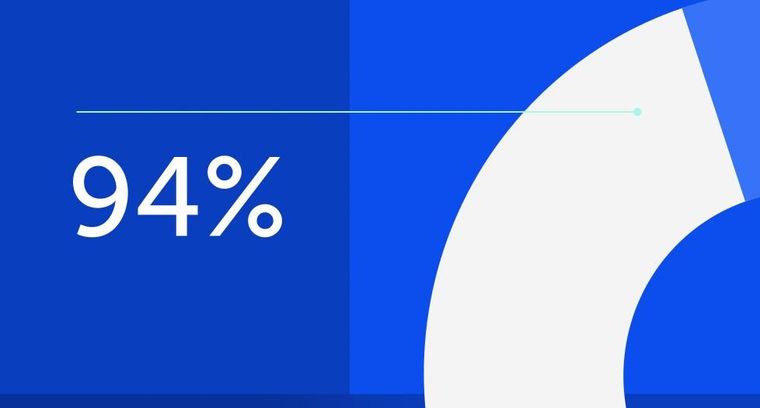
94% of researchers rate our articles as excellent or good
Learn more about the work of our research integrity team to safeguard the quality of each article we publish.
Find out more
ORIGINAL RESEARCH article
Front. Psychiatry, 22 August 2023
Sec. ADHD
Volume 14 - 2023 | https://doi.org/10.3389/fpsyt.2023.1237113
This article is part of the Research TopicDigital Technologies for Screening, Diagnosing, and Treating ADHD and Related DisordersView all 9 articles
Objective: To explore the specific alterations of white matter microstructure in children with attention-deficit/hyperactivity disorder (ADHD) by automated fiber quantification (AFQ) and tract-based spatial statistics (TBSS), and to analyze the correlation between white matter abnormality and impairment of executive function.
Methods: In this prospective study, a total of twenty-seven patients diagnosed with ADHD (20 males, 7 females; mean age of 8.89 ± 1.67 years) and twenty-two healthy control (HC) individuals (11 males, 11 females, mean age of 9.82 ± 2.13 years) were included. All participants were scanned with diffusion kurtosis imaging (DKI) and assessed for executive functions. AFQ and TBSS analysis methods were used to investigate the white matter fiber impairment of ADHD patients, respectively. Axial diffusivity (AD), radial diffusivity (RD), mean diffusivity (MD) and fractional anisotropy (FA) of 17 fiber properties were calculated using the AFQ. The mean kurtosis (MK), axial kurtosis (AK), radial kurtosis (RK), mean diffusivity (MDDKI), axial diffusivity (ADDKI), radial diffusivity (RDDKI) and fractional anisotropy (FADKI) of DKI and AD, RD, MD, and FA of diffusion tensor imaging (DTI) assessed the integrity of the white matter based on TBSS. Partial correlation analyses were conducted to evaluate the correlation between white matter abnormalities and clinical test scores in ADHD while taking age, gender, and education years into account. The analyses were all family-wise error rate (FWE) corrected.
Results: ADHD patients performed worse on the Behavior Rating Inventory of Executive Function (BRIEF) test (p < 0.05). Minor variances existed in gender and age between ADHD and HC, but these variances did not yield statistically significant distinctions. There were no significant differences in TBSS for DKI and DTI parameters (p > 0.05, TFCE-corrected). Compared to HC volunteers, the mean AD value of right cingulum bundle (CB_R) fiber tract showed a significantly higher level in ADHD patients following the correction of FWE. As a result of the point-wise comparison between groups, significant alterations (FWE correction, p < 0.05) were mainly located in AD (nodes 36–38, nodes 83–97) and MD (nodes 92–95) of CB_R. There was no significant correlation between white matter diffusion parameters and clinical test scores in ADHD while taking age, gender, and education years into account.
Conclusion: The AFQ method can detect ADHD white matter abnormalities in a specific location with greater sensitivity, and the CB_R played a critical role. Our findings may be helpful in further studying the relationship between focal white matter abnormalities and ADHD.
The estimated global incidence of attention-deficit/hyperactivity disorder (ADHD) is approximately 6% (1). ADHD is one of the common neurodevelopmental psychiatric disorders in children and adolescents (2). This disorder is characterized by inattention, excessive impulsivity and hyperactivity. The majority of patients who were diagnosed with ADHD in childhood still suffer from persistent, impairing symptoms in adulthood (3).
There is growing evidence that ADHD is associated with abnormal white matter microstructure (4). Following the advances in analytic techniques of diffusion magnetic resonance imaging (MRI), the use of diffusion MRI in ADHD research has contributed significantly to our understanding of the abnormalities in white matter ultrastructure (5).
At present, studies on white matter changes in ADHD are mostly based on diffusion tensor imaging (DTI) (6–8). Several DTI analytical approaches, such as tract-based spatial statistics (TBSS), voxel-based analysis (VBA), and regions of interest (ROI) analysis, have been widely applied to ADHD (9–11). Nevertheless, the inconsistent outcomes of these approaches can be attributed to variations in analytical techniques and the age disparity among subjects, resulting in diminished white matter integrity across diverse regions and extents. TBSS is a skeleton-based approach which can reduce the effects of local misregistration. A TBSS study showed that there was a decrease in FA in the regions of left genu internal capsule and the right forceps major in ADHD children (12). Unlike this, FA reduction was found to be in the cerebellum, anterior thalamic radiation, and corpus callosum genus in VBA studies (13, 14). In the ROI-based method, ADHD children who were not treated exhibited similar FA values to control children (6). There are advantages and drawbacks to each of these DTI analysis techniques.
It is possible that tissue diffusion characteristics vary along each node of the fiber tract, as diseases injury may occur in some local positions of the bundle. Despite the fact that the majority of studies have found that there are white matter injuries in ADHD, it remains unclear whether the specific properties of white matter abnormalities are different along each fiber tract. Thus, the application of a new automated fiber quantification (AFQ) analysis method is particularly important. Using deterministic tractography, AFQ recreates white matter tracts throughout the brain, so more node-detailed information about white matter changes along each fiber tract could be assessed at an individual level (15). Researchers have recently successfully applied AFQ to many cerebral diseases, such as Alzheimer’s disease. (16–18), epilepsy (19), Cushing disease (20), cerebral small vessel disease (4), and end-stage renal disease (21). To our knowledge, there is currently no AFQ research individually specialized for ADHD in children and adolescents.
Furthermore, crossing fibers can be detected much more accurately with diffusion kurtosis imaging (DKI) than with DTI (22–25). A comparative study found that the DKI parameters were more sensitive to detecting white matter abnormalities with coherent and complex arrangements of fibers than DTI (25). While a few DKI studies have been conducted on ADHD in the past (26, 27), comparative studies between DKI and DTI are lacking. Based on the above factors, we have a hypothesis that there are subtle changes in the microstructure of certain local segments of white matter fibers in ADHD patients. In this study, we plan to perform DKI scans on children with ADHD, and compare white matter fiber changes using TBSS (based on DKI and DTI data) and AFQ (based on DTI data) techniques.
The local ethics committee of Taihe Hospital in Shiyan City approved this prospective study. Informed consent forms were signed by all parents of participating children before the study.
Between August 2022 and February 2023, an MRI screening was conducted on a cohort comprising forty-one ADHD patients and thirty-three healthy controls (HC). The healthy controls were recruited through advertising, while the patients with confirmed ADHD were sourced from our outpatient ADHD clinic. A total of fourteen individuals diagnosed with ADHD and eight healthy control volunteers were excluded from the study due to inadequate image quality or noncompliance with the inspection procedures. Three additional volunteers diagnosed with arachnoid cysts were excluded from the HC group. This study finally included twenty-seven patients with ADHD (20 males, 7 females; 8.89 ± 1.67 years) and twenty-two healthy volunteers (11 males, 11 females; 9.82 ± 2.13 years). The clinical diagnostic criteria of ADHD were fulfilled according to the Diagnostic and Statistical Manual of Mental Disorders, 5th edition (DSM-5) and confirmed either by clinical assessment, both the ADHD indices of parent and teacher were ≥ 75th percentile, which was deemed to be positive for ADHD.
All participants were all 6–13 years of age, right-handed, and did not have any contraindications for MRI scanning. Patient exclusion criteria included: (a) psychiatric diseases other than ADHD, such as depression, anxiety, tic disorder, schizophrenia, etc. (b) medical conditions that may affect brain structure or function, such as craniocerebral trauma, intracranial space-occupying lesions, metabolic disease, hereditary diseases, or other disorders of the central nervous system. (c) MRI contraindications. (d) history of regularly consuming drugs. A healthy control group was recruited with similar age, gender, and education level to the ADHD group and excluded the diagnosis of ADHD. Exclusion criteria for HC: (a) psychiatric diseases, such as ADHD, depression, anxiety, tic disorder, schizophrenia, etc. (b) other exclusion criteria were the same as those for ADHD group.
Clinical data including age, gender, and education years were collected from all subjects. The clinical assessments were completed by an associate chief pediatrician who possesses 12 years of professional experience, including Swanson Nolan and pelham-IV rating scale (SNAP-IV) and the Behavior Rating Inventory of Executive Function (BRIEF).
The SNAP-IV evaluation includes an attention defect score and a hyperactivity score. BRIEF containing 86 items was used for children aged 6 and older to determine executive function abilities. There are two components to the questionnaire: a Behavioral Regulation Index (BRI) and a Metacognition Index (MCI). As part of the BRI, the following three clinical scales are described: inhibit, shift, and emotional control. MCI is composed of the initiate, working memory, plan, organization of materials, and monitor. The original scores of each factor were calculated as T scores after age and gender correction.
MRI examinations of all participants were performed on a 3.0-Tesla MRI scanner (Signa Architect, GE Healthcare, Milwaukee, WI, United States) equipped with a 48-channel phased-array head coil. In order to reduce noise and minimize head movement, earplugs were used, as well as tight foam padding.
DKI data were obtained using a single-shot spin echo planar imaging (EPI) sequence with the following parameters: diffusion encoding was applied in 60 directions with two b values (b = 1,000 and 2000 s/mm2) and one non-diffusion weighted image (b = 0 s/mm2), flip angle (FA) = 90o, repetition time (TR) = 8,819 ms, echo time (TE) = 90.4 ms, field of view (FOV) = 240 mm × 240 mm, matrix = 120 × 120, NEX = 1, voxel size = 2 mm × 2 mm × 2 mm, slice gap = 0 mm, 75 axial slices, the total acquisition time was 9 min 7 s. Anatomical 3D gradient-echo T1-weighted images were obtained using a 3D gradient-echo sequence to obtain a high-resolution volume image (FA = 12o, TE = 3.0 ms, TR = 7.4 ms, FOV = 240 mm × 240 mm, matrix = 240 × 240, slice thickness = 1.0 mm without gap, sagittal slices = 166, scan time = 3 min 47 s).
A visual inspection of all images was conducted to ensure that only images without visible artifacts were included in the analysis. DKI digital imaging and communications in medicine (DICOM) images were converted into NIfTI (.nii) format and automatically generated b-vectors and b-values using the dcm2nii tool in MRIcron.1 The Functional MRI of the Brain (FMRIB) Software Library (FSL) was used to correct distortions caused by eddy currents, correct motion artifacts, and strip skulls. DKI parameters including mean kurtosis (MK), axial kurtosis (AK), radial kurtosis (RK), mean diffusivity (MDDKI), axial diffusivity (ADDKI), radial diffusivity (RDDKI), and fractional anisotropy (FADKI) were calculated using the diffusional kurtosis estimator (DKE). Furthermore, images with b = 0 and 1,000 s/mm2 were extracted to calculate parameters [mean diffusivity (MD), axial diffusivity (DA), radial diffusivity (DR), and fractional anisotropy (FA)] of the DTI model by using FSL software. The dcm2nii tool in MRIcron was also used to convert 3D T1WI DICOM images into NIfTI format, then the script mrAnatAverageAcpcNifti and dtiMakeDt6FromFsl were used to align AC (anterior commissure) -PC (posterior commissure) and obtain a dt6 MATLAB format file.
The diffusion tensor imaging data underwent processing with the Functional MRI of the Brain (FMRIB) Software Library (FSL) version 5.0.9.2 To address eddy current-induced distortions and motion artifacts, each diffusion-weighted image was aligned to the non-diffusion weighted volume (b0 image) through affine alignment, employing the FDT-FMRIB’S Diffusion Toolbox 3.0 tool. The BET-Brain Extraction Tool-v2.1, a component of the FSL package, was utilized to extract a brain mask from the eddy corrected image in order to remove the skull and non-brain tissue. A threshold of 0.2 was set for this purpose. Following this, the DTIFIT tool was employed to estimate the diffusion tensor at each voxel, resulting in the creation of FA, MD, and eigenvalue (λ1, λ2, λ3) maps. The mv and fslmaths commands were subsequently used to generate the parameter graphs for AD and RD, respectively, based on these eigenvalues. The TBSS toolbox in FSL was used for voxel-wise statistical analysis of the FA maps. FA images of all subjects were transformed into Montreal Neurological Institute (MNI) space. An image of the mean FA was generated, then the skeletonized image was used to create a white matter tract skeleton, a FA threshold of 0.20 was used for skeletonization to avoid gray matter and cerebrospinal fluid interference, as well as intersubject variability. We projected aligned FA images of all subjects onto the skeleton. We then applied the same FA transformation to MK, AK, RK, MDDKI, ADDKI, RDDKI, FADKI, MD, AD, and RD images for statistical analysis. To assess the differences in voxel-wise FA, MK, AK, RK, MDDKI, ADDKI, RDDKI, FADKI, MD, AD, and RD between the two groups, the individual skeleton images were subjected to a general linear model (GLM) analysis. Age, sex, and years of education were included as covariates in the analysis. Nonparametric permutation-based testing was conducted using the randomize function in FSL.3 The results were considered significant at a p < 0.05 level after 5,000 permutations, employing permutation-based non-parametric inference. Threshold-free cluster enhancement (TFCE) and family-wise error (FWE) rate correction were applied to account for multiple comparisons.
In this study, we identified twenty major fiber tracts of the whole brain and applied AFQ software4 to quantify diffusion metrics along these tracts. There are five primary steps in the entire AFQ process: (1) tracking whole-brain fibers with deterministic algorithms. (2) segmentation of fibers based on waypoint region of interest (ROI). (3) refinement of fibers based on probability maps. (4) using the outlier rejection algorithm to clean fiber tracts. (5) measurement of diffusion metrics (FA, MD, AD, RD) at 100 equidistant fiber nodes along each fiber tract. As a result of the threshold setting utilized in fiber tracking, three specific fiber tracts, namely the bilateral cingulum hippocampus (CH_L, CH_R) and right arcuate fasciculus (AF_R), were excluded from further analysis due to their inability to be identified in a significant proportion of participants. This inability to track certain fibers may be attributed to their close proximity to gray matter. For further analysis, we focused on the remaining 17 fiber tracts identified. Following are the 17 identified fiber tracts: bilateral anterior thalamic radiation (ATR_L, ATR_R), corticospinal tract (CST_L, CST_R), cingulum bundle (CB_L, CB_R), splenium of the corpus callosum (CC_S), genu of the corpus callosum (CC_G), inferior fronto-occipital fasciculus (IFOF_L, IFOF_R), inferior longitudinal fasciculus (ILF_L, ILF_R), superior longitudinal fasciculus (SLF_L, SLF_R), uncinate fasciculus (UF_L, UF_R) and left arcuate fasciculus (AF_L). Figure 1 shows the fully identified 17 fiber tracts.
Figure 1. Tracking results from seventeen fiber tract identification. A red region of interest (ROI) represents a starting waypoint, while a blue ROI represents an ending waypoint.
The statistical package for social science (SPSS) version 25.0 was used to analyze the clinical data and the mean values of DTI parameters (AFQ) for each fiber tract between ADHD and HC group. Comparing normally and non-normally distributed variables was carried out using independent samples t-tests or Mann–Whitney U-tests. Statistical tests were conducted on enumeration data using Chi-squared or Fisher’s exact tests.
In the statistical analysis conducted using the AFQ method, the average values of DTI parameters (FA, MD, AD, RD) of each fiber tract in the two groups were compared, and multiple comparisons were corrected by FWE correction (p < 0.05/68). A point-wise analysis was performed using the “Randomize” command in FSL, as covariates in the GLM model, gender, age, and education years were controlled. Based on 5,000 permutations, a non-parametric permutation-based statistical analysis with FWE (p < 0.05) was performed on 1,700 points. As a result, we only reported the results of ≥3 adjacent nodes among those with significant differences. Partial correlation analyses were conducted to determine if the microstructural integrity of white matter correlated with clinical test scores while taking age, gender, and education years into account.
Statistical results for demographic and clinical characteristics can be found in Table 1. The groups did not differ significantly in terms of gender, age, and education years (p > 0.05). Compared with HC volunteers, ADHD patients performed worse on concentration tests and the BRIEF test and showed significant impairments in inhibit, shift, emotional control, initiate, working memory, plan, organization of materials, and monitor (p < 0.05).
There were no significant differences in tract-based spatial statistics for MK, AK, RK, MDDKI, ADDKI, RDDKI, FADKI, FA, MD, AD, and RD (P-FWE > 0.05, TFCE-corrected).
The mean value of each fiber tracts for FA, MD, AD, and RD were presented in Tables 2–5. Compared to HC, the mean AD value of the CB_R fiber tract showed a significant increase in ADHD patients following the correction of FWE (Table 4; Figure 2).
Figure 2. The mean axial diffusivity (AD) value of the right cingulum bundle showed a significant increase in attention-deficit hyperactivity disorder (ADHD) patients following the correction of family-wise error rate.
The mean AD values for the 16 remaining fiber tracts, as well as the mean FA, MD, and RD values for the 17 fiber tracts, all showed no significant differences after FWE correction (Tables 2–5).
In order to show detailed diffusion properties of tracts, pointwise comparisons between ADHD patients and HC volunteers were quantified based on AFQ software, white matter disruption is found in specific parts along the white matter tract in ADHD patients. As a result of the point-wise comparison between groups, significant alterations (FWE correction, p < 0.05) were mainly located in AD (nodes 36–38, nodes 83–97) and MD (nodes 92–95) of CB_R, details about the significant differences are shown in Figures 3, 4.
Figure 3. Line chart of the right cingulum bundle with significantly altered in point-wise comparisons of axial diffusivity (AD) and mean diffusivity (MD) values between attention-deficit hyperactivity disorder (ADHD) and healthy control (HC; p < 0.05, family-wise error rate correction). ADHD is represented by the blue line, while HC is represented by the yellow line; (the solid lines represent the means and the dotted lines represent the 95% confidence intervals). At the bottom of the graph are the red bars that represent fiber segments with significant differences between groups.
Figure 4. Violin chart showing the Significant results of the point-wise comparison between attention-deficit hyperactivity disorder (ADHD) and healthy control (HC) groups. Significant alterations (family-wise error rate correction, p < 0.05) were mainly located in axial diffusivity (AD; nodes 36–38, nodes 83–97) and mean diffusivity (MD; nodes 92–95) of right cingulum bundle.
For the altered AD (nodes 36–38, nodes 83–97) and MD (nodes 92–95) of CB_R, there was no significant correlation between white matter diffusion parameters and clinical test scores in ADHD while taking age, gender, and education years into account.
ADHD patients exhibit specific subcortical dysfunction, with prevailing belief that subcortical abnormalities play a role in the onset of ADHD, while cortical plasticity influences the diverse clinical trajectory of the disorder (28). The thalamus, as the largest subcortical structure in the brain, assumes significance as a crucial element within the cortico striato thalamo cortical circuit (29, 30). Dysfunction within this circuit has been recognized as a significant neural correlate of ADHD (31). Additionally, it is worth noting that the ventral and intralaminar thalamus have been identified as potential components of the somatomotor network (32, 33), and they exhibit connectivity with the anterior midcingulate cortex (34, 35). It is plausible that dysfunctions within these interconnected circuits or subcortical structures might play a role in the manifestation of clinical symptoms associated with attention deficit hyperactivity disorder (ADHD). Additional investigation into ADHD could contribute to a comprehensive comprehension of the disorder. This study exclusively focused on the alterations observed in diffusion imaging, thus necessitating future extensive examinations of ADHD from a multimodal standpoint (36–38).
This study examines the precise modifications in white matter microstructure in children with ADHD through the utilization of AFQ and TBSS. While previous neuroimaging investigations of ADHD have evaluated the integrity of the white matter, the TBSS study outcomes based on voxels did not survive following multiple comparison corrections in this particular study of children. One plausible explanation is that TBSS may be better suited for detecting significant white matter abnormalities (39). For instance, it exhibits sensitivity towards long-term exposure-induced structural alterations (40) or structural changes linked to severe injuries like Traumatic Brain Injury (41). However, the focus of the research cohort pertained predominantly to children who had recently received a diagnosis of ADHD. These individuals are of a tender age and presently in the nascent phases of the disorder, potentially exhibiting alterations solely at a microscopic scale in the white matter fiber bundles. Another possible explanation for the observed outcome could be attributed to the limited scope of the investigation. However, the AFQ analysis revealed the presence of abnormalities in the CB_R, which were more effectively detected by the AFQ method as compared to TBSS. The AD and MD values of CB_R exhibited significant increases at the node-wise level, while only AD values of CB_R showed a significant increase in the entire fiber tract, as per the AFQ study. These findings suggest that AFQ may serve as a complementary method to TBSS, offering a novel approach and providing new insights into ADHD. Notably, node-wise analysis is crucial as it can reveal local anomalies that may be obscured by the whole. Previous research has demonstrated an increase in AD in the right cingulum bundle (42). Our study similarly found a significant increase in the mean AD value of the CB_R fiber tract in individuals with ADHD. AD reflects diffusion coefficients parallel to fiber orientation and is known to be sensitive to axonal damage (43). Therefore, our findings suggest that the observed microstructure changes in the CB_R of individuals with ADHD may be primarily attributed to axonal damage.
The cingulum bundle is a white matter pathway that extends longitudinally from the anterior cingulate cortex (ACC) to the posterior cingulate cortex (PCC), linking the components of the default mode network (DMN) and providing important limbic connections, including those to supplementary motor and primary motor regions (44). This large-scale white matter system (45) is responsible for processing attention and controlling executive functions (46). An amygdala, hippocampus, and insular cortex receive projections from it (47).
There exists a substantial body of evidence indicating that ADHD is linked to deficiencies in various neurocognitive domains. Neuropsychological theories posit that ADHD is chiefly marked by executive dysfunction (48) and anomalous function of the default mode network (DMN) (49). The cingulum bundle is implicated in both executive dysfunction and the DMN theory of ADHD, as supported by these two theories. First, previous studies have shown that executive function processes play a critical role in ADHD (48, 50–52). In executive functioning, the cingulum bundle plays a key role. The cingulum bundle is strongly connected to the cingulate gyrus, which is associated with executive function (53, 54). Second, DMN nodes are connected by various white matter tracts, including anterior and posterior cingulum bundles. Previous studies have demonstrated a correlation between reduced functional connectivity of the cingulum bundle and ADHD. Specifically, researchers using rs-fMRI found that functional connectivity in the DMN and between the dorsal anterior cingulate and posterior region of the DMN was decreased in ADHD adults (55). Additionally, a reduction in brain connectivity has been observed in the anterior cingulate and temporal regions of the brain, which are associated with inattention (56).
At a point-wise level, the AFQ technique enables the measurement of diffusion at 100 equidistant nodes along the fiber tract. In contrast to prior methodologies, this approach affords a more precise analysis of tracts, yielding more accurate identification of damage segments and greater sensitivity to abnormalities in other indicators. The present investigation revealed significant alterations primarily in the AD (nodes 36–38, nodes 83–97) and MD (nodes 92–95) of CB_R. The findings of our study suggest that ADHD patients exhibit injury predominantly in the anterior and posterior regions of the CB_R fiber tract, which is manifested by elevated AD and MD values. Prior research has demonstrated that changes in AD and MD may be indicative of axonal damage (57) and myelin degradation (58). An elevated AD in the CC_R is significantly associated with a decrease in neuronal branching (59). Additionally, the MD metric reflects the diffusion rates of water throughout the brain, and an increase in MD values is indicative of a loss of axons, dendrites, and neuronal cell bodies, ultimately leading to structural alterations at the macroscopic level (60). There is evidence that a reduction in axonal density is linked to heightened MD values (61). Collectively, these findings suggest that the observed microstructural changes in the CB_R are primarily attributed to damage to myelin and axons. Previous research has indicated that the anterior portion of the cingulum bundles plays a crucial role in facilitating communication between limbic regions (62). Furthermore, lesions in this area have been shown to disrupt performance on the 5-Choice Serial Reaction Time Task, which is closely linked to cholinergic activity (63). Given that cholinergic activity is known to be associated with attention, these findings suggest that the anterior cingulum bundles may be involved in attentional processes. Additionally, a recent study has suggested that ADHD may be associated with alterations in the functional connectivity of the right posterior cingulate (64), which may indicate damage to the white matter microstructure of ADHD patients. Drawing upon extant research findings, our investigation uncovered significant alterations in the AD and MD values of CB_R in specific regions, suggesting that increased AD and MD of CB_R may represent early-phase abnormalities in ADHD. Our conjecture is that CB_R plays a pivotal role in the initial stages of ADHD, particularly in its anterior and posterior components. By means of AFQ analyses, more precise localization data can be ascertained regarding damaged fiber segments.
Furthermore, our study revealed no statistically significant alterations in FA along fiber tracts. Our findings are consistent with previous research, which has demonstrated that there are no significant differences in FA within the white matter structure of the cingulum tract between individuals with ADHD and healthy controls (65–68). However, prior investigations have produced inconsistent results, with some diffusion studies indicating reduced FA in the right cingulum bundle among individuals with ADHD in comparison to healthy controls (69, 70). The inconsistency of studies may be attributed to the ADHD subtype or study age range, as well as the potential influence of varying research methods and subject ages. Amlien’s review demonstrated that an increase in AD led to a reduction in FA differences between groups (71). It is plausible that a significant increase in AD may have impacted the FA results in our study, necessitating a larger sample size and replication of the study.
The results of our study indicate that the altered white matter parameters observed in individuals with ADHD were not found to be significantly correlated with clinical test scores. However, the ADHD group exhibited significantly higher scores in attention deficit and hyperactivity, as well as in the areas of inhibition, shifting, emotional control, initiation, working memory, planning, organization of materials, and monitoring, thereby confirming the presence of executive function deficits in this population.
An AFQ analysis of adults with ADHD found that there were no differences between ADHD and HC, and the ADHD group showed no significant association (72), this correlation result is consistent with our findings. Future research is needed to explore the relationship between changes in white matter microstructure and executive function.
To the best of our knowledge, this investigation represents a pioneering effort in scrutinizing the precise locations of individual fiber tracts in children with ADHD utilizing the AFQ. Nonetheless, it is imperative to acknowledge certain constraints of this study despite its merits. Firstly, our prospective inquiry was executed on a modest sample size, and additional research is necessary on a more comprehensive sample. Secondly, there was no categorization for ADHD, and future investigations will necessitate a thorough analysis of diverse subtypes of data. Thirdly, the present study was limited by the absence of longitudinal research as all patients were diagnosed during their initial visit. Future studies should investigate the impact of disease progression on white matter microstructure. Fourth, certain fibers, including CH_L, CH_R, and AF_R, were not trackable due to threshold settings in fiber tracking. Fifth, the correlation analyses indicated that the altered white matter parameters did not exhibit a significant correlation with clinical test scores in ADHD, possibly due to the small sample size and the effect of mixing multiple subtypes.
According to the AFQ method, capable of detecting mild changes in white matter, we identified white matter abnormalities in fiber tracts with a greater degree of spatial precision. As a surveillance tool for ADHD, the AFQ method can detect ADHD-related white matter abnormalities with greater sensitivity, and the CB_R played a critical role in furthering our understanding of ADHD-related white matter abnormalities. Our findings may be helpful in further studying the relationship between focal white matter abnormalities and ADHD.
The raw data supporting the conclusions of this article will be made available by the authors, without undue reservation.
The studies involving humans were approved by Taihe Hospital, Hubei University of Medicine, Shiyan, Hubei. The studies were conducted in accordance with the local legislation and institutional requirements. Written informed consent for participation in this study was provided by the participants’ legal guardians/next of kin.
RH and FT: study design, data collection and curation, image scanning and post-processing, formal analysis, visualization, and writing-original draft. WC, YW, YJ, WD, YZ, and BG: case collection, investigation, data curation, image post-processing, software application, formal analysis. QS: scanning specification, technical guidance, supervision. YM: conceptualization, study design, funding acquisition, supervision, and writing – review and editing. All authors contributed to the article and approved the submitted version.
The authors declare that the research was conducted in the absence of any commercial or financial relationships that could be construed as a potential conflict of interest.
All claims expressed in this article are solely those of the authors and do not necessarily represent those of their affiliated organizations, or those of the publisher, the editors and the reviewers. Any product that may be evaluated in this article, or claim that may be made by its manufacturer, is not guaranteed or endorsed by the publisher.
The Supplementary material for this article can be found online at: https://www.frontiersin.org/articles/10.3389/fpsyt.2023.1237113/full#supplementary-material
1. ^https://www.nitrc.org/projects/mricron
2. ^https://fsl.fmrib.ox.ac.uk/fsl/
3. ^https://fsl.fmrib.ox.ac.uk/fsl/fslwiki/Randomise/UserGuide
1. Zhou, YX. Integrative Imaging in Neuroplasticity, Wisdom and Neuropsychiatry: Science Meets Arts (Smart). United Kingdom: Ethics International Press (2023).
2. Chang, X, Liu, Y, Mentch, F, Glessner, J, Qu, H, Nguyen, K, et al. Mitochondrial DNA Haplogroups and risk of attention deficit and hyperactivity disorder in European Americans. Transl Psychiatry. (2020) 10:370. doi: 10.1038/S41398-020-01064-1
3. Okazaki, K, Ota, T, Makinodan, M, Kishimoto, N, Yamamuro, K, Ishida, R, et al. Associations of childhood experiences with event-related potentials in adults with autism Spectrum disorder. Sci Rep. (2020) 10:13447. doi: 10.1038/S41598-020-70409-Z
4. Connaughton, M, Whelan, R, O'hanlon, E, and Mcgrath, J. White matter microstructure in 0children and adolescents with ADHD. Neuroimage Clin. (2022) 33:102957. doi: 10.1016/J.Nicl.2022.102957
5. Bush, G. Attention-deficit/hyperactivity disorder and attention networks. Neuropsychopharmacology. (2010) 35:278–300. doi: 10.1038/Npp.2009.120
6. Bouziane, C, Caan, MWA, Tamminga, HGH, Schrantee, A, Bottelier, MA, De Ruiter, MB, et al. ADHD and maturation of brain white matter: a DTI study in medication naive children and adults. Neuroimage Clin. (2018) 17:53–9. doi: 10.1016/J.Nicl.2017.09.026
7. Ercan, ES, Suren, S, Bacanli, A, Yazici, KU, Calli, C, Ardic, UA, et al. Altered structural connectivity is related to attention deficit/hyperactivity subtypes: a DTI study. Psychiatry Res Neuroimaging. (2016) 256:57–64. doi: 10.1016/J.Pscychresns.2016.04.002
8. Van Ewijk, H, Heslenfeld, DJ, Zwiers, MP, Buitelaar, JK, and Oosterlaan, J. Diffusion tensor imaging in attention deficit/hyperactivity disorder: a systematic review and meta-analysis. Neurosci Biobehav Rev. (2012) 36:1093–106. doi: 10.1016/J.Neubiorev.2012.01.003
9. Aoki, Y, Yoncheva, YN, Chen, B, Nath, T, Sharp, D, Lazar, M, et al. Association of white matter structure with autism Spectrum disorder and attention-deficit/hyperactivity disorder. JAMA Psychiat. (2017) 74:1120–8. doi: 10.1001/Jamapsychiatry.2017.2573
10. Kessler, D, Angstadt, M, Welsh, RC, and Sripada, C. Modality-spanning deficits in attention-deficit/hyperactivity disorder in functional networks, gray matter, and white matter. J Neurosci. (2014) 34:16555–66. doi: 10.1523/Jneurosci.3156-14.2014
11. Ulke, C, Rullmann, M, Huang, J, Luthardt, J, Becker, GA, Patt, M, et al. Adult attention-deficit/hyperactivity disorder is associated with reduced norepinephrine transporter availability in right attention networks: a (S,S)-O-[(11)C]methylreboxetine positron emission tomography study. Transl Psychiatry. (2019) 9:301. doi: 10.1038/S41398-019-0619-Y
12. Zhou, R, Dong, P, Chen, S, Qian, A, Tao, J, Zheng, X, et al. The long-range white matter microstructural alterations in drug-naive children with ADHD: a tract-based spatial statistics study. Psychiatry Res Neuroimaging. (2022) 327:111548. doi: 10.1016/J.Pscychresns.2022.111548
13. Ameis, SH, Lerch, JP, Taylor, MJ, Lee, W, Viviano, JD, Pipitone, J, et al. A diffusion tensor imaging study in children with ADHD, autism Spectrum disorder, OCD, and matched controls: distinct and non-distinct white matter disruption and dimensional brain-behavior relationships. Am J Psychiatry. (2016) 173:1213–22. doi: 10.1176/Appi.Ajp.2016.15111435
14. Ashtari, M, Kumra, S, Bhaskar, SL, Clarke, T, Thaden, E, Cervellione, KL, et al. Attention-deficit/hyperactivity disorder: a preliminary diffusion tensor imaging study. Biol Psychiatry. (2005) 57:448–55. doi: 10.1016/J.Biopsych.2004.11.047
15. Yeatman, JD, Dougherty, RF, Myall, NJ, Wandell, BA, and Feldman, HM. Tract profiles of white matter properties: automating Fiber-tract quantification. PLoS One. (2012) 7:E49790. doi: 10.1371/Journal.Pone.0049790
16. Chen, H, Sheng, X, Qin, R, Luo, C, Li, M, Liu, R, et al. Aberrant white matter microstructure as a potential diagnostic marker in Alzheimer's disease by automated Fiber quantification. Front Neurosci. (2020) 14:570123. doi: 10.3389/Fnins.2020.570123
17. Dou, X, Yao, H, Feng, F, Wang, P, Zhou, B, Jin, D, et al. Characterizing white matter connectivity in Alzheimer's disease and mild cognitive impairment: an automated Fiber quantification analysis with two independent datasets. Cortex. (2020) 129:390–405. doi: 10.1016/J.Cortex.2020.03.032
18. Zhang, X, Sun, Y, Li, W, Liu, B, Wu, W, Zhao, H, et al. Characterization of white matter changes along fibers by automated Fiber quantification in the early stages of Alzheimer's disease. Neuroimage Clin. (2019) 22:101723. doi: 10.1016/J.Nicl.2019.101723
19. Shu, M, Yu, C, Shi, Q, Li, Y, Niu, K, Zhang, S, et al. Alterations in white matter integrity and asymmetry in patients with benign childhood epilepsy with Centrotemporal spikes and childhood absence epilepsy: an automated fiber quantification tractography study. Epilepsy Behav. (2021) 123:108235. doi: 10.1016/J.Yebeh.2021.108235
20. Cui, M, Zhou, T, Feng, S, Liu, X, Wang, F, Zhang, Y, et al. Altered microstructural pattern of white matter in Cushing's disease identified by automated Fiber quantification. Neuroimage Clin. (2021) 31:102770. doi: 10.1016/J.Nicl.2021.102770
21. Jiang, Y, Liu, Y, Gao, B, Che, Y, Lin, L, Jiang, J, et al. Segmental abnormalities of white matter microstructure in end-stage renal disease patients: an automated Fiber quantification Tractography study. Front Neurosci. (2021) 15:765677. doi: 10.3389/Fnins.2021.765677
22. Glenn, GR, Helpern, JA, Tabesh, A, and Jensen, JH. Optimization of white matter fiber tractography with diffusional kurtosis imaging. NMR Biomed. (2015) 28:1245–56. doi: 10.1002/Nbm.3374
23. Glenn, GR, Kuo, LW, Chao, YP, Lee, CY, Helpern, JA, and Jensen, JH. Mapping the orientation of white matter fiber bundles: a comparative study of diffusion tensor imaging, diffusional kurtosis imaging, and diffusion spectrum imaging. AJNR Am J Neuroradiol. (2016) 37:1216–22. doi: 10.3174/Ajnr.A4714
24. Jensen, JH, Helpern, JA, and Tabesh, A. Leading non-Gaussian corrections for diffusion orientation distribution function. NMR Biomed. (2014) 27:202–11. doi: 10.1002/nbm.3053
25. Zhu, J, Zhuo, C, Qin, W, Wang, D, Ma, X, Zhou, Y, et al. Performances of diffusion kurtosis imaging and diffusion tensor imaging in detecting white matter abnormality in schizophrenia. Neuroimage Clin. (2015) 7:170–6. doi: 10.1016/J.Nicl.2014.12.008
26. Adisetiyo, V, Tabesh, A, Di Martino, A, Falangola, MF, Castellanos, FX, Jensen, JH, et al. Attention-deficit/hyperactivity disorder without comorbidity is associated with distinct atypical patterns of cerebral microstructural development. Hum Brain Mapp. (2014) 35:2148–62. doi: 10.1002/Hbm.22317
27. Helpern, JA, Adisetiyo, V, Falangola, MF, Hu, C, Di Martino, A, Williams, K, et al. Preliminary evidence of altered gray and white matter microstructural development in the frontal lobe of adolescents with attention-deficit hyperactivity disorder: a diffusional kurtosis imaging study. J Magn Reson Imaging. (2011) 33:17–23. doi: 10.1002/Jmri.22397
28. Shaw, P, and Sudre, G. Adolescent attention-deficit/hyperactivity disorder: understanding teenage symptom trajectories. Biol Psychiatry. (2021) 89:152–61. doi: 10.1016/J.Biopsych.2020.06.004
29. Gunther, V, Pecher, J, Webelhorst, C, Bodenschatz, CM, Mucha, S, Kersting, A, et al. Non-conscious processing of fear faces: a function of the implicit self-concept of anxiety. BMC Neurosci. (2023) 24:12. doi: 10.1186/S12868-023-00781-9
30. Wang, Z, Liu, Y, Ruan, X, Li, Y, Li, E, Zhang, G, et al. Aberrant amplitude of low-frequency fluctuations in different frequency bands in patients with Parkinson's disease. Front Aging Neurosci. (2020) 12:576682. doi: 10.3389/Fnagi.2020.576682
31. Posner, J, Park, C, and Wang, Z. Connecting the dots: a review of resting connectivity MRI studies in attention-deficit/hyperactivity disorder. Neuropsychol Rev. (2014) 24:3–15. doi: 10.1007/S11065-014-9251-Z
32. Contreras, A, Khumnark, M, Hines, RM, and Hines, DJ. Behavioral arrest and a characteristic slow waveform are Hallmark responses to selective 5-Ht(2a) receptor activation. Sci Rep. (2021) 11:1925. doi: 10.1038/S41598-021-81552-6
33. Hong, SB. Thalamocortical functional connectivity in youth with attention-deficit/hyperactivity disorder. J Psychiatry Neurosci. (2023) 48:E50–60. doi: 10.1503/Jpn.220109
34. Horakova, A, Nemcova, H, Mohr, P, and Sebela, A. Structural, functional, and metabolic signatures of postpartum depression: a systematic review. Front Psych. (2022) 13:1044995. doi: 10.3389/Fpsyt.2022.1044995
35. Zhu, X, Ward, J, Cullen, B, Lyall, DM, Strawbridge, RJ, Lyall, LM, et al. Phenotypic and genetic associations between anhedonia and brain structure in UK biobank. Transl Psychiatry. (2021) 11:395. doi: 10.1038/S41398-021-01522-4
36. Bu, X, Cao, M, Huang, XQ, and He, Y. The structural connectome in ADHD. Psychoradiology. (2021) 1:257–71. doi: 10.1093/Psyrad/Kkab021
37. Bu, X, Gao, YX, Liang, KL, Chen, Y, Guo, LT, and Huang, XQ. Investigation of white matter functional networks underlying different behavioral profiles in attention-deficit/hyperactivity disorder. Psychoradiology. (2022) 2:69–77. doi: 10.1093/Psyrad/Kkac012
38. Zhou, YX. Multiparametric Imaging in Neurodegenerative Disease. New York: Nova Science Publishers (2019).
39. Focke, NK, Yogarajah, M, Bonelli, SB, Bartlett, PA, Symms, MR, and Duncan, JS. Voxel-based diffusion tensor imaging in patients with mesial temporal lobe epilepsy and hippocampal sclerosis. NeuroImage. (2008) 40:728–37. doi: 10.1016/J.Neuroimage.2007.12.031
40. Jiang, Y, Gao, Q, Liu, Y, Gao, B, Che, Y, Lin, L, et al. Reduced white matter integrity in patients with end-stage and non-end-stage chronic kidney disease: a tract-based spatial statistics study. Front Hum Neurosci. (2021) 15:774236. doi: 10.3389/Fnhum.2021.774236
41. Cho, MJ, and Jang, SH. Relationship between post-traumatic amnesia and white matter integrity in traumatic brain injury using tract-based spatial statistics. Sci Rep. (2021) 11:6898. doi: 10.1038/S41598-021-86439-0
42. Svatkova, A, Nestrasil, I, Rudser, K, Goldenring Fine, J, Bledsoe, J, and Semrud-Clikeman, M. Unique white matter microstructural patterns in ADHD presentations-a diffusion tensor imaging study. Hum Brain Mapp. (2016) 37:3323–36. doi: 10.1002/Hbm.23243
43. Bennett, IJ, and Madden, DJ. Disconnected aging: cerebral white matter integrity and age-related differences in cognition. Neuroscience. (2014) 276:187–205. doi: 10.1016/J.Neuroscience.2013.11.026
44. Venkatasubramanian, PN, Keni, P, Gastfield, R, Li, L, Aksenov, D, Sherman, SA, et al. Diffusion tensor imaging detects acute and subacute changes in Corpus callosum in blast-induced traumatic brain injury. ASN Neuro. (2020) 12:1759091420922929. doi: 10.1177/1759091420922929
45. Libero, LE, Burge, WK, Deshpande, HD, Pestilli, F, and Kana, RK. White matter diffusion of major Fiber tracts implicated in autism Spectrum disorder. Brain Connect. (2016) 6:691–9. doi: 10.1089/Brain.2016.0442
46. Makris, N, Buka, SL, Biederman, J, Papadimitriou, GM, Hodge, SM, Valera, EM, et al. Attention and executive systems abnormalities in adults with childhood ADHD: a DT-MRI study of connections. Cereb Cortex. (2008) 18:1210–20. doi: 10.1093/Cercor/Bhm156
47. Lee, MH, Sin, S, Lee, S, Park, H, Wagshul, ME, Zimmerman, ME, et al. Altered cortical structure network in children with obstructive sleep apnea. Sleep. (2022) 45:Zsac030. doi: 10.1093/Sleep/Zsac030
48. Barkley, RA. Behavioral inhibition, sustained attention, and executive functions: constructing a unifying theory of Adhd. Psychol Bull. (1997) 121:65–94. doi: 10.1037/0033-2909.121.1.65
49. Sonuga-Barke, EJ, and Castellanos, FX. Spontaneous attentional fluctuations in impaired states and pathological conditions: a neurobiological hypothesis. Neurosci Biobehav Rev. (2007) 31:977–86. doi: 10.1016/J.Neubiorev.2007.02.005
50. McCandless, S, and O’Laughlin, L. The clinical utility of the behavior rating inventory of executive function (Brief) in the diagnosis of ADHD. J Atten Disord. (2007) 10:381–9. doi: 10.1177/1087054706292115
51. Silverstein, MJ, Faraone, SV, Leon, TL, Biederman, J, Spencer, TJ, and Adler, LA. The relationship between executive function deficits and DSM-5-defined ADHD symptoms. J Atten Disord. (2020) 24:41–51. doi: 10.1177/1087054718804347
52. Steward, KA, Tan, A, Delgaty, L, Gonzales, MM, and Bunner, M. Self-awareness of executive functioning deficits in adolescents with ADHD. J Atten Disord. (2017) 21:316–22. doi: 10.1177/1087054714530782
53. Bubb, EJ, Metzler-Baddeley, C, and Aggleton, JP. The cingulum bundle: anatomy, function, and dysfunction. Neurosci Biobehav Rev. (2018) 92:104–27. doi: 10.1016/J.Neubiorev.2018.05.008
54. Schermuly, I, Fellgiebel, A, Wagner, S, Yakushev, I, Stoeter, P, Schmitt, R, et al. Association between cingulum bundle structure and cognitive performance: an observational study in major depression. Eur Psychiatry. (2010) 25:355–60. doi: 10.1016/J.Eurpsy.2010.05.001
55. Castellanos, FX, Margulies, DS, Kelly, C, Uddin, LQ, Ghaffari, M, Kirsch, A, et al. Cingulate-Precuneus interactions: a new locus of dysfunction in adult attention-deficit/hyperactivity disorder. Biol Psychiatry. (2008) 63:332–7. doi: 10.1016/J.Biopsych.2007.06.025
56. Hong, SB, Zalesky, A, Fornito, A, Park, S, Yang, YH, Park, MH, et al. Connectomic disturbances in attention-deficit/hyperactivity disorder: a whole-brain Tractography analysis. Biol Psychiatry. (2014) 76:656–63. doi: 10.1016/J.Biopsych.2013.12.013
57. Imagawa, KK, Hamilton, A, Ceschin, R, Tokar, E, Pham, P, Bluml, S, et al. Characterization of microstructural injury: a novel approach in infant abusive head trauma-initial experience. J Neurotrauma. (2014) 31:1632–8. doi: 10.1089/Neu.2013.3228
58. Tievsky, AL, Ptak, T, and Farkas, J. Investigation of apparent diffusion coefficient and diffusion tensor anisotrophy in acute and chronic multiple sclerosis lesions. Am J Neuroradiol. (1999) 20:1491–9.
59. Silk, TJ, Vance, A, Rinehart, N, Bradshaw, JL, and Cunnington, R. White-matter abnormalities in attention deficit hyperactivity disorder: a diffusion tensor imaging study. Hum Brain Mapp. (2009) 30:2757–65. doi: 10.1002/Hbm.20703
60. Mak, E, Gabel, S, Su, L, Williams, GB, Arnold, R, Passamonti, L, et al. Multi-modal MRI investigation of volumetric and microstructural changes in the Hippocampus and its subfields in mild cognitive impairment, Alzheimer's disease, and dementia with Lewy bodies. Int Psychogeriatr. (2017) 29:545–55. doi: 10.1017/S1041610216002143
61. Duan, Y, Norcia, AM, Yeatman, JD, and Mezer, A. The structural properties of major white matter tracts in Strabismic amblyopia. Invest Ophthalmol Vis Sci. (2015) 56:5152–60. doi: 10.1167/Iovs.15-17097
62. Peters, BD, Ikuta, T, Derosse, P, John, M, Burdick, KE, Gruner, P, et al. Age-related differences in white matter tract microstructure are associated with cognitive performance from childhood to adulthood. Biol Psychiatry. (2014) 75:248–56. doi: 10.1016/J.Biopsych.2013.05.020
63. Dalley, JW, Theobald, DE, Bouger, P, Chudasama, Y, Cardinal, RN, and Robbins, TW. Cortical cholinergic function and deficits in visual attentional performance in rats following 192 igg-Saporin-induced lesions of the medial prefrontal cortex. Cereb Cortex. (2004) 14:922–32. doi: 10.1093/cercor/bhh052
64. Kabukcu Basay, B, Buber, A, Basay, O, Alacam, H, Ozturk, O, Suren, S, et al. White matter alterations related to attention-deficit hyperactivity disorder and Comt Val(158)met polymorphism: children with valine homozygote attention-deficit hyperactivity disorder have altered white matter connectivity in the right cingulum (cingulate gyrus). Neuropsychiatr Dis Treat. (2016) 12:969–81. doi: 10.2147/Ndt.S104450
65. Cooper, M, Thapar, A, and Jones, DK. ADHD severity is associated with white matter microstructure in the subgenual cingulum. Neuroimage Clin. (2015) 7:653–60. doi: 10.1016/J.Nicl.2015.02.012
66. Fuelscher, I, Hyde, C, Anderson, V, and Silk, TJ. White matter tract signatures of Fiber density and morphology in ADHD. Cortex. (2021) 138:329–40. doi: 10.1016/J.Cortex.2021.02.015
67. Hamilton, LS, Levitt, JG, O'neill, J, Alger, JR, Luders, E, Phillips, OR, et al. Reduced white matter integrity in attention-deficit hyperactivity disorder. Neuroreport. (2008) 19:1705–8. doi: 10.1097/Wnr.0b013e3283174415
68. Lawrence, KE, Levitt, JG, Loo, SK, Ly, R, Yee, V, O'neill, J, et al. White matter microstructure in subjects with attention-deficit/hyperactivity disorder and their siblings. J Am Acad Child Adolesc Psychiatry. (2013) 52:431–440.e4. doi: 10.1016/J.Jaac.2013.01.010
69. Chiang, HL, Chen, YJ, Lo, YC, Tseng, WY, and Gau, SS. Altered white matter tract property related to impaired focused attention, sustained attention, cognitive impulsivity and vigilance in attention-deficit/ hyperactivity disorder. J Psychiatry Neurosci. (2015) 40:325–35. doi: 10.1503/Jpn.140106
70. Chiang, HL, Chen, YJ, Shang, CY, Tseng, WY, and Gau, SS. Different neural substrates for executive functions in youths with ADHD: a diffusion Spectrum imaging tractography study. Psychol Med. (2016) 46:1225–38. doi: 10.1017/S0033291715002767
71. Amlien, IK, and Fjell, AM. Diffusion tensor imaging of white matter degeneration in Alzheimer's disease and mild cognitive impairment. Neuroscience. (2014) 276:206–15. doi: 10.1016/J.Neuroscience.2014.02.017
72. Xu, F, Jin, C, Zuo, T, Wang, R, Yang, Y, and Wang, K. Segmental abnormalities of superior longitudinal fasciculus microstructure in patients with schizophrenia, bipolar disorder, and attention-deficit/hyperactivity disorder: an automated Fiber quantification Tractography study. Front Psych. (2022) 13:999384. doi: 10.3389/Fpsyt.2022.999384
Keywords: attention-deficit/hyperactivity disorder, diffusion kurtosis imaging, automated fiber quantification, white matter microstructure, right cingulum bundle
Citation: Hu R, Tan F, Chen W, Wu Y, Jiang Y, Du W, Zuo Y, Gao B, Song Q and Miao Y (2023) Microstructure abnormalities of the diffusion quantities in children with attention-deficit/hyperactivity disorder: an AFQ and TBSS study. Front. Psychiatry. 14:1237113. doi: 10.3389/fpsyt.2023.1237113
Received: 08 June 2023; Accepted: 08 August 2023;
Published: 22 August 2023.
Edited by:
Soohwan Oh, Sungkyunkwan University, Republic of KoreaReviewed by:
Szilvia Anett Nagy, University of Pécs, HungaryCopyright © 2023 Hu, Tan, Chen, Wu, Jiang, Du, Zuo, Gao, Song and Miao. This is an open-access article distributed under the terms of the Creative Commons Attribution License (CC BY). The use, distribution or reproduction in other forums is permitted, provided the original author(s) and the copyright owner(s) are credited and that the original publication in this journal is cited, in accordance with accepted academic practice. No use, distribution or reproduction is permitted which does not comply with these terms.
*Correspondence: Yanwei Miao, eXdtaWFvNzE2QDE2My5jb20=
†These authors have contributed equally to this work
Disclaimer: All claims expressed in this article are solely those of the authors and do not necessarily represent those of their affiliated organizations, or those of the publisher, the editors and the reviewers. Any product that may be evaluated in this article or claim that may be made by its manufacturer is not guaranteed or endorsed by the publisher.
Research integrity at Frontiers
Learn more about the work of our research integrity team to safeguard the quality of each article we publish.