- 1Institute for Mental Health Policy Research, Centre for Addiction and Mental Health, Toronto, ON, Canada
- 2Campbell Family Mental Health Research Institute, Centre for Addiction and Mental Health, Toronto, ON, Canada
- 3Department of Epidemiology and Biostatistics, Schulich School of Medicine & Dentistry, Western University, London, ON, Canada
- 4Dalla Lana School of Public Health, University of Toronto, Toronto, ON, Canada
- 5Department of Psychiatry, University of Toronto, Toronto, ON, Canada
Background: Machine learning is a promising tool in the area of suicide prevention due to its ability to combine the effects of multiple risk factors and complex interactions. The power of machine learning has led to an influx of studies on suicide prediction, as well as a few recent reviews. Our study distinguished between data sources and reported the most important predictors of suicide outcomes identified in the literature.
Objective: Our study aimed to identify studies that applied machine learning techniques to administrative and survey data, summarize performance metrics reported in those studies, and enumerate the important risk factors of suicidal thoughts and behaviors identified.
Methods: A systematic literature search of PubMed, Medline, Embase, PsycINFO, Web of Science, Cumulative Index to Nursing and Allied Health Literature (CINAHL), and Allied and Complementary Medicine Database (AMED) to identify all studies that have used machine learning to predict suicidal thoughts and behaviors using administrative and survey data was performed. The search was conducted for articles published between January 1, 2019 and May 11, 2022. In addition, all articles identified in three recently published systematic reviews (the last of which included studies up until January 1, 2019) were retained if they met our inclusion criteria. The predictive power of machine learning methods in predicting suicidal thoughts and behaviors was explored using box plots to summarize the distribution of the area under the receiver operating characteristic curve (AUC) values by machine learning method and suicide outcome (i.e., suicidal thoughts, suicide attempt, and death by suicide). Mean AUCs with 95% confidence intervals (CIs) were computed for each suicide outcome by study design, data source, total sample size, sample size of cases, and machine learning methods employed. The most important risk factors were listed.
Results: The search strategy identified 2,200 unique records, of which 104 articles met the inclusion criteria. Machine learning algorithms achieved good prediction of suicidal thoughts and behaviors (i.e., an AUC between 0.80 and 0.89); however, their predictive power appears to differ across suicide outcomes. The boosting algorithms achieved good prediction of suicidal thoughts, death by suicide, and all suicide outcomes combined, while neural network algorithms achieved good prediction of suicide attempts. The risk factors for suicidal thoughts and behaviors differed depending on the data source and the population under study.
Conclusion: The predictive utility of machine learning for suicidal thoughts and behaviors largely depends on the approach used. The findings of the current review should prove helpful in preparing future machine learning models using administrative and survey data.
Systematic review registration: https://www.crd.york.ac.uk/prospero/display_record.php?ID=CRD42022333454 identifier CRD42022333454.
Introduction
Suicide is a significant public health problem. In 2019, approximately 703,000 people died by suicide worldwide, for a global age-standardized suicide mortality rate of 9.0 per 100,000 population (1). Given this burden, international goals to reduce the suicide mortality rate have been set. Prevention is critical to achieving such goals (2, 3). In 2021, the World Health Organization released the LIVE LIFE: an implementation guide for suicide prevention in countries, describing four effective evidence-based interventions to prevent suicide (4). These include 1) limiting access to the means of suicide, 2) interacting with the media for responsible reporting of suicide, 3) fostering socio-emotional life skills in adolescents, and 4) early identification, assessment, management, and follow-up of anyone at-risk of suicidal behavior. With respect to the latter, identifying those at-risk of suicidal behaviors (i.e., a target population) is critical. While it has been suggested that history of suicidal ideation and past suicide attempts could help predict future suicidal behavior (5), it is evident that many more factors are involved, which makes traditional prediction approaches inefficient (6).
While conventional prediction approaches apply statistical models with a limited number of predictors, mediators, and interactions, in the last decade, machine learning has become a valuable tool with a lot of promise in the area of suicide prevention due to its ability to combine the effects of multiple risk factors and complex interactions (7). In addition, conventional prediction techniques depend on the researcher’s definition of the relationship between predictors and outcomes. However, machine learning approaches can examine all potential relationships repetitively and detect the most accurate prediction algorithm (7).
Given the power of machine learning for prediction purposes, many studies have applied this technique to identify risk factors that are predictive of suicidal thoughts and behaviors in recent years. Thus, to extract applicable findings, existing systematic reviews have investigated the extent to which these machine learning techniques have been used and assessed the predictive validity of published models (8–10). However, the existing systematic reviews did not differentiate between data sources and have largely failed to summarize the most important risk factors listed in the studies.
Considering the accessibility of administrative and survey data, the current study aimed to identify all existing studies that applied machine learning techniques to such data to predict suicidal thoughts and behaviors. The objective was two-fold, to: i) summarize performance metrics reported in the studies by study design, data source, sample size and type of machine learning methods; and ii) enumerate predictors identified in the studies as important contributors to the model performance at predicting suicidal thoughts and behaviors by study design, data source, and suicide outcomes.
Methods
The current systematic review is reported according to the Preferred Reporting Items for Systematic Reviews and Meta-Analyses (PRISMA) 2020 statement (11). The protocol was registered with the International Prospective Register of Systematic Reviews (PROSPERO, registration number CRD42022333454).
Outcome definitions
The following outcomes were of interest: suicidal ideation, suicide plan, suicide attempts, and death by suicide; the definitions of which are in line with those of the Center for Disease Control and Prevention (12). The term suicidal thoughts is used herein to capture suicidal ideation and suicide plans. Suicidal ideation was defined as thoughts of engaging in suicide-related behavior; and suicide plan was defined as a thought regarding a self-initiated action that facilitates self-harm behavior or a suicide attempt; this will often include an organized manner of engaging in suicidal behavior, such as a description of a time frame and method. Suicide attempt was defined as a non-fatal self-directed potentially injurious behavior with any intent to die as a result of the behavior, and death by suicide was defined as death caused by self-directed injurious behavior with any intent to die as a result of the behavior.
Search strategy and selection criteria
A systematic literature search of PubMed, Medline, Embase, PsycINFO, Web of Science, Cumulative Index to Nursing and Allied Health Literature (CINAHL), and Allied and Complementary Medicine Database (AMED) was performed to identify all studies that have used machine learning to predict suicidal thoughts and behaviors with administrative or survey data (keywords are presented in Appendix 1). The searches were not limited geographically or by language of publication. Considering that three recent systematic reviews (8–10) have been published in this field, we conducted our search for articles published between January 1, 2019 and May 11, 2022. We retained all articles identified in the respective systematic reviews, if they met our inclusion criteria. The citations in all included articles were also manually screened. To ascertain our findings, we conducted an independent search (i.e., ignoring the results of the three systematic reviews) using the same keywords and including published studies through May 11, 2022.
Inclusion and exclusion criteria
Articles were included if they i) consisted of original, quantitative research published in a peer-reviewed journal or scholarly report; ii) used a machine learning method to predict a suicide outcome (suicidal ideation, suicide plan, suicide attempt, and/or death by suicide); and iii) used either an administrative or survey dataset containing individual-level data.
Articles were excluded if they performed a machine learning technique (e.g., natural language processing) to scan social media platforms to detect suicidal thoughts and behaviors. There was no restriction on population or study setting.
Study selection and data extraction
Two investigators performed title and abstract screening and full-text reviews independently. Conflicts in study identification were resolved in conjunction with the third investigator. All screening was completed using EndNote 20, and data extraction was completed by one investigator using a template created in Microsoft Excel and cross-checked by the other two investigators. The following variables were extracted: country, study design, study duration, data source, study population, sample size, number of cases and controls, suicidal outcome, validation technique, machine learning methods, relevant risk factors (or most important predictive risk factors), and performance statistics, including accuracy, sensitivity, specificity, positive predictive value, negative predictive value, and area under the receiver operating characteristic curve (AUC).
Risk of bias
Two investigators assessed the risk of bias using the Prediction model study Risk of Bias Assessment Tool (PROBAST) (13). In the presence of conflicts in evaluating the risk of bias in a study, the third investigator was consulted, and the discrepancies were discussed until a unanimous decision was taken. PROBAST contains twenty signaling questions in four domains: participants, predictors, outcome, and analysis. It also investigates the applicability of studies in participants, predictors, and outcome domains. Based on PROBAST, included studies were classified into one of three categories: low, high, and unclear risk of bias and applicability.
Statistical analysis
Our statistical analysis aims to summarize performance metrics reported in the included studies. Meta-analysis is beyond the scope here; more details can be found in (14, 15). Our descriptive analysis includes two different steps. First, we used a box plot of the most frequently reported performance statistics (i.e., the AUC) to explore the predictive power of machine learning methods in predicting suicidal thoughts and behaviors. Box plots were used to summarize the distribution of the AUC values by suicide outcome and machine learning method. Additionally, box plots helped identify AUC values that differed significantly from the rest of the AUCs (i.e., outliers). The presence of these outliers may be due to the fact that we did not consider only the AUCs for the best model – all AUCs in the selected studies were reported. Note that an AUC of 0.5 indicates that the machine learning method performance is no better than chance (i.e., random guesses). AUCs above 0.9 indicate excellent prediction, between 0.80 and 0.89 good prediction, 0.70 to 0.79 fair prediction, 0.60 to 0.69 poor prediction and 0.50 to 0.59 extremely poor prediction (16). AUC interpretation can vary widely depending on studies objectives and the trade-off between false positives and false negatives. For example, suppose the objective was to minimize the false negatives in suicidal thoughts and behaviors. In that case, one might lower the above thresholds so that more positive events are classified as positive. However, accounting for the specific context of each included study is above the scope of this review as the information was not reported in the included articles. We then considered the thresholds abovementioned to assess the predictive power of each model and compare the models. Second, we computed mean AUCs for all the models and the mean AUCs for the best-performing models (i.e., with the higher AUCs) in each article with 95% confidence intervals (CIs) by suicide outcomes (i.e., suicidal thoughts, suicide attempt, and death by suicide, as well as all suicide outcomes combined) across different study subgroups and machine learning methods. We regrouped the studies by study design (cross-sectional and longitudinal), study data source (administrative, survey, or both), total sample size (≤1,000, 1,000 to 10,000 and >10,000), and sample size of cases (≤200, 200 to 1,000, and >1,000). We also regrouped the AUCs by machine learning methods employed, namely Bayesian algorithms (naïve Bayes, Bayesian network, or Bayesian additive regression trees), boosting algorithms [gradient boosting tree, adaptive boosting (AdaBoost), or extreme gradient boosting (XGBoost)], cox regression, decision tree, K-nearest neighbors, linear discriminant analysis, logistic regression, neural network, random forest, regularized regressions (elastic net, least absolute shrinkage and selection operator (LASSO) or ridge regression), super learner (i.e., a combination of machine learning algorithms), and support vector machine.
Results
Study selection
The search strategy identified a total of 2,200 unique records, of which 168 full-text articles were retrieved (Figure 1). After full-text assessment, 64 were excluded (the reasons for their exclusion are presented in Appendix 2), and 104 articles were included.
Study characteristics
Over 85% (n=90) of eligible studies were published since 2017. The majority of studies were from the United States (n=43, 41.4%), followed by South Korea (n=17, 16.4%) and Canada (n=5, 4.81%). Twenty-six studies used machine learning to predict suicidal thoughts, 55 studies aimed to predict suicide attempts, and 19 studies predicted death by suicide (it should be noted that some studies predicted more than one suicide outcome of interest). Survey data (57.69%) was used more often than administrative data (n=41, 39.4% and n=60, 57.7%, respectively), and three (2.9%) studies used both types of data. Over 60% (n=63) used a longitudinal study design, while the remainder used a cross-sectional study design (one study did not provide sufficient information to determine the study design). Existing studies applied various machine learning methods, most of which were supervised learning techniques, including logistic regression (n=38, 17.9%), random forest (n=37, 17.4%) and decision tree (n=30, 14.1%). K-fold cross-validation techniques (with K=5 or 10) were the most used model’s performance evaluation methods (n=67, 64%), followed by the hold-out method (n=12, 12%) and bootstrap-based optimism correction methods (n=6, 6%). 19 (18%) studies did not provide the algorithm validation method. Cross-validation is usually the preferred method because it allows the model to train on multiple train-test splits, better indicating the model performance on unseen data. Please see Supplementary Table 1 for more details on the characteristics of each study included in the Supplemental Material.
Performance of applied machine learning methods
Different metrics were used to evaluate model performance; of 104 included studies, a total of 84 studies reported the AUC (Mean = 0.807, 95% CI: 0.787-0.826, SD = 0.088, Median = 0.818, Range = 0.588-0.987). Additionally, 49 studies reported Accuracy (Mean = 0.822, 95% CI: 0.782-0.863, SD = 0.168, Median = 0.838, Range = 0.250-0.996). Sixty-four studies reported sensitivity or prediction of the positive class (Mean = 0.682, 95% CI: 0.628-0.735, SD = 0.215, Median = 0.742, Range = 0.128-1), and 59 studies described specificity or prediction of the negative class (Mean = 0.809, 95% CI: 0.765-0.853, SD = 0.168, Median = 0.820, Range = 0.25-1), separately. Positive predictive value (PPV), or the percentage of positively categorized cases that were actually positive was assessed in 46 articles (Mean = 0.412, 95% CI: 0.315-0.508, SD = 0.325, Median = 0.385, Range = 0.02-1), and 36 studies reported negative predictive value (NPV), the proportion of negatively categorized cases that were actually negative (Mean = 0.911, 95% CI: 0.875-0.947, SD = 0.107, Median = 0.963, Range = 0.600-0.999).
These results suggest that, on average, machine learning algorithms achieved good prediction of suicidal thoughts and behaviors. However, machine learning methods’ predictive power appears to differ across suicide outcomes. Figures 2, 3 present the distribution of the AUCs by machine learning methods for suicidal thoughts, suicide attempts, and death by suicide, as well as all suicide outcomes combined in a box plot. Although the figure displays a few outliers, the AUCs for boosting algorithms were closer to 0.9 than the other algorithm’s AUCs when predicting suicidal thoughts, death by suicide, and all suicide outcomes combined (Figure 3). Neural network algorithm AUCs were concentrated around the highest AUC value in predicting suicide attempts (with nearly all the AUC values between 0.8 and 0.9, indicating good prediction). This algorithm was the second best at predicting death by suicide and all suicide outcomes combined. Random forest and support vector machine were the second-best algorithms at predicting suicide attempts and suicidal thoughts, respectively, with the majority of the AUCs between 0.8 and 0.9. It should be noted that the AUCs of the support vector machine were around 0.7 (the smallest) for death by suicide and suicide attempt prediction. The AUCs of the K-nearest neighbors algorithm were between 0.6 and 0.79 for suicidal thoughts, suicide attempts, and all suicide outcomes combined.
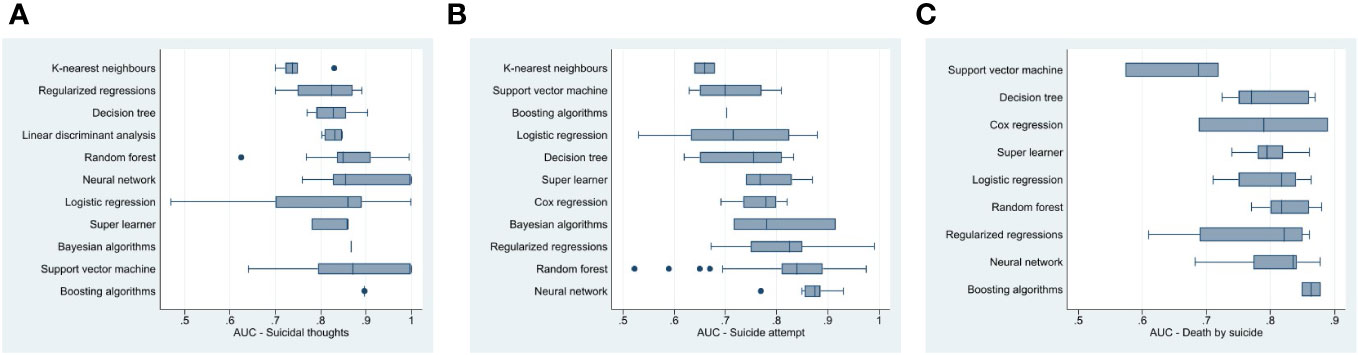
Figure 2 Box plot of machine learning method area under the receiver operating characteristic curve for (A) suicidal thoughts, (B) suicide attempt and (C) death by suicide.
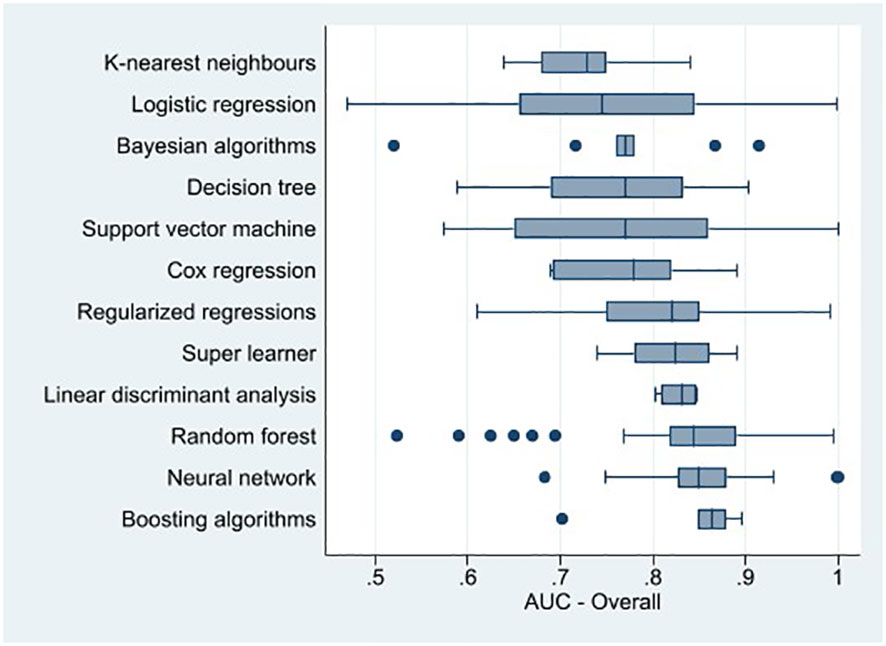
Figure 3 Box plot of machine learning method area under the receiver operating characteristic curve for suicidal thoughts and behaviors combined.
In addition, logistic regression displayed relatively lower AUCs and more variability than the other machine learning methods overall, except for death by suicide. However, boosting algorithms and neural network performed better than the logistic regression at predicting death by suicide (Figure 2). The mean AUCs in Table 1 indicate that overall, logistic regression, neural network, boosting algorithms, neural network, K-nearest neighbors, regularized regressions, and random forest had an average AUC of between 0.80 and 0.89 (i.e., good prediction of suicidal thoughts and behaviors). Table 1 also shows heterogeneity in the performance of machine learning methods across suicide outcomes.
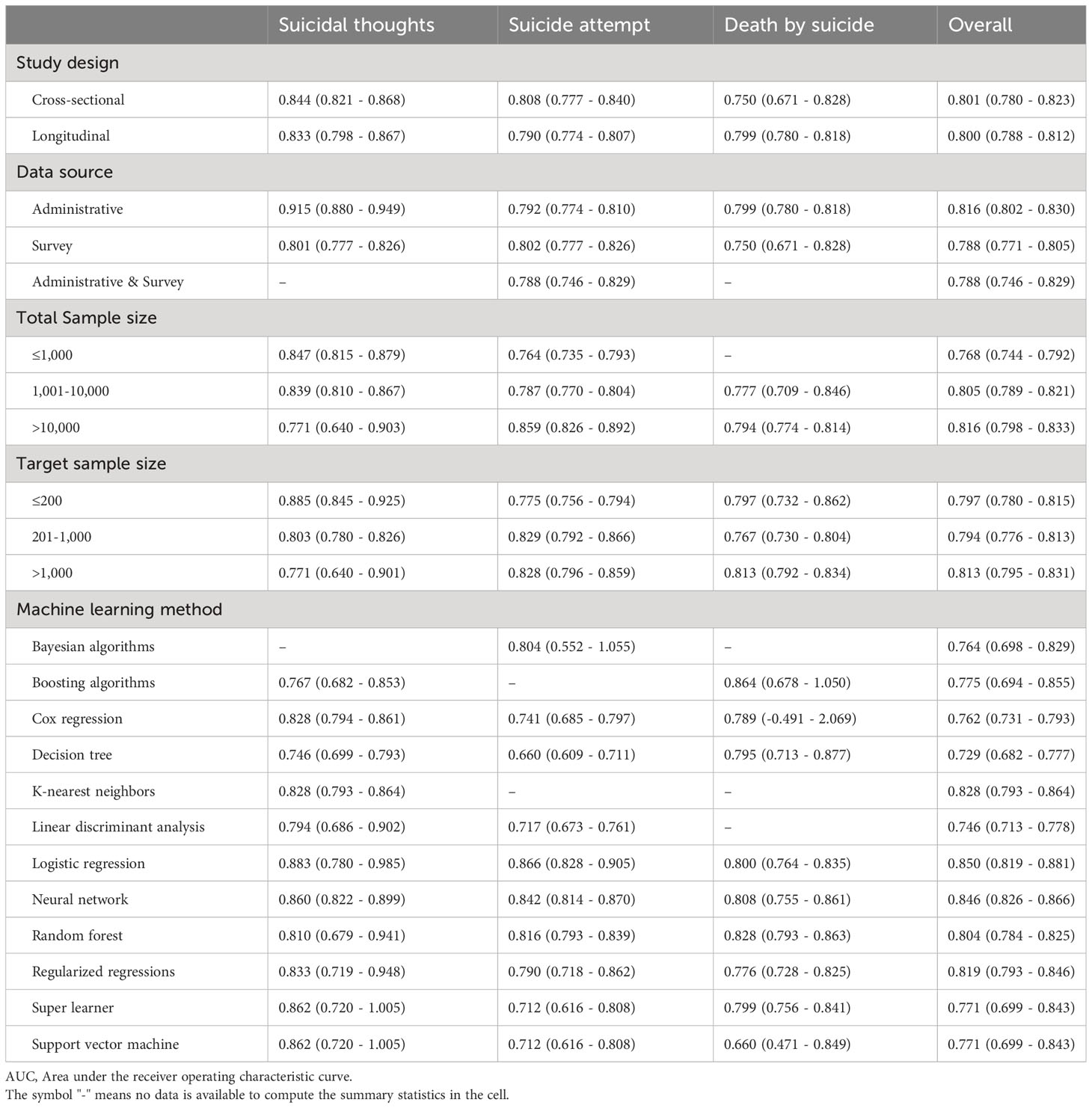
Table 1 The mean AUC of all the models (95% confidence interval) for predicting suicide outcomes by study design, data source, sample size, and type of machine learning methods.
The average performance of machine learning methods for predicting suicidal thoughts and behaviors by study design, data source, and sample size
The performance of machine learning methods at predicting suicidal thoughts and behaviors, on average, was similar across the type of study design and suicide outcomes, with mean AUCs ranging from 0.75 to 0.84 (Table 1). However, machine learning methods achieved the highest average AUC (0.915, 95% CI: 0.880-0.949) at predicting suicidal thoughts with administrative data (vs 0.801, 95% CI: 0.777-0.826 with survey data). The average performance of machine learning methods was similar in predicting death by suicide and suicide attempt across the two data sources, with mean AUCs between 0.75 and 0.80. Overall, the prediction of suicidal thoughts and suicide behaviors displayed higher AUCs on average as the sample size increased (Table 1). Similar results were found with the target sample size (i.e., the sample of individuals with suicidal thoughts or suicide behaviors).
The best-performing machine learning methods average performance in predicting suicidal thoughts and behaviors by study design, data source, and sample size
Overall, the average best-performing methods for higher AUC were support vector machine, random forest, boosting algorithms, neural network, regularized regressions and super learner with AUCs greater than 0.8 (Table 2). Logistic regression had the lowest AUC (0.789, 95% CI: 0.737-0.841). However, logistic regression performed better than the support vector machine in the prediction of suicide attempts. Regarding suicidal thoughts, the best-performing method remained the support vector machine (AUC=0.930, 95% CI: 0.040-1.819) on average. Table 2 also indicated that the performance of the best-performing models was, on average, similar across the study design, the data source, and the sample size for all the suicide outcomes. However, we found no evidence of an upward trend of AUCs over time, suggesting that our study did not observe the positive influence of technological and model improvements over time on the machine learning model’s predictive power.
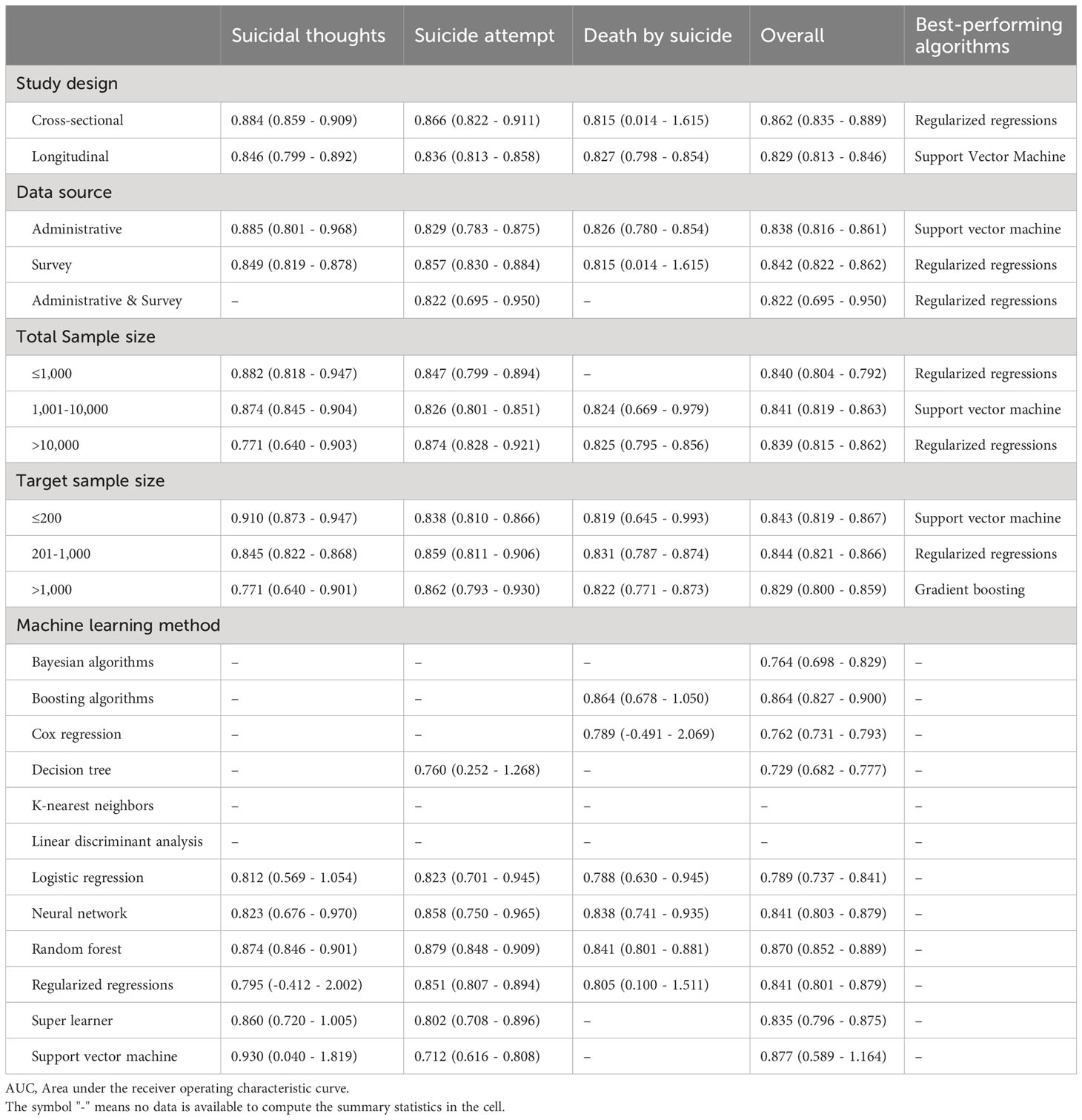
Table 2 The mean AUC of the best-performing models (95% confidence interval) for predicting suicide outcomes by study design, data source, sample size, and type of machine learning methods.
The most important predictive risk factors of suicidal thoughts and behaviors reported in the included studies
The best-performing algorithm was used in each study to identify important predictive risk factors for suicide outcomes. The methods used depend on the algorithms. For support vector machine, decision tree, boosting algorithms and random forest to evaluate the importance of each predictor, the criteria used was the mean decrease in accuracy values, which represents the reduction in accuracy if a predictor were randomly permuted (also known as permutation feature importance methods). The most important predictors had a more considerable mean decrease in accuracy. For regularized regressions, the magnitude of the estimated weights associated with a predictor indicated how influential the predictor is in predicting the outcome. The neural networks also used weight-based methods – predictors with higher weights contributed more to the final predictions of the model.
Administrative data and survey studies
Administrative. Among studies that aimed to predict suicidal thoughts using administrative data, Peis et al. (17) and McKernan et al. (18) showed that mental illness, related inpatient utilization, and previous suicidal thoughts and attempt(s) are common risk factors in addition to some social factors like shared residence and living with offspring or siblings. Some somatic factors like low levels of free thyroxine, free triiodothyronine, temporary disability, feeling heart race/pound, and polysomatic symptoms (fatigue, dizziness, weakness) were also reported as risk factors for suicidal thoughts (17–20). Studies that used administrative data to predict suicide attempts identified the most important risk factors to be age (21–24), history of suicidal thoughts and behaviors (23–26), mental health conditions like anxiety, depression, helplessness, hopelessness and substance use (18, 21, 24–28), and having an emergency room visit or inpatient admission (18, 22, 26, 28). When the aim was to predict death by suicide using administrative data, the most important risk factors were record/indication of mental or behavioral disorders such as schizophrenia, antipsychotic medication use, depression, anxiety, stress disorders, and alcohol use (26, 29–33), followed by a prior suicide attempt or self-harm (26, 29, 30, 34), and age (30–33, 35). Four studies predicted suicidal thoughts and behaviors combined, and based on their findings, schizophrenia, personality disorders (borderline), depressive disorder, substance use disorder, family history of these disorders, related medications (such as antipsychotics and antidepressants), and intentional self-harm were the most important risk factors (36, 37) (see Supplementary Table 2).
Survey. In studies utilizing survey data, depression (sadness, hopelessness) (38–44), anger attacks (44), anxiety (41), perceived burdensomeness (38, 45), post-traumatic stress disorder (43), self-esteem (39, 42), perceived stress level (41, 46), history of suicidal ideation, attempt or familial history of suicide (38, 40, 44), and hours of sleep (40, 42) were the most important predictors identified for suicidal thoughts. In another study, Burke et al. (47) found non-suicidal self-injury (NSSI), depression, desire to cease NSSI, NSSI likelihood, and number of NSSI scars, and revenge function of NSSI as factors predictive of suicide plans when using survey data. Kuroki et al. (48) found anxiety disorder, depressive disorder, family cohesion, and family conflict to be important risk factors for suicidal ideation. Some studies predicted suicidal thoughts and suicide attempts together. However, the identified risk factors were similar to those mentioned (49–51) (Supplementary Table 2). Studies predicting suicide attempts found that current or past suicide plans (47, 52–55), suicidal ideation (54–58), suicide attempt(s) (59), non-suicidal self-injury (47, 55), and positive familial or friend history of suicide (57, 58, 60–62) were the established risk factors. In addition, history of mental or personality disorders such as depression (sadness/hopelessness) (45, 54, 60, 63–67), anxiety (68), was bullied or violated (57, 66, 67), borderline personality disorder (56, 69, 70), drug abuse or dependence (62, 63, 67, 71), affective dyscontrol (72), impulsivity (56, 60), post-traumatic stress disorder (69, 73), number of hospitalizations (70, 71, 73), demographic characteristics (such as age (64–66), and being female (57, 63, 68)), alcohol drinking (54, 57, 61, 64, 66), smoking (57, 63, 64, 66) were the other important risk factors for predicting suicide attempt. Regarding death by suicide, Choi et al. (74) found anxiety, depression, resilience, and self-esteem as predictive factors when using survey data.
Study populations
Adolescents. Eleven studies assessed predictive risk factors of suicidal thoughts and behaviors in children or adolescents (38, 40, 49, 50, 58, 63, 66, 67, 75, 76). Czyz et al. (38) and Hill et al. (40) reported depressive symptoms and a history of suicidal ideation or suicide attempts as risk factors for suicidal thoughts among adolescents. Further, the existing studies identified sadness or hopelessness (63, 66, 67), stress level (66, 75), number of lifetime mental disorders (59), violence or fighting (63, 66, 67), substance abuse (63, 66), cigarette smoking (63, 66), alcohol drinking (66), prior suicide attempt(s) (59), suicidal ideation (58), familial life (58, 66, 75), and demographic factors such as sex (63, 66), age (66), and belonging to a minority group (58, 63) as risk factors for suicide attempt. Three studies (49, 50, 76) investigated predictive risk factors of suicidal thoughts and suicide attempts together, and their findings were similar to the aforementioned factors (Supplementary Table 2).
Soldiers. Seven studies aimed to predict suicidal thoughts and behaviors among soldiers (19, 43, 44, 53, 68, 77, 78), all of which were conducted in Canada and the United States. Depression (43), post-traumatic stress disorder (43), sexual harassment in females (43), mental disorders (43, 44), taking any medication for mental disorders (19), somatic complaints (43) [including upset stomach during last attack (19) or feeling heart race/pound (19)], past suicidal ideation (44), and violence during deployment (44) were found to be important risk factors for suicidal thoughts (Supplementary Table 2). History and number of mental disorders (68, 77), anxiety disorders (68), self-reported lifetime suicide plan (68), military service factors (77, 78), and demographic characteristics (i.e., sex (68), age (77, 78), and racial/ethnic minority status (77, 78)) as important risk factors for suicide attempt.
Elderly. In those studies conducted among an elderly population, quality of life (39, 46), restriction of activity (46), income level (46), stress level (46), depression (39), self-esteem (39), satisfaction with family relationships (39), and health status (39) were found to be risk factors for suicidal thoughts (Supplementary Table 2). Further, Cho et al. (33) found that history of taking benzodiazepines, body mass index, age, and history of taking sleeping pills were significant risk factors for death by suicide in an elderly population.
Persons with mental health disorders. Researchers found that in patients with obsessive-compulsive disorder, previous suicide plans or thoughts, lifetime depressive episodes, and intermittent explosive disorder were risk factors for suicide attempts (25). Among individuals with substance use disorders, males with a brief psychotic disorder diagnosis or antipsychotic prescription or females older than 30 with a ‘poisoning diagnosis’ had a higher risk of death by suicide (31). Antipsychotic and antidepressant medications, a diagnosis of autistic disorder, schizophrenic disorder, and substance use disorder were reported by Fan et al. (37) as risk factors for suicidal thoughts, suicide attempts, or death by suicide among patients with post-traumatic stress disorder and bipolar disorder. Hettige et al. (71) reported the number of hospitalizations, duration of illness, childhood trauma (such as physical and emotional abuse), and substance abuse or dependence as risk factors for suicide attempts among individuals with schizophrenia. In patients with lifetime major depressive episodes, previous suicide attempts (69), borderline personality disorder (69), and hospitalization due to depressive symptoms (69) were identified as risk factors for suicide attempts. Low free triiodothyronine, low free thyroxine, severity of depressive symptoms, and work status were found to be important risk factors for suicidal thoughts (20). Age (21, 23, 79), history of mental disorder (22) (such as anxiety (21)), having a suicide plan, intent or positive familial suicidal history (23, 52, 61), number of outpatient, inpatient, and emergency room visits (22), and educational level (21, 79) were identified as predictive risk factors for suicidal thoughts or suicide attempt among individuals with a history of suicide attempt. Based on the studies that aimed to predict suicidal thoughts and behaviors among individuals with a mood disorder, depression (hopelessness and helplessness) (27, 56, 72, 73, 80), borderline personality disorder (56, 80), prior suicidal ideation, attempt or history of suicide in their family (26, 56, 80), substance abuse or dependence (26, 62, 73), aggression (56), affective dyscontrol (72), loss of cognitive control (72), history of psychosis (73), post-traumatic stress disorder comorbidity (73), having an emergency department visit or inpatient hospitalization with a high-lethality diagnosis (28), and a history of physical illnesses (81) were identified as risk factors for suicide attempts. In addition, hospitalized for schizophrenia-spectrum and bipolar disorders (34), previous self-harm (34, 80) or suicidal attempt or thoughts (26), prior hospitalization or emergency mental health care (26, 80) and substance abuse (26) were mentioned as risk factors for death by suicide in this group (Supplementary Table 2).
General population. Fourteen studies sought to predict the most important risk factors of suicidal thoughts and behaviors among the general population. Kuroki et al. (48), Ryu et al. (41), and Peis et al. (17) found that depression (41, 48), anxiety (41, 48), stress (41), previous suicidal thoughts or suicide attempts (17), and shared residence or living with siblings or offspring (17) were risk factors for suicidal thoughts (Supplementary Table 2). Depression (45, 60, 64, 65), impulsivity (60), borderline personality disorder (69), post-traumatic stress disorder (69), alcohol use disorders identification test (AUDIT) score (64) or frequency of drinking (64), previous history of suicide attempt (82) or suicide among family members (60) or friends (82), lower family support or higher familial conflict (48), substance use in the previous two weeks (82), and demographic characteristics including age (64, 65, 83), lower educational level (65, 82, 83), and being female (82, 83) were important when predicting suicide attempt.
Also, age (32, 35), sex (30, 35), depression (29, 30, 74), anxiety (29, 74), self-esteem (74), alcohol consumption (32), prior self-harm (29) or suicide attempt (30), stress disorders (30), and schizophrenia in females or antipsychotics in males were reported as risk factors for death by suicide among the general population.
Risk of bias
Based on PROBAST, two articles had a high risk of bias, twenty-seven had an unclear risk of bias (mostly due to unclear information in the predictors, outcome, and analysis domains), and the others were classified as having a low risk of bias. Regarding applicability, all included articles were categorized as being of low concern. The results of these evaluations are summarized in Appendix 3.
Discussion
The current systematic review summarized studies that applied machine learning methods to administrative and/or survey data to predict suicidal thoughts and behaviors. This review included 104 articles, all published within the last 25 years. Although the predictive power of models differed across suicide outcomes (i.e., suicidal thoughts, suicide attempt, and death by suicide), overall, machine learning algorithms achieved good prediction of suicidal thoughts and behaviors when using administrative and/or survey data. Many machine learning algorithms performed better than logistic regression in predicting suicide outcomes, including boosting algorithms and neural networks. Additionally, studies with greater total and target sample sizes reported higher prediction accuracies. We also found that the risk factors for suicidal thoughts and behaviors appear to differ depending on the data source and the population under study.
Considering that many individuals who have attempted suicide or died by suicide visited their physician or emergency room prior to (22, 61, 84), the application of machine learning techniques using administrative data is a promising tool, as it could help improve early detection of individuals who are at high risk for suicidal behaviors. There is also the potential for such techniques to relieve an already over burden healthcare system by providing clinicians with a tool for suicide risk identification. In fact, it has been shown that risk stratification via electronic medical records data predicts suicide risk better than clinical evaluation (28). Thus, a combination of machine learning and routine triage could optimize clinical decision-making and conserve human and financial resources. Further, the application of machine learning techniques on survey data from a variety of populations could be helpful in detecting and preventing potential high-risk subpopulations.
To the best of our knowledge, this is the largest (104 included studies) systematic literature review on the application of machine learning techniques to predict suicidal thoughts and behaviors. Further, this is the first review study to summarize the predictive power of machine learning in predicting suicidal thoughts and behaviors by type of method, study design, data source, and sample size and to summarize extensively the most important risk factors identified. It should be noted that even though most studies had a large sample distribution of participants, around 30% of studies had a sample size of less than 1000, which could escalate the risk of overfitting and affect the interpretation of their results (investigating a large number of risk factors in a small sample of participants). Further, the majority of studies were from the United States (>40%); thus, studies need to be conducted elsewhere. For instance, very few studies (n=5) (19, 71, 85–87) used data from Canada to predict suicidal thoughts and behaviors using machine learning. Only three of these studies used population-level data from the province of Alberta. To fill this gap and advance the application of machine learning in detecting suicide risk, more studies are needed, focusing on larger and more comprehensive population-based datasets that can facilitate complex modeling incorporating relevant risk factors.
Furthermore, very few studies (n=5) (30, 31, 36, 43, 88) stratified their analyses by sex. This represents a significant gap in the literature, as the gender paradox in suicide (i.e., women tend to attempt suicide more often, while men tend to die by suicide more often) is well-known. The gender paradox in suicide is a culture-bound phenomenon, meaning that cultural expectations about gender and suicide strongly determine both its existence and magnitude (89, 90). As such, the risk factors predictive of suicidal thoughts and behaviors will likely differ for men and women. Gender-specific analyses have the potential to identify the risk factors that may be predictive of suicidal thoughts and behaviors in one gender but not the other and thus can further inform targeted suicide prevention strategies.
Overall, the findings of the current review should prove helpful in preparing future machine learning models using survey/administrative data to predict suicide outcomes, their application in clinical decision-making, and planning prevention interventions. As indicated by the sheer number of studies available, the application of machine learning for predicting suicidal thoughts and behaviors represents an area of research which has seen significant growth. This growth has significant implications for the prevention of suicide and, thus, the reduction of the suicide mortality rate globally.
However, in many studies, the complete list of predictors used in the machine learning algorithms was not available. To advance this field, we suggest that future studies using machine learning to predict suicide outcomes enumerate all the predictors in their models (in the supplement) and the list of the most important predictors identified by the best-performing model. This will help other researchers to carefully select their predictors and investigate other variables that might improve models’ prediction. It will also help decision-makers or program planners to translate findings into more practical tools to enhance suicide prevention. Additionally, to improve the model’s performance, future studies must account for the data imbalance issues caused by the rarity of suicide outcomes using more sophisticated sampling methods such as the Synthetic Minority Over-sampling Technique (91) or ensemble learning techniques (92). Our study has revealed that most included studies using longitudinal data have failed to account for the correlation of individual observations over time. Thus, the assumption that training data is independent and identically distributed may be violated, making supervised machine learning algorithms inefficient. We recommend that future studies aiming to predict suicide outcomes with longitudinal data consider using mixed-effect machine learning algorithms. These algorithms are robust to correlated data and predict change of a longitudinal outcome with high accuracy (93). Finally, future studies need to rely on novel causal inference methods based on machine learning to help understand causal relationships between important predictors and suicide outcomes (94, 95).
Data availability statement
The original contributions presented in the study are included in the article/Supplementary Material. Further inquiries can be directed to the corresponding author.
Author contributions
NHS: Conceptualization, Investigation, Methodology, Project administration, Supervision, Validation, Visualization, Data curation, Formal analysis, Writing – original draft, Writing – review & editing. PN: Data curation, Formal analysis, Investigation, Validation, Writing – original draft. SL: Conceptualization, Investigation, Methodology, Project administration, Resources, Supervision, Validation, Visualization, Writing – original draft, Writing – review & editing.
Funding
The author(s) declare that no financial support was received for the research, authorship, and/or publication of this article.
Conflict of interest
The authors declare that the research was conducted in the absence of any commercial or financial relationships that could be construed as a potential conflict of interest.
Publisher’s note
All claims expressed in this article are solely those of the authors and do not necessarily represent those of their affiliated organizations, or those of the publisher, the editors and the reviewers. Any product that may be evaluated in this article, or claim that may be made by its manufacturer, is not guaranteed or endorsed by the publisher.
Supplementary material
The Supplementary Material for this article can be found online at: https://www.frontiersin.org/articles/10.3389/fpsyt.2024.1291362/full#supplementary-material
References
1. WHO. Suicide worldwide in 2019: global health estimates(2021). Available at: https://www.who.int/publications/i/item/9789240026643.
2. WHO. Preventing suicide: a global imperative(2014). Available online at: https://www.who.int/publications/i/item/9789241564779.
3. UNGA. Resolution adopted by the General Assembly on 25 September 2015: Transforming our world: the 2030 Agenda for Sustainable Development. UN General Assembly(2015). Available online at: https://sdgs.un.org/2030agenda.
4. WHO. LIVE LIFE: an implementation guide for suicide prevention in countries. Geneva: World Health Organization (2021). Available at: https://www.who.int/publications/i/item/9789240026629.
5. Ribeiro JD, Franklin JC, Fox KR, Bentley KH, Kleiman EM, Chang BP, et al. Self-injurious thoughts and behaviors as risk factors for future suicide ideation, attempts, and death: a meta-analysis of longitudinal studies. Psychol Med. (2016) 46:225–36. doi: 10.1017/S0033291715001804
6. Franklin JC, Ribeiro JD, Fox KR, Bentley KH, Kleiman EM, Huang X, et al. Risk factors for suicidal thoughts and behaviors: A meta-analysis of 50 years of research. Psychol Bull. (2017) 143:187–232. doi: 10.1037/bul0000084
7. Ritschard G, McArdle JJ. Contemporary issues in exploratory data mining in the behavioral sciences. New York, NY: Routledge (2014).
8. Bernert RA, Hilberg AM, Melia R, Kim JP, Shah NH, Abnousi F. Artificial intelligence and suicide prevention: A systematic review of machine learning investigations. Int J Environ Res Public Health. (2020) 17(16):5929. doi: 10.3390/ijerph17165929
9. Burke TA, Ammerman BA, Jacobucci R. The use of machine learning in the study of suicidal and non-suicidal self-injurious thoughts and behaviors: A systematic review. J Affect Disord. (2019) 245:869–84. doi: 10.1016/j.jad.2018.11.073
10. Belsher BE, Smolenski DJ, Pruitt LD, Bush NE, Beech EH, Workman DE, et al. Prediction models for suicide attempts and deaths: A systematic review and simulation. JAMA Psychiatry. (2019) 76:642–51. doi: 10.1001/jamapsychiatry.2019.0174
11. Page MJ, Moher D, Bossuyt PM, Boutron I, Hoffmann TC, Mulrow CD, et al. PRISMA 2020 explanation and elaboration: updated guidance and exemplars for reporting systematic reviews. BMJ. (2021) 372:n160. doi: 10.1136/bmj.n160
12. Crosby A, Ortega L, Melanson C. Self-directed Violence Surveillance: Uniform Definitions and Recommended Data Elements, Version 1.0. Atlanta (GA): Centers for Disease Control and Prevention, National Center for Injury Prevention and Control. (2011).
13. Wolff RF, Moons KGM, Riley RD, Whiting PF, Westwood M, Collins GS, et al. PROBAST: A tool to assess the risk of bias and applicability of prediction model studies. Ann Intern Med. (2019) 170:51–8. doi: 10.7326/M18-1376
14. Corke M, Mullin K, Angel-Scott H, Xia S, Large M. Meta-analysis of the strength of exploratory suicide prediction models; from clinicians to computers. BJPsych Open. (2021) 7:e26. doi: 10.1192/bjo.2020.162
15. Kusuma K, Larsen M, Quiroz JC, Gillies M, Burnett A, Qian J, et al. The performance of machine learning models in predicting suicidal ideation, attempts, and deaths: A meta-analysis and systematic review. J Psychiatr Res. (2022) 155:579–88. doi: 10.1016/j.jpsychires.2022.09.050
17. Peis I, Olmos PM, Vera-Varela C, Barrigon ML, Courtet P, Baca-Garcia E, et al. Deep sequential models for suicidal ideation from multiple source data. IEEE J BioMed Health Inform. (2019) 23:2286–93. doi: 10.1109/JBHI.6221020
18. McKernan LC, Lenert MC, Crofford LJ, Walsh CG. Outpatient engagement and predicted risk of suicide attempts in fibromyalgia. Arthritis Care Res (Hoboken). (2019) 71:1255–63. doi: 10.1002/acr.23748
19. Colic S, JR D, James Reilly P, Gary Hasey M. Using machine learning algorithms to enhance the management of suicide ideation. Annu Int Conf IEEE Eng Med Biol Soc. (2018) 2018:4936–9. doi: 10.1109/EMBC38410.2018
20. Ge FF, Jiang JW, Wang Y, Yuan C, Zhang W. Identifying suicidal ideation among Chinese patients with major depressive disorder: evidence from a real-world hospital-based study in China. Neuropsychiatr Dis Treat. (2020) 16:665–72. doi: 10.2147/NDT
21. Lopez-Castroman J, Perez-Rodriguez Mde L, Jaussent I, Alegria AA, Artes-Rodriguez A, Freed P, et al. Distinguishing the relevant features of frequent suicide attempters. J Psychiatr Res. (2011) 45:619–25. doi: 10.1016/j.jpsychires.2010.09.017
22. Walsh CG, Ribeiro JD, Franklin JC. Predicting risk of suicide attempts over time through machine learning. Clin Psychol Sci. (2017) 5:457–69. doi: 10.1177/2167702617691560
23. Wei Y-X, Liu B-P, Zhang J, Wang X-T, Chu J, Jia C-X. Prediction of recurrent suicidal behavior among suicide attempters with Cox regression and machine learning: a 10-year prospective cohort study. J Psychiatr Res. (2021) 144:217–24. doi: 10.1016/j.jpsychires.2021.10.023
24. Zheng L, Wang O, Hao SY, Ye CY, Liu MD, Xia MJ, et al. Development of an early-warning system for high-risk patients for suicide attempt using deep learning and electronic health records. Trans Psychiatry. (2020) 10(1):72. doi: 10.1038/s41398-020-0684-2
25. Agne NA, Tisott CG, Ballester P, Passos IC, Ferrao YA. Predictors of suicide attempt in patients with obsessive-compulsive disorder: An exploratory study with machine learning analysis. Psychol Med. (2022) 52(4):715–25. doi: 10.1017/S0033291720002329
26. Simon GE, Johnson E, Lawrence JM, Rossom RC, Ahmedani B, Lynch FL, et al. Predicting suicide attempts and suicide deaths following outpatient visits using electronic health records. Am J Psychiatry. (2018) 175:951–60. doi: 10.1176/appi.ajp.2018.17101167
27. Modai I, Greenstain S, Weizman A, Mendel S. Backpropagation and adaptive resonance theory in predicting suicidal risk. Med Inform (Lond). (1998) 23:325–30. doi: 10.3109/14639239809025368
28. Tran T, Luo W, Phung D, Harvey R, Berk M, Kennedy RL, et al. Risk stratification using data from electronic medical records better predicts suicide risks than clinician assessments. BMC Psychiatry. (2014) 14:76. doi: 10.1186/1471-244X-14-76
29. DelPozo-Banos M, John A, Petkov N, Berridge DM, Southern K, LL K, et al. Using neural networks with routine health records to identify suicide risk: feasibility study. JMIR Ment Health. (2018) 5:e10144. doi: 10.2196/10144
30. Gradus JL, Rosellini AJ, Horvath-Puho E, Street AE, Galatzer-Levy I, Jiang T, et al. Prediction of sex-specific suicide risk using machine learning and single-payer health care registry data from Denmark. JAMA Psychiatry. (2020) 77:103–. doi: 10.1001/jamapsychiatry.2019.2905
31. Adams RS, Jiang T, Rosellini AJ, Horváth-Puhó E, Street AE, Keyes KM, et al. Sex-specific risk profiles for suicide among persons with substance use disorders in Denmark. Addiction. (2021) 116:2882–92. doi: 10.1111/add.15455
32. Cho S-E, Geem ZW, Na K-S. Prediction of suicide among 372,813 individuals under medical check-up. J Psychiatr Res. (2020) 131:9–14. doi: 10.1016/j.jpsychires.2020.08.035
33. Cho S-E, Geem ZW, Na K-S. Development of a suicide prediction model for the elderly using health screening data. Int J Environ Res Public Health. (2021) 18(19):10150. doi: 10.3390/ijerph181910150
34. Fazel S, Wolf A, Larsson H, Mallett S, Fanshawe TR. The prediction of suicide in severe mental illness: development and validation of a clinical prediction rule (OxMIS). Transl Psychiatry. (2019) 9:98. doi: 10.1038/s41398-019-0428-3
35. Choi SB, Lee W, Yoon JH, Won JU, Kim DW. Ten-year prediction of suicide death using Cox regression and machine learning in a nationwide retrospective cohort study in South Korea. J Affect Disord. (2018) 231:8–14. doi: 10.1016/j.jad.2018.01.019
36. Barak-Corren Y, Castro VM, Javitt S, Hoffnagle AG, Dai Y, Perlis RH, et al. Predicting suicidal behavior from longitudinal electronic health records. Am J OF Psychiatry. (2017) 174:154–62. doi: 10.1176/appi.ajp.2016.16010077
37. Fan PH, Guo XJ, Qi XG, Matharu M, Patel R, Sakolsky D, et al. Prediction of suicide-related events by analyzing electronic medical records from PTSD patients with bipolar disorder. Brain Sci. (2020) 10(11):784. doi: 10.3390/brainsci10110784
38. Czyz EK, Koo HJ, Al-Dajani N, King CA, Nahum-Shani I. Predicting short-term suicidal thoughts in adolescents using machine learning: developing decision tools to identify daily level risk after hospitalization. Psychol Med. (2023) 53(7):2982–91. doi: 10.1017/S0033291721005006
39. Na K-S, Geem ZW, Cho S-E. The development of a suicidal ideation predictive model for community-dwelling elderly aged >55 years. Neuropsychiatr Dis Treat. (2022) 18:163–72. doi: 10.2147/NDT.S336947
40. Hill RM, Oosterhoff B, Kaplow JB. Prospective identification of adolescent suicide ideation using classification tree analysis: Models for community-based screening. J Consult Clin Psychol. (2017) 85:702–11. doi: 10.1037/ccp0000218
41. Ryu S, Lee H, Lee D-K, Park K. Use of a machine learning algorithm to predict individuals with suicide ideation in the general population. Psychiatry Invest. (2018) 15:1030–6. doi: 10.30773/pi.2018.08.27
42. Jordan P, Shedden-Mora MC, Löwe B. Predicting suicidal ideation in primary care: An approach to identify easily assessable key variables. Gen Hosp Psychiatry. (2018) 51:106–11. doi: 10.1016/j.genhosppsych.2018.02.002
43. Gradus JL, King MW, Galatzer-Levy I, Street AE. Gender differences in machine learning models of trauma and suicidal ideation in veterans of the Iraq and Afghanistan wars. J Trauma Stress. (2017) 30:362–71. doi: 10.1002/jts.22210
44. Rosellini AJ, Stein MB, Benedek DM, Bliese PD, Chiu WT, Hwang I, et al. Predeployment predictors of psychiatric disorder-symptoms and interpersonal violence during combat deployment. Depress Anxiety. (2018) 35:1073–80. doi: 10.1002/da.22807
45. van Mens K, de Schepper C, Wijnen B, Koldijk SJ, Schnack H, de Looff P, et al. Predicting future suicidal behavior in young adults, with different machine learning techniques: A population-based longitudinal study. J Affect Disord. (2020) 271:169–77. doi: 10.1016/j.jad.2020.03.081
46. 홍 세 훈, 이 동 원. 의사결정나무 분석법을 이용한 우울 노인 중 자살 고위험군 규명. J Korean Acad Community Health Nursing/Jiyeog Sahoe Ganho Hakoeji. (2019) 30:130–40.
47. Burke TA, Jacobucci R, Ammerman BA, Piccirillo M, McCloskey MS, Heimberg RG, et al. Identifying the relative importance of non-suicidal self-injury features in classifying suicidal ideation, plans, and behavior using exploratory data mining. Psychiatry Res. (2018) 262:175–83. doi: 10.1016/j.psychres.2018.01.045
48. Kuroki Y, Tilley JL. Recursive partitioning analysis of lifetime suicidal behaviors in Asian Americans. Asian Am J Psychol. (2012) 3:17–28. doi: 10.1037/a0026586
49. Harman G, Kliamovich D, Morales AM, Gilbert S, Barch DM, Mooney MA, et al. Prediction of suicidal ideation and attempt in 9 and 10 year-old children using transdiagnostic risk features. PloS One. (2021) 16:e0252114. doi: 10.1371/journal.pone.0252114
50. Jung JS, Park SJ, Kim EY, Na K-S, Kim YJ, Kim KG. Prediction models for high risk of suicide in Korean adolescents using machine learning techniques. PloS One. (2019) 14:e0217639. doi: 10.1371/journal.pone.0217639
51. Naghavi A, Teismann T, Asgari Z, Mohebbian MR, Mansourian M, Mañanas M. Accurate diagnosis of suicide ideation/behavior using robust ensemble machine learning: A university student population in the middle east and north Africa (MENA) region. Diagnost (Basel). (2020) 10(11):956. doi: 10.3390/diagnostics10110956
52. Huang XYN, Ribeiro JD, Franklin JC. The differences between individuals engaging in nonsuicidal self-injury and suicide attempt are complex (vs. Complicated or simple). Front Psychiatry. (2020) 11:239. doi: 10.3389/fpsyt.2020.00239
53. Stanley IH, Chu C, Gildea SM, Hwang IH, King AJ, Kennedy CJ, et al. Predicting suicide attempts among U.S. Army soldiers after leaving active duty using information available before leaving active duty: results from the Study to Assess Risk and Resilience in Servicemembers-Longitudinal Study (STARRS-LS). Mol Psychiatry. (2022) 27(3):1631–9. doi: 10.1038/s41380-021-01423-4
54. Shen YM, Zhang WY, Chan BSM, Zhang YR, Meng FC, Kennon EA, et al. Detecting risk of suicide attempts among Chinese medical college students using a machine learning algorithm. J Affect Disord. (2020) 273:18–23. doi: 10.1016/j.jad.2020.04.057
55. Burke TA, Jacobucci R, Ammerman BA, Alloy LB, Diamond G. Using machine learning to classify suicide attempt history among youth in medical care settings. J Affect Disord. (2020) 268:206–14. doi: 10.1016/j.jad.2020.02.048
56. Mann JJ, Ellis SP, Waternaux CM, Liu X, Oquendo MA, Malone KM, et al. Classification trees distinguish suicide attempters in major psychiatric disorders: a model of clinical decision making. J Clin Psychiatry. (2008) 69:23–31. doi: 10.4088/JCP.v69n0104
57. Marcon G, Massaro Carneiro Monteiro G, Ballester P, Cassidy RM, Zimerman A, Brunoni AR, et al. Who attempts suicide among medical students? Acta Psychiatrica Scand. (2020) 141(3):254–64. doi: 10.1111/acps.13137
58. Hill RM, Oosterhoff B, Do C. Using machine learning to identify suicide risk: A classification tree approach to prospectively identify adolescent suicide attempters. Arch Suicide Res. (2020) 24:218–35. doi: 10.1080/13811118.2019.1615018
59. Miché M, Studerus E, Meyer AH, Gloster AT, Beesdo-Baum K, Wittchen HU, et al. Prospective prediction of suicide attempts in community adolescents and young adults, using regression methods and machine learning. J Affect Disord. (2020) 265:570–8. doi: 10.1016/j.jad.2019.11.093
60. Lyu JC, Zhang J. BP neural network prediction model for suicide attempt among Chinese rural residents. J OF Affect Disord. (2019) 246:465–73. doi: 10.1016/j.jad.2018.12.111
61. Baca-Garcia E, Perez-Rodriguez MM, Saiz-Gonzalez D, Basurte-Villamor I, Saiz-Ruiz J, Leiva-Murillo JM, et al. Variables associated with familial suicide attempts in a sample of suicide attempters. Prog Neuropsychopharmacol Biol Psychiatry. (2007) 31:1312–6. doi: 10.1016/j.pnpbp.2007.05.019
62. Modai I, Valevski A, Solomish A, Kurs R, Hines IL, Ritsner M, et al. Neural network detection of files of suicidal patients and suicidal profiles. Med Inform Internet Med. (1999) 24:249–56. doi: 10.1080/146392399298276
63. Wei ZY, Mukherjee S. Health-behaviors associated with the growing risk of adolescent suicide attempts: A data-driven cross-sectional study. Am J OF Health Promotion. (2021) 35:688–93. doi: 10.1177/0890117120977378
64. Ryu S, Lee H, Lee D-K, Kim S-W, Kim C-E. Detection of suicide attempters among suicide ideators using machine learning. Psychiatry Invest. (2019) 16:588–93. doi: 10.30773/pi.2019.06.19
65. García de la Garza Á, Blanco C, Olfson M, Wall MM. Identification of suicide attempt risk factors in a national US survey using machine learning. JAMA Psychiatry (Chicago Ill). (2021) 78:398–406. doi: 10.1001/jamapsychiatry.2020.4165
66. Lee J, Jang H, Kim J, Min S. Development of a suicide index model in general adolescents using the South Korea 2012-2016 national representative survey data. Sci Rep. (2019) 9:1846. doi: 10.1038/s41598-019-38886-z
67. Lee Y, Kim H, Lee Y, Jeong H. Comparison of the prediction model of adolescents’ suicide attempt using logistic regression and decision tree: secondary data analysis of the 2019 youth health risk behavior web-based survey. J Korean Acad Nursing. (2021) 51:40–53. doi: 10.4040/jkan.20207
68. Rosellini AJ, Stein MB, Benedek DM, Bliese PD, Chiu WT, Hwang I, et al. Using self-report surveys at the beginning of service to develop multi-outcome risk models for new soldiers in the U. S Army Psychol Med. (2017) 47:2275–87. doi: 10.1017/S003329171700071X
69. MaChado CD, Ballester PL, Cao B, Mwangi B, Caldieraro MA, Kapczinski F, et al. Prediction of suicide attempts in a prospective cohort study with a nationally representative sample of the US population. Psychol Med. (2022) 52(14):2985–96. doi: 10.1017/S0033291720004997
70. Horvath A, Dras M, Lai CCW, Boag S. Predicting suicidal behavior without asking about suicidal ideation: Machine learning and the role of borderline personality disorder criteria. Suicide Life Threat Behav. (2021) 51(3):455–66. doi: 10.1111/sltb.12719
71. Hettige NC, Nguyen TB, Yuan C, Rajakulendran T, Baddour J, Bhagwat N, et al. Classification of suicide attempters in schizophrenia using sociocultural and clinical features: A machine learning approach. Gen Hosp Psychiatry. (2017) 47:20–8. doi: 10.1016/j.genhosppsych.2017.03.001
72. Parghi N, Chennapragada L, Barzilay S, Newkirk S, Ahmedani B, Lok B, et al. Assessing the predictive ability of the suicide crisis inventory for near-term suicidal behavior using machine learning approaches. Int J Methods Psychiatr Res. (2021) 30(1):e1863. doi: 10.1002/mpr.1863
73. Passos IC, Mwangi B, Cao B, Hamilton JE, Wu M-J, Zhang XY, et al. Identifying a clinical signature of suicidality among patients with mood disorders: A pilot study using a machine learning approach. J Affect Disord. (2016) 193:109–16. doi: 10.1016/j.jad.2015.12.066
74. Choi KS, Kim S, Kim B-H, Jeon HJ, Kim J-H, Jang JH, et al. Deep graph neural network-based prediction of acute suicidal ideation in young adults. Sci Rep. (2021) 11:15828. doi: 10.1038/s41598-021-99825-5
75. Bae SM, Lee SA, Lee SH. Prediction by data mining, of suicide attempts in Korean adolescents: a national study. Neuropsychiatr Dis Treat. (2015) 11:2367–75. doi: 10.2147/NDT
76. Weller O, Sagers L, Hanson C, Barnes M, Snell Q, Shannon Tass E. Predicting suicidal thoughts and behavior among adolescents using the risk and protective factor framework: A large-scale machine learning approach. PloS One. (2021) 16:e0258535. doi: 10.1371/journal.pone.0258535
77. Bernecker SL, Zuromski KL, Gutierrez PM, Joiner TE, King AJ, Liu H, et al. Predicting suicide attempts among soldiers who deny suicidal ideation in the Army Study to Assess Risk and Resilience in Servicemembers (Army STARRS). Behav Res Ther. (2019) 120:103350. doi: 10.1016/j.brat.2018.11.018
78. Buchman-Schmitt JM, Stanley IH, Gallyer AJ, Chu C, Gutierrez PM, Hanson JE, et al. Military Suicide Research Consortium common data elements: Bifactor analysis and longitudinal predictive ability of suicidal ideation and suicide attempts within a clinical sample. Psychol Assess. (2020) 32:609–22. doi: 10.1037/pas0000817
79. Indrawan G, Sudiarsa I, Agustini K, Sariyasa S. Smooth support vector machine for suicide-related behaviors prediction. Int J Electrical Comput Eng. (2018) 8:3399. doi: 10.11591/ijece.v8i5
80. Chen Q, Zhang-James YL, Barnett EJ, Lichtenstein P, Jokinen J, D’Onofrio BM, et al. Predicting suicide attempt or suicide death following a visit to psychiatric specialty care: A machine learning study using Swedish national registry data. PloS Med. (2020) 17(11):e1003416. doi: 10.1371/journal.pmed.1003416
81. Karmakar C, Luo W, Tran T, Berk M, Venkatesh S. Predicting risk of suicide attempt using history of physical illnesses from electronic medical records. JMIR Ment Health. (2016) 3:e19. doi: 10.2196/mental.5475
82. Haroz EE, Walsh CG, Goklish N, Cwik MF, O’Keefe V, Barlow A. Reaching Those at Highest Risk for Suicide: Development of a Model Using Machine Learning Methods for use With Native American Communities. Suicide Life Threat Behav. (2020) 50:422–36. doi: 10.1111/sltb.12598
83. Ballester PL, Cardoso TA, Moreira FP, da Silva RA, Mondin TC, Araujo RM, et al. 5-year incidence of suicide-risk in youth: A gradient tree boosting and SHAP study. J Affect Disord. (2021) 295:1049–56. doi: 10.1016/j.jad.2021.08.033
84. Smith EG, Kim HM, Ganoczy D, Stano C, Pfeiffer PN, Valenstein M. Suicide risk assessment received prior to suicide death by Veterans Health Administration patients with a history of depression. J Clin Psychiatry. (2013) 74:226–32. doi: 10.4088/JCP.12m07853
85. Sanderson M, Bulloch AGM, Wang JL, Williamson T, Patten SB. Predicting death by suicide using administrative health care system data: Can feedforward neural network models improve upon logistic regression models? J OF Affect Disord. (2019) 257:741–7. doi: 10.1016/j.jad.2019.07.063
86. Sanderson M, Bulloch AGM, Wang J, Williamson T, Patten SB. Predicting death by suicide using administrative health care system data: Can recurrent neural network, one-dimensional convolutional neural network, and gradient boosted trees models improve prediction performance? J Affect Disord. (2020) 264:107–14. doi: 10.1016/j.jad.2019.12.024
87. Sanderson M, Bulloch AGM, Wang JL, Williams KG, Williamson T, Patten SB. Predicting death by suicide following an emergency department visit for parasuicide with administrative health care system data and machine learning. E Clin Med. (2020) 20:100281. doi: 10.1016/j.eclinm.2020.100281
88. Wallace GT, Conner BT, Shillington AM. Classification trees identify shared and distinct correlates of nonsuicidal self-injury and suicidal ideation across gender identities in emerging adults. Clin Psychol Psychother. (2021) 28(3):682–93. doi: 10.1002/cpp.2530
89. Canetto SS. Women and suicidal behavior: a cultural analysis. Am J Orthopsychiatry. (2008) 78:259–66. doi: 10.1037/a0013973
90. Canetto SS, Sakinofsky I. The gender paradox in suicide. Suicide Life Threat Behav. (1998) 28:1–23. doi: 10.1111/j.1943-278X.1998.tb00622.x
91. Chawla NV, Bowyer KW, Hall LO, Kegelmeyer WP. SMOTE: synthetic minority over-sampling technique. J Artif Intell Res. (2002) 16:321–57. doi: 10.1613/jair.953
92. Liu L, Wu X, Li S, Li Y, Tan S, Bai Y. Solving the class imbalance problem using ensemble algorithm: application of screening for aortic dissection. BMC Med Inf Decision Making. (2022) 22:82. doi: 10.1186/s12911-022-01821-w
93. Ngufor C, Van Houten H, Caffo BS, Shah ND, McCoy RG. Mixed effect machine learning: A framework for predicting longitudinal change in hemoglobin A1c. J Biomed Inform. (2019) 89:56–67. doi: 10.1016/j.jbi.2018.09.001
94. Lecca P. Machine learning for causal inference in biological networks: perspectives of this challenge. Front Bioinf. (2021) 1. doi: 10.3389/fbinf.2021.746712
95. Karmakar S, Majumder SG, Gangaraju D. (2023). Causal Inference and Causal Machine Learning with Practical Applications: The paper highlights the concepts of Causal Inference and Causal ML along with different implementation techniques. In Proceedings of the 6th Joint International Conference on Data Science & Management of Data (10th ACM IKDD CODS and 28th COMAD). New York, NY, USA: Association for Computing Machinery. pp. 324–6. doi: 10.1145/3570991.3571052
Keywords: death by suicide, suicidal thoughts, suicide attempt, machine learning, predictive risk factors
Citation: Somé NH, Noormohammadpour P and Lange S (2024) The use of machine learning on administrative and survey data to predict suicidal thoughts and behaviors: a systematic review. Front. Psychiatry 15:1291362. doi: 10.3389/fpsyt.2024.1291362
Received: 09 September 2023; Accepted: 12 February 2024;
Published: 04 March 2024.
Edited by:
Ashwani Kumar Mishra, All India Institute of Medical Sciences, IndiaReviewed by:
Robert Suchting, University of Texas Health Science Center at Houston, United StatesAnna Ceraso, University of Brescia, Italy
Copyright © 2024 Somé, Noormohammadpour and Lange. This is an open-access article distributed under the terms of the Creative Commons Attribution License (CC BY). The use, distribution or reproduction in other forums is permitted, provided the original author(s) and the copyright owner(s) are credited and that the original publication in this journal is cited, in accordance with accepted academic practice. No use, distribution or reproduction is permitted which does not comply with these terms.
*Correspondence: Nibene H. Somé, Nsome@uwo.ca