- 1Joint Institute of Tobacco and Health, Kunming, Yunnan, China
- 2State Key Laboratory for Diagnosis and Treatment of Infectious Diseases, The First Affiliated Hospital, Collaborative Innovation Center for Diagnosis and Treatment of Infectious Diseases, Zhejiang University School of Medicine, Hangzhou, Zhejiang, China
Backgrounds: Tobacco smoking is an important risk factor for coronary artery disease (CAD), but the genetic mechanisms linking smoking to CAD remain largely unknown.
Methods: We analyzed summary data from the genome-wide association study (GWAS) of the UK Biobank for CAD, plasma lipid concentrations (n = 184,305), and smoking (n = 337,030) using different biostatistical methods, which included LD score regression and Mendelian randomization (MR).
Results: We identified SNPs shared by CAD and at least one smoking behavior, the genes where these SNPs are located were found to be significantly enriched in the processes related to lipoprotein metabolic, chylomicron-mediated lipid transport, lipid digestion, mobilization, and transport. The MR analysis revealed a positive correlation between smoking cessation and decreased risk for CAD when smoking cessation was considered as exposure (p = 0.001), and a negative correlation between the increased risk for CAD and smoking cessation when CAD was considered as exposure (p = 2.95E-08). This analysis further indicated that genetic liability for smoking cessation increased the risk of CAD.
Conclusion: These findings inform the concomitant conditions of CAD and smoking and support the idea that genetic liabilities for smoking behaviors are strongly associated with the risk of CAD.
Introduction
Tobacco smoking is one of the most important public health problems world-wide, accounting for 9% of deaths (1). Several epidemiologic studies have proved that tobacco smoking is a major risk factor for many diseases such as lung cancer and cardiovascular and respiratory diseases (1). Lung cancer is the most prevalent smoking-associated cause of death, followed by ischemic heart disease and chronic airway obstruction. Tobacco smoking and its impact on the respiratory system caused an estimated 8 million deaths per year, with more than 10% of these deaths related to second-hand smoke (2). In the United States, tobacco smoking is associated with 30% of all CAD-related deaths each year (3) and also doubles the risk of premature cardiovascular deaths (4).
Several epidemiologic studies have revealed that tobacco smoking increases the incidence of fatal CAD and associates with various cardiovascular diseases (5–8). Extensive clinical evidence has supported the idea that tobacco smoking causes multiple genetic and epigenetic abnormalities in the respiratory epithelium (9, 10). In a previous study, Sabater-Ileal and colleagues identified a genetic locus that influences both lung function and CAD (11), although the findings were not genome-wide in scale and were underpowered due to a small sample size.
Tobacco contains more than 4,000 chemicals (12), and the exact toxic components and the mechanisms involved in tobacco-related CAD and cardiovascular dysfunction are still unknown. Recently, a genetic predisposition to the development of atherogenesis in individuals exposed to cigarette smoke has been reported. The commonly documented examples are CYP1A1 MSP polymorphism and certain endothelial NO synthase intron 4 polymorphisms. Both increase the susceptibility to cigarette smoke exposure-related atherosclerotic diseases including multi-vessel CAD and myocardial infarction (MI) (13, 14). Given that much of the available data were derived from observational studies, which are unable to account fully for confounding and reverse causation, the genetic correlation and causal relations between smoking and cardiovascular diseases remain to be determined.
The principle of Mendelian randomization (MR) relies on the basic laws of Mendelian genetics, segregation, and independent association. When these principles hold at a population level, the influence of confounding factors can be reduced because religion, growth, environment, and other confounding factors are considered to be random (15). Given that alleles are randomly allocated and become fixed at conception, MR studies are less susceptible to reverse causality than are observational studies.
In this study, we examined the pleiotropic effect of tobacco smoking and CAD using publicly available GWAS summary statistics. Then, we used bidirectional MR method to reveal the nature of the causal relations between CAD and tobacco smoking. Finally, we determined the biological processes or pathways involved in the comorbidity of these two diseases.
Materials and methods
GWAS summary data sets
The GWAS summary data for CAD and plasma lipid concentrations have been described in a previous report (16). Briefly, the summary statistics of a large GWAS meta-analysis comprising more than 120,000 CAD cases and 339,115 controls were obtained from CARDIoGRAMplusC4D Consortium website (http://www.cardiogramplusc4d.org/data-downloads/). A total of 9,149,595 variants were included either in the CARDIoGRAMplusC4D 1,000 Genomes–imputed GWAS or the MIGen/CARDIoGRAM Exome chip study. The smoking data were obtained from the large GSCAN summary statistics (17). The GSCAN investigated four smoking-related phenotypes, including age at initiation of regular smoking (AgeSmk; n = 341,427), whether an individual had ever smoked regularly (SmkInit; n = 1,232,091), cigarettes smoked per day (CPD; n = 337,334), and smoking cessation (SmkCes; n = 547,219). The GWAS summary statistics for different smoking phenotypes can be found at https://conservancy.umn.edu/handle/11299/201564.
Further, we obtained published GWAS meta-analysis association data for lipid concentrations from the Center for Statistical Genetics, which was a joint analysis that examined 188,577 individuals whose genomic DNA samples were genotyped with two platforms from multiple studies (18). Complete GWAS summary statistics were downloaded from webpage http://csg.sph.umich.edu/willer/public/lipids2013/.
Estimation of genetic correlation by LD score regression (LDSC)
The genetic correlations (rg) between CAD and smoking behaviors were estimated by LDSC (19). Pairwise LD r2 among SNPs was conducted using pre-computed LD scores with the 1,000 Genomes Project reference panel of subjects of European ancestry. Quality control steps were adopted from LD scores default procedures, including imputation quality >0.9 and minor allele frequency > 0.1. Moreover, all SNPs retained for further analysis were merged with SNPs in the HapMap 3 reference panel. Correlation was considered significant at a corrected p value of <0.05 by Bonferroni correction.
Mendelian randomization (MR)
We extracted the effect estimates and standard errors (Ses) from relevant GWAS and employed TwoSampleMR (v. 0.4.22) R package to clarify the potential causal effect for both smoking and CAD (20). The following strategies were used to identify genetic instruments. First, we filtered GWAS summary datasets to require shared susceptible loci in both smoking and CAD. The variants showing genome-wide significance (p < 5 × 10−8) in GWAS for CAD were considered to be candidate variants, and then we checked the significance of these genetic loci separately in four other smoking behaviors: smoking initiation age, smoking cessation, CPD, and age of initiation in GSCAN studies. The common SNPs were harmonized using default parameters within the built-in “harmonize data” function and then trimmed by PLINK (v. 1.07) to obtain independent risk variants for each disease (21).
To start, the MR analysis was performed by generating instrumental variable estimates for each SNP. The averaged causal estimate of each SNP was calculated using the inverse–variance-weighted (IVW) method, i.e., specifically defined as the beta coefficient associated with SNP-CAD divided by the beta coefficient associated with SNP-smoking behaviors (22). In addition, we used a series of sensitivity analyses, which included weighted median and MR Egger, to evaluate the reliability of our results.
Gene and pathway analysis
The gene-based analysis that links SNPs to genes was conducted using MAGMA with default settings. To gain biological insights into shared genes, we used the WebGestalt tool (23) to assess enrichment of the identified shared gene set in the Gene Ontology (GO) biological processes with redundant GO terms been removed. Both analyses were based on shared genes that were identified from cross-trait meta-analysis. Pathways with a false discovery rate (FDR) < 0.05 were considered significant.
Results
Susceptible loci shared by CAD and smoking behaviors
To investigate the genetic overlap between CAD and smoking behaviors, we used GWAS summary data from large-scale genome-wide studies (Supplementary Table S1). We detected a great number of significantly associated SNPs overlapped between CAD risk loci and at least one smoking phenotype (Supplementary Table S2). Of the CAD GWAS loci, 2091 SNPs (35.01%) showed nominal significance in CPD, 526 SNPs (25.16%) showed nominal significance for smoking initiation, 317 SNPs (15.16%) for smoking cession, and 85 SNPs (4.07%) for age at smoking initiation. Notably, 24 SNPs reached genome-wide significance (Figure 1).
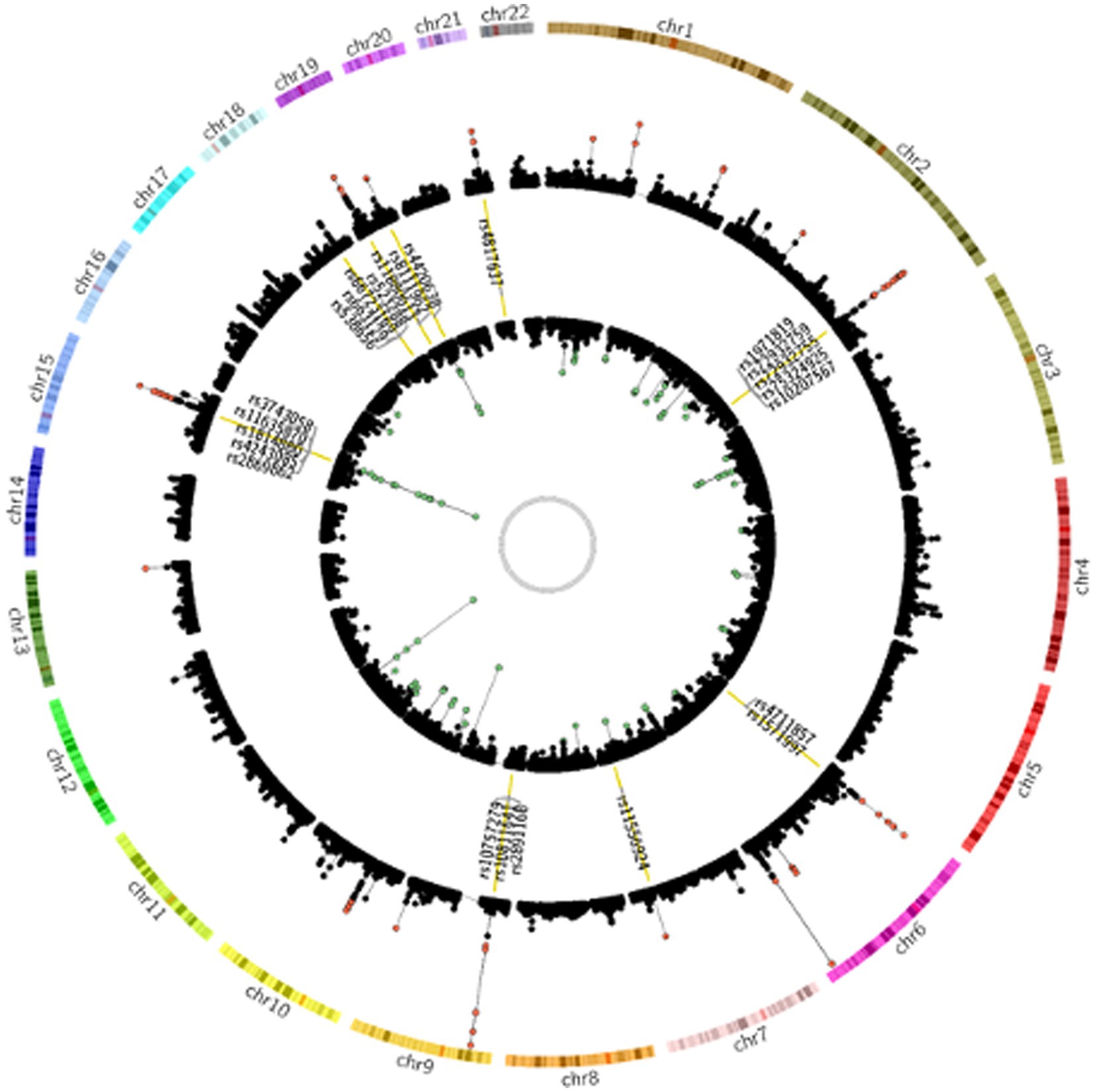
Figure 1. Genetic susceptibility map for CAD and smoking behaviors. Outer ring defines location of 22 human autosomes. Scatter plots in second and fourth rings demonstrate analogy of Manhattan plot for association results from CAD and smoking behaviors, respectively. Altitude of each dot represents statistical significance as −log10 (P). SNPs that reached genome-wide significance are colored red for CAD and green for smoking behaviors. Yellow bars in third ring mark 24 CAD risk loci at least nominally associated with smoking behaviors, and tag SNPs in these loci are labeled.
Genetic correlations between CAD and smoking behaviors
We used the LDSC method to test for the genome-wide correlations between CAD and smoking behaviors. Significant genetic correlations were found between CAD and all the smoking-related traits with the smallest p values <1 × 10−18 (Figure 2). We observed significant positive genetic correlations between CAD and CPD, smoking initiation, and smoking cessation (rg > 0.2), but a negative genetic correlation between CAD and age at initiation (rg < −0.2).
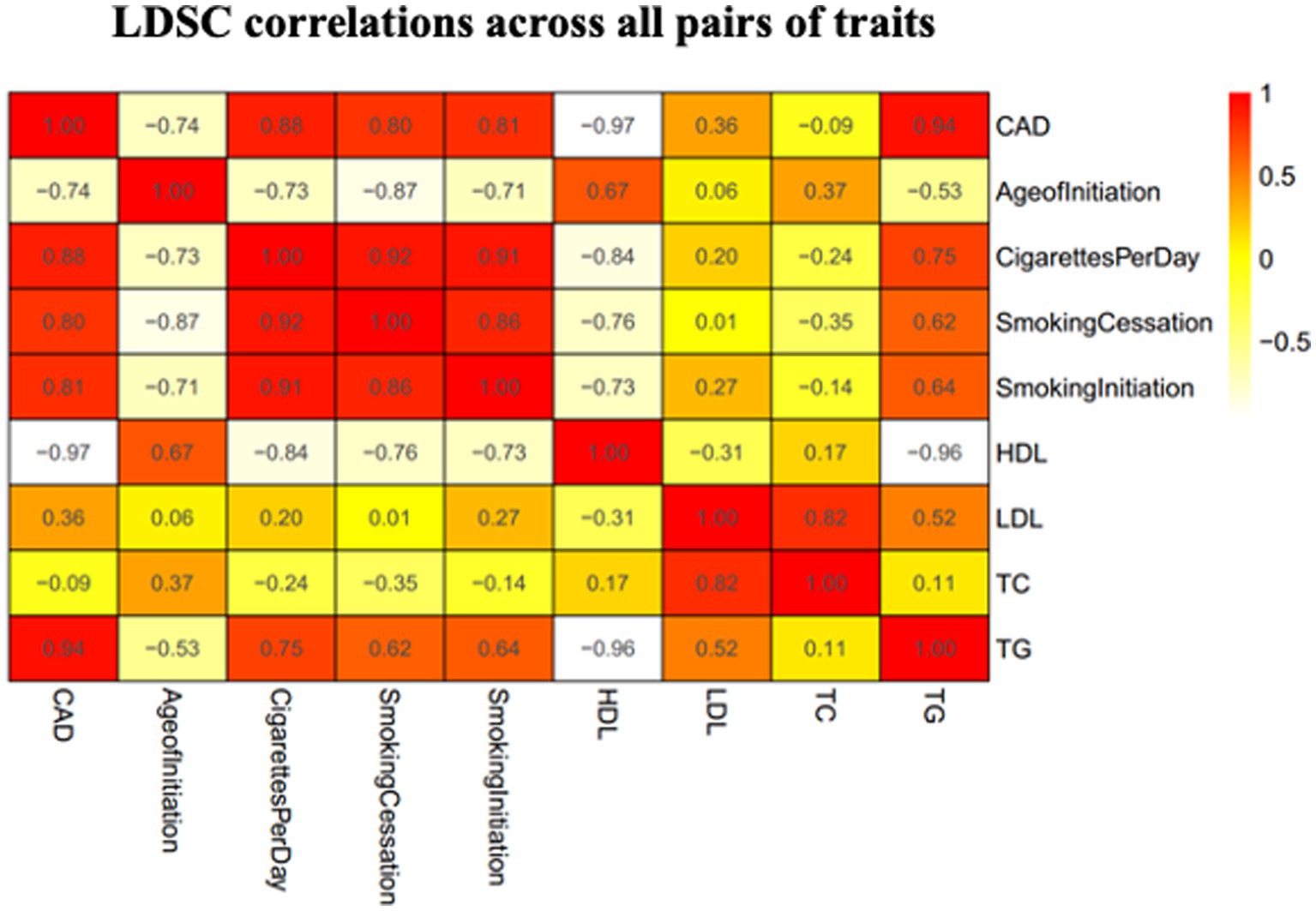
Figure 2. Heatmap showing patterns of genetic correlations across CAD and smoking. Red color indicates a positive correlation and yellow color indicates a negative correlation. CAD, coronary artery disease; HDL, high-density lipoprotein; AgeofInitiation, age of Initiation; CigarettesPerDay, cigarettes per day; SmokingCessation, smoking cessation; SmokingInitiation, smoking Initiation; LDL, low-density lipoprotein; TG, triglycerides; TC, total cholesterol.
Mendelian randomization analysis
Considering the presence of potential LD relations among those significant SNPs of interest, we performed p value-informed LD pruning with the goal of obtaining independent GWAS SNPs. This led to the identification of 15, 63, 18, and 152 independent SNPs for Age of Initiation, CPD, Smoking Cessation and Smoking Initiation, respectively. Bi-directional MR analysis provided strong evidence that smoking initiation increased the risk of CAD (IVW: β = 0.191; p = 2.59 × 10−6) with a consistent direction of effect in all three MR methods (Table 1). There also was evidence for a consistent but weaker genetic liability for smoking cessation on CAD (IVW: β = 0.234; p = 0.001). The same findings were observed for age at initiation as the instrument on CAD (IVW: β = 0.295; p = 0.039).
On the other hand, we obtained negative correlations between CPD and CAD, although the statistic was less significant. Only the result of MR Egger was significant. When treating CAD as an instrument, strong evidence of decreased risk of smoking cessation by CAD was observed (IVW: β = −0.046; p = 2.95 × 10−8; Table 2). The effects of CAD on other smoking behaviors became even weaker and inconsistent across the different methods.
Biological pathway and enrichment analysis
We performed pathway analyses to identify biological pathways enriched for shared genetic loci related to smoking and CAD based on significant cross-trait meta-analysis results. For a detailed list of the overlapped genes and SNPs between CAD and smoking-related phenotypes, please refer to Table 3. Pathway analysis showed that the SNP-related genes were significantly enriched in lipoprotein metabolic, chylomicron-mediated lipid transport, lipid digestion, mobilization, and transport (Table 4). The GO analysis suggested that shared genes in CAD and smoking behaviors were enriched in triglyceride-rich lipoprotein particle clearance, blood vessel development, and very-low-density lipoprotein particle clearance (Table 5).
Discussion
In this study, we revealed the genetic correlation and causal relations between smoking and CAD, providing a comprehensive evaluation of the shared genetic etiology of tobacco smoking and cardiovascular diseases. Our findings have highlighted the discovery that different smoking behaviors have strong associations with CAD, specifically, the correlation between smoking initiation, smoking cessation, and CAD.
The approach to MR is based on the assumptions that: (1) the genetic marker is associated with the exposure; (2) the genetic marker is independent of any confounding factors; and (3) there is no association between the genetic marker and outcome except through confounding factors. However, it should be acknowledged that these assumptions generally are not easy to evaluate. Results from the present MR study were based on data from the GWAS, which has corroborated the results obtained from conventional prospective observational studies that confirmed that tobacco smoking is a risk factor for CAD (5–8).
To our knowledge, this study represents one of a few large-scale genome-wide analysis to investigate the genetic overlap between smoking and CAD (24–26). Similar to the findings from these reports, our analyses also revealed strong associations between smoking initiation, smoking cessation, and CAD. Further, we found a significant positive association between smoking initiation and CAD when smoking initiation was considered as exposure (inverse–variance-weighted: β = 0.191; p = 2.59E-06; weighted median: β = 0.193; p = 3.35E-06), suggesting that smokers are more susceptible to CAD. In addition, we found a negative correlation between CAD and smoking cessation when CAD was considered as exposure (inverse–variance-weighted: β = −0.046; p = 2.95E-08; weighted median: β = 0.193; p = 3.35E-06). This indicates that patients with CAD are less likely to quit smoking, possibly because of tobacco addiction. Together, these findings demonstrate the presence of shared genetic etiologies between tobacco smoking and CAD.
Moreover, we found strong evidence of a genetic correlation between CAD and serum high-density lipoprotein (HDL) and low-density lipoprotein (LDL) as well, which is consistent with the results reported previously (27–29). We observed a positive correlation between LDL and CAD when LDL was considered as the exposure (inverse–variance-weighted: β = 0.387; p = 4.96E-43; weighted median: β = 0.406; p = 7.41E-39), and a negative correlation between HDL and CAD when HDL was considered as the exposure (inverse–variance-weighted: β = −0.245; p = 1.19E-14; weighted median: β = −0.188; p = 2.66E-10). As is well documented in the literature, LDL is a strong risk factor for CAD (27–29) whereas HDL is an anti-atherosclerotic plasma lipoprotein and a protective factor against CAD (30–32).
Further, we found that smoking has significant associations with HDL and LDL. A positive correlation between CPD and LDL was observed when CPD was considered as exposure (inverse–variance-weighted: β =0.06; p = 0.01; weighted median: β = 0.06; p = 0.02), and a negative correlation between CPD and HDL when CPD was considered as exposure (inverse–variance-weighted: β = −0.06; p = 0.005; weighted median: β = −0.06; p = 0.02). There also is a positive correlation between LDL and smoking cessation when LDL was considered as exposure (inverse–variance-weighted: β = −0.03; p = 0.002; weighted median: β = −0.04; p = 2.43E-5). Taken together, these findings provided a clear indication that smoking increases the risk of CAD by affecting the regulation of LDL and HDL, which needs to be further investigated.
We also performed GO enrichment and KEGG pathway analyses based on the genes where the SNPs overlapped between CAD and smoking-related phenotypes are located. We found several functions and pathways to be related to the lipoprotein metabolic and blood vessel development, which are all closely associated with CAD. It has been reported that the APOE–APOC1–APOC2–APOC4 cluster was significantly related to lipoprotein-associated phospholipase A2 mass and activity and CAD (33). Interestingly, the SNP rs4420638 located downstream of the APOC1 gene was found to be significantly related to smoking cessation (p = 7.4E-6) (34). Moreover, as a brain eQTL based on the information from BRAINEAC, rs4420638, this SNP has been linked to Alzheimer’s disease (35–40) and cognitive decline (41).
We used non-overlapping data sources in the context of summary-level MR analysis of exposure and outcome, which greatly improved the confidence in the causal effect estimates. In addition, through a range of sensitivity analysis methods, similar causal estimates and consistent causal inferences could be drawn. However, this study had limitations as well. First, there was a stark difference in sample sizes among different phenotypes, which might contribute to discrepancies in statistical power. Second, the information available on the summary-level GWAS data had limited us to divide samples into subgroups, which prevented us from studying the age-related heterogeneity.
In conclusion, this was a systematic analysis of the shared etiology and possible causal relations of smoking and CAD by employing large-scale GWASs. Genetic methods represent another option for assessing causality when there are too many confounding factors in randomized controlled traits, and our findings strongly support the hypothesis that smoking behavior is causally related to CAD risk. We found significant genetic overlap and correlations between CAD and smoking at the SNP level. Taken together, the data from this study enhance the understanding of the genetic etiology of the relations between CAD and smoking and might help to dissect smoking behaviors and develop preventive strategies to reduce the burden of cardiovascular disease in public health.
Data availability statement
The original contributions presented in the study are included in the article/Supplementary material, further inquiries can be directed to the corresponding authors.
Author contributions
ZZ: Writing – original draft, Writing – review & editing, Data curation, Formal analysis. QL: Data curation, Formal analysis, Writing – original draft, Methodology. MeL: Data curation, Formal analysis, Methodology, Writing – review & editing. YY: Data curation, Formal analysis, Methodology, Software, Writing – original draft. FQ: Data curation, Formal analysis, Software, Writing – original draft. YX: Data curation, Formal analysis, Methodology, Software, Writing – original draft. SL: Data curation, Formal analysis, Methodology, Writing – review & editing. ZY: Data curation, Project administration, Writing – review & editing. YG: Project administration, Writing – review & editing, Investigation, Resources, Supervision. MiL: Funding acquisition, Investigation, Resources, Writing – review & editing, Conceptualization, Supervision, Writing – original draft. JY: Funding acquisition, Investigation, Project administration, Resources, Writing – review & editing.
Funding
The author(s) declare financial support was received for the research, authorship, and/or publication of this article. This study was supported by the Joint Institute of Tobacco and Health Open Project Fund, the China Precision Medicine Initiative (2016YFC0906300), Research Center for Air Pollution and Health of Zhejiang University, and the State Key Laboratory for Diagnosis and Treatment of Infectious Diseases of the First Affiliated Hospital of Zhejiang University.
Acknowledgments
This research was conducted using GWAS summary data from PGC, GSCAN, and UK Biobank resources. We are deeply grateful to the investigators who comprised the PGC, GSCAN, and UK Biobank research teams and to all the subjects recruited into these projects.
Conflict of interest
The authors declare that the research was conducted in the absence of any commercial or financial relationships that could be construed as a potential conflict of interest.
The author(s) declared that they were an editorial board member of Frontiers, at the time of submission. This had no impact on the peer review process and the final decision.
Publisher’s note
All claims expressed in this article are solely those of the authors and do not necessarily represent those of their affiliated organizations, or those of the publisher, the editors and the reviewers. Any product that may be evaluated in this article, or claim that may be made by its manufacturer, is not guaranteed or endorsed by the publisher.
Supplementary material
The Supplementary material for this article can be found online at: https://www.frontiersin.org/articles/10.3389/fpsyt.2023.1279962/full#supplementary-material
References
1. CDC. Current cigarette smoking among adults–United States. MMWR Morb Mortal Wkly Rep. (2012) 61:889–94.
2. World Health Organization. WHO report on the global tobacco epidemic, 2011: warning about the dangers of tobacco. Geneva, Switzerland: World Health Organization (2011).
3. National Center for Chronic Disease Prevention and Health Promotion (US) Office on Smoking and Health In: The health consequences of Smoking-50 years of progress: a report of the surgeon general. Atlanta, GA: Centers for Disease Control and Prevention (US) (2014).
4. Mons, U, Muezzinler, A, Gellert, C, Schottker, B, Abnet, CC, Bobak, M, et al. Impact of smoking and smoking cessation on cardiovascular events and mortality among older adults: meta-analysis of individual participant data from prospective cohort studies of the CHANCES consortium. BMJ. (2015) 350:h1551. doi: 10.1136/bmj.h1551
5. Doll, R, and Hill, AB. Lung cancer and other causes of death in relation to smoking; a second report on the mortality of British doctors. Br Med J. (1956) 2:1071–81. doi: 10.1136/bmj.2.5001.1071
6. Jonas, MA, Oates, JA, Ockene, JK, and Hennekens, CH. Statement on smoking and cardiovascular disease for health care professionals. Am Heart Assoc Circul. (1992) 86:1664–9. doi: 10.1161/01.cir.86.5.1664
7. Price, JF, Mowbray, PI, Lee, AJ, Rumley, A, Lowe, GD, and Fowkes, FG. Relationship between smoking and cardiovascular risk factors in the development of peripheral arterial disease and coronary artery disease: Edinburgh artery study. Eur Heart J. (1999) 20:344–53. doi: 10.1053/euhj.1998.1194
8. Willett, WC, Green, A, Stampfer, MJ, Speizer, FE, Colditz, GA, Rosner, B, et al. Relative and absolute excess risks of coronary heart disease among women who smoke cigarettes. N Engl J Med. (1987) 317:1303–9. doi: 10.1056/NEJM198711193172102
9. Franklin, WA, Gazdar, AF, Haney, J, Wistuba, II, La Rosa, FG, Kennedy, T, et al. Widely dispersed p53 mutation in respiratory epithelium. A novel mechanism for field carcinogenesis. J Clin Invest. (1997) 100:2133–7. doi: 10.1172/JCI119748
10. Wistuba, II, and Lam, S., Behrens, C., Virmani, A.K., Fong, K.M., LeRiche, J.,, et al. (1997). Molecular damage in the bronchial epithelium of current and former smokers. J Natl Cancer Inst 89, 1366–1373. doi: 10.1093/jnci/89.18.1366
11. Sabater-Lleal, M, Malarstig, A, Folkersen, L, Soler Artigas, M, Baldassarre, D, Kavousi, M, et al. Common genetic determinants of lung function, subclinical atherosclerosis and risk of coronary artery disease. PLoS One. (2014) 9:e104082. doi: 10.1371/journal.pone.0104082
12. Burns, DM. Cigarettes and cigarette smoking. Clin Chest Med. (1991) 12:631–42. doi: 10.1016/S0272-5231(21)00813-3
13. Wang, XL, Greco, M, Sim, AS, Duarte, N, Wang, J, and Wilcken, DE. Effect of CYP1A1 MspI polymorphism on cigarette smoking related coronary artery disease and diabetes. Atherosclerosis. (2002) 162:391–7. doi: 10.1016/s0021-9150(01)00723-7
14. Wang, XL, Sim, AS, Wang, MX, Murrell, GA, Trudinger, B, and Wang, J. Genotype dependent and cigarette specific effects on endothelial nitric oxide synthase gene expression and enzyme activity. FEBS Lett. (2000) 471:45–50. doi: 10.1016/s0014-5793(00)01356-9
15. Smith, GD, and Ebrahim, S. 'Mendelian randomization': can genetic epidemiology contribute to understanding environmental determinants of disease? Int J Epidemiol. (2003) 32:1–22. doi: 10.1093/ije/dyg070
16. Chen, L, Yao, Y, Jin, C, Wu, S, Liu, Q, Li, J, et al. Integrative genomic analysis identified common regulatory networks underlying the correlation between coronary artery disease and plasma lipid levels. BMC Cardiovasc Disord. (2019) 19:310. doi: 10.1186/s12872-019-01271-9
17. Liu, M, Jiang, Y, Wedow, R, Li, Y, Brazel, DM, Chen, F, et al. Association studies of up to 1.2 million individuals yield new insights into the genetic etiology of tobacco and alcohol use. Nat Genet. (2019) 51:237–44. doi: 10.1038/s41588-018-0307-5
18. Willer, CJ, Schmidt, EM, Sengupta, S, Peloso, GM, Gustafsson, S, Kanoni, S, et al. Discovery and refinement of loci associated with lipid levels. Nat Genet. (2013) 45:1274–83. doi: 10.1038/ng.2797
19. Bulik-Sullivan, B, Finucane, HK, Anttila, V, Gusev, A, Day, FR, Loh, PR, et al. An atlas of genetic correlations across human diseases and traits. Nat Genet. (2015) 47:1236–41. doi: 10.1038/ng.3406
20. Hemani, G, Zheng, J, Elsworth, B, Wade, KH, Haberland, V, Baird, D, et al. The MR-base platform supports systematic causal inference across the human phenome. elife. (2018) 7. doi: 10.7554/eLife.34408
21. Purcell, S, Neale, B, Todd-Brown, K, Thomas, L, Ferreira, MA, Bender, D, et al. PLINK: a tool set for whole-genome association and population-based linkage analyses. Am J Hum Genet. (2007) 81:559–75. doi: 10.1086/519795
22. Burgess, S, Butterworth, A, and Thompson, SG. Mendelian randomization analysis with multiple genetic variants using summarized data. Genet Epidemiol. (2013) 37:658–65. doi: 10.1002/gepi.21758
23. Zhang, B, Kirov, S, and Snoddy, J. WebGestalt: an integrated system for exploring gene sets in various biological contexts. Nucleic Acids Res. (2005) 33:W741–8. doi: 10.1093/nar/gki475
24. Chen, S, Yang, F, Xu, T, Wang, Y, Zhang, K, Fu, G, et al. Smoking and coronary artery disease risk in patients with diabetes: a mendelian randomization study. Front Immunol. (2023) 14:891947. doi: 10.3389/fimmu.2023.891947
25. Jia, Y, Wang, R, Guo, D, Sun, L, Shi, M, Zhang, K, et al. Contribution of metabolic risk factors and lifestyle behaviors to cardiovascular disease: a mendelian randomization study. Nutr Metab Cardiovasc Dis. (2022) 32:1972–81. doi: 10.1016/j.numecd.2022.04.019
26. Levin, MG, Klarin, D, Assimes, TL, Freiberg, MS, Ingelsson, E, Lynch, J, et al. Genetics of smoking and risk of atherosclerotic cardiovascular diseases: a mendelian randomization study. JAMA Netw Open. (2021) 4:e2034461. doi: 10.1001/jamanetworkopen.2020.34461
27. Moon, JY, Kwon, HM, Kwon, SW, Yoon, SJ, Kim, JS, Lee, SJ, et al. Lipoprotein(a) and LDL particle size are related to the severity of coronary artery disease. Cardiology. (2007) 108:282–9. doi: 10.1159/000099097
28. van den Berg, VJ, Vroegindewey, MM, Kardys, I, Boersma, E, Haskard, D, Hartley, A, et al. Anti-oxidized LDL antibodies and coronary artery disease: a systematic review. Antioxidants. (2019) 8. doi: 10.3390/antiox8100484
29. Zhao, X, Zhang, HW, Xu, RX, Guo, YL, Zhu, CG, Wu, NQ, et al. Oxidized-LDL is a useful marker for predicting the very early coronary artery disease and cardiovascular outcomes. Per Med. (2018) 15:521–9. doi: 10.2217/pme-2018-0046
30. Heinecke, JW. The HDL proteome: a marker--and perhaps mediator--of coronary artery disease. J Lipid Res. (2009) 50:S167–71. doi: 10.1194/jlr.R800097-JLR200
31. Natarajan, P, Collier, TS, Jin, Z, Lyass, A, Li, Y, Ibrahim, NE, et al. Association of an HDL Apolipoproteomic score with coronary atherosclerosis and cardiovascular death. J Am Coll Cardiol. (2019) 73:2135–45. doi: 10.1016/j.jacc.2019.01.073
32. Weissglas-Volkov, D, and Pajukanta, P. Genetic causes of high and low serum HDL-cholesterol. J Lipid Res. (2010) 51:2032–57. doi: 10.1194/jlr.R004739
33. Grallert, H, Dupuis, J, Bis, JC, Dehghan, A, Barbalic, M, Baumert, J, et al. Eight genetic loci associated with variation in lipoprotein-associated phospholipase A2 mass and activity and coronary heart disease: meta-analysis of genome-wide association studies from five community-based studies. Eur Heart J. (2012) 33:238–51. doi: 10.1093/eurheartj/ehr372
34. Horwitz, T, Lam, K, Chen, Y, Xia, Y, and Liu, C. A decade in psychiatric GWAS research. Mol Psychiatry. (2019) 24:378–89. doi: 10.1038/s41380-018-0055-z
35. Coon, KD, Dunckley, TL, and Stephan, DA. A generic research paradigm for identification and validation of early molecular diagnostics and new therapeutics in common disorders. Mol Diagn Ther. (2007) 11:1–14. doi: 10.1007/BF03256218
36. Herold, C, Hooli, BV, Mullin, K, Liu, T, Roehr, JT, Mattheisen, M, et al. Family-based association analyses of imputed genotypes reveal genome-wide significant association of Alzheimer's disease with OSBPL6, PTPRG, and PDCL3. Mol Psychiatry. (2016) 21:1608–12. doi: 10.1038/mp.2015.218
37. Kamboh, MI, Barmada, MM, Demirci, FY, Minster, RL, Carrasquillo, MM, Pankratz, VS, et al. Genome-wide association analysis of age-at-onset in Alzheimer's disease. Mol Psychiatry. (2012) 17:1340–6. doi: 10.1038/mp.2011.135
38. Kamboh, MI, Demirci, FY, Wang, X, Minster, RL, Carrasquillo, MM, Pankratz, VS, et al. Genome-wide association study of Alzheimer's disease. Transl Psychiatry. (2012) 2:e117. doi: 10.1038/tp.2012.45
39. Li, H, Wetten, S, Li, L, St Jean, PL, Upmanyu, R, Surh, L, et al. Candidate single-nucleotide polymorphisms from a genomewide association study of Alzheimer disease. Arch Neurol. (2008) 65:45–53. doi: 10.1001/archneurol.2007.3
40. Webster, JA, Myers, AJ, Pearson, JV, Craig, DW, Hu-Lince, D, Coon, KD, et al. Sorl1 as an Alzheimer's disease predisposition gene? Neurodegener Dis. (2008) 5:60–4. doi: 10.1159/000110789
Keywords: smoking, CAD, coronary artery disease, genome-wide association study, mendelian randomization analysis
Citation: Zhu Z, Liu Q, Li M, Yao Y, Qi F, Xu Y, Lu S, Yang Z, Guan Y, Li MD and Yao J (2023) Determination of genetic correlation between tobacco smoking and coronary artery disease. Front. Psychiatry. 14:1279962. doi: 10.3389/fpsyt.2023.1279962
Edited by:
Huiping Zhang, Boston University, United StatesReviewed by:
Yunlong Ma, Wenzhou Medical University, ChinaXiangning Chen, University of Texas Health Science Center at Houston, United States
Copyright © 2023 Zhu, Liu, Li, Yao, Qi, Xu, Lu, Yang, Guan, Li and Yao. This is an open-access article distributed under the terms of the Creative Commons Attribution License (CC BY). The use, distribution or reproduction in other forums is permitted, provided the original author(s) and the copyright owner(s) are credited and that the original publication in this journal is cited, in accordance with accepted academic practice. No use, distribution or reproduction is permitted which does not comply with these terms.
*Correspondence: Ming D. Li, bWwya21Aemp1LmVkdS5jbg==; bGltZDU4NkBvdXRsb29rLmNvbQ==; Jianhua Yao, amh5YW9fMjAwN0AxMjYuY29t