- 1Human Molecular Genetics Laboratory, Rajiv Gandhi Centre for Biotechnology, Thiruvananthapuram, Kerala, India
- 2Research Center, University of Kerala, Thiruvananthapuram, Kerala, India
- 3Mental Health Centre, Thiruvananthapuram, Kerala, India
- 4Nair’s Hospital, Maradu, Kerala, India
Schizophrenia is a complex condition with entwined genetic and epigenetic risk factors, posing a challenge to disentangle the intermixed pathological and therapeutic epigenetic signatures. To resolve this, we performed 850K methylome-wide and 700K genome-wide studies on the same set of schizophrenia patients by stratifying them into responders, non-responders, and drug-naïve patients. The key genes that signified the response were followed up using real-time gene expression studies to understand the effect of antipsychotics at the gene transcription level. The study primarily implicates hypermethylation in therapeutic response and hypomethylation in the drug-non-responsive state. Several differentially methylated sites and regions colocalized with the schizophrenia genome-wide association study (GWAS) risk genes and variants, supporting the convoluted gene–environment association. Gene ontology and protein–protein interaction (PPI) network analyses revealed distinct patterns that differentiated the treatment response from drug resistance. The study highlights the strong involvement of several processes related to nervous system development, cell adhesion, and signaling in the antipsychotic response. The ability of antipsychotic medications to alter the pathology by modulating gene expression or methylation patterns is evident from the general increase in the gene expression of response markers and histone modifiers and the decrease in class II human leukocyte antigen (HLA) genes following treatment with varying concentrations of medications like clozapine, olanzapine, risperidone, and haloperidol. The study indicates a directional overlap of methylation markers between pathogenesis and therapeutic response, thereby suggesting a careful distinction of methylation markers of pathogenesis from treatment response. In addition, there is a need to understand the trade-off between genetic and epigenetic observations. It is suggested that methylomic changes brought about by drugs need careful evaluation for their positive effects on pathogenesis, course of disease progression, symptom severity, side effects, and refractoriness.
1 Introduction
Schizophrenia is a severe neuropsychiatric condition with a lifetime weighted prevalence of 0.6% and a current weighted prevalence of 0.12% among the population of Kerala (1). This condition is characterized by diverse positive, negative, and cognitive symptoms resulting from a complicated interaction between genetic and environmental factors (2). Antipsychotic drugs are extensively used for reducing the symptomatic burden of psychosis. Research indicates that insufficient clinical intervention in the early stages of psychosis raises the mortality rate among young individuals (3). Even when antipsychotic therapy was considered a primary treatment, refractoriness to medication was observed in 23% of the patients (4, 5). Treatment resistance is the persistence of symptoms after two medication trials with appropriate dosage, duration, and proper adherence (6). Clozapine is regarded as the primary pharmacological strategy, but the adverse effects associated with clozapine have raised concerns over its use (7). In patients unresponsive to clozapine treatment, atypical antipsychotic polypharmacy is suggested as an alternative, albeit with the potential drawback of metabolic syndrome and other side effects (8, 9). Studies have indicated the use of quetiapine over clozapine to treat individuals with significant negative symptoms due to clozapine’s adverse effects (10, 11). Therefore, identifying markers contributing to the positive response or lack of response helps in the early prediction of treatment outcomes to avoid the impact of multiple drug treatments.
Genome-wide association study (GWAS) conclusively demonstrates the heritability of schizophrenia contributed by multiple variants that were predominantly spread across genes expressed in the central nervous system neurons (12). These risk loci and their risk alleles do demonstrate differential expression that can further be influenced by environmental variables and thereby may play a role in the etiology of schizophrenia. Gene expression is tightly regulated, and epigenetic processes such as DNA methylation can influence it by altering chromatin composition (13). The gene regulation through epigenetic mechanisms is responsive to the environmental risk variables linked to the development of schizophrenia (13–15). The decreased concordance rate in monozygotic twin studies corroborates the involvement of environmental risk factors (16, 17). Genome-wide methylation variations have been extensively studied in association with schizophrenia in several surrogate and brain tissues (18–21), and these associated differentially methylated positions strongly colocalized with schizophrenia risk loci, further supporting the intertwined genetic and epigenetic regulation of multifactorial disorders (22). Evidence also implies that antipsychotic drugs can affect the epigenome (23–26).
In general, large cohort studies give less emphasis on antipsychotic treatment response; therefore, disease-associated methylation markers are likely to be confounded by drug-induced alterations. Studies have indicated that antipsychotic drugs could mire these epigenetic observations; in addition, the schizophrenia pool could also comprise of drug response and resistant pool (24). In a conventional study protocol, it is difficult to have monotherapy patients in assessing the epigenetic landscape. Therefore, in the current study, we aimed to identify the DNA methylation signatures of treatment response and resistance in an ethnically stratified population (27, 28) by examining aberrations in the DNA methylation level of drug-naïve patients, treatment responders, and treatment non-responders using whole blood DNA.
2 Materials and methods
2.1 Study subjects for DNA methylation study
Blood samples from a Malayalam-speaking Kerala population, with 48 participants diagnosed with schizophrenia, were collected using the Diagnostic and Statistical Manual of Mental Disorders (DSM)-IV criteria. The participants were divided into treatment responders (n = 20) and non-responders (n = 20) based on their Brief Psychiatric Rating Scale (BPRS) score improvement after 1 year of follow-up as described earlier (29). All patients received multidrug therapy except for drug-naïve participants (n = 8). The drugs prescribed to the study subjects were olanzapine, clozapine, haloperidol, and risperidone. The average antipsychotic dose was converted to CPZeq. The responders were given an average CPZeq of 537.21 ± 48.37 mg/day, while non-responders were given 622.22 ± 109.8 mg/day. Demographic information of the study samples is listed in Supplementary Table S1. Except for the drug-naïve participants, all other participants’ sampling was conducted after confirming their treatment response status. DNA was isolated from peripheral blood using the phenol–chloroform method. The Institutional Ethics Committee approved the study for biomedical subjects as per the Indian Council of Medical Research (ICMR) and Helsinki protocol.
2.2 DNA methylation analysis
To investigate the DNA methylation patterns in drug-naïve, treatment responder, and non-responder schizophrenia patients, the Illumina Methylation EPIC 850K BeadChip (Illumina, San Diego, CA, USA) was used. The whole blood DNA was bisulfite-converted using the EZ DNA Methylation™ kit (Zymo Research, Irvine, CA, USA) following the standard protocol. The converted DNA was amplified and enzymatically fragmented before being applied to the array for hybridization, followed by a single-base extension. The Illumina iScan system was used to scan the fluorescently labeled BeadChip. The intensity data files from Illumina were imported into the R programming environment version 4.1.0 (30) and analyzed using the ChAMP v2.28.0 package (31, 32). The following were excluded: probes that had a detection p-value greater than 1e−16 and failed p-value threshold above 0.01, probes with a bead count of less than 3 in more than 5% of the samples, non-CpG probes, probes located near single-nucleotide polymorphisms (SNPs) defined by their allele frequency in Indian Telugu in the UK (ITU) population data, cross-reacting probes provided by maxprobes v0.0.2 R package, and sex chromosome probes. Samples with a failed p-value above the proportion of the NA ratio threshold of 5% were filtered out. The list of probes filtered out from the study is provided in Supplementary Table S2. One sample from the non-responder category was eliminated based on the discrepancy between the estimated and reported sex. On filtered probes, the SWAN normalization technique was applied (33). The “estimateCellCounts2” function in the FlowSorted.Blood.EPIC v2.2.0 package was used for cell count estimation (Supplementary Figure S1) (34). The comBat batch correction accounted for array and batch variations (35). Using the “champ.SVD” function, the confounding variables were identified and regressed. The flowchart of the analysis is shown in Figure 1.
2.3 Identification of differentially methylated probes
The DNA methylation level was quantified as a beta score. The function “champ.DMP” uses the limma package to pairwise compare the phenotype of interest to identify differentially methylated areas (36). Potential inflation and bias were controlled using the R package Bacon v1.26.0 (37). The conservative Bonferroni method was employed to control the family-wise error rate (FWER) (p < 0.1) and the Benjamini and Yekutieli method (p < 0.05) to control the false discovery rate (FDR). Additionally, the Psychiatric Genomics Consortium-EWAS pipeline was utilized to enhance the sensitivity of our findings (38). The significance of the overlap between the comparisons was evaluated using Fisher’s exact test, and the similarity between the probe list was defined in terms of the Jaccard index using the R package GeneOverlap v1.38.0 and HelloRanges v1.27 (39, 40). BECon was used to determine the relationship between the differences in CpG sites between surrogate tissue, blood, and the brain (41). Using the EWAS-atlas platform, a correlation between probe methylation and a trait was inferred (42).
2.4 Identification of the differentially methylated region
The Bumphunter algorithm through the “champ.DMR” function was used to estimate the difference in DNA methylation with regard to a genomic region (43). The minimum number of probes per differentially methylated region (DMR) was set at 7, while the maximum length of the DMR was set at 300. Alternatively, the DMRcate algorithm was also used to estimate DMRs (44). The array manifest file was used to annotate all DMRs above the p-value limit of 0.05. Assessment of the functional role of the top 1,000 DMRs was made through the gene ontology (GO) enrichment and Kyoto Encyclopedia of Genes and Genomes (KEGG) pathway analyses using the DMR annotation tool provided by the scMethBank (45). Using the DOSE v3.28.1 package function “enrichDGN”, all the publicly available disease–gene associations listed in the DisGeNET platform were probed, and an overrepresentation analysis among the differentially methylated genes was performed (46).
2.5 Functional enrichment analysis of DMPs
The “gometh” function from the missMethyl v1.32.0 package was used for differentially methylated probe (DMP) enrichment analysis of all probes with |Δβ| > 0.05 to eliminate bias resulting from the presence of varying numbers of CpG probes per gene (47). The Benjamini and Hochberg method was used to adjust p value the significance level was set to 0.05.
2.6 Protein–protein interaction network of DMP- and DMR-associated genes
A protein–protein interaction network of genes associated with DMPs (|Δβ| ≥ 0.05), as well as the DMRs, was constructed using the STRING database, and the network was loaded into Cytoscape v3.9.1 to identify hub genes using the CytoHubba plugin (combined score >0.6) (48–50). The MCODE plugin’s default parameters were utilized to discover dense gene subnetworks (degree cutoff = 2, mode score cutoff = 5, max.depth = 100, k score = 2) among the DMP and DMR genes (51).
2.7 SNP genotyping and methylation QTL analysis
Infinium Global Screening Array-24 v3.0 BeadChip comprising 700k genotypes was used to screen in genomic DNA isolated from the whole blood of responders (n = 18) and non-responders (n = 16) (Illumina, USA) following the standard protocol. Denatured DNA underwent enzymatic fragmentation, followed by hybridization with a bead chip. Hybridized primers were then extended and stained for detection and analysis. Finally, the BeadChips were scanned using Illumina iScan to generate the Illumina Intensity Data files or IDAT. The IAAP Genotyping Command Line Interface (CLI) generated vcf files using Illumina intensity data. Quality control was performed using the R package plinkQC v0.3.4 (52), omitting samples with >1% missing variants and SNPs with >1% missingness, Hardy–Weinberg equilibrium p-value <0.05, and a minor allele frequency of 5%. Variants on chromosomes 1–22 were only considered for this investigation. The probes that were excluded from the analysis are shown in Supplementary Table S2. R package MatrixEQTL v2.3 was used to identify the association between SNP–methylation probe pairs; sex, age, batch, slide, cell type composition, and principal components (first 10) from the genotype data were included as covariates (53). All significant DMPs were examined for association with SNPs. All the SNPs located within 50 kb of the CpG sites were considered cis–methylation quantitative trait locus (meQTL), and the significance threshold was set as FDR ≤ 0.1. The meQTL variants or variants in linkage disequilibrium (LD) within them were searched for reported association with the disease phenotype: schizophrenia (population-GIH, ITU; R′22 ≥ 0.2; 50-kb base pair window), and association to tissue-specific gene expression (blood; population-GIH, ITU; R′2 ≥ 0.8; p < 0.05; 50-kb base pair window) were enquired using the LDlink suite (54). Colocalization analysis was performed for the cis-meQTLs and treatment response GWAS variants using the coloc package v5.2.2 (55).
2.8 Peripheral blood mononuclear cell culture and antipsychotic treatment
Peripheral blood mononuclear cells (PBMCs) were isolated from 10 ml of blood obtained from a healthy donor by density gradient centrifugation using a low-viscosity medium (HiSepTM LS 1077, HiMedia, Thane, India). Blood was diluted (1:1) with 1× phosphate-buffered saline (PBS) carefully placed over a low-viscosity medium and centrifuged at 750 g for 30 min at 27°C. The PBMC-containing buffy coat was removed and washed with 1× PBS 350 g for 10 min. PBMC was suspended in 1× red blood cell (RBC) lysis buffer for 5 min to eliminate any residual RBC from the separation procedure. The PBMC pellets were rinsed twice with 1× PBS buffer and resuspended in RPMI-1640 media with 2 mM l-glutamate (HiMedia), antibiotics (1× penicillin/streptomycin, Thermo Scientific, Waltham, MA, USA), and 10% heat-inactivated fetal bovine serum (Gibco, Grand Island, NY, USA). Viable cells were plated at a density of 2 * 106/ml in a 6-well plate and cultured for 72 hours at 37°C and 5% CO2 with different concentrations of the following antipsychotic drugs (Sigma): haloperidol (12 μM, 6 μM, and 3 μM), olanzapine (3.2 μM, 1.6 μM, and 0.8 μM), clozapine (8 μM, 4 μM, and 2 μM), and risperidone (4 μM, 2 μM, and 1 μM). The concentration of antipsychotic medicine used for the drug treatment was based on an earlier study (56). The drugs were dissolved in dimethyl sulfoxide (DMSO), and 0.1% DMSO-treated cells were used as the assay control in our study. After 72 hours of drug treatment, cells were pelted for RNA extraction.
2.9 Gene expression study
Total RNA was extracted from the PBMCs with the TRIzol reagent (Invitrogen, Carlsbad, CA, USA: 15596026) and treated with “RQ1 RNase-Free DNase” (Promega, Madison, WI, USA: M6101) according to the manufacturers’ protocol. Following the quantification using NanoDrop (Thermo Scientific), 1 µg of RNA was reverse transcribed to cDNA using the “PrimeScript 1st Strand cDNA Synthesis Kit” (Takara Bio, Mountain View, CA, USA: 6110A) based on the manufacturer’s instructions. A SYBR-based assay using the “TB Green Premix Ex Taq II (Tli RNaseH Plus)” kit (Takara Bio: RR820W) was used to estimate the mRNA level. A 5-μl reaction was performed with “1× TB Green Premix Ex Taq II (Tli RNaseH Plus)” mix, 0.4 μM forward and reverse primers, 1× ROX reference dye, RNase-free water, and 5 ng of RNA. The National Center for Biotechnology Information (NCBI) primer blast and IDT primer designing software were used to design primers. For each sample, triplicates were employed for running qRT-PCR experiments in Quant Studio 5 (Applied Biosystems, Foster City, CA, USA) as per the manufacturer’s protocol: 95°C for 3 min, followed by 40 cycles with 95°C for 5 s, 60°C for 34 s at a ramp rate of 1.6°C/s. Conditions for the melt curve were as follows: 95°C for 15 s, 60°C for 1 min at 1.6°C/s, and 95°C for 0.01 s at a ramp rate of 0.15°C/s. The endogenous control β-actin was used to normalize gene expression, and its relative expression was determined using the comparative Ct (2−ΔΔCt) method. Sequence details of primers used in this study are listed in Supplementary Table S3. Differences between groups were evaluated by one-way ANOVA with Sidak’s multiple-comparison test using GraphPad prism software v8.1, and the data are presented as mean ± standard error of the mean of two independent experiments.
3 Results
3.1 Differential DNA methylation analysis
The DNA methylation levels differentiating drug responders and drug non-responders are referred to as markers of treatment response (Figure 2), those between drug responders and drug-naïve patients are referred to as markers of treatment effectiveness (Figure 3), and those between drug-naïve patients and drug-non-responders are referred to as markers of treatment resistance (Figure 4). Table 1 details the number of differential sites identified in various comparisons.
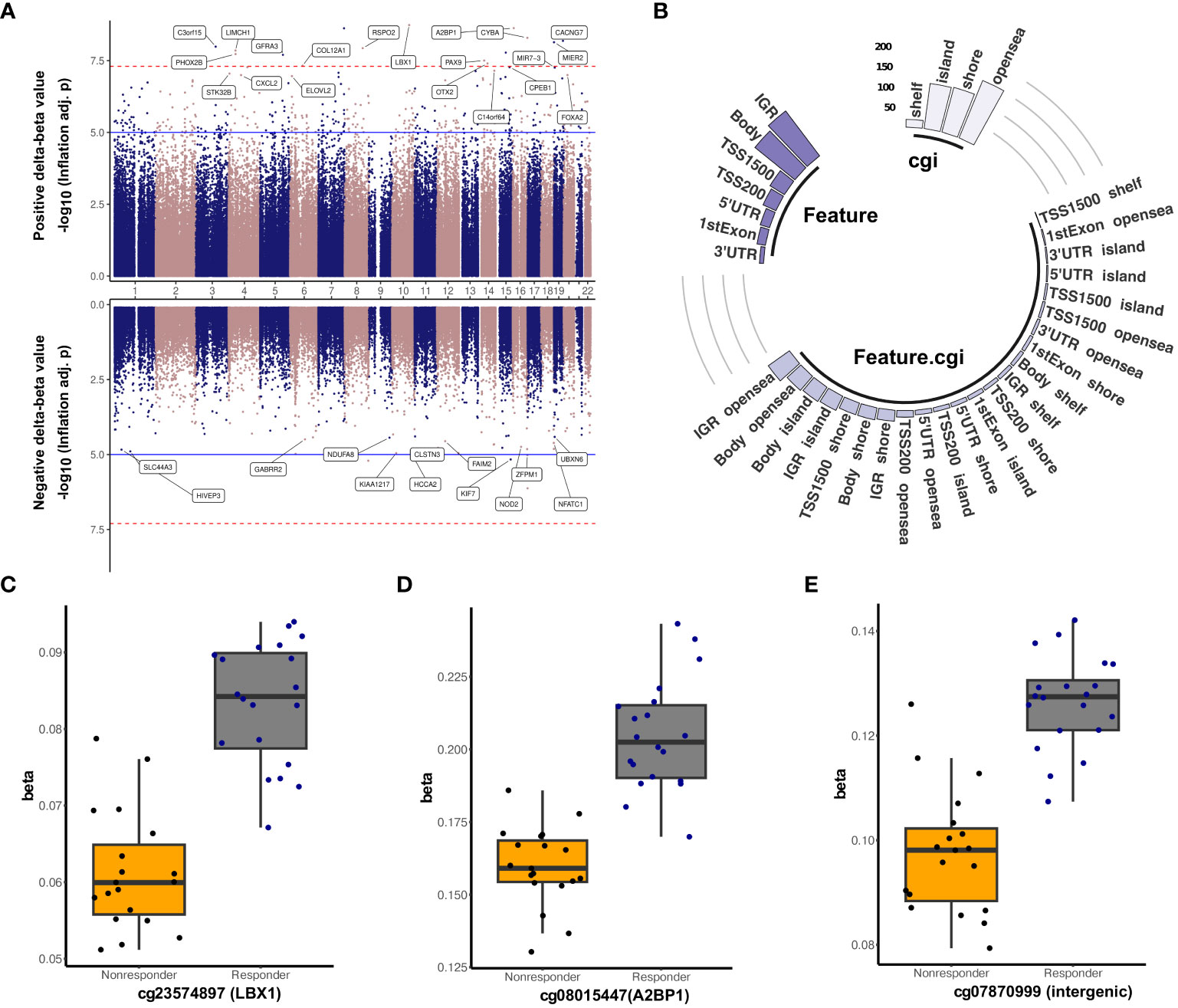
Figure 2 Differentially methylated sites marking treatment response. (A) Miami plot illustrating differentially methylated probes between antipsychotic treatment responders and non-responders. The top panel represents the probes with a positive delta-beta value (|Δβ|) (hypermethylated), while the bottom panel presents the probes with a negative delta-beta value (hypomethylated). The blue line represents the p-value cutoff of 1e−05 (suggestive line), and the dashed red line denotes the p-value cutoff of 5e−8 (genome line). The top 20 CpG sites are labeled except for those probes located in the intergenomic region. (B) A circular bar plot depicting the distribution of significant CpG sites differentially methylated between responders and non-responders, with |Δβ| ≥ 5% into different genomic region features such as transcription start site (200 and 1,500 bp from start site), 1stExon, gene body, intergenomic region, 5′ and 3′ untranslated regions and different CpG island (CGI) classifications like island, open sea, shore, and shelf. Boxplot of the top three significant differentially methylated sites: (C) cg23574897, (D) cg08015447, and (E) cg07870999 associated with response.
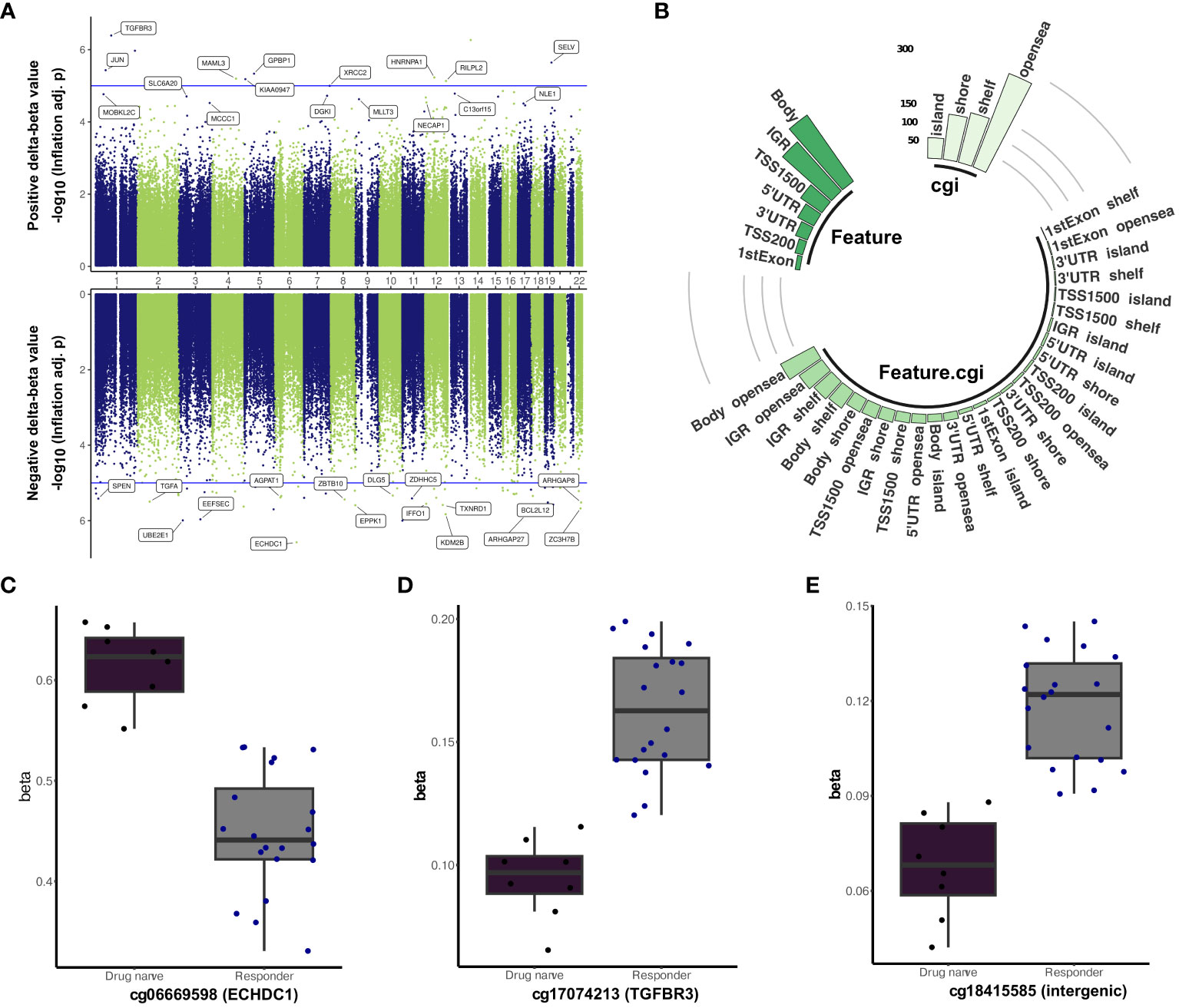
Figure 3 Differentially methylated sites marking treatment effectiveness. (A) Miami plot depicting the differentially methylated probes between antipsychotic responders and drug-naïve. The top and bottom panels represent positive and negative delta-beta values, respectively. The blue line indicates the p-value cutoff of 1e−05 (suggestive line), while the dashed red line represents the p-value cutoff of 5e−8 (genome line). (B) A circular bar plot showing the distribution of differentially methylated CpG sites between responders and drug-naïve patients with mean methylation difference above 5%. Boxplot of the top-ranked CpG markers linked with treatment effectiveness: (C) cg06669598, (D) cg17074213, and (E) cg18415585.
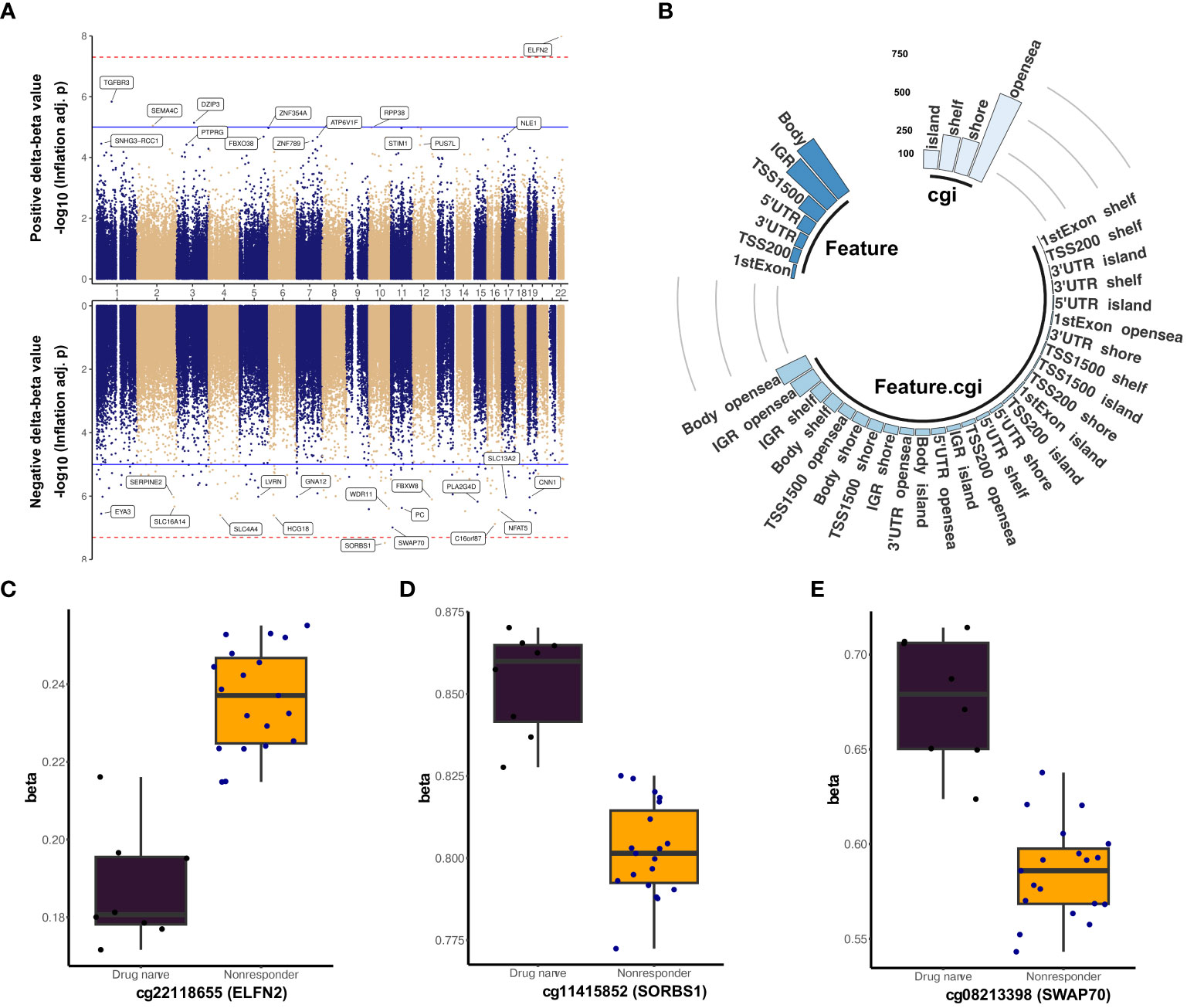
Figure 4 Differentially methylated sites marking treatment resistance. (A) Miami plot of differentially methylated sites identified from non-responders and drug-naïve comparison. The top and bottom panel denotes positive and negative delta-beta values, respectively. The blue line indicates the p-value cutoff of 1e−05 (suggestive line), and the red line represents the p-value cutoff of 5e−8 (genome line). (B) Distribution of differentially methylated CpG sites between non-responders and drug-naïve with mean methylation difference above 5% are represented in a circular bar plot. Boxplot showing the top three CpG markers of treatment resistance: (C) cg22118655, (D) cg11415852, and (E) cg08213398.
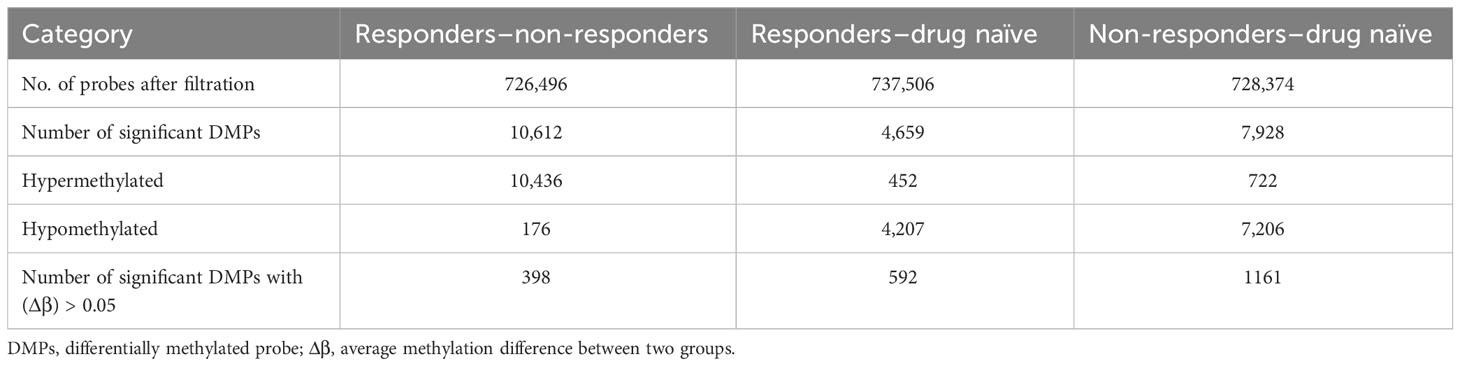
Table 1 Distribution pattern of the significant differentially methylated sites identified in various comparisons.
3.1.1 Methylation signatures differentiating the treatment responders and non-responders
Differential DNA methylation analysis following the adjustment of covariates such as age, sex, count of B cell, neutrophil, CD8T, natural killer, and monocytes (Supplementary Figure S1A) we identified 10,612 differentially methylated probes (FDR-adjusted p-value <0.05) (Table 1, Figures 2A, B and Supplementary Table S4) with an inflation of 1.4 and bias of 0.35 (Supplementary Figure S2). After the Bonferroni correction, 380 CpG sites remained significant, and the top three sites were cg23574897 (LBX1, TSS200, |Δβ| = 0.02, Bonferroni adj. p-value = 9.62E−05), cg08015447 (A2BP1, 5′UTR, |Δβ| = 0.04, Bonferroni adj. p-value = 1.13E−04), and cg07870999 (intergenic, |Δβ| = 0.03, Bonferroni adj. p-value = 1.14E−04) (Figures 2C–E). Among them, 398 loci exhibited a mean methylation difference (Δβ) greater than 5%, and the distribution pattern of these selected loci revealed enrichment within the open sea region (38.44%) as shown in Figure 2B. Probe distribution displayed a higher prevalence in the intergenomic region (34.92%) compared to the gene body (31.91%) and promoter (24.62%), with a predominant localization on chromosome 1 (12.56%). Furthermore, a substantial proportion of probes (n = 396) displayed significantly elevated DNA methylation levels in treatment responders. The probe, cg08617160, situated within the promoter region of the transcriptional repressor gene MIER2 exhibited the most significant hypermethylation, evidenced by its inflation-adjusted p-value of 7.24E−09. Following in significance were cg00630212 in the promoter of inflammatory gene CXCL2 (Inflation adj. p-value = 1.01E−07) and cg21184711 within the gene body of CADPS2 (Inflation adj. p-value = 1.18E−07) (Supplementary Figures S3A–E). Notably, seven hypermethylated probes were mapped to the genomic coordinates of the filamin A binding protein-encoding gene, FILIP1, followed by PM20D1 (n = 6), GALNT9 (n = 5), FMOD (n = 4), and SLC17A9 (n = 4). The highest group-wise methylation differences were observed for cg20415053 (CATSPER4, body, |Δβ| = 0.17), cg19637330 (intergenic, |Δβ| = 0.16), and cg09044981 (CDH13, body, |Δβ| = 0.13) (Table 2, Supplementary Figures S4A–C). Furthermore, enrichment analysis of hypermethylated probes identified associations with specific trait categories, including childhood stress, maternal factors, folate supplementation, ethnicity and gestational events (Supplementary Table S5). In contrast, only two hypomethylated sites, cg11309454 annotated to CCBL2 and cg07679219 associated with E2F7, displayed mean DNA methylation differences above 5% (Supplementary Figures S3D, E).
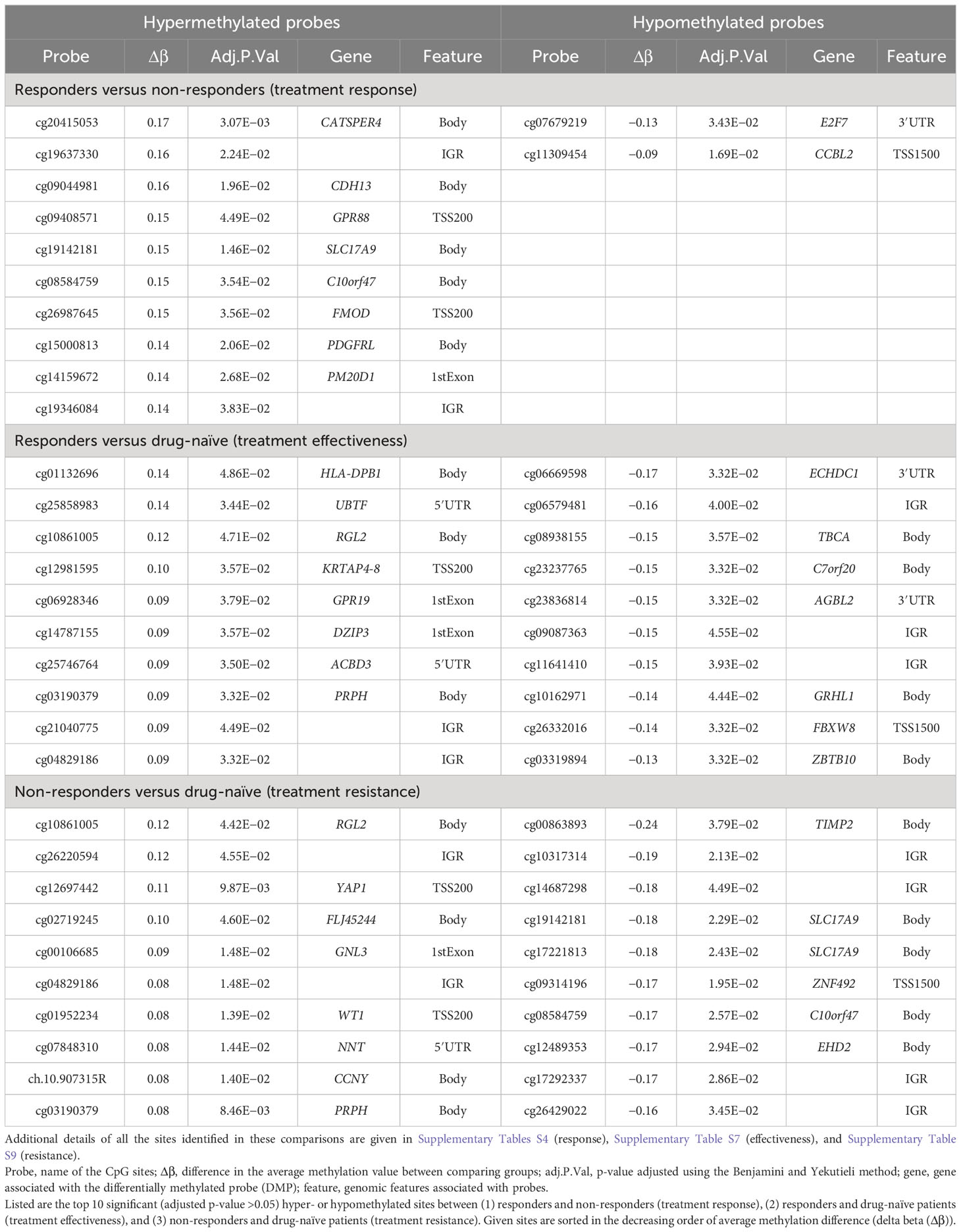
Table 2 Top differentially methylated positions associated with treatment response, effectiveness, and resistance.
DMR analysis identified 1,072 regions (mapped to 1,176 genes), characterized by at least seven significantly different probes between the targeted groups, and 96.08% (1030) of these DMRs were independently replicated through DMR analysis using DMRcate (Supplementary Table S6). CTNNA2 (32 probes) and IGF2 (53 probes) were the most represented regions, harboring three distinct DMRs each. Notably, the most significant DMR, located distally intergenic to TBX3, harbored 55 DMPs. The majority of identified regions (97.29%) reside within gene promoters, and 12.24% of the genes associated with these DMRs have been previously implicated in schizophrenia susceptibility.
3.1.2 Identifying the markers for treatment effectiveness
To elucidate the methylomic underpinnings of antipsychotic efficacy and symptom improvement, a comparative analysis of DNA methylation profiles between drug-responsive and drug-naïve patients was performed. After covariate adjustment for family history, age, Sex, CD8T and neutrophil cell composition differential methylation was identified in 4,659 sites (FDR < 0.05) (Figures 3A, B, Supplementary Table S7). The estimated inflation factor and bias were 1.5 and −0.074, respectively (Supplementary Figure S5). Eleven CpG sites remained significant after the Bonferroni correction (p-value <0.1), and the top three significant sites were cg06669598 (ECHDC1, 3′UTR, Δβ = −0.17, Bonferroni adj. p-value = 0.02), cg17074213 (TGFBR3, 1stExon, Δβ = 0.07, Bonferroni adj. p-value = 0.04), and cg18415585 (intergenic, Δβ = 0.05, Bonferroni adj. p-value = 0.05) (Figures 3C–E). Within the singificant DMPs, 592 sites showed average DNA methylation difference exceeding the threshold (|Δβ| > 5%). These highly variable sites were mainly positioned within the open sea region with 46.11% and 92.06% of them displaying reduced DNA methylation in responders (Figure 3B). The hypermethylated probe cg01132696 in the gene body of HLA-DPB1 exhibited the greatest mean methylation change (|Δβ| = 0.14), followed by cg25858983 (UBTF, |Δβ| = 0.14) and cg10861005 (RGL2, |Δβ| = 0.12) (Supplementary Figures S6A–C). Conversely, cg06669598 in the 3′UTR of ECHDC1 was identified as the most varied hypomethylated site, followed by cg06579481 (intergenic, |Δβ| = 0.16) and cg08938155 (TBCA, |Δβ| = 0.15) (Supplementary Figures S6D–F).
In a comparative analysis between drug-responsive and drug-naïve individuals, 1,381 DMRs were identified, and 1,450 (95.24%) of these regions were reproduced by independent DMR analysis using DMRcate (Supplementary Table S8). Among the significant DMRs, the top three regions were located in the BRD2 (62 DMPs), KLLN (50 DMPs), and MRPS18B/PPP1R10 (35 DMPs) genes. Effectiveness-related regions were annotated to 1,929 genes, with 142 (7%) previously implicated as schizophrenia susceptibility loci in the GWAS catalog, suggesting a potential link between differential methylation and genetic predisposition to schizophrenia in the context of antipsychotic response.
3.1.3 Identifying the markers for treatment resistance
Differential methylation analysis between non-responders and drug-naïve individuals identified 7,928 CpG sites (Figures 4A, B, Supplementary Table S9) with significant differences in DNA methylation levels (FDR < 0.05) with estimated inflation of 1.5 and bias −0.016 after controlling for the covariates sex, family history, neutrophil, CD4T, CD8T, NK, and B-cell count (Supplementary Figure S7). However, only 88 sites were found significant after the Bonferroni correction (p-value <0.1), and the top three sites were the following: cg22118655 (ELFN2, TSS200, Δβ = 0.05, Bonferroni adj. p-value = 1.57E−03), cg11415852 (SORBS1, 5′UTR, Δβ = −0.05, Bonferroni adj. p-value = 3.01E−03), and cg08213398 (SWAP70, Body, Δβ = −0.09, Bonferroni adj. p-value = 6.26E−03) (Figures 4C–E). A large share of the sites (n = 1,118) with average DNA methylation levels greater than 0.05 (n = 1,161) displayed decreased DNA methylation in non-responders. These resistance DMPs were dispersed across the open sea region, occupying 50.04% of the identified loci (Figure 4B). Moreover, these DMPs showed enrichment within the gene body (35.75%) and chromosome 1 (10.51%). RGL2 harbored the most varied hypermethylated probe, cg10861005, with a |Δβ| of 0.12 (Table 2), followed by cg26220594 (intergenic, |Δβ| = 0.12) and cg12697442 (YAP1 |Δβ| = 0.1) (Supplementary Figures S8A–C). Likewise, hypomethylated probes cg00863893 (TIMP2, |Δβ| = 0.24), cg10317314 (intergenic, |Δβ| = 0.19), and cg14687298 (intergenic, |Δβ| = 0.18) displayed the highest between-group differences in the DNA methylation level (Supplementary Figures S8D–F). C13orf26 harbored the most number of hypomethylated sites within its coordinates (n = 5), followed by SLC17A9 (n = 4), PTPRN2 (n = 4), ARHGEF10, TNXB, ADAM5P, C6orf10, PKP3, and SDK1 (n = 3 each).
Differential methylation analysis revealed 892 resistance-related regions mapped to 1,211 genes, with 97.53% (n = 870) of these regions being replicated through independent analysis using DMRcate (Supplementary Table S10). The top three significant regions were annotated to FAM132A, BRD2, and HOXA4, while the resistance DMRs containing a maximum number of CpG probes were represented by genes such as BRD2 (n = 47), BAT2 (n = 33), GPR75-ASB3 (n = 30), HLA-DPB2 (n = 30), and LOC100302652 (n = 30). Furthermore, three differentially methylated regions were reported in the BAT2 gene. Interestingly, only 82 of the DMR genes have been previously implicated as schizophrenia risk loci.
3.1.4 Gene ontology
Gene ontology analysis of DMPs for the treatment response group identified enrichment for cellular processes linked to cell adhesion, nervous system development, and cell-to-cell signaling (Figure 5A, Supplementary Table S11). In contrast, the DMR genes are enriched for processes such as neuron differentiation, pattern specification, regionalization, and specific KEGG pathways including “neuroactive ligand-receptor interaction” (FDR = 4.93E−15) and “axon guidance” (FDR = 0.02) (Supplementary Table S12). Additionally, an overrepresentation of disease–gene networks pertaining to drug dependence and substance-related disorders was observed for the DMR genes (Figure 5B, Supplementary Table S13).
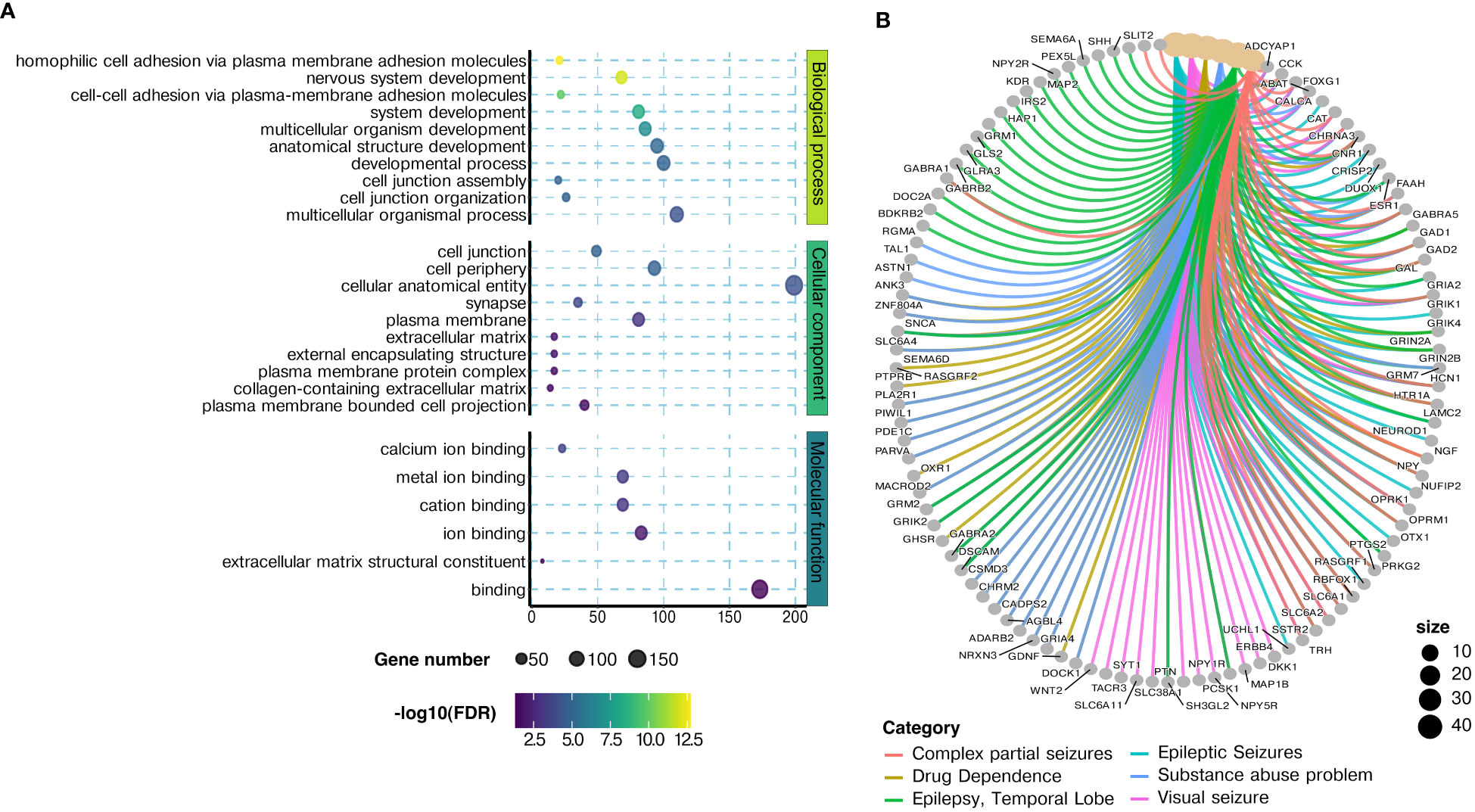
Figure 5 Gene ontology terms related to differentially methylated probes. (A) Bubble graph of the top 10 ontology terms enriched for the significant differentially methylated sites with |Δβ| ≥ 5% between the responders and non-responders. The X-axis denotes the number of differentially methylated genes associated with gene ontology (GO) terms, and the Y-axis displays the GO terms of the categories: biological process (BP), cellular component (CC), and molecular function (MF). The color of the bubble denotes the −log10 (p-value) of the significance of enrichment, and the size represents the count of differentially methylated probe (DMP) genes. (B) Cnet plot of ontology terms enriched with a p-value <0.05 after differentially methylated region (DMR) gene–disease association enrichment analysis. Top six ontology terms and the genes associated with each term are depicted in the circular plot, and the node size denotes the number of genes in each category.
Gene ontology analysis of DMPs for treatment effectiveness identified enrichment for terms connected to various cellular processes involved in cellular communication and its regulation (Supplementary Table S14), while the key ontology terms related to DMR-associated genes included RNA catabolism, ribosome subunit synthesis, arrangement, and assembly, suggesting a potential role of altered epigenetic regulation in these processes contributing to treatment effectiveness (Supplementary Table S15). Similarly, pathway analysis revealed a significant association between DMRs linked to effective antipsychotic response and genes involved in the ribosome pathway (FDR = 7.59E−03).
Gene ontology analysis of DMPs for the treatment resistance group displayed enrichment for processes related to DNA methylation, miRNA metabolism, and its regulation (Supplementary Table S16). Enrichment of pathways such as metabolism of riboflavin (p-value = 8.95E−04) and biosynthesis of mucin-type O-glycan (p-value = 2.21E−02) was found unique to resistance DMPs, although it was not significant after FDR correction. Additionally, the resistance DMRs displayed enrichment of pathways related to spliceosomes (FDR = 5.53E−03) and GO terms like DNA synthesis, non-coding RNA processing, and metabolism of RNA, rRNA, and piRNA (Supplementary Table S17). Several sites were observed to overlap across the comparisons, as depicted in the Upset plot (Figure 6), and this was reflected in the overlap between GO terms (FDR > 0.05) between the resistance DMPs and treatment-effective DMPs (overlapping p-value = 2.7e−138, Jaccard index = 0.3). The shared list of terms includes cell adhesion, communication, and regulation.
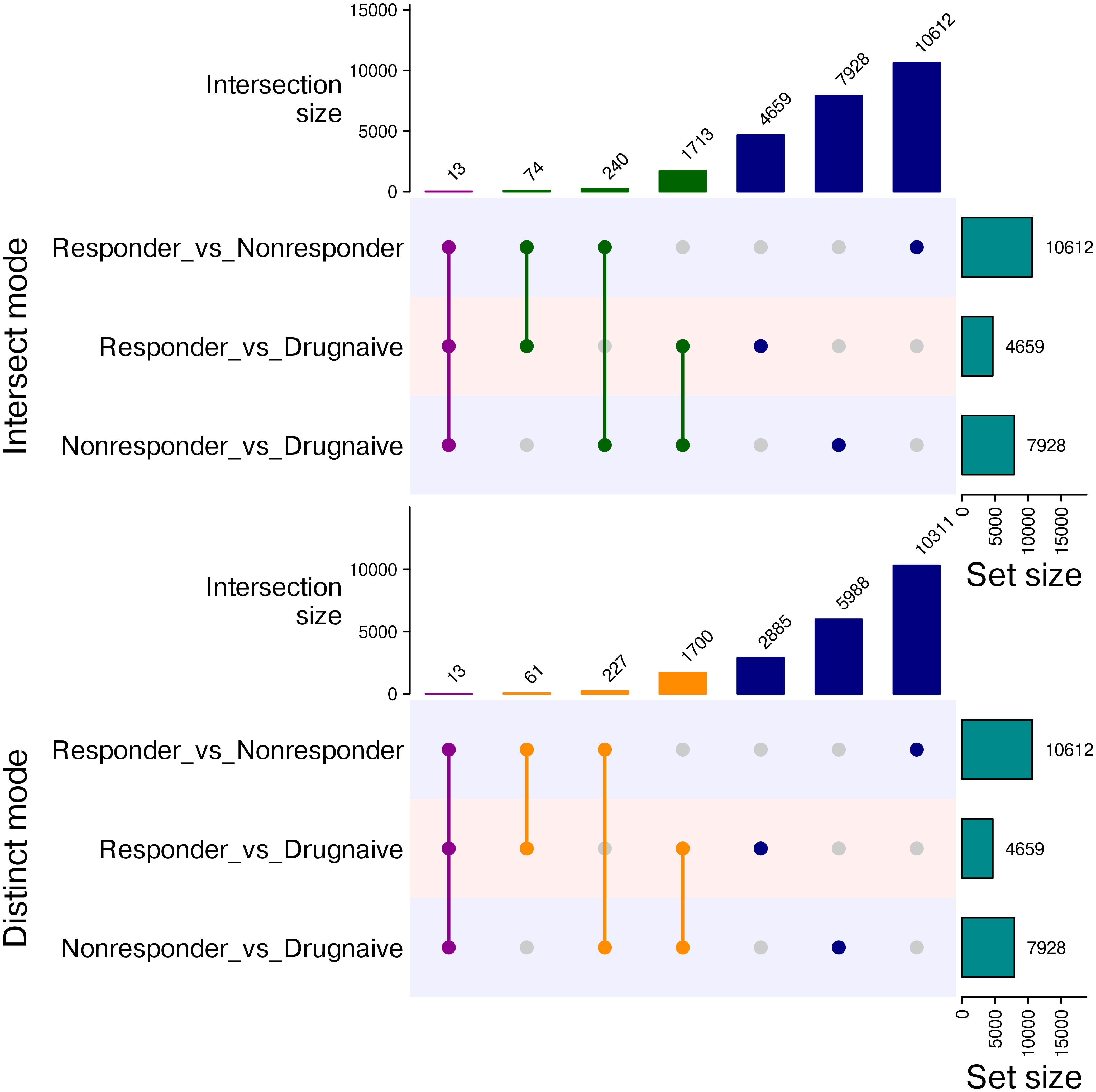
Figure 6 Overlapping differentially methylated sites between various comparisons. Upset plot showing the number of overlapping and distinct CpG sites in the comparisons between (1) responders and non-responders, (2) responders and drug-naïve patients, and (3) non-responders and drug-naïve patients; the bar graph on the side shows the total number of differentially methylated probes (DMPs) in each comparison.
3.1.5 Protein–protein interaction network
STRING analysis of protein encoded by treatment response DMR genes revealed a high degree of connectivity; therefore, Cytoscape plugin MCODE was used to identify subnetworks with a combined score >0.6, and two distinct clusters centered around proteins associated with GABAergic signaling: GABRA4 (GABA receptor subunit) and GAD1 (glutamate decarboxylase enzyme) with node score >5 were identified.
The DMPs of treatment effectiveness formed a single network, with the ribonucleoprotein SNRPD3 acting as the central seed protein. In contrast, DMR-associated proteins formed 12 distinct modules with ribosomal protein RPS6, nucleosomal protein CENPA, and transcription coactivator protein MED22, serving as the seed for the top clusters.
Similarly, treatment resistance DMPs formed a single cluster with ribosomal protein RPL3 as seed. However, DMR proteins formed nine clusters, and the top three were centered around ribonuclease RPP38, mismatch repair protein MLH1, and ATP5PF. CytoHubba was used to identify and rank the hub proteins from proteins encoded by DMP and DMR: DMR-associated genes were unique to effectiveness and resistance (Figures 7A, B). Notably, ribosomal proteins RPS6, RIL16, and RPL11 were recognized as the hub protein among the effectiveness markers. Conversely, histone modifiers HDAC4, HDAC3, and nucleosome component H2BC5 were ranked in the top three positions connected to resistance DMR markers. The results of the protein–protein interaction (PPI) analysis of all DMP- and DMR-associated genes for all three comparisons are given in Supplementary Table S18.
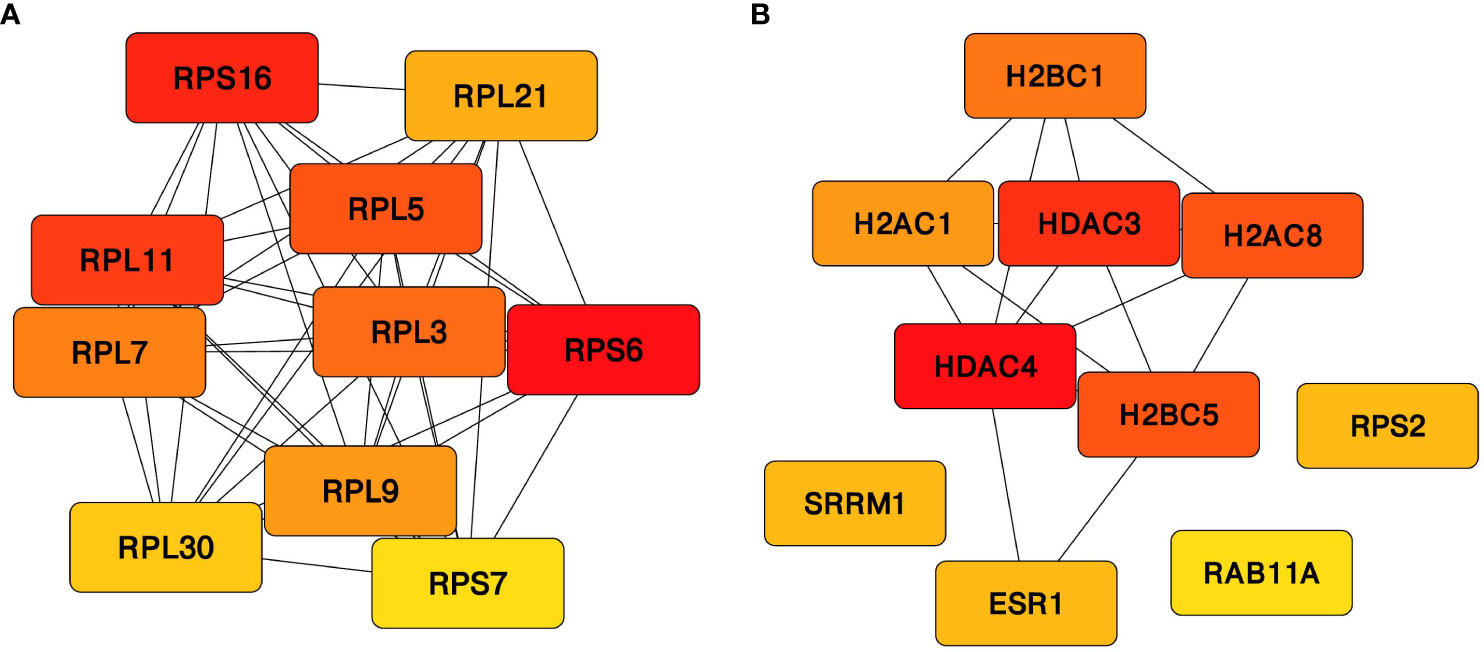
Figure 7 Hub proteins. Top 10 hub proteins identified from the unique genes associated with the regions differentially methylated between the (A) responder and drug-naïve patients and (B) non-responder and drug-naïve patients using Maximal Clique Centrality (MCC) algorithm. Directionality of the ranking is represented by the color gradient ranging from red (ranked first) to yellow.
3.2 Methylation quantitative trait loci associated with treatment response based on GSA
To investigate variants that influence DNA methylation signatures and modulate treatment response, we performed a meQTL analysis and identified 23 cis and trans CpG–SNP pairs (FDR ≤ 0.1) associated with the significant DMPs related to the antipsychotic treatment response. We demonstrated that based on SNPs mapped in the study, our population groups are of South Asian ancestry (Supplementary Figure S9). These reported cis-meQTLs were positioned within 50 kb of 17 significant DMPs, while the trans-meQTLs were linked with four CpG sites (cg07796745, cg15035716, cg03073851, and cg27380599). The two cis-meQTLs involved missense variants: rs36092215 (cg21934190, GPR20) and rs6657616 (cg08133631 and cg20415053, CATSPER4). Although these meQTLs were not directly associated with treatment response or schizophrenia risk, the DNA methylation level at cg22705746 (FMOD, TSS1500) and cg26987645 (FMOD, TSS200) were influenced by rs10494841, which is in LD with variants rs16851364 and rs4971252 that are reportedly associated with serum fibromodulin levels. All eQTLs related to response-related cis- and trans-DNA methylation quantitative trait loci are given in Supplementary Tables S19-21.
3.3 Effect of antipsychotic drugs on the mRNA level of differentially methylated genes
We investigated the expression pattern of some of the critical differentially methylated (|Δβ| ≥ 0.10) genes expressed in PBMC to study the impact of drug-induced DNA methylation. We examined their expression changes after clozapine, olanzapine, risperidone, and haloperidol treatment (Table 3, Supplementary Figures S10-13). We observed a varying expression pattern of the response markers SET, ECHDC1, GET4, TBCA, ZFAND2A, CORO1C, and C7orf50 to the antipsychotic drugs. Clozapine and haloperidol treatments significantly elevated the expression of most response markers. We then checked the variance in mRNA levels of classes I histone deacetylases (HDAC1, HDAC2, and HDAC3), class IIa (HDAC 9), class IIb (HDAC6), and class III (SIRT2), histone acetylase (KAT5), and methyltransferases (KMT2E, KDM5A, PRMT1, and PRMT5). Olanzapine promoted concentration-dependent gene expression of all histone deacetylase genes, while risperidone normalized HDAC expression. Although haloperidol, clozapine, and risperidone raised KAT5 expression, only the haloperidol treatment was significant. Similarly, haloperidol treatment introduced a significant upregulation of KDM5A. Clozapine, haloperidol, and olanzapine elevated the KMT2E transcript with increasing concentration. PRMT1 expression increased significantly with increased concentrations of clozapine, risperidone, and olanzapine; at the same time, PRMT5 showed a concentration-dependent increase with clozapine, olanzapine, and haloperidol treatment. We also explored the influence of antipsychotic medications on the mRNA levels of human leukocyte antigen (HLA) genes such as HLA-A, HLA-B, HLA-C, HLA-E, HLA-DPA1, HLA-DPB1, HLA-DQA1, HLA-DQB1, HLA-DRA, and HLA-DMB. The gene expression profile indicates the upregulation of class I HLA and the downregulation of class II HLA, with clozapine having the maximum impact, followed by haloperidol and risperidone.
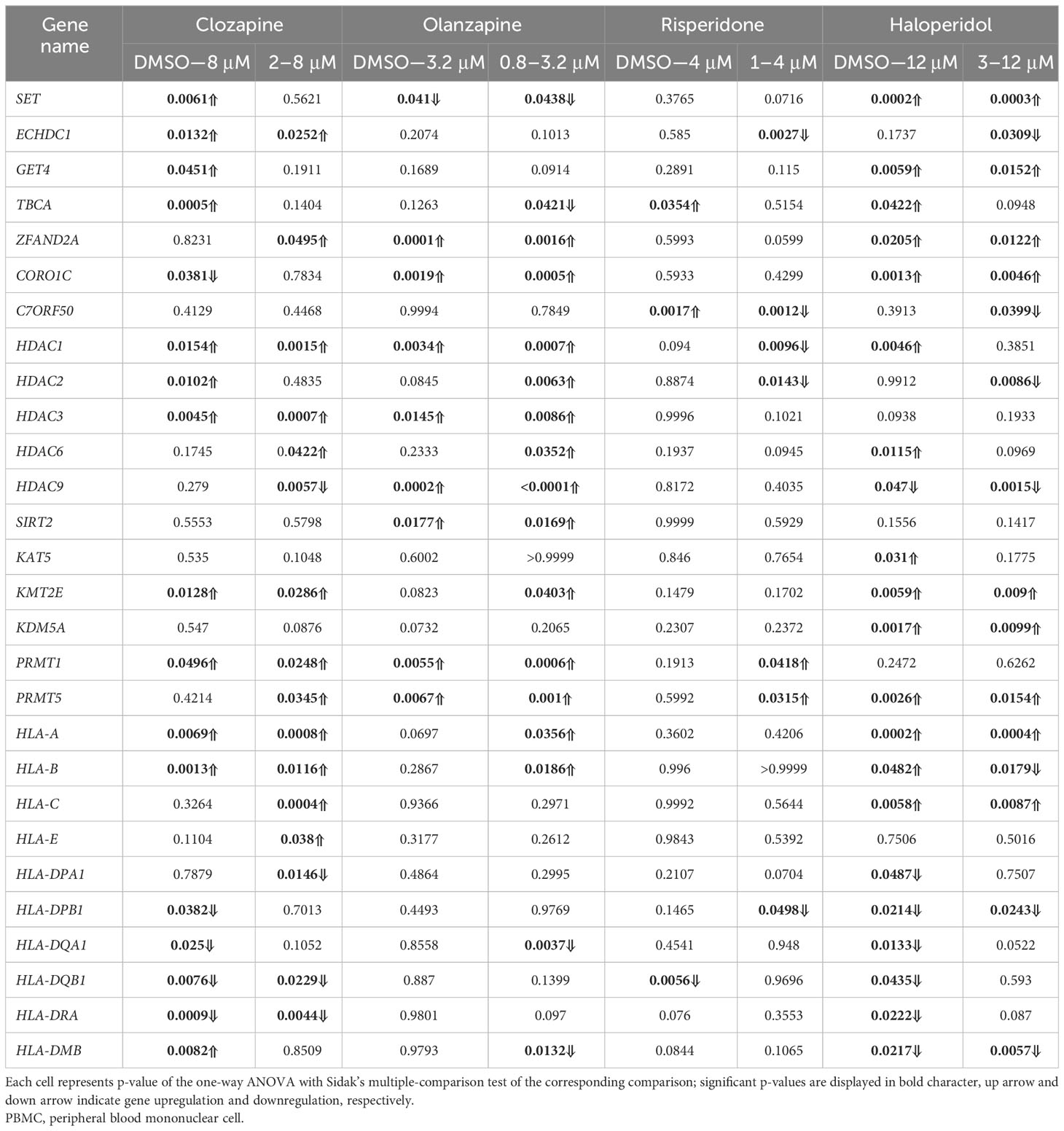
Table 3 Effect of antipsychotic drugs on the mRNA level of treatment response, histone modifiers, and human leukocyte antigen genes in PBMC.
4 Discussion
The current study represents the first genome-wide DNA methylation study ever conducted in an Indian population aimed at understanding the epigenetic markers of schizophrenia pathology and distinguishing them from markers of therapeutic response and resistance. Antipsychotic drugs target neurotransmitter pathways and mediate therapeutic responses. However, the lack of consistent observations and failure to relate response to genetic variants in these neurotransmitter pathways (57) strongly indicate alternate mechanisms, like epigenetics, for therapeutic response. The previous epigenetic studies employed peripheral blood or post-mortem samples from patients with conventional treatment backgrounds, potentially influencing the identification of pathogenesis-related epigenetic markers due to the modulatory effect of antipsychotics on DNA methylation (58). In a clinical scenario, it is difficult to control the medication and test for its impact on the host epigenome; therefore, to overcome this challenge, we conducted methylome-wide studies on schizophrenia patients by stratifying them into responders, non-responders, and drug-naïve patients. The study identified several unique DMPs and DMRs as methylomic markers for drug response, effectiveness, and refractoriness.
We investigated the impact of conventional treatment protocol on the methylome and found that the state of hypermethylation is largely implicated in drug response and hypomethylation with drug resistance or non-responsiveness. This was also evident in an earlier observation based on the overexpression of DNMT genes and its resultant global hypermethylation upon antipsychotic treatment (24). The methylome-wide observations on treatment responder and non-responder groups when compared with previous studies on case–control DNA methylation variations (18, 20, 22, 59–61) had contrasting and conflicting DNA methylation patterns (hyper and hypo) and the direction of DNA methylation difference (hypo/hyper or hyper/hypo) (Supplementary Table S22). Therefore, it is important to carefully assess the medication-induced methylomic alterations for their favorable effect on etiology, course of illness development, intensity of symptoms, adverse reactions, and refractoriness.
A comprehensive understanding of the extent of differences between responder and non-responder DNA methylomes is necessary to depict treatment response heterogeneity accurately. The most significant probe identified in the study was localized to LBX1, a transcription factor encoding gene that is essential for GABAergic differentiation in the dorsal spinal cord (62) and midbrain dopaminergic neuron specification, potentially through the regulation of cholesterol biosynthesis (63). Another significant probe was localized to A2BP1, which is a schizophrenia risk gene and reported to have a reduced expression in the cortical regions of schizophrenia patients (64) and genetic association with olanzapine-induced weight gain in the Chinese Han population (65), suggesting its involvement in both pathogenesis and treatment response. The most significant hypermethylated response-linked DMP was found within the MIER2 gene, encoding a nuclear protein that recruits HDACs (66). Interestingly, MIER2 hypomethylation has been reported in relation to fetal membrane infection (67). In addition, traits associated with hypermethylated response DMPs hint at the significance of early environmental insults occurring at the gestational stages or during childhood in shaping DNA methylation patterns and heightening the susceptibility to schizophrenia. We observed multiple response-related probes aligning with genes involved in neuronal development and function, including FILIP1, PM20D1, and GALNT9. Hypermethylation of FILIP1, a filamin A-binding protein regulating cortical neuron migration and dendritic spine morphology, is significant, as it could impair these major processes (68, 69). Similarly, PM20D1, involved in the amide biosynthesis and regulation of neuron death, has been related to enhanced glucose homeostasis in mice (70) and also DNA methylation changes in PM20D1 linked to PTSD and Alzheimer’s disease (71, 72). Another top-hit hypermethylated gene, GALNT9, has been implicated in schizophrenia through glycosylation dysregulation (73). Interestingly, first-episode schizophrenia patients undergoing risperidone treatment reported differential methylation in the gene region involved with O-linked glycosylation; also, first-episode drug-naïve schizophrenia-associated differential methylation marker cg15150970 was replicated in our study between responders and non-responders (74). Several of the peripheral tissue-based DNA methylation patterns associated with treatment response were found to parallel the brain pattern (Supplementary Table S23).
Several cell adhesion molecules belonging to the protocadherin cluster were found to be differentially methylated between responsive and non-responsive subjects. Cell adhesion molecules coordinate the trafficking of immune components across the blood–brain barrier, which is deregulated in psychosis conditions (75, 76). We have reported the involvement of proinflammatory factors in schizophrenia (77), and interestingly, antipsychotics are known to influence the immune response (78). The association of DNA methylation differences around the cell adhesion molecule cadherin with treatment response stresses the role of antipsychotic-mediated regulation of peripheral inflammation and its long-range effect on the neuronal tissues. It has been reported that cadherin expression can be regulated by the hsa-miR-29b-3p/DNMT3b/MMP-9 pathway in human brain microvascular endothelial cells (79), and interestingly, miR-29b and DNMT expression have been reported to be influenced by antipsychotic drugs (24).
Our analysis identified multiple DMRs mapping to IGF2 and CTNNA2. CTNNA2, encoding an actin regulator, α-catenin, is important for neuronal migration and neurite length (80), and an intronic variant within this gene serves as a biomarker for lurasidone response in the European population (81). Moreover, DNA methylation difference in the CTNNA2 probe has also been reported in monozygotic twins discordant for psychosis (82). These findings suggest that while certain genetic markers are significant, the same gene might still be affected by an epigenetic trade-off, functioning as a substitute even in the absence of such markers. Association between IGF2 enhancer hypomethylation in prefrontal cortex neurons and increased tyrosine hydroxylase protein has been established in schizophrenia and bipolar patients, indicating epigenetic regulation of dopamine synthesis through IGF2 methylation (83). This aligns with the reports of IGF2 downregulation in the dorsolateral prefrontal cortex (84) and lower IGF2 serum levels in schizophrenia patients (85). In addition, the serum level of IGF2 is negatively correlated to the negative symptoms in patients while being positively associated with working memory and executive function, highlighting the importance of IGF2 in memory dysfunction (85).
The top hits on the GO terms for response indicated the role of synaptic interaction, glycosylation, extracellular matrix components, histone modifications, and cell-to-cell adhesion; interestingly, these cellular components were also reported to be implicated in schizophrenia (12, 86). Our findings revealed a directional overlap between pathogenesis and therapeutic response when they were compared over previously reported pathogenesis markers, indicating the necessity for a rigorous assessment of the DNA methylation markers of pathogenesis for the epigenetic indicators of treatment response. Understanding the trade-offs between genetic and epigenetic findings is also necessary.
We observed increased DNA methylation in drug-naïve subjects, while individuals under medications displayed reduced DNA methylation, aligning with previous studies (23). Drug-mediated changes in the methylome can have diverse on- or off-target effects, influencing the treatment efficacy by modulating the non-target gene. Comparison between responders and drug-naïve patients revealed several off-target effects of antipsychotics with hypermethylation at sites within HLA-DPB1, UBTF, and RGL2 in responders. The HLA-DPB1 has been linked to clozapine-induced agranulocytosis (87, 88). The most significant hypomethylated sites were observed in metabolic repair enzyme, ECHDC1, and a transmembrane proteoglycan encoding gene TGFBR3, which is known to take part in neuronal differentiation (89). Earlier studies in the blood of schizophrenia patients have identified DMPs within TGFBR3 (90) and reported its downregulation (91). The hypermethylation status of HLA-DPB1 and TGFBR3 may indicate a positive response, while ECHDC1 hypomethylation specifies metabolic stress upon antipsychotic use. Therefore, careful monitoring of antipsychotics can help differentiate the positive and negative effects of immunomodulatory and metabolic events.
While assessing the differential DNA methylation markers between the drug-naïve and non-responders, we observed hypomethylation as a dominant signature associated with treatment resistance, with genes like ELFN2, SORBS1, SWAP70, PTPRN2, and TIMP2 being the top hits. Interestingly, ELFN2, a postsynaptic cell adhesion molecule, hypermethylated in non-responders has been previously linked to neuropsychiatric behavior (92). SORBS1, a major player in the insulin signaling pathway, displayed increased expression in schizophrenia patients with high inflammation (93, 94). Resistance-related hypomethylation in our study corroborates the damaging effects of schizophrenia risk genes TIMP2 (95) and SORBS1 on aggravating pathology. In contrast, SWAP70 involved in the cell adhesion suppressed inflammatory responses (96). PTPRN2, an inflammation marker, was reportedly found to be differentially methylated in response to risperidone treatment (74, 97). Similarly, the resistance marker cg17221813, located in the gene body of the transport protein SLC17A9, has been associated with treatment-resistant schizophrenia (98). Collectively, this study highlights the significance of differential DNA methylation in metabolic and inflammatory pathways in driving non-response and possibly drug-induced side effects.
GO keywords related to cellular communication and regulation were repeated in treatment effectiveness and resistance comparisons, reflecting the major mechanism of drug action (99). Notably, pathway and network analyses revealed a strong correlation between DMRs associated with an effective antipsychotic response and genes implicated in the ribosome pathway, providing more evidence to support the hypothesis that treatment efficacy may be influenced by epigenetic modulation of ribosome-related genes (100). Interestingly, HDAC proteins were ranked top among resistance DMR proteins, suggesting their potential role in treatment resistance.
A few top-hit DMPs were further assessed for their impact on gene expression by grouping them into response markers, histone modifiers, and HLA markers. Interestingly, response markers and histone modifiers show increased expression in most drug treatments, while in HLA, the class I HLA markers show increased expression in sharp contrast to the decreased expression of HLA class II gene expression. Response markers like SET (101), ECHDC1 (102), GET4 (103), TBCA (104, 105), ZFAND2A (18, 106), CORO1C (107), and C7orf50 (108) are known to impact schizophrenia, neuronal function, cytoskeleton dynamicity, or brain metabolism. Therefore, differential expression upon antipsychotic treatment provides new insight into the downstream effect of drug-mediated methylomic changes, and it indicates that antipsychotic drugs can reverse the pathology by influencing gene expression or impacting DNA methylation patterns. HDACs have a regulatory role in synaptic plasticity, neurodegeneration, and cognitive function (109), and decreased expression of class I HDAC has been reported in schizophrenia (110, 111). Most studies on HDAC dysregulation in the brain come from postmortem brains, unsure of the responder or non-responder category. Such observations need to be relooked, as we observed hypomethylation of HDAC9 among the resistance markers.
In recent times, the HLA region has gained significance because of its role in schizophrenia, its comorbid conditions, and the adverse effects of antipsychotic drugs. DNA methylation analysis indicated that HLA-DPB1 hypermethylation and HLA-E hypomethylation were largely associated with a good therapeutic response, while non-responders displayed hypomethylation in HLA-DPB2, HLA-DMA, and HLA-DMB. Furthermore, the gene expression profiles of HLA genes post-antipsychotic treatment indicate that class I genes are often upregulated, while class II genes are often downregulated, with clozapine having the maximum impact, followed by haloperidol and risperidone. Studies have indicated that HLA genes are also associated with schizophrenia (112–115). Certain HLA alleles, such as HLA-DRB1*04:02, HLA-DPB1*05:02, HLA-DQB1 (126Q), HLA-B (158T), and HLA-B*59:01, have been reported to be associated with clozapine-induced agranulocytosis (116–118). The majority of these studies were restricted to identifying genetic markers of schizophrenia or clozapine response, but possibly the DNA methylation and gene expression patterns of HLA genes may help in understanding the precise role of antipsychotics in mediating therapeutic response and distinguishing them from drug-induced side effects.
The current study is possibly the first comprehensive study on the DNA methylation signature of therapeutic response in schizophrenia, backed by genomic and gene expression profiles of some of the most critically methylated genes. Some key processes that could help us distinguish the response from non-response include alterations in neurodevelopment, immune system, ribosome biogenesis, cell-to-cell adhesion and signaling, and metabolic changes. The meQTL analysis underlines the gene–environment interplay in complex disorders to create heterogeneous phenotypes; therefore, we performed the study on a genetically and epigenetically homogenous Malayalam-speaking population to minimize the variables in a complex condition like schizophrenia. Many of the signatures listed in our study are schizophrenia risk loci, indicating that specific genetic hallmarks are essential. However, even without genetic markers, epigenetic trade-offs can influence the same gene and act as a surrogate. The study needs replication with a larger sample size and cross-validation with other ancestral and epigenetic backgrounds, environmental variables, antipsychotic use, pathology, and comorbid conditions. A precise drug-specific DNA methylation signature may further help in differentiating the individual effects of each drug on multidrug therapy that drive patients into treatment response, treatment effectiveness, and treatment resistance.
Data availability statement
All data pertaining to the manuscript is attached in the supplementary files and additional files are also available through ZENODO repository 10.5281/zenodo.10719137.
Ethics statement
Institutional Ethics Committee of RGCB approved the study for biomedical subjects as per the ICMR and Helsinki protocol. The studies were conducted in accordance with the local legislation and institutional requirements. The participants provided their written informed consent to participate in this study.
Author contributions
BP: Data curation, Formal analysis, Investigation, Methodology, Validation, Visualization, Writing – original draft, Resources, Software, Writing – review & editing. NV: Data curation, Investigation, Methodology, Writing – original draft. IN: Data curation, Formal analysis, Investigation, Resources, Writing – original draft. CN: Conceptualization, Data curation, Formal analysis, Investigation, Resources, Supervision, Writing – review & editing. MB: Conceptualization, Formal analysis, Funding acquisition, Investigation, Methodology, Project administration, Resources, Supervision, Validation, Visualization, Writing – review & editing, Writing – original draft.
Funding
The author(s) declare financial support was received for the research, authorship, and/or publication of this article. The work was supported by SERB, and intramural support of RGCB from Department of Biotechnology.
Acknowledgments
BP acknowledges the Department of Biotechnology (DBT), Government of India, for providing the research fellowship. We also acknowledge the SERB and RGCB, Department of Biotechnology, for providing intra-mural support to carry out the work.
Conflict of interest
The authors declare that the research was conducted in the absence of any commercial or financial relationships that could be construed as a potential conflict of interest.
Publisher’s note
All claims expressed in this article are solely those of the authors and do not necessarily represent those of their affiliated organizations, or those of the publisher, the editors and the reviewers. Any product that may be evaluated in this article, or claim that may be made by its manufacturer, is not guaranteed or endorsed by the publisher.
Supplementary material
The Supplementary Material for this article can be found online at: https://www.frontiersin.org/articles/10.3389/fpsyt.2024.1297760/full#supplementary-material
Supplementary Figure S1 | Blood cell type comparison in each comparison Plot showing the difference in the proportion of blood cell types between various groups in each comparison, (A) responders and nonresponders, (B) responders and drug-naïve (C) nonresponders and drug-naïve; violin plot shows the distribution of value; and boxplot produces the summary statistics. X axis represents the type of cell population and the Y axis denotes the proportion of each cell type.
Supplementary Figure S2 | QQ plot of the treatment response-related differentially methylated CpG sites before and after adjusting for inflation and bias.
Supplementary Figure S3 | Boxplot of top three most significant hypermethylated (A) cg08617160 (B) cg00630212 (C) cg21184711 and hypomethylated (D) cg11309454 (E) cg07679219 sites between responders and nonresponders with |Δβ|≥ 5%.
Supplementary Figure S4 | Boxplot of the top three most differed hypermethylated (A) cg20415053 (B) cg19637330 (C) cg09044981 sites between responders and nonresponders with |Δβ|≥ 5%.
Supplementary Figure S5 | QQ plot of the treatment effectiveness-linked differentially methylated CpG sites before and after adjusting for inflation and bias.
Supplementary Figure S6 | Boxplot of the top three most differed hypermethylated (A) cg01132696 (B) cg25858983 (C) cg10861005 and hypomethylated (D) cg06669598 (E) cg06579481 (F) cg08938155 sites between responders and drug-naïve with |Δβ|≥ 5%.
Supplementary Figure S7 | QQ plot of the treatment resistance-associated differentially methylated CpG sites before and after adjusting for inflation and bias.
Supplementary Figure S8 | Boxplot showing the top three most differed hypermethylated (A) cg10861005 (B) cg26220594 (C) cg12697442 and hypomethylated (D) cg00863893 (E) cg10317314 (F) cg14687298 sites between nonresponders and drug-naïve with |Δβ|≥ 5%.
Supplementary Figure S9 | PCA plot of population grouping based on SNPs reflect south Asian ancestry: PCA-bi plot (A) principal component 1 and 2 (B) principal component 1 and 3.
Supplementary Figure S10 | In vitro assessment of antipsychotic drug treatment on treatment response markers. Expression level of treatment response markers in peripheral blood mononuclear cells following the treatment of (A) clozapine, (B) olanzapine, (C) risperidone, and (D) haloperidol. Data presented as the mean ± SEM between two independent experiments (*p ≤0.05, **p ≤0.01, ***p ≤ 0.001 ****p ≤ 0.0001).
Supplementary Figure S11 | In vitro assessment of antipsychotic drug treatment on histone acetylation and deacetylation genes. Expression level of histone acetylation and deacetylation genes in peripheral blood mononuclear cells following the treatment of (A) clozapine, (B) olanzapine, (C) risperidone, and (D) haloperidol. Data presented as the mean ± SEM between two independent experiments (*p ≤0.05, **p ≤0.01, ***p ≤ 0.001 ****p ≤ 0.0001).
Supplementary Figure S12 | In vitro assessment of antipsychotic drug treatment on histone methylase and demethylase genes. Expression level of histone methylase and demethylase genes in peripheral blood mononuclear cells following the treatment of (A) clozapine, (B) olanzapine, (C) risperidone, and (D) haloperidol. Data presented as the mean ± SEM between two independent experiments (*p ≤0.05, **p ≤0.01, ***p ≤ 0.001 ****p ≤ 0.0001).
Supplementary Figure S13 | In vitro assessment of antipsychotic drug treatment on Human Leukocyte Antigen genes. Expression level of Human Leukocyte Antigen genes in peripheral blood mononuclear cells following the treatment of (A) clozapine, (B) olanzapine, (C) risperidone, and (D) haloperidol. Data presented as the mean ± SEM between two independent experiments (*p ≤0.05, **p ≤0.01, ***p ≤ 0.001 ****p ≤ 0.0001).
References
1. Shibukumar T, Thavody J. National mental health survey of India 2015–’16: Kerala state report. IMHANS Kozhikode Kerala. (2017).
2. McCutcheon RA, Reis Marques T, Howes OD. Schizophrenia—An overview. JAMA Psychiatry. (2020) 77:201–10. doi: 10.1001/jamapsychiatry.2019.3360
3. Schoenbaum M, Sutherland JM, Chappel A, Azrin S, Goldstein AB, Rupp A, et al. Twelve-month health care use and mortality in commercially insured young people with incident psychosis in the United States. Schizophr. Bull. (2017) 43:1262–72. doi: 10.1093/schbul/sbx009
4. Demjaha A, Lappin JM, Stahl D, Patel MX, MacCabe JH, Howes OD, et al. Antipsychotic treatment resistance in first-episode psychosis: prevalence, subtypes and predictors. Psychol Med. (2017) 47:1981–9. doi: 10.1017/S0033291717000435
5. Howes OD, Thase ME, Pillinger T. Treatment resistance in psychiatry: state of the art and new directions. Mol Psychiatry. (2022) 27:58–72. doi: 10.1038/s41380-021-01200-3
6. Howes OD, McCutcheon R, Agid O, De Bartolomeis A, Van Beveren NJ, Birnbaum ML, et al. Treatment-resistant schizophrenia: treatment response and resistance in psychosis (TRRIP) working group consensus guidelines on diagnosis and terminology. Am J Psychiatry. (2017) 174:216–29. doi: 10.1176/appi.ajp.2016.16050503
7. De Berardis D, Rapini G, Olivieri L, Di Nicola D, Tomasetti C, Valchera A, et al. Safety of antipsychotics for the treatment of schizophrenia: a focus on the adverse effects of clozapine. Ther Adv Drug Saf. (2018) 9:237–56. doi: 10.1177/2042098618756261
8. Jeon SW, Kim Y-K. Unresolved issues for utilization of atypical antipsychotics in schizophrenia: Antipsychotic polypharmacy and metabolic syndrome. Int J Mol Sci. (2017) 18:2174. doi: 10.3390/ijms18102174
9. Tandon R, Lenderking WR, Weiss C, Shalhoub H, Barbosa CD, Chen J, et al. The impact on functioning of second-generation antipsychotic medication side effects for patients with schizophrenia: A worldwide, cross-sectional, web-based survey. Ann Gen Psychiatry. (2020) 19:42. doi: 10.1186/s12991-020-00292-5
10. Kumar M, Chavan B, Sidana A, Das S. Efficacy and tolerability of clozapine versus quetiapine in treatment-resistant schizophrenia. Indian J Psychol Med. (2017) 39:770–6. doi: 10.4103/IJPSYM.IJPSYM_111_17
11. Kane JM, Agid O, Baldwin ML, Howes O, Lindenmayer J-P, Marder S, et al. Clinical guidance on the identification and management of treatment-resistant schizophrenia. J Clin Psychiatry. (2019) 80:2783. doi: 10.4088/JCP.18com12123
12. Trubetskoy V, Pardiñas AF, Qi T, Panagiotaropoulou G, Awasthi S, Bigdeli TB, et al. Mapping genomic loci implicates genes and synaptic biology in schizophrenia. Nature. (2022) 604:502–8. doi: 10.1038/s41586-022-04434-5
13. Bohacek J, Mansuy IM. Molecular insights into transgenerational non-genetic inheritance of acquired behaviours. Nat Rev Genet. (2015) 16:641–52. doi: 10.1038/nrg3964
14. Keil KP, Lein PJ. DNA methylation: a mechanism linking environmental chemical exposures to risk of autism spectrum disorders? Environ Epigenet. (2016) 2:dvv012. doi: 10.1093/eep/dvv012
15. Richetto J, Meyer U. Epigenetic modifications in schizophrenia and related disorders: molecular scars of environmental exposures and source of phenotypic variability. Biol Psychiatry. (2021) 89:215–26. doi: 10.1016/j.biopsych.2020.03.008
16. Pantelis C, Papadimitriou GN, Papiol S, Parkhomenko E, Pato MT, Paunio T, et al. Biological insights from 108 schizophrenia-associated genetic loci. Nature. (2014) 511:421–7. doi: 10.1038/nature13595
17. Hilker R, Helenius D, Fagerlund B, Skytthe A, Christensen K, Werge TM, et al. Heritability of schizophrenia and schizophrenia spectrum based on the nationwide Danish twin register. Biol Psychiatry. (2018) 83:492–8. doi: 10.1016/j.biopsych.2017.08.017
18. Wockner LF, Noble EP, Lawford BR, Young RM, Morris CP, Whitehall VLJ, et al. Genome-wide DNA methylation analysis of human brain tissue from schizophrenia patients. Transl Psychiatry. (2014) 4:e339. doi: 10.1038/tp.2013.111
19. Castellani CA, Laufer BI, Melka MG, Diehl EJ, O’Reilly RL, Singh SM. DNA methylation differences in monozygotic twin pairs discordant for schizophrenia identifies psychosis related genes and networks. BMC Med Genomics. (2015) 8:1–12. doi: 10.1186/s12920-015-0093-1
20. Jaffe AE, Gao Y, Deep-Soboslay A, Tao R, Hyde TM, Weinberger DR, et al. Mapping DNA methylation across development, genotype and schizophrenia in the human frontal cortex. Nat Neurosci. (2016) 19:40–7. doi: 10.1038/nn.4181
21. Heine Staunstrup N, Starnawska A, Nyegaard M, Lade Nielsen A, Børglum A, Mors O. Saliva as a blood alternative for genome-wide DNA methylation profiling by methylated DNA immunoprecipitation (MeDIP) sequencing. Epigenomes. (2017) 1:14. doi: 10.3390/epigenomes1030014
22. Hannon E, Dempster E, Viana J, Burrage J, Smith AR, Macdonald R, et al. An integrated genetic-epigenetic analysis of schizophrenia: evidence for co-localization of genetic associations and differential DNA methylation. Genome Biol. (2016) 17:1–16. doi: 10.1186/s13059-016-1041-x
23. Kinoshita M, Numata S, Tajima A, Yamamori H, Yasuda Y, Fujimoto M, et al. Effect of clozapine on DNA methylation in peripheral leukocytes from patients with treatment-resistant schizophrenia. Int J Mol Sci. (2017) 18:632. doi: 10.3390/ijms18030632
24. Swathy B, Saradalekshmi KR, Nair IV, Nair C, Banerjee M. Understanding the influence of antipsychotic drugs on global methylation events and its relevance in treatment response. Epigenomics. (2018) 10:233–47. doi: 10.2217/epi-2017-0086
25. Hannon E, Dempster EL, Mansell G, Burrage J, Bass N, Bohlken MM, et al. DNA methylation meta-analysis reveals cellular alterations in psychosis and markers of treatment-resistant schizophrenia. Elife. (2021) 10:e58430. doi: 10.7554/eLife.58430
26. Li M, Li Y, Qin H, Tubbs JD, Li M, Qiao C, et al. Genome-wide DNA methylation analysis of peripheral blood cells derived from patients with first-episode schizophrenia in the Chinese Han population. Mol Psychiatry. (2021) 26:4475–85. doi: 10.1038/s41380-020-00968-0
27. Thomas R, Nair SB, Banerjee M. A crypto-Dravidian origin for the nontribal communities of South India based on human leukocyte antigen class I diversity. Tissue Antigens. (2006) 68:(3) 225–234. doi: 10.1111/j.1399-0039.2006.00652.x
28. Saradalekshmi KR, Neetha NV, Sathyan S, Nair IV, Nair CM, Banerjee M. DNA MethylTransferase (DNMT) gene polymorphisms could be a primary event in epigenetic susceptibility to Schizophrenia. PloS One. (2014) 9:e98182. doi: 10.1371/journal.pone.0098182
29. Vijayan NN, Mathew A, Balan S, Natarajan C, Nair CM, Allencherry PM, et al. Antipsychotic drug dosage and therapeutic response in schizophrenia is influenced by ABCB1 genotypes: a study from a south Indian perspective. Pharmacogenomics. (2012) 13:1119–27. doi: 10.2217/pgs.12.86
30. R Core Team. R: A language and Environment for Statistical Computing. Vienna, Austria: R Foundation for Statistical Computing (2023). Available at: https://www.R-project.org/.
31. Morris TJ, Butcher LM, Feber A, Teschendorff AE, Chakravarthy AR, Wojdacz TK, et al. ChAMP: 450k chip analysis methylation pipeline. Bioinformatics. (2014) 30:428–30. doi: 10.1093/bioinformatics/btt684
32. Tian Y, Morris TJ, Webster AP, Yang Z, Beck S, Feber A, et al. ChAMP: updated methylation analysis pipeline for Illumina BeadChips. Bioinforma. Oxf. Engl. (2017) 33:3982–4. doi: 10.1093/bioinformatics/btx513
33. Maksimovic J, Gordon L, Oshlack A. SWAN: Subset-quantile within array normalization for illumina infinium HumanMethylation450 BeadChips. Genome Biol. (2012) 13:1–12. doi: 10.1186/gb-2012-13-6-r44
34. Salas L, Koestler D. FlowSorted. Blood. EPIC: Illumina EPIC data on immunomagnetic sorted peripheral adult blood cells. (2023). doi: 10.18129/B9.bioc.FlowSorted.Blood.EPIC
35. Johnson WE, Li C, Rabinovic A. Adjusting batch effects in microarray expression data using empirical Bayes methods. Biostatistics. (2007) 8:118–27. doi: 10.1093/biostatistics/kxj037
36. Ritchie ME, Phipson B, Wu D, Hu Y, Law CW, Shi W, et al. limma powers differential expression analyses for RNA-sequencing and microarray studies. Nucleic Acids Res. (2015) 43:e47. doi: 10.1093/nar/gkv007
37. van Iterson M, van Zwet EW, Heijmans BT, the BIOS Consortium. Controlling bias and inflation in epigenome- and transcriptome-wide association studies using the empirical null distribution. Genome Biol. (2017) 18:19. doi: 10.1186/s13059-016-1131-9
38. Ratanatharathorn A, Boks MP, Maihofer AX, Aiello AE, Amstadter AB, Ashley-Koch AE, et al. Epigenome-wide association of PTSD from heterogeneous cohorts with a common multi-site analysis pipeline. Am J Med Genet Part B Neuropsychiatr Genet Off Publ. Int Soc Psychiatr Genet. (2017) 174, 619–30, 174. doi: 10.1002/ajmg.b.32568
39. Lawrence M. HelloRanges: Introduce *Ranges to bedtools users. (2023). doi: 10.18129/B9.bioc.HelloRanges
40. Shen L, Sinai IS. GeneOverlap: Test and Visualize Gene Overlaps. (2023). doi: 10.18129/B9.bioc.GeneOverlap
41. Edgar RD, Jones MJ, Meaney MJ, Turecki G, Kobor MS. BECon: a tool for interpreting DNA methylation findings from blood in the context of brain. Transl Psychiatry. (2017) 7:e1187–7. doi: 10.1038/tp.2017.171
42. Xiong Z, Yang F, Li M, Ma Y, Zhao W, Wang G, et al. EWAS Open Platform: Integrated data, knowledge and toolkit for epigenome-wide association study. Nucleic Acids Res. (2021) 50:D1004–9. doi: 10.1093/nar/gkab972
43. Jaffe AE, Murakami P, Lee H, Leek JT, Fallin MD, Feinberg AP, et al. Bump hunting to identify differentially methylated regions in epigenetic epidemiology studies. Int J Epidemiol. (2012) 41:200–9. doi: 10.1093/ije/dyr238
44. Peters TJ, Buckley MJ, Statham AL, et al. De novo identification of differentially methylated regions in the human genome. Epigenet Chromatin. (2015) 8:6. doi: 10.1186/1756-8935-8-6
45. Zong W, Kang H, Xiong Z, Ma Y, Jin T, Gong Z, et al. scMethBank: A database for single-cell whole genome DNA methylation maps. Nucleic Acids Res. (2021) 50:D380–6. doi: 10.1093/nar/gkab833
46. Yu G, Wang L-G, Yan G-R, He Q-Y. DOSE: An R/Bioconductor package for disease ontology semantic and enrichment analysis. Bioinforma. Oxf. Engl. (2015) 31:608–9. doi: 10.1093/bioinformatics/btu684
47. Phipson B, Maksimovic J, Oshlack A. missMethyl: An R package for analyzing data from Illumina’s HumanMethylation450 platform. Bioinforma. Oxf. Engl. (2016) 32:286–8. doi: 10.1093/bioinformatics/btv560
48. Shannon P, Markiel A, Ozier O, Baliga NS, Wang JT, Ramage D, et al. Cytoscape: a software environment for integrated models of biomolecular interaction networks. Genome Res. (2003) 13:2498–504. doi: 10.1101/gr.1239303
49. Chin C-H, Chen S-H, Wu H-H, Ho C-W, Ko M-T, Lin C-Y. cytoHubba: identifying hub objects and sub-networks from complex interactome. BMC Syst Biol. (2014) 8:1–7. doi: 10.1186/1752-0509-8-S4-S11
50. Szklarczyk D, Gable AL, Nastou KC, Lyon D, Kirsch R, Pyysalo S, et al. The STRING database in 2021: Customizable protein–protein networks, and functional characterization of user-uploaded gene/measurement sets. Nucleic Acids Res. (2020) 49:D605–12. doi: 10.1093/nar/gkaa1074
51. Bader GD, Hogue CW. An automated method for finding molecular complexes in large protein interaction networks. BMC Bioinf. (2003) 4:1–27. doi: 10.1186/1471-2105-4-2
52. Meyer H. plinkQC: Genotype Quality Control In Genetic Association Studies. (2021). Available at: https://CRAN.R-project.org/package=plinkQC.
53. Shabalin AA. Matrix eQTL: Ultra fast eQTL analysis via large matrix operations. Bioinforma. Oxf. Engl. (2012) 28:1353–8. doi: 10.1093/bioinformatics/bts163
54. Machiela MJ, Chanock SJ. LDlink: a web-based application for exploring population-specific haplotype structure and linking correlated alleles of possible functional variants. Bioinformatics. (2015) 31:3555–7. doi: 10.1093/bioinformatics/btv402
55. Giambartolomei C, Vukcevic D, SChadt EE, Franke L, Hingorani AD, Wallace C, et al. Bayesian test for colocalisation between pairs of genetic association studies using summary statistics. PloS Genet. (2014) 10:e1004383. doi: 10.1371/journal.pgen.1004383
56. Wysokiński A, Kozłowska E, Szczepocka E, Łucka A, Agier J, Brzezińska-Błaszczyk E, et al. Expression of dopamine D1–4 and serotonin 5-HT1A-3A receptors in blood mononuclear cells in schizophrenia. Front Psychiatry. (2021) 12:645081. doi: 10.3389/fpsyt.2021.645081
57. Basile VS, Masellis M, Potkin SG, Kennedy JL. Pharmacogenomics in schizophrenia: the quest for individualized therapy. Hum Mol Genet. (2002) 11:2517–30. doi: 10.1093/hmg/11.20.2517
58. Swathy B, Banerjee M. Understanding epigenetics of schizophrenia in the backdrop of its antipsychotic drug therapy. Epigenomics. (2017) 9:721–36. doi: 10.2217/epi-2016-0106
59. van Eijk KR, de Jong S, Boks MP, Langeveld T, Colas F, Veldink JH, et al. Genetic analysis of DNA methylation and gene expression levels in whole blood of healthy human subjects. BMC Genomics. (2012) 13:636. doi: 10.1186/1471-2164-13-636
60. Kinoshita M, Numata S, Tajima A, Ohi K, Hashimoto R, Shimodera S, et al. Aberrant DNA methylation of blood in schizophrenia by adjusting for estimated cellular proportions. Neuromolecular Med. (2014) 16:697–703. doi: 10.1007/s12017-014-8319-5
61. Montano C, Taub MA, Jaffe A, Briem E, Feinberg JI, Trygvadottir R, et al. Association of DNA methylation differences with schizophrenia in an epigenome-wide association study. JAMA Psychiatry. (2016) 73:506–14. doi: 10.1001/jamapsychiatry.2016.0144
62. Cheng L, Samad OA, Xu Y, Mizuguchi R, Luo P, Shirasawa S, et al. Lbx1 and Tlx3 are opposing switches in determining GABAergic versus glutamatergic transmitter phenotypes. Nat Neurosci. (2005) 8:1510–5. doi: 10.1038/nn1569
63. Ramos BG, Ohnmacht J, Lange N, Ginolhac A, Valceschini E, Rakovic A, et al. Multi-omics analysis identifies LBX1 and NHLH1 as central regulators of human midbrain dopaminergic neuron differentiation. EMBO Rep (2024) 25, 254–285. doi: 10.1101/2023.01.27.525898
64. O’Leary A, Fernàndez-Castillo N, Gan G, Yang Y, Yotova AY, Kranz TM, et al. Behavioural and functional evidence revealing the role of RBFOX1 variation in multiple psychiatric disorders and traits. Mol Psychiatry. (2022) 27:4464–73. doi: 10.1038/s41380-022-01722-4
65. Dong L, Yan H, Huang X, Hu X, Yang Y, Ma C, et al. A2BP1 gene polymorphisms association with olanzapine-induced weight gain. Pharmacol Res. (2015) 99:155–61. doi: 10.1016/j.phrs.2015.06.003
66. Derwish R, Paterno GD, Gillespie LL. Differential HDAC1 and 2 recruitment by members of the MIER family. PloS One. (2017) 12:e0169338. doi: 10.1371/journal.pone.0169338
67. Fong G, Gayen nee’Betal S, Murthy S, Favara M, Chan JS, Addya S, et al. DNA methylation profile in human cord blood mononuclear leukocytes from term neonates: effects of histological chorioamnionitis. Front Pediatr. (2020) 8:437. doi: 10.3389/fped.2020.00437
68. Gulsuner S, Walsh T, Watts AC, Lee MK, Thornton AM, Casadei S, et al. Spatial and temporal mapping of de novo mutations in schizophrenia to a fetal prefrontal cortical network. Cell. (2013) 154:518–29. doi: 10.1016/j.cell.2013.06.049
69. Yagi H, Oka Y, Komada M, Xie M-J, Noguchi K, Sato M. Filamin A interacting protein plays a role in proper positioning of callosal projection neurons in the cortex. Neurosci Lett. (2016) 612:18–24. doi: 10.1016/j.neulet.2015.11.049
70. Long JZ, Svensson KJ, Bateman LA, Lin H, Kamenecka T, Lokurkar IA, et al. The secreted enzyme PM20D1 regulates lipidated amino acid uncouplers of mitochondria. Cell. (2016) 166:424–35. doi: 10.1016/j.cell.2016.05.071
71. Sanchez-Mut JV, Glauser L, Monk D, Gräff J. Comprehensive analysis of PM20D1 QTL in Alzheimer’s disease. Clin Epigenet. (2020) 12:20. doi: 10.1186/s13148-020-0814-y
72. Ensink JBM, Keding TJ, Henneman P, Venema A, Papale LA, Alisch RS, et al. Differential DNA methylation is associated with hippocampal abnormalities in pediatric posttraumatic stress disorder. Biol Psychiatry Cogn. Neurosci Neuroimaging. (2021) 6:1063–70. doi: 10.1016/j.bpsc.2021.04.016
73. Howrigan DP, Rose SA, Samocha KE, Fromer M, Cerrato F, Chen WJ, et al. Exome sequencing in schizophrenia-affected parent-offspring trios reveals risk conferred by protein-coding de novo mutations. Nat Neurosci. (2020) 23:185–93. doi: 10.1038/s41593-019-0564-3
74. Hu M, Xia Y, Zong X, Sweeney JA, Bishop JR, Liao Y, et al. Risperidone-induced changes in DNA methylation in peripheral blood from first-episode schizophrenia patients parallel changes in neuroimaging and cognitive phenotypes. Psychiatry Res. (2022) 317:114789. doi: 10.1016/j.psychres.2022.114789
75. Cai HQ, Weickert TW, Catts VS, Balzan R, Galletly C, Liu D, et al. Altered levels of immune cell adhesion molecules are associated with memory impairment in schizophrenia and healthy controls. Brain. Behav Immun. (2020) 89:200–8. doi: 10.1016/j.bbi.2020.06.017
76. Sheikh MA, O’Connell KS, Lekva T, Szabo A, Akkouh IA, Osete JR, et al. Systemic cell adhesion molecules in severe mental illness: Potential role of intercellular CAM-1 in linking peripheral and neuroinflammation. Biol Psychiatry. (2023) 93:187–96. doi: 10.1016/j.biopsych.2022.06.029
77. Srinivas L, Vellichirammal NN, Alex AM, Nair C, Nair IV, Banerjee M. Pro-inflammatory cytokines and their epistatic interactions in genetic susceptibility to schizophrenia. J Neuroinflamm. (2016) 13:105. doi: 10.1186/s12974-016-0569-8
78. Leykin I, Mayer R, Shinitzky M. Short and long-term immunosuppressive effects of clozapine and haloperidol. Immunopharmacology. (1997) 37:75–86. doi: 10.1016/S0162-3109(97)00037-4
79. Bhardwaj U, Singh SK. Zika virus NS1 suppresses VE-cadherin via hsa-miR-29b-3p/DNMT3b/MMP-9 pathway in human brain microvascular endothelial cells. Cell Signal. (2023) 106:110659. doi: 10.1016/j.cellsig.2023.110659
80. Schaffer AE, Breuss MW, Caglayan AO, Al-Sanaa N, Al-Abdulwahed HY, Kaymakçalan H, et al. Biallelic loss of human CTNNA2, encoding αN-catenin, leads to ARP2/3 complex overactivity and disordered cortical neuronal migration. Nat Genet. (2018) 50:1093–101. doi: 10.1038/s41588-018-0166-0
81. Li J, Loebel A, Meltzer HY. Identifying the genetic risk factors for treatment response to lurasidone by genome-wide association study: A meta-analysis of samples from three independent clinical trials. Schizophr. Res. (2018) 199:203–13. doi: 10.1016/j.schres.2018.04.006
82. Dempster EL, Pidsley R, Schalkwyk LC, Owens S, Georgiades A, Kane F, et al. Disease-associated epigenetic changes in monozygotic twins discordant for schizophrenia and bipolar disorder. Hum Mol Genet. (2011) 20:4786–96. doi: 10.1093/hmg/ddr416
83. Pai S, Li P, Killinger B, Marshall L, Jia P, Liao J, et al. Differential methylation of enhancer at IGF2 is associated with abnormal dopamine synthesis in major psychosis. Nat Commun. (2019) 10:2046. doi: 10.1038/s41467-019-09786-7
84. Fromer M, Roussos P, Sieberts SK, Johnson JS, Kavanagh DH, Perumal TM, et al. Gene expression elucidates functional impact of polygenic risk for schizophrenia. Nat Neurosci. (2016) 19:1442–53. doi: 10.1038/nn.4399
85. Yang Y-J, Luo T, Zhao Y, Jiang S-Z, Xiong J-W, Zhan J-Q, et al. Altered insulin-like growth factor-2 signaling is associated with psychopathology and cognitive deficits in patients with schizophrenia. PloS One. (2020) 15:e0226688. doi: 10.1371/journal.pone.0226688
86. O’Dushlaine C, Kenny E, Heron E, Donohoe G, Gill M, Morris D, et al. Molecular pathways involved in neuronal cell adhesion and membrane scaffolding contribute to schizophrenia and bipolar disorder susceptibility. Mol Psychiatry. (2011) 16:286–92. doi: 10.1038/mp.2010.7
87. Corzo D, Yunis JJ, Salazar M, Lieberman JA, Howard A, Awdeh Z, et al. The major histocompatibility complex region marked by HSP70-1 and HSP70-2 variants is associated with clozapine-induced agranulocytosis in two different ethnic groups. Blood. (1995) 86:3835–40. doi: 10.1182/blood.V86.10.3835.bloodjournal86103835
88. Nudel R, Benros ME, Krebs MD, Allesøe RL, Lemvigh CK, Bybjerg-Grauholm J, et al. Immunity and mental illness: findings from a Danish population-based immunogenetic study of seven psychiatric and neurodevelopmental disorders. Eur J Hum Genet. (2019) 27:1445–55. doi: 10.1038/s41431-019-0402-9
89. Knelson EH, Gaviglio AL, Tewari AK, Armstrong MB, Mythreye K, Blobe GC. Type III TGF-β receptor promotes FGF2-mediated neuronal differentiation in neuroblastoma. J Clin Invest. (2013) 123:4786. doi: 10.1172/JCI69657
90. Chan RF, Shabalin AA, Montano C, Hannon E, Hultman CM, Fallin MD, et al. Independent methylome-wide association studies of schizophrenia detect consistent case-control differences. Schizophr. Bull. (2020) 46:319–27. doi: 10.1093/schbul/sbz056
91. Pan S, Zhou Y, Yan L, Xuan F, Tong J, Li Y, et al. TGF-β1 is associated with deficits in cognition and cerebral cortical thickness in first-episode schizophrenia. J Psychiatry Neurosci JPN. (2022) 47:E86–98. doi: 10.1503/jpn.210121
92. Dunn HA, Zucca S, Dao M, Orlandi C, Martemyanov KA. ELFN2 is a postsynaptic cell adhesion molecule with essential roles in controlling group III mGluRs in the brain and neuropsychiatric behavior. Mol Psychiatry. (2019) 24:1902–19. doi: 10.1038/s41380-019-0512-3
93. Catts VS, Wong J, Fillman SG, Fung SJ, Shannon Weickert C. Increased expression of astrocyte markers in schizophrenia: Association with neuroinflammation. Aust N Z. J Psychiatry. (2014) 48:722–34. doi: 10.1177/0004867414531078
94. Chang T-J, Wang W-C, Hsiung CA, He C-T, Lin M-W, Sheu WH-H, et al. Genetic variation of SORBS1 gene is associated with glucose homeostasis and age at onset of diabetes: A SAPPHIRe Cohort Study. Sci Rep. (2018) 8:10574. doi: 10.1038/s41598-018-28891-z
95. John J, Sharma A, Kukshal P, Bhatia T, Nimgaonkar VL, Deshpande SN, et al. Rare variants in tissue inhibitor of metalloproteinase 2 as a risk factor for schizophrenia: evidence from familial and cohort analysis. Schizophr. Bull. (2019) 45:256–63. doi: 10.1093/schbul/sbx196
96. Ocaña-Morgner C, Götz A, Wahren C, Jessberger R. SWAP-70 restricts spontaneous maturation of dendritic cells. J Immunol Baltim. Md 1950. (2013) 190:5545–58. doi: 10.4049/jimmunol.1203095
97. Du H, Ma J, Zhou W, Li M, Huai C, Shen L, et al. Methylome-wide association study of different responses to risperidone in schizophrenia. Front Pharmacol. (2022) 13:1078464. doi: 10.3389/fphar.2022.1078464
98. Lu AK-M, Lin J-J, Tseng H-H, Wang X-Y, Jang F-L, Chen P-S, et al. DNA methylation signature aberration as potential biomarkers in treatment-resistant schizophrenia: Constructing a methylation risk score using a machine learning method. J Psychiatr Res. (2023) 157:57–65. doi: 10.1016/j.jpsychires.2022.11.008
99. Rok-Bujko P. Molecular mechanisms of antipsychotics – their influence on intracellular signaling pathways, and epigenetic and post-transcription processes. Adv Psychiatry Neurol. (2022) 31:74–84. doi: 10.5114/ppn.2022.117963
100. Liu ZSJ, Truong TTT, Bortolasci CC, Spolding B, Panizzutti B, Swinton C, et al. Effects of psychotropic drugs on ribosomal genes and protein synthesis. Int J Mol Sci. (2022) 23:7180. doi: 10.3390/ijms23137180
101. Kim D-W, Kim K-B, Kim J-Y, Lee K-S, Seo S-B. Negative regulation of neuronal cell differentiation by INHAT subunit SET/TAF-Iβ. Biochem Biophys Res Commun. (2010) 400:419–25. doi: 10.1016/j.bbrc.2010.08.093
102. Dewulf JP, Paquay S, Marbaix E, Achouri Y, Van Schaftingen E, Bommer GT. ECHDC1 knockout mice accumulate ethyl-branched lipids and excrete abnormal intermediates of branched-chain fatty acid metabolism. J Biol Chem. (2021) 297:101083. doi: 10.1016/j.jbc.2021.101083
103. Zhang Y, You X, Li S, Long Q, Zhu Y, Teng Z, et al. Peripheral blood leukocyte RNA-seq identifies a set of genes related to abnormal psychomotor behavior characteristics in patients with schizophrenia. Med Sci Monit Int Med J Exp Clin Res. (2020) 26:e922426. doi: 10.12659/MSM.922426
104. Nolasco S, Bellido J, Gonçalves J, Zabala JC, Soares H. Tubulin cofactor A gene silencing in mammalian cells induces changes in microtubule cytoskeleton, cell cycle arrest and cell death. FEBS Lett. (2005) 579:3515–24. doi: 10.1016/j.febslet.2005.05.022
105. Hanson MG, Aiken J, Sietsema DV, Sept D, Bates EA, Niswander L, et al. Novel α-tubulin mutation disrupts neural development and tubulin proteostasis. Dev Biol. (2016) 409:406–19. doi: 10.1016/j.ydbio.2015.11.022
106. Mill J, Tang T, Kaminsky Z, Khare T, Yazdanpanah S, Bouchard L, et al. Epigenomic profiling reveals DNA-methylation changes associated with major psychosis. Am J Hum Genet. (2008) 82:696–711. doi: 10.1016/j.ajhg.2008.01.008
107. King ZT, Butler MT, Hockenberry MA, Subramanian BC, Siesser PF, Graham DM, et al. Coro1B and Coro1C regulate lamellipodia dynamics and cell motility by tuning branched actin turnover. J Cell Biol. (2022) 221:e202111126. doi: 10.1083/jcb.202111126
108. Wu C, Pan W. Integration of methylation QTL and enhancer–target gene maps with schizophrenia GWAS summary results identifies novel genes. Bioinformatics. (2019) 35:3576–83. doi: 10.1093/bioinformatics/btz161
109. Wey H-Y, Gilbert TM, Zürcher NR, She A, Bhanot A, Taillon BD, et al. Insights into neuroepigenetics through human histone deacetylase PET imaging. Sci Transl Med. (2016) 8:351ra106. doi: 10.1126/scitranslmed.aaf7551
110. Schroeder FA, Gilbert TM, Feng N, Taillon BD, Volkow ND, Innis RB, et al. Expression of HDAC2 but not HDAC1 transcript is reduced in dorsolateral prefrontal cortex of patients with schizophrenia. ACS Chem Neurosci. (2017) 8:662–8. doi: 10.1021/acschemneuro.6b00372
111. Gilbert TM, Zürcher NR, Wu CJ, Bhanot A, Hightower BG, Kim M, et al. PET neuroimaging reveals histone deacetylase dysregulation in schizophrenia. J Clin Invest. (2019) 129:364–72. doi: 10.1172/JCI123743
112. Shi J, Levinson DF, Duan J, Sanders AR, Zheng Y, Pe’er I, et al. Common variants on chromosome 6p22.1 are associated with schizophrenia. Nature. (2009) 460:753–7. doi: 10.1038/nature08192
113. Stefansson H, Ophoff RA, Steinberg S, Andreassen OA, Cichon S, Rujescu D, et al. Common variants conferring risk of schizophrenia. Nature. (2009) 460:744–7. doi: 10.1038/nature08186
114. International Schizophrenia Consortium, Purcell SM, Wray NR, Stone JL, Visscher PM, O'Donovan MC, et al. Common polygenic variation contributes to risk of schizophrenia and bipolar disorder. Nature. (2009) 460: 748–52. doi: 10.1038/nature08185
115. Li J, Yoshikawa A, Alliey-Rodriguez N, Meltzer HY. Schizophrenia risk loci from xMHC region were associated with antipsychotic response in chronic schizophrenic patients with persistent positive symptom. Transl Psychiatry. (2022) 12:1–9. doi: 10.1038/s41398-022-01854-9
116. Goldstein JI, Jarskog LF, Hilliard C, Alfirevic A, Duncan L, Fourches D, et al. Clozapine-induced agranulocytosis is associated with rare HLA-DQB1 and HLA-B alleles. Nat Commun. (2014) 5:4757. doi: 10.1038/ncomms5757
117. Girardin FR, Poncet A, Perrier A, Vernaz N, Pletscher M, F Samer C, et al. Cost-effectiveness of HLA-DQB1/HLA-B pharmacogenetic-guided treatment and blood monitoring in US patients taking clozapine. Pharmacogenomics J. (2019) 19:211–8. doi: 10.1038/s41397-017-0004-2
Keywords: schizophrenia, epigenetics, methylation, SNP genotyping, gene expression, treatment response, antipsychotics
Citation: Polakkattil BK, Vellichirammal NN, Nair IV, Nair CM and Banerjee M (2024) Methylome-wide and meQTL analysis helps to distinguish treatment response from non-response and pathogenesis markers in schizophrenia. Front. Psychiatry 15:1297760. doi: 10.3389/fpsyt.2024.1297760
Received: 21 September 2023; Accepted: 06 February 2024;
Published: 07 March 2024.
Edited by:
Massimo Tusconi, University of Cagliari, ItalyReviewed by:
Fanglin Guan, Xi’an Jiaotong University Health Science Center, ChinaJosé Jaime Martínez-Magaña, Yale University, United States
Yan Xia, Yale University, United States
Copyright © 2024 Polakkattil, Vellichirammal, Nair, Nair and Banerjee. This is an open-access article distributed under the terms of the Creative Commons Attribution License (CC BY). The use, distribution or reproduction in other forums is permitted, provided the original author(s) and the copyright owner(s) are credited and that the original publication in this journal is cited, in accordance with accepted academic practice. No use, distribution or reproduction is permitted which does not comply with these terms.
*Correspondence: Moinak Banerjee, mbanerjee@rgcb.res.in; moinak@gmail.com