator effects. HRV is proposed to reflect autonomic resilience and capacity to adapt to stressors (84), so our findings suggest that Veterans with poorer autonomic regulation had better PTSD outcome with SKY whereas those with better autonomic regulation did better with CPT. This is consistent with our recent study demonstrating that SKY significantly improved HRV whereas CPT did not reliably improve HRV (20): it makes sense that those with the poorest autonomic function would gain the largest benefits from an intervention that directly improves autonomic function. It is also possible that this autonomic dysregulation might affect an individual’s ability to engage effectively in psychological treatment. Among emotional disorders, no extant study has demonstrated HRV as a true moderator. However, it is interesting to note that our findings are consistent with another non-specific predictor study where, among individuals with clinically significant PTSD symptoms (primary SUD), those with high baseline HRV had better PTSD symptom outcome with psychological therapy (36). In contrast, another study found, among individuals with predominantly panic with or without agoraphobia, those with low-to-average baseline HRV had better anxiety symptom outcome with psychological therapy (35). One possible reason for these discrepancies is the clinical characteristics of the sample, particularly the predominance of PTSD symptoms. Another is the HRV measure: our findings are consistent with Soder et al., and both they and we found effects for absolute HF-HRV, whereas Davies et al. found an opposite pattern with normalized HF-HRV (and we found no predictor/moderator effects). We have previously shown that normalized and absolute HF-HRV do not always align (37). Future research could explore if these differences in baseline HRV are associated with different PTSD symptom clusters, for example, individuals with lower baseline HRV might have more severe and/or a higher number of symptoms within the autonomic arousal/reactivity cluster.
The major strength of our study is the investigation of both non-specific predictors and treatment moderators, a practice that is highly recommended yet rarely performed (85, 86). Non-specific predictors are baseline factors that determine which individuals are more likely to respond to treatment, regardless of type (13), whereas true treatment moderators more precisely identify which treatment works best for whom (13, 14). In the primary outcomes study, we demonstrated that SKY was not clinically inferior to evidence-based CPT at end-of-treatment for symptoms of PTSD, depression, and negative affect among US Veterans (39). Thus, identifying treatment moderators is particularly useful when comparing these two efficacious interventions, as it could help guide treatment choice via precision medicine and potentially improve both treatment efficacy and cost effectiveness (85, 86). Another strength is our exploration of both self-reported ER and HRV as predictors and moderators. While self-reported ER was a significant treatment moderator, HRV had the strongest effects and was most clearly able to identify at baseline which Veterans would respond best to either CPT or SKY. Although other objective markers of ER such as neuroimaging and event-related brain potentials show promise as potential targets for precision medicine among emotional disorders (e.g., 87, 88), HRV is significantly faster, easier, and less expensive to use, making it more easily translatable to clinical practice.
Our study has some limitations. First, the sample size is moderate and there is a large amount of missing HRV data, which may explain some of our trend-level effects and why we did not find effects for normalized HF-HRV. Nevertheless, our study is fully powered for the non-inferiority primary outcome analyses, is similar to (if not larger than) the extant ER/HRV moderator studies (23, 35, 36), and demonstrates consistent patterns across measures. As ambulatory methods typically incur large proportions of missing data (e.g., poor signal quality due to issues with motion or skin adherence) (89, 90)—and indeed, our proportion of missing data (25%) is comparable with other ambulatory/sleep HRV studies (91, 92)—this should be considered in design planning of future studies, such as longer assessment durations (1–2 weeks) to maximize opportunities for usable data. Second, our 5-min HRV indices were recorded during sleep, rather than the typical “awake at-rest” condition, and might therefore be more influenced by sleep than wake physiology. Furthermore, as these were ambulatory rather than lab-based assessments, we cannot rule out the possible influence of nighttime substance use (e.g., alcohol, nicotine). However, while replication is recommended, sleep assessment has the advantage of removing cognitive and behavioral influences on physiology (20) and our findings are consistent with those of Soder et al. (36) who examined 5-min awake at-rest. In addition, while self-report findings from our sample (93) suggested an average 66%–69% likelihood of sleep apnea (as measured by the Multivariate Apnea Prediction Index [MAPI]; 94), restless legs syndrome (as measured by the Restless Legs Syndrome Diagnostic Index [RLS-DI]; 95) was absent (ruling out this potential cause of movement artifact) and there were no group differences across subjective self-reported sleep diary or PTSD sleep symptom (insomnia/nightmares) measures (93). Third, our inclusion criterion was clinically significant PTSD symptoms rather than a PTSD diagnosis and as such, not all individuals met diagnostic criteria for PTSD. However, this aligns with clinical practice models and research that focus more on dimensional distress rather than categorical diagnoses, where subthreshold symptoms are often predictive of future diagnosis and warrant intervention (96). Finally, as treatment moderator studies are rare, it is unclear whether our CPT findings generalize to all trauma-focused or psychological therapies or if our SKY findings generalize to all yoga-based or mindfulness-based interventions. This highlights the need for more treatment moderator analyses across various clinical demographics and interventions to improve treatment development, delivery, and outcome (85, 86).
In conclusion, to our knowledge, this is the first study to examine both self-reported ER and HRV, within the same study, as both non-specific predictors and moderators of PTSD treatment outcome. While we found evidence for self-reported ER acting as a non-specific predictor and treatment moderator, all significant HRV indices were stronger moderators than all DERS scales. Across SDNN, RMSSD, and absolute (but not normalized) HF-HRV, Veterans with lower baseline HRV (poorer autonomic function) had better PTSD treatment response with SKY, whereas Veterans with higher or average-to-high baseline HRV (better autonomic function) had better PTSD treatment response with CPT, across both short- and long-term. Absolute HF-HRV was the strongest moderator overall. Findings show potential for the use of HRV in clinical practice to personalize PTSD treatment.
Author’s note
A subset of preliminary data from this study were delivered in an oral presentation at the 21st World Congress of International Organization of Psychophysiology in Geneva, Switzerland in June 2023 and published in abstract form at International Journal of Psychophysiology, 188(Supp2023), 80.
Data availability statement
The datasets generated and/or analyzed during the current study are not publicly available due to institutional regulations protecting service member data but are available from the corresponding (DCM) or senior (PJB) authors on reasonable written request (may require data use agreements to be developed).
Ethics statement
The studies involving humans were approved by Stanford University Institutional Review Board. The studies were conducted in accordance with the local legislation and institutional requirements. The participants provided their written informed consent to participate in this study.
Author contributions
DCM: Conceptualization, Data curation, Formal analysis, Investigation, Methodology, Validation, Visualization, Writing – original draft, Writing – review & editing. JZ: Methodology, Resources, Software, Supervision, Validation, Writing – review & editing. RJS-H: Data curation, Investigation, Writing – review & editing. TJA: Investigation, Visualization, Writing – review & editing. PB: Conceptualization, Funding acquisition, Investigation, Methodology, Project administration, Resources, Supervision, Validation, Writing – review & editing.
Funding
The author(s) declare financial support was received for the research, authorship, and/or publication of this article. Department of Veterans Affairs RR&D Merit Review (1 I01 RX001485-01; PJB) and Department of Veterans Affairs Advanced Fellowships in the War Related Illness and Injury Study Center (DCM, RJS-H, TJA). Funding bodies have not and will not participate in the study design, the collection, management, analysis, or interpretation of data, nor the writing of findings for publication. The contents of this manuscript do not represent the views of the US Department of Veterans Affairs or the United States Government.
Acknowledgments
The authors would like to thank the participants for their time. We also thank Julia S. Tang, Kamini Dixit, Melinda Wong, Rachael H. Cho, Marcelle A. Friedman, Jennifer Hanft, and Adam S. Burn for assistance with data collection.
Conflict of interest
The authors declare that the research was conducted in the absence of any commercial or financial relationships that could be construed as a potential conflict of interest.
The author(s) declared that they were an editorial board member of Frontiers, at the time of submission. This had no impact on the peer review process and the final decision.
Publisher’s note
All claims expressed in this article are solely those of the authors and do not necessarily represent those of their affiliated organizations, or those of the publisher, the editors and the reviewers. Any product that may be evaluated in this article, or claim that may be made by its manufacturer, is not guaranteed or endorsed by the publisher.
Supplementary material
The Supplementary Material for this article can be found online at: https://www.frontiersin.org/articles/10.3389/fpsyt.2024.1331569/full#supplementary-material
Supplementary Figure 1 | Baseline DERS-Non-Acceptance (where “low”/”high” refer to ±1SD from the mean and “av.” refers to the mean) as a moderator of PTSD outcome with (A) CPT and (B) SKY, over the long-term.
Supplementary Figure 2 | Baseline HRV (where “low”/”high” refer to ±1SD from the mean and “av.” refers to the mean) as moderators of PTSD outcome with (A, C, E; left side) CPT and (B, D, F; right side) SKY, over the long-term: (A, B; top) SDNN, (C, D; middle) RMSSD, (E, F; bottom) HF-HRV.
References
1. APA. Diagnostic and statistical manual of mental disorders. 5th ed. Washington, DC: American Psychiatric Publishing (2013).
2. Lee DJ, Schnitzlein CW, Wolf JP, Vythilingam M, Rasmusson AM, Hoge CW. Psychotherapy versus pharmacotherapy for posttraumatic stress disorder: Systemic review and meta-analyses to determine first-line treatments. Depression Anxiety (2016) 33(9):792–806. doi: 10.1002/da.22511
3. NICE, NationalClinicalPracticeGuideline. Post-traumatic stress disorder: The management of PTSD in adults and children in primary and secondary care Vol. 26. Leicester, UK: Gaskell Royal College of Psychiatrists and The British Psychological Society (2005).
4. ISTSS. Effective treatments for PTSD: Practice guidelines from the International Society for Traumatic Stress Studies. 2nd ed. Foa EB, Keane TM, Friedman MJ, Cohen JA, editors. New York, NY: Guilford Press (2008).
5. Benedek DM, Friedman MJ, Zatzick D, Ursano RJ. Guideline watch (March 2009): Practice guideline for the treatment of patients with acute stress disorder and posttraumatic stress disorder. Focus (2009) 7(2):204–13. doi: 10.1176/foc.7.2.foc204
6. ACPMH, AustralianCentreforPosttraumaticMentalHealth. Australian guidelines for the treatment of acute stress disorder and posttraumatic stress disorder. Melbourne, Australia: Phoenix Australia (2013).
7. WHO. Guidelines for the management of conditions specifically related to stress. Geneva, Switzerland: World Health Organization Press (WHO Press (2013).
8. VA/DoD, TheManagementofPosttraumaticStressDisorderWorkGroup. VA/DoD clinical practice guideline for the management of posttraumatic stress disorder and acute stress disorder Vol. 3.0. Washington, DC: Department of Veterans Affairs and Department of Defense (2017).
9. Varker T, Kartal D, Watson L, Freijah I, O’Donnell M, Forbes D, et al. Defining response and nonresponse to posttraumatic stress disorder treatments: A systematic review. Clin Psychol.: Sci Pract (2020) 27(4):e12355. doi: 10.1111/cpsp.12355
10. Steenkamp MM, Litz BT, Hoge CW, Marmar CR. Psychotherapy for military-related PTSD: A review of randomized clinical trials. JAMA - J Am Med Assoc (2015) 314(5):489–500. doi: 10.1001/jama.2015.8370
11. Schottenbauer MA, Glass CR, Arnkoff DB, Tendick V, Gray SH. Nonresponse and dropout rates in outcome studies on PTSD: Review and methodological considerations. Psychiatry (2008) 71(2):134–68. doi: 10.1521/psyc.2008.71.2.134
12. Varker T, Jones KA, Arjmand HA, Hinton M, Hiles SA, Freijah I, et al. Dropout from guideline-recommended psychological treatments for posttraumatic stress disorder: A systematic review and meta-analysis. J Affect Disord Rep (2021) 4:100093. doi: 10.1016/j.jadr.2021.100093
13. Kraemer HC, Wilson GT, Fairburn CG, Agras WS. Mediators and moderators of treatment effects in randomized clinical trials. Arch Gen Psychiatry (2002) 59:877–83. doi: 10.1001/archpsyc.59.10.877
14. Kraemer HC, Kiernan M, Essex M, Kupfer DJ. How & why criteria defining moderators & mediators differ between the Baron & Kenny & MacArthur approaches. Health Psychol (2008) 27:S101–8. doi: 10.1037/0278-6133.27.2(Suppl.).S101
15. Gratz KL, Roemer L. Multidimensional assessment of emotion regulation and dysregulation: Development, factor structure, and initial validation of the DERS. J Psychopathol Behav Assess (2004) 26:41–54. doi: 10.1023/b:joba.0000007455.08539.94
16. Aldao A, Nolen-Hoeksema S, Schweizer S. Emotion-regulation strategies across psychopathology: A meta-analytic review. Clin Psychol Rev (2010) 30:217–37. doi: 10.1016/j.cpr.2009.11.004
17. Gross JJ. Emotion regulation: Conceptual and empirical foundations. In: Gross JJ, editor. Handbook of Emotion Regulation, 2nd ed. New York, NY: The Guilford Press (2014). p. 3–20.
18. Sloan E, Hall K, Moulding R, Bryce S, Mildred H, Staiger PK. Emotion regulation as a transdiagnostic treatment construct across anxiety, MDD, substance, eating & BPD: A systematic review. Clin Psychol Rev (2017) 57:141–63. doi: 10.1016/j.cpr.2017.09.002
19. Bullis JR, Boettcher H, Sauer-Zavala S, Farchione TJ, Barlow DH. What is an emotional disorder? A transdiagnostic mechanistic definition with implications for assessment, treatment, and prevention [Review]. Clin Psychol.: Sci Pract (2019) 26(2):e12278. doi: 10.1111/cpsp.12278
20. Mathersul DC, Dixit K, Schulz-Heik RJ, Avery TJ, Zeitzer JM, Bayley PJ. Emotion dysregulation and heart rate variability improve in US veterans undergoing treatment for posttraumatic stress disorder: Secondary exploratory analyses from a randomised controlled trial. BMC Psychiatry (2022) 22(1):268. doi: 10.1186/s12888-022-03886-3
21. Nielsen SKK, Hageman I, Petersen A, Daniel SIF, Lau M, Winding C, et al. Do emotion regulation, attentional control, and attachment style predict response to cognitive behavioral therapy for anxiety disorders? An investigation in clinical settings. Psychother Res (2019) 29(8):999–1009. doi: 10.1080/10503307.2018.1425933
22. Barrio-Martínez S, González-Blanch C, Priede A, Muñoz-Navarro R, Medrano LA, Moriana JA, et al. Emotion regulation as a moderator of outcomes of transdiagnostic group cognitive-behavioral therapy for emotional disorders [Article]. Behav Ther (2022) 53(4):628–41. doi: 10.1016/j.beth.2022.01.007
23. Hien DA, Lopez-Castro T, Papini S, Gorman B, Ruglass LM. Emotion dysregulation moderates the effect of cognitive behavior therapy with prolonged exposure for co-occurring PTSD and substance use disorders. J Anxiety Disord (2017) 52:53–61. doi: 10.1016/j.janxdis.2017.10.003
24. Althubaiti A. Information bias in health research: Definition, pitfalls, and adjustment methods [Review]. J Multidiscip Healthcare (2016) 9:211–7. doi: 10.2147/JMDH.S104807
25. Zilverstand A, Parvaz MA, Goldstein RZ. Neuroimaging cognitive reappraisal in clinical populations to define neural targets for enhancing emotion regulation. A systematic review. NeuroImage (2017) 151:105–16. doi: 10.1016/j.neuroimage.2016.06.009
26. Malik M, Camm AJ, Bigger JT Jr., Breithardt G, Cerutti S, Cohen RJ, et al. Heart rate variability: Standards of measurement, physiological interpretation, and clinical use. Task Force of The European Society of Cardiology and The North American Society of Pacing and Electrophysiology. Eur Heart J (1996) 17:354–81. doi: 10.1093/oxfordjournals.eurheartj.a014868
27. Shaffer F, Ginsberg JP. An overview of heart rate variability metrics and norms. Front Public Health (2017) 5:258. doi: 10.3389/fpubh.2017.00258
28. Balzarotti S, Biassoni F, Colombo B, Ciceri MR. Cardiac vagal control as a marker of emotion regulation in healthy adults: A review. Biol Psychol (2017) 130:54–66. doi: 10.1016/j.biopsycho.2017.10.008
29. Holzman JB, Bridgett DJ. HRV indices as biomarkers of top-down self-regulatory mechanisms: A meta-analytic review. Neurosci Biobehav Rev (2017) 74:233–55. doi: 10.1016/j.neubiorev.2016.12.032
30. Beauchaine TP, Thayer JF. Heart rate variability as a transdiagnostic biomarker of psychopathology. Int J Psychophysiol (2015) 98:338–50. doi: 10.1016/j.ijpsycho.2015.08.004
31. Ge F, Yuan M, Li Y, Zhang W. Posttraumatic stress disorder and alterations in resting heart rate variability: A systematic review and meta-analysis. Psychiatry Invest (2020) 17(1):9–20. doi: 10.30773/pi.2019.0112
32. Schneider M, Schwerdtfeger A. Autonomic dysfunction in posttraumatic stress disorder indexed by heart rate variability: A meta-analysis. psychol Med (2020) 50(12):1937–48. doi: 10.1017/S003329172000207X
33. Thayer JF, Åhs F, Fredrikson M, Sollers JJ, Wager TD. A meta-analysis of heart rate variability and neuroimaging studies: Implications for heart rate variability as a marker of stress and health. Neurosci Biobehav Rev (2012) 36:747–56. doi: 10.1016/j.neubiorev.2011.11.009
34. Kemp AH, Quintana DS. The relationship between mental and physical health: Insights from the study of heart rate variability. Int J Psychophysiol (2013) 89:288–96. doi: 10.1016/j.ijpsycho.2013.06.018
35. Davies CD, Niles AN, Pittig A, Arch JJ, Craske MG. Physiological and behavioral indices of emotion dysregulation as predictors of outcome from cognitive behavioral therapy and acceptance and commitment for anxiety. J Behav Ther Exp Psychiatry (2015) 46:35–43. doi: 10.1016/j.jbtep.2014.08.002
36. Soder HE, Wardle MC, Schmitz JM, Lane SD, Green C, Vujanovic AA. Baseline HRV predicts PTSD treatment outcomes in adults with co-occurring SUD & PTS. Psychophysiology (2019) 56:e13377. doi: 10.1111/psyp.13377
37. Mathersul DC, Dixit K, Avery TJ, Schulz-Heik RJ, Zeitzer JM, Mahoney LA, et al. Heart rate and heart rate variability as outcomes and longitudinal moderators of treatment for pain across follow-up in Veterans with Gulf War Illness. Life Sci (2021) 277(119604):1–11. doi: 10.1016/j.lfs.2021.119604
38. Gallegos AM, Crean HF, Pigeon WR, Heffner KL. Meditation & yoga for PTSD: meta-analysis. Clin Psychol Rev (2017) 58:115–24. doi: 10.1016/j.cpr.2017.10.004
39. Bayley PJ, Schulz-Heik RJ, Tang J, Mathersul DC, Avery TJ, Wong M, et al. Randomised clinical non-inferiority trial of breathing-based meditation and cognitive processing therapy for symptoms of posttraumatic stress disorder in military veterans. BMJ Open (2022) 12:e056609. doi: 10.1136/bmjopen-2021-056609
40. Weathers FW, Litz BT, Keane TM, Palmieri PA, Marx BP, Schnurr PP. The PTSD checklist for DSM-5 (PCL-5) (2013). Available at: www.ptsd.va.gov.
41. Mathersul DC, Tang JS, Jay Schulz-Heik R, Avery TJ, Seppälä EM, Bayley PJ. Study protocol for a non-inferiority randomised controlled trial of SKY breathing meditation versus cognitive processing therapy for posttraumatic stress disorder among veterans. BMJ Open (2019) 9(4):27150. doi: 10.1136/bmjopen-2018-027150
42. Weathers FW, Litz BT, Herman D, Huska J, Keane TM. The PTSD checklist-civilian version (PCL-C). Boston, MA: National Center for PTSD (1994). Available at: www.ptsd.va.gov.
43. APA. Diagnostic and statistical manual of mental disorders, 4th ed. Washington, DC: American Psychiatric Association. (1994).
44. Blanchard EB, Jones-Alexander J, Buckley TC, Forneris CA. Psychometric properties of the PTSD checklist (PCL). Behav Res Ther (1996) 34:669–73. doi: 10.1016/0005-7967(96)00033-2
45. Ruggiero KJ, Del Ben K, Scotti JR, Rabalais AE. Psychometric properties of the PTSD checklist - civilian version. J Trauma. Stress (2003) 16:495–502. doi: 10.1023/A:1025714729117
46. Adkins JW, Weathers FW, McDevitt-Murphy M, Daniels JB. Psychometric properties of seven self-report measures of posttraumatic stress disorder in college students with mixed civilian trauma exposure. J Anxiety Disord (2008) 22:1393–402. doi: 10.1016/j.janxdis.2008.02.002
47. Conybeare D, Behar E, Solomon A, Newman MG, Borkovec TD. The PTSD checklist-civilian version: Reliability, validity, and factor structure in a nonclinical sample. J Clin Psychol (2012) 68:699–713. doi: 10.1002/jclp.21845
48. Preece DA, Becerra R, Robinson K, Dandy J, Allan A. Measuring emotion regulation ability across negative and positive emotions: The Perth Emotion Regulation Competency Inventory (PERCI) [Article]. Pers Individ Dif (2018) 135:229–41. doi: 10.1016/j.paid.2018.07.025
49. Preece DA, Becerra R, Sauer-Zavala S, Boyes M, McEvoy P, Villanueva C, et al. Assessing emotion regulation ability for negative and positive emotions: psychometrics of the perth emotion regulation competency inventory in United States adults. J Affect Disord (2021) 294:558–67. doi: 10.1016/j.jad.2021.07.055
50. Tarvainen MP, Ranta-aho PO, Karjalainen PA. An advanced detrending method with application to HRV analysis. IEEE Trans Biomed Eng (2002) 49(2):172–5. doi: 10.1109/10.979357
51. Niskanen JP, Tarvainen MP, Ranta-Aho PO, Karjalainen PA. Software for advanced HRV analysis. Comput Methods Prog. Biomed. (2004) 76(1):73–81. doi: 10.1016/j.cmpb.2004.03.004
52. Tarvainen MP, Niskanen JP, Lipponen JA, Ranta-aho PO, Karjalainen PA. Kubios HRV - Heart rate variability analysis software. Comput Methods Prog. Biomed. (2014) 113(1):210–20. doi: 10.1016/j.cmpb.2013.07.024
53. Lipponen JA, Tarvainen MP. A robust algorithm for heart rate variability time series artefact correction using novel beat classification. J Med Eng Technol (2019) 43(3):173–81. doi: 10.1080/03091902.2019.1640306
54. Stampfer HG. The relationship between psychiatric illness and the circadian pattern of heart rate. Aust New Z J Psychiatry (1998) 32(2):187–98. doi: 10.3109/00048679809062728
55. Iverson GL, Stampfer HG, Gaetz M. Reliability of circadian heart pattern analysis in psychiatry. Psychiatr Q (2002) 73(3):195–203. doi: 10.1023/A:1016036704524
56. Haley RW, Vongpatanasin W, Wolfe GI, Bryan WW, Armitage R, Hoffmann RF, et al. Blunted circadian variation in autonomic regulation of sinus node function in veterans with Gulf War syndrome. Am J Med (2004) 117:469–78. doi: 10.1016/j.amjmed.2004.03.041
57. Haley RW, Charuvastra E, Shell WE, Buhner DM, Marshall WW, Biggs MM, et al. Cholinergic autonomic dysfunction in veterans with gulf war illness: Confirmation in a population-based sample. JAMA Neurol (2013) 70:191–200. doi: 10.1001/jamaneurol.2013.596
58. Cole RJ, Kripke DF, Gruen W, Mullaney DJ, Gillin JC. Automatic sleep/wake identification from wrist activity [Article]. Sleep (1992) 15(5):461–9. doi: 10.1093/sleep/15.5.461
59. Campbell AA, Wisco BE, Silvia PJ, Gay NG. Resting respiratory sinus arrhythmia and posttraumatic stress disorder: A meta-analysis [Article]. Biol Psychol (2019) 144:125–35. doi: 10.1016/j.biopsycho.2019.02.005
60. Sadeghi M, Sasangohar F, McDonald AD. Toward a taxonomy for analyzing the heart rate as a physiological indicator of posttraumatic stress disorder: Systematic review and development of a framework. JMIR Ment Health (2020) 7(7):e16654. doi: 10.2196/16654
61. Thayer JF, Yamamoto SS, Brosschot JF. The relationship of autonomic imbalance, heart rate variability and cardiovascular disease risk factors. Int J Cardiol (2010) 141:122–31. doi: 10.1016/j.ijcard.2009.09.543
62. Meteyard L, Davies RAI. Best practice guidance for linear mixed-effects models in psychological science. J Memory Lang (2020) 112:104092. doi: 10.1016/j.jml.2020.104092
63. Winter B. Linear models and linear mixed effects models in R with linguistic applications. (2013). arXiv:1308.5499. doi: 10.48550/arXiv.1308.5499
64. Wolitzky-Taylor KB, Arch JJ, Rosenfield D, Craske MG. Moderators and non-specific predictors of treatment outcome for anxiety disorders: A comparison of cognitive behavioral therapy to acceptance and commitment therapy. J Consult. Clin Psychol (2012) 80(5):786–99. doi: 10.1037/a0029418
65. Kraemer HC, Blasey CM. Centring in regression analyses: A strategy to prevent errors in statistical inference. Int J Methods Psychiatr Res (2004) 13(3):141–51. doi: 10.1002/mpr.170
66. Kraemer HC. Discovering, comparing, and combining moderators of treatment on outcome after randomized clinical trials: A parametric approach. Stat Med (2013) 32(11):1964–73. doi: 10.1002/sim.5734
67. Singer JD, Willett JB. Modeling the days of our lives: Using survival analysis when designing and analyzing longitudinal studies of duration and the timing of events. psychol Bull (1991) 110(2):268–90. doi: 10.1037/0033-2909.110.2.268
68. Dawson JF. Moderation in management research: What, why, when, and how. J Business Psychol (2014) 29:1–19. doi: 10.1007/s10869-013-9308-7
69. Shek DTL, Ma CMS. Application of SPSS linear mixed methods to adolescent development research: Basic concepts and steps. Int J Disabil. Hum Dev (2014) 13(2):169–82. doi: 10.1515/ijdhd-2014-0303
70. Guttman I. Premium and protection of several procedures for dealing with outliers when sample sizes are moderate to large [Article]. Technometrics (1973) 15(2):385–404. doi: 10.1080/00401706.1973.10489051
71. Shapiro SL, Brown KW, Thoresen C, Plante TG. The moderation of Mindfulness-based stress reduction effects by trait mindfulness: Results from a randomized controlled trial. J Clin Psychol (2011) 67(3):267–77. doi: 10.1002/jclp.20761
72. Abar CC, Jackson KM, Wood M. Reciprocal relations between perceived parental knowledge and adolescent substance use and delinquency: The moderating role of parent–teen relationship quality. Dev Psychol (2014) 50:2176–87. doi: 10.1037/a0037463
73. Sinclair KL, Kiser E, Ratcliff CG, Chaoul A, Hall MH, Rinpoche TW, et al. Sleep moderates the effects of Tibetan yoga for women with breast cancer undergoing chemotherapy. Support. Care Cancer (2022) 30(5):4477–84. doi: 10.1007/s00520-022-06861-6
74. Dawson JF, Richter AW. Probing three-way interactions in moderated multiple regression: Development and application of a slope difference test [Article]. J Appl Psychol (2006) 91(4):917–26. doi: 10.1037/0021-9010.91.4.917
75. Bayley PJ, Cho RH, Schulz-Heik RJ, Mathersul DC, Collery LM, Shankar K, et al. Yoga is effective in treating symptoms of Gulf War Illness. J Psychiatr Res (2021) 143:563–71. doi: 10.1016/j.jpsychires.2020.11.024
76. Niles AN, Mesri B, Burklund LJ, Lieberman MD, Craske MG. Attentional bias and emotional reactivity as predictors and moderators of behavioral treatment for social phobia. Behav Res Ther (2013) 51(10):669–79. doi: 10.1016/j.brat.2013.06.005
77. Frewen PA, Dozois DJA, Neufeld RWJ, Lanius RA. Meta-analysis of alexithymia in posttraumatic stress disorder. J Trauma. Stress (2008) 21(2):243–6. doi: 10.1002/jts.20320
78. Edwards ER. Posttraumatic stress and alexithymia: A meta-analysis of presentation and severity. psychol Trauma: Theory Res. Pract. Policy. (2019) 14:1192. doi: 10.1037/tra0000539
79. Resick PA, Galovski TE, Uhlmansiek MO, Scher CD, Clum GA, Young-Xu Y. A randomized clinical trial to dismantle components of cognitive processing therapy for posttraumatic stress disorder in female victims of interpersonal violence. J Consult. Clin Psychol (2008) 76(2):243–58. doi: 10.1037/0022-006X.76.2.243
80. Resick PA, Monson CM, Chard KM. Cognitive processing therapy for PTSD: A comprehensive manual. New York, NY: The Guilford Press (2017).
81. Gilmore AK, Lopez C, Muzzy W, Brown WJ, Grubaugh A, Oesterle DW, et al. Emotion dysregulation predicts dropout from prolonged exposure among women veterans with military sexual trauma-related PTSD [Article]. Women's Health Issues (2020) 30(6):462–9. doi: 10.1016/j.whi.2020.07.004
82. Galatzer-Levy IR, Bryant RA. 636,120 ways to have posttraumatic stress disorder. Perspect psychol Sci (2013) 8(6):651–62. doi: 10.1177/1745691613504115
83. Contractor AA, Weiss NH, Natesan Batley P, Elhai JD. Clusters of trauma types as measured by the life events checklist for DSM-5. Int J Stress Manage. (2020) 27:380–93. doi: 10.1037/str0000179
84. Appelhans BM, Luecken LJ. Heart rate variability as an index of regulated emotional responding [Review]. Rev Gen Psychol (2006) 10:229–40. doi: 10.1037/1089-2680.10.3.229
85. Kazdin AE. Mediators and mechanisms of change in psychotherapy research. Annu Rev Clin Psychol (2007) 3:1–27. doi: 10.1146/annurev.clinpsy.3.022806.091432
86. Onken LS, Carroll KM, Shoham V, Cuthbert BN, Riddle M. Reenvisioning clinical science: Unifying the discipline to improve the public health. Clin psychol Sci (2014) 2:22–34. doi: 10.1177/2167702613497932
87. Paul S, Kathmann N, Elsner B, Reuter B, Barnow S, Simon D, et al. Neural correlates of emotional reactivity predict response to cognitive-behavioral therapy in obsessive-compulsive disorder. J Affect Disord (2022) 308:398–406. doi: 10.1016/j.jad.2022.04.024
88. Picó-Pérez M, Fullana MA, Albajes-Eizagirre A, Vega D, Marco-Pallarés J, Vilar A, et al. Neural predictors of cognitive-behavior therapy outcome in anxiety-related disorders: A meta-analysis of task-based fMRI studies. psychol Med (2022) 53:3387-95. doi: 10.1017/S0033291721005444
89. Steinberg JS, Varma N, Cygankiewicz I, Aziz P, Balsam P, Baranchuk A, et al. 2017 ISHNE-HRS expert consensus statement on ambulatory ECG and external cardiac monitoring/telemetry. Heart Rhythm (2017) 14(7):e55–96. doi: 10.1016/j.hrthm.2017.03.038
90. Smets E, De Raedt W, Van Hoof C. Into the wild: the challenges of physiological stress detection in laboratory and ambulatory settings. IEEE J Biomed Health Inf (2019) 23(2):463–73. doi: 10.1109/JBHI.2018.2883751
91. Gu Z, Zarubin VC, Mickley Steinmetz KR, Martsberger C. Heart rate variability in healthy subjects during monitored, short-term stress followed by 24-hour cardiac monitoring. Front Physiol (2022) 13:897284. doi: 10.3389/fphys.2022.897284
92. Daffre C, Oliver KI, Nazareno JRS, Mäder T, Seo J, Dominguez JP, et al. Rapid eye movement sleep parasympathetic activity predicts wake hyperarousal symptoms following a traumatic event. J Sleep Res (2023) 32(1):e13685. doi: 10.1111/jsr.13685
93. Mathersul DC, Schulz-Heik RJ, Avery TJ, Allende S, Zeitzer JM, Bayley PJ. US Veterans show improvements in subjective but not objective sleep following treatment for posttraumatic stress disorder: Secondary analyses from a randomised controlled trial. Depression Anxiety (2023) 2023:1-13. doi: 10.1155/2023/7001667
94. Maislin G, Pack AI, Kribbs NB, Smith PL, Schwartz AR, Kline LR, et al. A survey screen for prediction of apnea. Sleep (1995) 18:158–66. doi: 10.1093/sleep/18.3.158
95. Beneš H, Kohnen R. Validation of an algorithm for the diagnosis of Restless Legs Syndrome: The Restless Legs Syndrome-Diagnostic Index (RLS-DI). Sleep Med (2009) 10:515–23. doi: 10.1016/j.sleep.2008.06.006
Keywords: emotion regulation, heart rate variability, posttraumatic stress disorder, yoga, cognitive processing therapy, moderator, predictor, precision medicine
Citation: Mathersul DC, Zeitzer JM, Schulz-Heik RJ, Avery TJ and Bayley PJ (2024) Emotion regulation and heart rate variability may identify the optimal posttraumatic stress disorder treatment: analyses from a randomized controlled trial. Front. Psychiatry 15:1331569. doi: 10.3389/fpsyt.2024.1331569
Received: 01 November 2023; Accepted: 10 January 2024;
Published: 08 February 2024.
Edited by:
Giulio Maria Pasinetti, Icahn School of Medicine at Mount Sinai, United StatesReviewed by:
Vrinda Saxena, Mount Sinai Hospital, United StatesAntonio Luque, University of Almeria, Spain
Barbara L. Niles, United States Department of Veterans Affairs, United States
Arpi Minassian, University of California, San Diego, United States
Copyright © 2024 Mathersul, Zeitzer, Schulz-Heik, Avery and Bayley. This is an open-access article distributed under the terms of the Creative Commons Attribution License (CC BY). The use, distribution or reproduction in other forums is permitted, provided the original author(s) and the copyright owner(s) are credited and that the original publication in this journal is cited, in accordance with accepted academic practice. No use, distribution or reproduction is permitted which does not comply with these terms.
*Correspondence: Danielle C. Mathersul, danielle.mathersul@murdoch.edu.au
†Present addresses: R. Jay Schulz-Heik, Peninsula Behavioral Health, Palo Alto, CA, United States
Timothy J. Avery, Peninsula Vet Center, Department of Veterans Affairs, Menlo Park, CA, United States