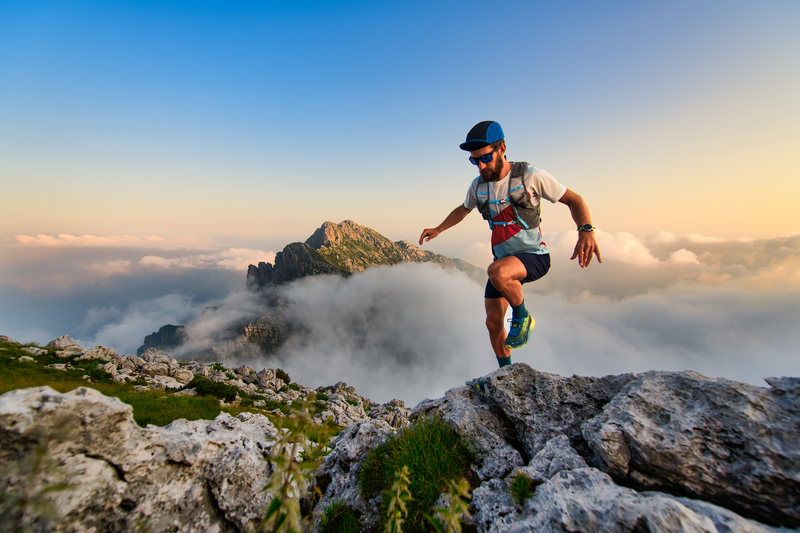
95% of researchers rate our articles as excellent or good
Learn more about the work of our research integrity team to safeguard the quality of each article we publish.
Find out more
ORIGINAL RESEARCH article
Front. Psychiatry , 30 August 2023
Sec. Forensic Psychiatry
Volume 14 - 2023 | https://doi.org/10.3389/fpsyt.2023.1231851
This article is part of the Research Topic Applications of Artificial Intelligence in Forensic Mental Health: Opportunities and Challenges View all 5 articles
Background: Suffering from schizophrenia spectrum disorder (SSD) has been well-established as a risk factor for offending. However, the majority of patients with an SSD do not show aggressive or criminal behavior. Yet, there is little research on clinical key features distinguishing offender from non-offender patients. Previous results point to poorer impulse control, higher levels of excitement, tension, and hostility, and worse overall cognitive functioning in offender populations. This study aimed to detect the most indicative distinguishing clinical features between forensic and general psychiatric patients with SSD based on the course of illness and the referenced hospitalization in order to facilitate a better understanding of the relationship between violent and non-violent offenses and SSD.
Methods: Our study population consisted of forensic psychiatric patients (FPPs) with a diagnosis of F2x (ICD-10) or 295.x (ICD-9) and a control group of general psychiatric patients (GPPs) with the same diagnosis, totaling 740 patients. Patients were evaluated regarding their medical (and, if applicable, criminal) history and the referenced psychiatric hospitalization. Supervised machine learning (ML) was used to exploratively evaluate predictor variables and their interplay and rank them in accordance with their discriminative power.
Results: Out of 194 possible predictor variables, the following 6 turned out to have the highest influence on the model: olanzapine equivalent at discharge from the referenced hospitalization, a history of antipsychotic prescription, a history of antidepressant, benzodiazepine or mood stabilizer prescription, medication compliance, outpatient treatment(s) in the past, and the necessity of compulsory measures. Out of the seven algorithms applied, gradient boosting emerged as the most suitable, with an AUC of 0.86 and a balanced accuracy of 77.5%.
Discussion: Our study aimed to identify the most influential illness-related predictors, distinguishing between FPP and GPP with SSD, thus shedding light on key differences between the two groups. To our knowledge, this is the first study to compare a homogenous sample of FPP and GPP with SSD regarding their symptom severity and course of illness using highly sophisticated statistical approaches with the possibility of evaluating the interplay of all factors at play.
Unlawful behavior imposes huge social and economic costs on society, affecting individuals, businesses, and institutions. This is especially relevant in regard to violent crimes: interpersonal violence is considered to be among the top 20 leading causes of disability-adjusted life years worldwide, thus posing a highly relevant burden on public health (1, 2). Since 1996, the World Health Assembly has repeatedly emphasized the problematic contribution of fatal and non-fatal interpersonal violence to global mortality and morbidity (3, 4). Apart from the incalculable human toll it takes, violence is also an economic burden. It has been estimated that interpersonal violence accounts for over seven times the cost of collective violence, e.g., in the context of armed conflicts or terrorist attacks (5). However, while the costs of violent crime may seem more tangible, non-violent crimes, such as theft or criminal damage, are by no means trivial offenses without victims (6). As with all criminal acts, they result in direct burdens on the victim, in the form of property damage and loss of value, on the criminal justice system, in the form of correctional programs, and on the perpetrator in the form of lost life opportunities (7). Therefore, with the intent of prevention, research in the past decades has increasingly focused on identifying risk factors for violent and non-violent criminal behaviors. Apart from certain biographical experiences, such as bullying or parental neglect, as well as poor moral judgment, neuropsychiatric disorders have been known to significantly increase both the relative and absolute risks for the perpetration of violent and non-violent criminal behaviors (8–10). Among those, substance use disorders, personality disorders, and psychotic disorders have been shown to have the strongest associations with violent and antisocial behaviors (10–12). While the latter are well-established as risk factors, the majority of all patients suffering from schizophrenia spectrum disorders (SSDs) do not engage in criminal behavior (13, 14). For instance, in a large retrospective study by Seena Fazel and Martin Grann (15), ~33% of all patients with schizophrenia had committed some form of violent offense, thus resulting in an attributable risk fraction of 2.3% (15). It should also be noted that patients suffering from severe mental disorders, in general, and psychotic disorders, in particular, are also at higher risk of victimization, although current research features some methodological difficulties (16, 17). As the occurrence of violence in patients with SSD and the subsequent stigmatization may also contribute to an elevated burden of disease, it is important to identify and address risk factors for violence in those patients (18, 19). In a meta-analysis by Yee et al. (14), only 22% of individuals with psychosis engaged in any form of criminal offending (including non-violent offending) (14), which raises the question of which factors contribute to an individual committing a crime in the course of their SSD, and which factors may have a protective effect. Yet, there is a scarcity of corresponding research: first, previous results often lack consistency regarding the roles of certain risk factors (20). Second, while individuals diagnosed with SSD pose a non-negligible subgroup of offenders, studies have seldom focused on this specific population, evaluating rather diagnostically heterogeneous samples, e.g., psychotic disorders in general (21–24). Those who did often times had a limited number of cases, thus having an increased risk of being subjected to a type II error (25–28). To date, poorer impulse control; higher levels of excitement, tension, and hostility; and worse overall cognitive functioning appear to draw a dividing line between offenders and non-offenders with SSD (20, 25, 26). This, however, leads to another issue: all of these factors are complex, multifactorial, and oftentimes interdependent and have yet to be comprehensively understood. Null hypothesis significance testing or simple linear regressions, assuming linear relationships between variables, may not accommodate the exploration of the complex phenomenon of offending in mental illness.
Therefore, the objectives of this study were as follows:
I) To exploratively evaluate clinical key factors from the patient's history as well as their referenced hospitalization, distinguishing offenders and non-offenders with SSD based on items regarding the underlying mental illness, using supervised machine learning (ML).
II) To quantify the performance of the calculated ML model.
The study was approved by the ethics committee in Zurich, Switzerland, under the reference number KEK-ZH-NR 2014–0480 as part of a larger, ongoing project investigating the characteristics of offender patients with SSD.
Our study group consisted of 370 male and female forensic psychiatric patients (FPPs) with a diagnosis of SSD according to ICD-10 (F2x) or ICD-9 (295.x) (29, 30). All of them had been in court-mandated inpatient treatment at the Center for Inpatient Forensic Therapies of the University Hospital of Psychiatry Zurich, Switzerland, between 1982 and 2016, with 296 of those cases being treated after 2000. The legal basis for such inpatient forensic therapy in Switzerland is provided by Article 59 of the Swiss Penal Code, and patients subjected to court-mandated treatment can either be referred to forensic psychiatric institutions (as in this case) or even to suitable residential facilities and specialized prison departments (31). Offenses leading to forensic psychiatric hospitalization included both violent and non-violent crimes.
The comparison group—which was matched to the study group by gender as indicated in the medical file as well as by the year of admission—comprised 370 male and female general psychiatric patients (GPPs), with a diagnosis of SSD according to ICD-10 (F2x) or ICD-9 (295.x) (29, 30), who had been in inpatient treatment at the Center for Integrative Psychiatry of the University Hospital of Psychiatry Zurich. This institution focuses on the sub-acute treatment of chronically ill patients with established initial pharmacotherapeutic treatment, making them a population quite suitable for comparison with FPP, who are mostly admitted from a custodial setting in which they too have received initial antipsychotic treatment.
Both facilities have secured and open wards, though, given the legal context of their institutionalization, the forensic psychiatric patients were partially and temporarily subjected to a more tightly secured treatment setting, starting in high-security wards and progressing to progressively more open wards if improving clinically.
The outcome variable (y) “forensic psychiatric patient (FPP)” was dichotomized (true vs. untrue), with “FPP – untrue” being defined as the positive class in further analysis.
All of our data came from the case files of the patients, which included professionally documented medical histories, psychiatric and psychological reports, inpatient and outpatient reports of both hospitalizations and outpatient treatments, extensive interdisciplinary progress reports, and—for the forensic psychiatric population—testimonies, court proceedings, and data regarding previous imprisonments and detentions. The retrospective data assessment was performed independently by two experienced psychiatrists applying a directed qualitative content analysis as described by Hsieh and Shannon (32).
As part of the aforementioned ongoing overarching research project, over 500 variables were extracted (for an extensive list of all variables, including definitions, please refer to the data availability statement). As we wanted to focus on key differences related to the underlying psychiatric disorder, we selected 194 illness-related variables out of all of these variables for this analysis. A list of those variables included in the further analysis is provided in the Appendix. For a specific definition of the predictor variables, please refer to the coding protocol provided in the data availability statement.
As described in the Section 1, we aimed to exploratively identify the most indicative predictors capable of discriminating between forensic and general psychiatric patients with SSD. Thus, we chose a supervised machine learning (ML) approach for the statistical analysis. Figures 1, 2 navigate through the statistical process, which is described in further detail below.
In an initial step, all data underwent preprocessing for ML purposes (Figure 1, Step 1): Variables with >33% missing values in the total population were eliminated from further analysis. This was performed to reduce the negative influence of missing variables on the accuracy of the model (33). Categorical variables were converted to binary code. As described in Section 2.2, our outcome variable (y) was defined as “forensic psychiatric patient – true/untrue”. The dataset was then divided into two subsets: the training subset, comprising 70% of all cases, and the validation subset with the remaining 30% (Figure 1, Step 2). The validation subset was set aside for later application of the trained algorithm, which underwent the following learning process (Steps 3–5) strictly on the training subset. This step was crucial to providing the algorithms with an optimized “training ground” for the building of the model and to still have an untouched set of data for later validation purposes.
Missing values in the training set were imputed by mean for continuous variables and mode for categorical variables, as provided by the features of the MLR package (Figure 1, Step 3). The coefficients used in the imputation process were also stored for later application in the imputation process on the validation set. To spare computational resources and increase the overall performance of the model, we performed a dimension reduction through the random forest algorithm (randomForestSRC package implemented in the MLR package, evaluating variable importance) (Figure 1, Step 4). This dimensionality reduction was performed up to the point where the AUC did not improve by >5% when adding another variable. After the completion of the preprocessing features, seven different algorithms were applied to the training set for discriminative model building: logistic regression, trees, random forest, gradient boosting, k-nearest neighbor (KNN), support vector machines (SVMs), and naïve Bayes as an easily applicable generative model. These algorithms were assessed in terms of their balanced accuracy (the average of the true-positive and true-negative rates) and goodness of fit (measured with the receiver operating characteristic, balanced curve area under the curve method, ROC-balanced AUC) (Figure 1, Step 5). The model with the highest AUC— the algorithm most suitable for the data structure—was selected for the final model validation. In the next step, we aimed to reduce a common obstacle in ML, which is so-called “overfitting”. Broadly speaking, overfitting occurs when a model learns noise in the training data to such an extent that the predictive ability of the model is compromised (34, 35). To counteract this phenomenon, we performed a nested resampling. Data processing and model training were performed while embedded in cross-validation, and the performance of these models was tested in an outer loop also embedded in cross-validation. Thus, we artificially created different subsamples of the same dataset, all while the validation subset remained untouched (Figure 1, Step 6). In doing so, we averaged the error estimation over all six trials to get the total effectiveness of the model. This completed the learning process. Further steps were performed on the validation subset previously stored aside (Figure 2).
On the validation set, missing values were imputed with the same weights as on the training set, which had been previously stored (Figure 2, Step 1). Then, the gradient boosting model, earlier identified as most suitable to our data, was applied to the validation set and evaluated in terms of its ROC parameters (Figure 2, Step 2). As a final step, the variables constituting the model were ranked according to their indicative power (Figure 2, Step 3).
Patients in both groups were predominantly male (>90%), in their mid-30s, and single at the time of their admission to the referenced hospitalization. Compared to FPPs, GPPs were born in Switzerland more often. Regarding diagnoses, almost three-quarters of cases in both groups had been diagnosed with schizophrenia (F20.x according to ICD-10), while other diagnoses from the psychosis spectrum, for example, schizoaffective disorder, were less prevalent. The majority of patients also had some kind of substance use disorder as a comorbidity, with a higher prevalence among FPP. Comorbid personality disorders, while in general relatively uncommon, were also more frequently diagnosed in the FPP group. Table 1 provides an overview of these basic characteristics in both groups.
Out of all of the seven algorithms applied in the learning process, gradient boosting emerged as the one with the best performance parameters on the training set, yielding a balanced accuracy of 78.5% and an AUC of 0.88 (Table 2).
After the reduction in dimensionality through the application of random forest down to the point where the AUC of the model did not improve by >5% when adding another variable, six variables emerged as most discriminative regarding the outcome variable (see Table 3).
Applied to the validation subset (30% of all cases), gradient boosting was performed with a balanced accuracy of 77.5% and an AUC of 0.86; it can therefore be considered that the model features an excellent ability to distinguish between the two groups (36). Both patients with forensic and general psychiatric backgrounds were correctly identified in most cases, as indicated by a sensitivity of 71.4% and a specificity of 83.5% (see Table 4).
In the ranking of their contribution to the model, the olanzapine equivalent upon discharge emerged as the most indicative, whereas the remaining five variables had a similar influence (see one-sided tornado graph, Figure 3).
While certain risk factors for offending as a negative outcome of a mental disorder are well-established and reproducible, there is still uncertainty and inconsistency about which patients affected by SSDs tend to perform some kind of illegal act during the course of their illness. The purpose of this study was, therefore, to evaluate clinical key factors distinguishing offenders and non-offenders with SSD based on items regarding the underlying mental illness, using supervised machine learning (ML) as a statistical approach with the ability to analyze the interplay of a large quantity of potentially influential variables. Out of 194 possible predictor variables, six emerged as defining a gradient boosting model yielding a balanced accuracy of 77.5% and an AUC of 0.86.
The cumulative antipsychotic dose, defined by the prescribed olanzapine equivalent upon discharge from the referenced hospitalization, emerged as a variable with by far the highest relative influence: With a mean dose of 22 mg, FPP had higher antipsychotic doses when released from the institution than their GPP counterparts (mean dose: 19 mg). While the literature on prescription patterns in forensic psychiatric settings is sparse, higher doses of antipsychotics in offender populations in comparison with the general population have been sporadically reported by other authors: Stone-Brown et al. (37) described a high-dose antipsychotic rate of ~30% in a mixed-diagnosis prison population (37). In an Italian forensic psychiatric sample, also with diagnostic heterogeneity, high-dose antipsychotics were even administered in around half the cases (38). In contrast, in general psychiatry, high-dose antipsychotic rates of ~20% are reported, although generally, there is a lack of consensus as to how exactly high-dose prescription is defined, thus making a comparison between studies difficult (39–42). There are several possible explanations for these differences in dosing between general and forensic psychiatric populations. It has been previously suggested that the prescription patterns in offender populations with mental health problems are not merely, maybe not even primarily, driven by illness-related aspects. For instance, Mandarelli et al. (38) reported an association between aggressive behavior and higher doses of antipsychotics but could find no diagnostic correlation (38). They hypothesized that pharmacotherapeutic interventions are one of the few treatment options available in a forensic psychiatric setting in which there is oftentimes, especially in the initial treatment phase, a lack of cooperation and insights into the necessity of treatment on the patient's side. With the forensic psychiatrist's responsibility to not only tend to the individual needs of the patient but to reduce their social dangerousness (fulfill legal obligations/impositions) and prevent illness-driven reoffending, an intensified pharmacotherapeutic approach may be the result of said double-mandate (43, 44). That being said, it has to be noted that, although plasma levels of antipsychotic medication were regularly obtained in the FPP sample, the retrospective data may not sufficiently illustrate whether the patient was regularly taking the medication prescribed.
At the same time, GPPs were characterized by a higher rate of prescriptions of other psychopharmacologic substances, e.g., benzodiazepines and antidepressants, than FPP. This is highly contradictory to an Italian prison study on offenders with mental illness, which observed that psychopharmacologic agents additional to antipsychotics were administered in even 92% of cases (45). A small-sample UK prison study reported concurrent psychopharmacotherapies in 85% of cases (46). However, both the UK and the Italian study populations were quite heterogenous in their diagnoses, and there was a high rate of off-label prescriptions common in prison settings, such as anxiolytics and hypnotics (46, 47). Due to the considerable diagnostic differences, robust comparability of our results is hardly given. Yet, it does, indeed, seem contradictory that GPPs were more likely to receive additional sedatives than FPPs, even though the latter can be considered to be at higher risk of impulsivity and agitation. The authors have previously suggested that the already high doses of antipsychotics in FPP could have made further sedation, i.e., with benzodiazepines, unnecessary. Another possible explanation may lie in the concern of a paradoxical reaction to hypnotics, which—although generally rare at <1%—are more likely in patients with a history of aggressive behavior (48). Further research on prescription patterns in the forensic psychiatric treatment of patients with SSD is necessary to evaluate these hypotheses before drawing clinical conclusions.
FPPs were less likely to have had outpatient treatment and a prescription for antipsychotic medication before the referenced hospitalization than their GPP comparison group. Insufficient embedment into the mental healthcare system and thus insufficient treatment is a known correlate of criminal behavior as a negative outcome during the course of SSD (49). Previous research has also demonstrated a particularly high risk of offending during the first psychotic episode, with untreated impulsivity as a major mediator of aggressiveness toward others, but also toward oneself. Nielssen et al. (50) reported that over a third of all fatal and non-fatal serious assaults committed by patients with SSD occurred during an untreated first psychotic episode (50). Poor executive functioning and poor self-care skills—both also associated with violence in psychosis—may further hinder affected individuals from seeking help (51).
FPP also had a much lower rate of pharmacotherapeutic compliance (11% vs. 55%). This finding supports the previous literature, according to which violent and non-violent offenses are associated with lower levels of treatment adherence (or vice versa) (37, 52–54). Intuitively, one would assume that lower levels of insight into the underlying mental illness within forensic psychiatric populations are responsible for the reported lack of adherence. A lack of insight into their mental illness and subsequently into the need for treatment is common for individuals with SSD, especially with active symptoms, as the perception of the surroundings and one's self are altered due to the underlying pathology (55). However, forensic psychiatric patients with SSD appear to be similarly aware of their condition as general psychiatric patients, as demonstrated by an Australian study (56). Alia-Klein et al. (57) argued that a binary definition fails to cover the complexity of the phenomenon of insight into illness (57). In a forensic psychiatric sample of patients suffering from psychosis, they found that non-adherence was, in fact, independent of poor insights. Instead, they suggested that patients could be cognitively aware of their mental disorder, but, at the same time, lack affective concern regarding it. In their evaluation of offenders with schizophrenia, Rezansoff et al. (54) too argued against the binary definition of treatment compliance and found an increased risk of violent and non-violent offenses in individuals with lower levels of compliance (54). Authors investigating the construct of awareness and compliance in general psychiatric populations, such as Dam et al. (58), have also argued that a dichotomous definition fails to cover the many layers of insight and compliance and that the two are certainly interwoven but cannot be assumed to be synonymous (58). It seems, therefore, worthwhile to further explore factors driving (non-)adherence in forensic psychiatric samples.
FPP proved to have a higher rate of compulsory measures during the referenced hospitalization, such as mechanical restraint, isolation/seclusion, or compulsory application of medication. In Switzerland, coercive measures are taken as a last resort either in emergency situations, i.e., to avert immediate danger to oneself or others, or electively in the case of patients who are incapable of giving consent and are no longer able to make a decision themselves (59, 60). One hypothesis for the higher rate of compulsory measures in the FPP sample is the higher prevalence of self-harm in offender populations (61). The more frequent use of coercion in FPP is also unsurprising given that violence or threat of violence—with a high prevalence in FPP populations—is among the most frequently identified factors associated with coercive measures (62–65). As this parameter reflects coercive measures during forensic hospitalization, thus, after the committed offense, clinicians may also be quicker to opt for coercive measures if the patient has been deemed particularly dangerous. This may be especially the case for patients whose behavior is closely linked to the dynamics of their offense. At the same time, reported rates of coercive measures show a wide range both in forensic and general psychiatry, and a higher prevalence of such interventions has not been well-established for forensic psychiatric populations (66). This research gap results from a lack of coherent definitions of coercion and different research methods altogether. Thus, empirical research on coercive measures in forensic psychiatry is scarce and needs to be expanded further, especially since ethical aspects are stressed in the forensic psychiatric context with already restricted treatment settings.
Strikingly, all of the six most distinguishing items related to treatment, e.g., the severity of psychopathology, type of symptoms, or comorbidities such as substance use and personality disorders did not emerge as dominant in the model. This seems surprising at first glance; after all, a higher prevalence of substance use disorders and certain personality disorders in offender populations than in the general population has been described by several previous research groups (67–70). The comorbidities are also known risk factors for violent behavior, which also makes a consequential involvement with the criminal justice system more likely (20, 71). The same goes for the prevalence of certain symptoms of SSD, which are considered to increase the likelihood of offending, such as a higher expression of positive symptoms and general severity of symptoms—although there is still controversy over which types of symptoms exactly promote criminal behavior in SSD, and corresponding findings are oftentimes inconsistent (72, 73). The fact that our findings diverge from these previous results by no means indicates that known and well-established risk factors for problematic and potentially illegal behavior are contradicted. Psychopathology, for instance, might be featured indirectly in the model, i.e., through the items regarding psychopharmacologic prescriptions and higher doses. Furthermore, with the forensic psychiatric institution being much more structured and restrictive than the general psychiatric facility, the setting of care may have influenced the outcome regarding the expressiveness and influence of psychopathology. Nevertheless, while a certain indirect influence of said domains seems likely and cannot by any means be ruled out entirely, our findings suggest that criminal development does not merely depend on the presence of unfavorable symptomatology and morbidity. In fact, it seems as if the likelihood of offense as a negative outcome can be meaningfully reduced if the affected patient is well integrated into the mental healthcare system and adequately and sufficiently treated. This aspect of compensability of certain traits as well-established stand-alone risk factors for violence and offending has already been discussed in light of previous results of the authors regarding aggression as the negative outcome of an SSD but has not been widely covered in other existing literature (44). The crime-preventive strength of a sufficient embedment in the mental healthcare and support system emphasizes the importance of early-on detection of mental disorders and potential comorbidities and the proper integration of the affected individual into appropriate and available structures. In this context, clinicians need to make an effort to promote understanding and awareness of individual needs, risk factors, and protective resources that could be strengthened.
Regarding the potential limitations of the presented study, the retrospective design needs to be addressed. Retrospective designs are considered inferior to prospective studies for numerous reasons. First of all, the quality of the data depends highly on the quality and accuracy of the documentation (74). This can be especially problematic for variables that lack a clear definition, e.g., “negative attitude toward staff”, which may be interpreted differently when assessed by different clinicians. Furthermore, as a common obstacle in retrospective designs, some variables had a high rate of missing values and had to be excluded from further analysis. Thus, there is a certain risk of bias from the resources in this study. One could also argue that, with the development of, for example, other pharmacological agents, the broad timeframe for case inclusion (spanning over 30 years) could skew the results in some respects. However, with the duration of inpatient forensic treatment in Switzerland being rather long (Article 59 foresees 5 years of treatment, which may be prolonged), a shorter timeframe would have led to a much smaller sample, thus reducing the robustness of the results. Regarding statistical limitations, one has to be aware that while the dataset is rather larger from a forensic psychiatric point of view, it is merely moderate for ML purposes. The larger the dataset, the better the performance of an ML algorithm. As a result, our model is more likely to be subjected to overfitting than an even larger sample would be—a risk we have attempted to counteract through the application of nested cross-validation (35). However, while the cross-validation limits overfitting, it does not completely solve the issue—although with the small delta in performance parameters between the training and validation sets, the effect of cross-validation appears to be significant. Again, a training process on an even larger sample would be an advisable approach for future research. Less as a limitation and more as a fact that should be kept in mind when interpreting the results, possible multicollinearity has to be considered, meaning that items that did not emerge as most indicative in the gradient boosting model could have had an indirect influence as well. For instance, the higher dose of antipsychotics in the FPP group may have been the result of a higher prevalence of aggressive behavior or a more severe degree of symptomatology. Another important note is that the mediators for violent vs. non-violent crime may be different for individuals with SSD—as both types of offenders were included in this study, no discrimination in that regard can be made based on our results. Finally, since our population consisted of male patients in over 90% of cases, it is not possible to derive implications for female populations from our results. The same goes for differentiation between forensic and general psychiatric outpatients, as we only focused on patients in inpatient settings. Yet, we opted to include the small available number of women to represent a patient population that corresponds to the reality of the penitentiary system (which, with that in mind, could be considered a strength of our study). Further strengths lie in the study's ability to close a significant research gap: Comparative studies on forensic psychiatric patients with SSD are scarce, even though this population can be considered highly relevant due to its well-established elevated risk of aggressive and violent behavior (11, 75). With a total of 740 rather homogenous cases, this is—to the authors' knowledge—one of the largest comparative analyses of said group. Furthermore, the application of ML allowed the analysis of a large number of variables as well as their interplay in a multidimensional model, while most commonly used statistical procedures are limited in that regard (e.g., null-hypothesis significance testing) (76–78).
In summary, the study presented sheds light on factors distinguishing individuals with SSD who end up involved with the judicial system from those who do not. Forensic psychiatric patients showed worse integration into outpatient treatment facilities, a lower ratio of medication compliance, and prescriptions of antipsychotics as well as other psychopharmacotherapies substances but a higher mean antipsychotic dose and a higher likelihood of undergoing coercive measures during hospitalizations than their comparison group from general psychiatry. At the same time, the domain of psychopathology does not seem to be a major distinguishing factor between the two groups. Through the application of artificial intelligence, the complex interplay of risk and protective factors in the development of criminal behavior in individuals with SSD could be further explored. Without a profound understanding of both, the establishment of effective preventive measures is not possible. While this research gap, which is well in need of closing, will remain unless the results are reproduced, validated, and thus proven robust in larger populations, our findings promote, once again, the efforts of clinical and health political agents to integrate individuals affected by SSD into the mental healthcare system. This advocation includes low financial and administrative barriers for entering therapeutic institutions, for both in- and out-patients.
The datasets presented in this study can be found in online repositories. The names of the repository/repositories and accession number(s) can be found at: https://www.researchgate.net/publication/363044110_Coding_protocol_Pathways_into_delinquency_in_offenders_suffering_from_schizophrenia_spectrum_disorders.
The studies involving humans were approved by the Ethikkommission des Kantons Zürich (KEK Zürich), Switzerland. The studies were conducted in accordance with local legislation and institutional requirements. The Ethics Committee/institutional review board waived the requirement of written informed consent for participation from the participants or the participants' legal guardians/next of kin because this research project was reviewed and approved by the Ethics Committee Zurich under the reference number KEK-ZH-NR 2014-0480. Patient consent was waived due to the retrospective design, for which formal consent is not necessarily required in the Canton of Zurich.
LM and JK: conceptualization, methodology, validation, formal analysis, investigation, and data curation. JK: software, resources, supervision, and project administration. LM: writing—original draft preparation and visualization. LM, AH, JM, and JK: writing—reviewing and editing. All authors contributed to the article and approved the submitted version.
The authors declare that the research was conducted in the absence of any commercial or financial relationships that could be construed as a potential conflict of interest.
All claims expressed in this article are solely those of the authors and do not necessarily represent those of their affiliated organizations, or those of the publisher, the editors and the reviewers. Any product that may be evaluated in this article, or claim that may be made by its manufacturer, is not guaranteed or endorsed by the publisher.
The Supplementary Material for this article can be found online at: https://www.frontiersin.org/articles/10.3389/fpsyt.2023.1231851/full#supplementary-material
1. Lopez AD, Mathers CD, Ezzati M, Jamison DT, Murray CJ. Global and regional burden of disease and risk factors, 2001: systematic analysis of population health data. Lancet. (2006) 367:1747–57. doi: 10.1016/S0140-6736(06)68770-9
2. Dahlberg LL, Krug EG. Violence a global public health problem. Ciência Saúde Coletiva. (2006) 11:277–92. doi: 10.1590/S1413-81232006000200007
3. Williams DJ, Donnelly PD. Is violence a disease? Situating violence prevention in public health policy and practice. Public Health. (2014) 128:960–7. doi: 10.1016/j.puhe.2014.09.010
4. Krug EG, Mercy JA, Dahlberg LL, Zwi AB. The world report on violence and health. Lancet. (2002) 360:1083–8. doi: 10.1016/S0140-6736(02)11133-0
5. Hoeffler M. What are the costs of violence? Polit Philos Econ. (2017) 16:422–45. doi: 10.1177/1470594X17714270
7. McCollister KE, French MT, Fang H. The cost of crime to society: New crime-specific estimates for policy and program evaluation. Drug Alcohol Depend. (2010) 108:98–109. doi: 10.1016/j.drugalcdep.2009.12.002
8. Ttofi MM, Farrington DP, Lösel F. School bullying as a predictor of violence later in life: A systematic review and meta-analysis of prospective longitudinal studies. Aggress Violent Behav. (2012) 17:405–18. doi: 10.1016/j.avb.2012.05.002
9. Hoeve M, Dubas JS, Eichelsheim VI, van der Laan PH, Smeenk W, Gerris JR. The relationship between parenting and delinquency: a meta-analysis. J Abnorm Child Psychol. (2009) 37:749–75. doi: 10.1007/s10802-009-9310-8
10. Fazel S, Smith EN, Chang Z, Geddes JR. Risk factors for interpersonal violence: an umbrella review of meta-analyses. Br J Psychiatry. (2018) 213:609–14. doi: 10.1192/bjp.2018.145
11. Fazel S, Gulati G, Linsell L, Geddes JR, Grann M. Schizophrenia and violence: systematic review and meta-analysis. PLoS Med. (2009) 6:e1000120. doi: 10.1371/journal.pmed.1000120
12. Assink M, Van Der Put CE, Hoeve M, Vries SLA, Stams GJJM, Oort FJ. Risk factors for persistent delinquent behavior among juveniles: a meta-analytic review. Clin Psychol Rev. (2015) 42:47–61. doi: 10.1016/j.cpr.2015.08.002
13. Whiting D, Lichtenstein P, Fazel S. Violence and mental disorders: a structured review of associations by individual diagnoses, risk factors, and risk assessment. Lancet Psychiatry. (2021) 8:150–61. doi: 10.1016/S2215-0366(20)30262-5
14. Yee N, Matheson S, Korobanova D, Large M, Nielssen O, Carr V, et al. A meta-analysis of the relationship between psychosis and any type of criminal offending, in both men and women. Schizophr Res. (2020) 220:16–24. doi: 10.1016/j.schres.2020.04.009
15. Seena Fazel MBCB, Martin Grann CP. The population impact of severe mental illness on violent crime. Am J Psychiatr. (2006) 163:1397–403. doi: 10.1176/ajp.2006.163.8.1397
16. K. Latalova, D. Kamaradova, J. Prasko, Violent victimization of adult patients with severe mental illness: a systematic review. Neuropsychiatr Dis Treatment. (2014) 25:1925–39. doi: 10.2147/NDT.S68321
17. Fazel S, Sariaslan A. Victimization in people with severe mental health problems: the need to improve research quality, risk stratification and preventive measures. World Psychiatry. (2021) 20:437. doi: 10.1002/wps.20908
18. Yanos PT, DeLuca JS, Roe D, Lysaker PH. The impact of illness identity on recovery from severe mental illness: a review of the evidence. Psychiatry Res. (2020) 288:112950. doi: 10.1016/j.psychres.2020.112950
19. Barlati S, Morena D, Nibbio G, Cacciani P, Corsini P, Mosca A, et al. Internalized stigma among people with schizophrenia: relationship with socio-demographic, clinical and medication-related features. Schizophr Res. (2022) 243:364–71. doi: 10.1016/j.schres.2021.06.007
20. Witt K, Van Dorn R, Fazel S. Risk factors for violence in psychosis: systematic review and meta-regression analysis of 110 studies. PLoS ONE. (2013) 8:e55942. doi: 10.1371/journal.pone.0055942
21. Link BG, Stueve A, Phelan J. Psychotic symptoms and violent behaviors: probing the components of “threat/control-override” symptoms. Soc Psychiatry Psychiatr Epidemiol. (1998) 33:S55–60. doi: 10.1007/s001270050210
22. Taylor PJ, Gunn J. Violence and psychosis. I Risk of violence among psychotic men. Br Med J. (1984) 288:1945–9. doi: 10.1136/bmj.288.6435.1945
23. Douglas KS, Guy LS, Hart SD. Psychosis as a risk factor for violence to others: a meta-analysis. Psychol Bull. (2009) 135:679. doi: 10.1037/a0016311
24. Foley SR, Kelly BD, Clarke M, McTigue O, Gervin M, Kamali M. Incidence and clinical correlates of aggression and violence at presentation in patients with first episode psychosis. Schizophr Res. (2005) 72:161–8. doi: 10.1016/j.schres.2004.03.010
25. Landgraf S, Blumenauer K, Osterheider M, Eisenbarth H. A clinical and demographic comparison between a forensic and a general sample of female patients with schizophrenia. Psychiatry Res. (2013) 210:1176–83. doi: 10.1016/j.psychres.2013.09.009
26. Barlati S, Nibbio G, Stanga V, Giovannoli G, Calzavara-Pinton I. Cognitive and clinical characteristics of offenders and non-offenders diagnosed with schizophrenia spectrum disorders: results of the recoviwel observational study. Eur Arch Psychiatry Clin Neurosci. (2022) 30:1–10. doi: 10.1007/s00406-022-01510-9
27. Akobeng AK. Understanding type I and type II errors, statistical power and sample size. Acta Paediatr. (2016) 105:605–9. doi: 10.1111/apa.13384
28. Abu-Akel A, Abushua'leh K. ‘Theory of mind'in violent and nonviolent patients with paranoid schizophrenia. Schizophrenia Res. (2004) 69:45–53. doi: 10.1016/S0920-9964(03)00049-5
29. WHO. ICD-10: International Statistical Classification of Diseases and Related Health Problems: Tenth Revision. Geneva: World Health Organization (2004).
30. Slee VN. The International classification of diseases: ninth revision (ICD-9). Am Coll Phys. (1978) 23:424–6. doi: 10.7326/0003-4819-88-3-424
31. Eidgenossenschaft DBS. Schweizerisches Strafgesetzbuch (2022). Available online at: https://www.fedlex.admin.ch/eli/cc/54/757_781_799/de#art_59
32. Hsieh H-F, Shannon SE. Three approaches to qualitative content analysis. Qual Health Res. (2005) 15:1277–88. doi: 10.1177/1049732305276687
33. Saar-Tsechansky M, Provost F. Handling missing values when applying classification models. J Mach Learn Res. (2007) 8:1625–57.
34. Dietterich T. Overfitting and under computing in machine learning. ACM computing Sur. (1995) 27:326–7. doi: 10.1145/212094.212114
35. Ying X. An overview of overfitting and its solutions. J Phys. (2019) 1168:022022. doi: 10.1088/1742-6596/1168/2/022022
36. Marzban C. The ROC curve and the area under it as performance measures. Weather Forecasting. (2004) 19:1106–14. doi: 10.1175/825.1
37. Stone-Brown K, Naji M, Francioni A, Myers K, Samarendra H, Mushtaq-Chaudhry H. Psychotropic prescribing in seriously violent men with schizophrenia or personality disorder in a UK high security hospital. CNS Spectr. (2016) 21:60–9. doi: 10.1017/S1092852915000784
38. Mandarelli G, Carabellese F, Sciascio GDi, Catanesi R. Antipsychotic Polypharmacy and high-dose antipsychotic regimens in the residential italian forensic psychiatric population (REMS). Front Psychol. (2022) 13:722985. doi: 10.3389/fpsyg.2022.722985
39. Harrington M, Lelliott P, Paton C, Okocha C, Duffett R, Sensky T. The results of a multi-centre audit of the prescribing of antipsychotic drugs for in-patients in the UK. Psychiatric Bulletin. (2002) 26:414–8. doi: 10.1192/pb.26.11.414
40. Adesola A, Anozie I, Erohubie P, James B. Prevalence and correlates of “high dose” antipsychotic prescribing: findings from a hospital audit. Ann Med Health Sci Res. (2013) 3:62–6. doi: 10.4103/2141-9248.109499
41. Lelliott P, Paton C, Harrington M, Konsolaki M, Sensky T, Okocha C. The influence of patient variables on polypharmacy and combined high dose of antipsychotic drugs prescribed for in-patients. Psychiatric Bulletin. (2002) 26:411–4. doi: 10.1192/pb.26.11.411
42. McMillan SS, Jacobs S, Wilson L, Theodoros T, Robinson G, Anderson C. Antipsychotic prescribing for vulnerable populations: a clinical audit at an acute Australian mental health unit at two-time points. BMC Psychiatry. (2017) 17:139. doi: 10.1186/s12888-017-1295-1
43. Habermeyer E, Dreßing H, Seifert D, Lau S. Praxishandbuch Therapie in der Forensischen Psychiatrie und Psychologie. New York, NY: Elsevier (2021).
44. Kirchebner J. Offenders and non-offenders with schizophrenia-spectrum-disorders: do they really differ in known risk factors for aggression? Front Psychiatr. (2023) 14:1145644. doi: 10.3389/fpsyt.2023.1145644
45. Pelizza L, Maestri D, Paulillo G, Pellegrini P. Prevalence and appropriateness of antipsychotic prescribing in an Italian prison: is everything always really overprescribed? J Clin Psychopharmacol. (2022) 42:31–6. doi: 10.1097/JCP.0000000000001495
46. Carey L, Barlow S. Review of antipsychotic prescribing at HMP/YOI low newton. BJPsych Bull. (2022) 46:26–32. doi: 10.1192/bjb.2020.80
47. Brown GP, Stewart LA, Rabinowitz T, Boudreau H, Wright AJ. Approved and off-label use of prescribed psychotropic medications among federal Canadian inmates. Can J Psychiatry. (2018) 63:683–91. doi: 10.1177/0706743718773734
48. Mancuso CE, Tanzi MG, Gabay M. Paradoxical reactions to benzodiazepines: literature review and treatment options. Pharmacotherapy. (2004) 24:1177–85. doi: 10.1592/phco.24.13.1177.38089
49. Fazel S, Zetterqvist J, Larsson H, Långström N, Lichtenstein P. Antipsychotics, mood stabilisers, and risk of violent crime. Lancet. (2014) 384:1206–14. doi: 10.1016/S0140-6736(14)60379-2
50. Nielssen OB, Malhi GS, McGorry PD, Large MM. Overview of violence to self and others during the first episode of psychosis. J Clin Psychiatry. (2012) 73:13675. doi: 10.4088/JCP.11r07036
51. Silverstein SM, Del Pozzo J, Roché M, Boyle D, Miskimen T. Schizophrenia and violence: realities and recommendations. Crime Psychol Rev. (2015) 1:21–42. doi: 10.1080/23744006.2015.1033154
52. J.C. Duncan, R. Rogers, Medication compliance in patients with chronic schizophrenia: implications for the community management of mentally disordered offenders. J Foren Sci. (1998) 43:1133–7. doi: 10.1520/JFS14375J
53. Citrome L, Volavka J. Pharmacological management of acute and persistent aggression in forensic psychiatry settings. CNS Drugs. (2011) 25:1009–21. doi: 10.2165/11596930-000000000-00000
54. Rezansoff SN, Moniruzzaman A, Fazel S, McCandless L, Somers JM. Adherence to antipsychotic medication and criminal recidivism in a Canadian provincial offender population. Schizophr Bull. (2017) 43:1002–10. doi: 10.1093/schbul/sbx084
55. Mintz AR, Dobson KS, Romney DM. Insight in schizophrenia: a meta-analysis. Schizophr Res. (2003) 61:75–88. doi: 10.1016/S0920-9964(02)00316-X
56. Carroll A, Pantelis C, Harvey C. Insight and hopelessness in forensic patients with schizophrenia. J Psychiatr. (2004) 38:169–73. doi: 10.1080/j.1440-1614.2004.01315.x
57. Alia-Klein N, O'Rourke TM, Goldstein RZ, Malaspina D. Insight into illness and adherence to psychotropic medications are separately associated with violence severity in a forensic sample. Aggress Behav. (2007) 33:86–96. doi: 10.1002/ab.20170
58. Dam J. Insight in schizophrenia: a review. Nord J Psychiatry. (2006) 60:114–20. doi: 10.1080/08039480600600185
59. Hachtel. Zwangsmassnahmen im massnahmenvollzug. Bulletin des Médecins Suisses. (2015) 96:245–7. doi: 10.4414/bms.2015.03162
60. Hoff P. Compulsory interventions are challenging the identity of psychiatry. Front Psychiatr. (2019) 10:783. doi: 10.3389/fpsyt.2019.00783
61. James K, Stewart D, Bowers L. Self-harm and attempted suicide within inpatient psychiatric services: a review of the Literature. Int J Ment Health Nurs. (2012) 21:301–9. doi: 10.1111/j.1447-0349.2011.00794.x
62. Dumais A, Larue C, Drapeau A, Ménard G, Giguère Allard M. Prevalence and correlates of seclusion with or without restraint in a Canadian psychiatric hospital: a 2-year retrospective audit. J Psychiatr Ment Health Nurs. (2011) 18:394–402. doi: 10.1111/j.1365-2850.2010.01679.x
63. Bowers L, Van Der Merwe M, Nijman H, Hamilton B, Noorthorn E, Stewart D. The practice of seclusion and time-out on English acute psychiatric wards: the City-128 Study. Arch Psychiatr Nurs. (2010) 24:275–86. doi: 10.1016/j.apnu.2009.09.003
64. Migon MN, Coutinho ES, Huf G, Adams CE, Cunha GM, Allen MH. Factors associated with the use of physical restraints for agitated patients in psychiatric emergency rooms. Gen Hosp Psychiatry. (2008) 30:263–8. doi: 10.1016/j.genhosppsych.2007.12.005
65. Zhu XM, Xiang YT, Zhou JS, Gou L, Himelhoch S, Ungvari GS. Frequency of physical restraint and its associations with demographic and clinical characteristics in a Chinese psychiatric institution. Perspect Psychiatr Care. (2014) 50:251–6. doi: 10.1111/ppc.12049
66. Hui H, Middleton B, Völlm B. The Uses of Coercive Measures in Forensic Psychiatry: A Literature Review In: Völlm B, Nedopil N, editors The Use of Coercive Measures in Forensic Psychiatric Care: Legal, Ethical and Practical Challenges. Cham: Springer International Publishing (2016), pp. 151–84.
67. Goethals KR, Vorstenbosch EC, van Marle HJ. Diagnostic comorbidity in psychotic offenders and their criminal history: a review of the literature. Int J Forensic Ment Health. (2008) 7:147–56. doi: 10.1080/14999013.2008.9914411
68. Putkonen A, Kotilainen I, Joyal CC, Tiihonen J. Comorbid personality disorders and substance use disorders of mentally ill homicide offenders: a structured clinical study on dual and triple diagnoses. Schizophr Bull. (2004) 30:59–72. doi: 10.1093/oxfordjournals.schbul.a007068
69. Baranyi G, Scholl C, Fazel S, Patel V, Priebe S, Mundt AP. Severe mental illness and substance use disorders in prisoners in low-income and middle-income countries: a systematic review and meta-analysis of prevalence studies. The Lancet Global Health. (2019) 7:e461–71. doi: 10.1016/S2214-109X(18)30539-4
70. Baranyi G, Fazel S, Langerfeldt SD, Mundt AP. The prevalence of comorbid serious mental illnesses and substance use disorders in prison populations: a systematic review and meta-analysis. The Lancet Public Health. (2022) 7:e557–68. doi: 10.1016/S2468-2667(22)00093-7
71. Fazel S, Långström N, Hjern A, Grann M, Lichtenstein P. Schizophrenia substance abuse, and violent crime. JAMA. (2009) 301:2016–23. doi: 10.1001/jama.2009.675
72. Wu Y, Kang R, Yan Y, Gao K, Li Z, Jiang J, et al. Epidemiology of schizophrenia and risk factors of schizophrenia-associated aggression from 2011 to 2015. J Int Med Res. (2018) 46:4039–49. doi: 10.1177/0300060518786634
73. Ellouze F, Ayedi S, Masmoudi S, Bakri L, Chérif W, Zramdini R. Schizophrenia and violence, incidence and risk factors: a Tunisian sample. Encephale. (2009) 35:347–52. doi: 10.1016/j.encep.2008.04.006
75. Fazel S, Yu R. Psychotic disorders and repeat offending: systematic review and meta-analysis. Schizophr Bull. (2011) 37:800–10. doi: 10.1093/schbul/sbp135
76. Iniesta R, Stahl D, McGuffin P. Machine learning, statistical learning and the future of biological research in psychiatry. Psychol Med. (2016) 46:2455–65. doi: 10.1017/S0033291716001367
77. Oquendo MA, Baca-Garcia E, Artés-Rodríguez A, Perez-Cruz F, Galfalvy HC, Blasco-Fontecilla H. Machine learning and data mining: strategies for hypothesis generation. Mol Psychiatry. (2012) 17:956–9. doi: 10.1038/mp.2011.173
Keywords: schizophrenia spectrum disorders, offender patients, forensic psychiatry, PANSS, symptom severity, offending
Citation: Machetanz L, Hofmann AB, Möhrke J and Kirchebner J (2023) Offenders and non-offenders with schizophrenia spectrum disorders: the crime-preventive potential of sufficient embedment in the mental healthcare and support system. Front. Psychiatry 14:1231851. doi: 10.3389/fpsyt.2023.1231851
Received: 31 May 2023; Accepted: 04 August 2023;
Published: 30 August 2023.
Edited by:
Giovanna Parmigiani, Sapienza University of Rome, ItalyReviewed by:
Gabriele Nibbio, University of Brescia, ItalyCopyright © 2023 Machetanz, Hofmann, Möhrke and Kirchebner. This is an open-access article distributed under the terms of the Creative Commons Attribution License (CC BY). The use, distribution or reproduction in other forums is permitted, provided the original author(s) and the copyright owner(s) are credited and that the original publication in this journal is cited, in accordance with accepted academic practice. No use, distribution or reproduction is permitted which does not comply with these terms.
*Correspondence: Lena Machetanz, bGVuYS5ob2ZtYW5uQHB1a3poLmNo
Disclaimer: All claims expressed in this article are solely those of the authors and do not necessarily represent those of their affiliated organizations, or those of the publisher, the editors and the reviewers. Any product that may be evaluated in this article or claim that may be made by its manufacturer is not guaranteed or endorsed by the publisher.
Research integrity at Frontiers
Learn more about the work of our research integrity team to safeguard the quality of each article we publish.