- 1Department of Neuropsychiatry, Kyushu University Hospital, Fukuoka, Japan
- 2Department of Neuropsychiatry, Graduate School of Medical Sciences, Kyushu University, Fukuoka, Japan
- 3Integrated Center for Educational Research and Development, Faculty of Education, Saga University, Saga, Japan
- 4Graduate School of Human-Environment Studies, Kyushu University, Fukuoka, Japan
- 5Center for Health Sciences and Counseling, Kyushu University, Fukuoka, Japan
Background: Patients with obsessive-compulsive disorder (OCD) have deficits in decision-making in the Iowa Gambling Task (IGT). However, no study has investigated the parameters of the prospect valence learning (PVL) model in the IGT for OCD.
Aims: This study aimed to investigate deficits in decision-making in OCD using the PVL model and identify whether the parameters of the PVL model were associated with obsessive-compulsive severity.
Methods: Forty-seven medication-free patients with OCD were compared with 47 healthy controls (HCs). Decision-making was measured using the total net and block net scores of the IGT. A PVL model with a decay-reinforcement learning rule (PVL-DecayRI) was used to investigate the parameters of the model. Correlation analysis was conducted between each parameter of the PVL-DecayRL and obsessive-compulsive symptoms.
Results: The total net score of patients with OCD was significantly lower than that of the HCs. The block net scores of the OCD group did not differ across the five blocks, whereas in the HCs, the fifth block net score was significantly higher than the block net scores of the first and second blocks. The values of the recency and response consistency parameters of the PVL-DecayRI in patients with OCD were significantly lower than those in HCs. The recency parameter positively correlated with the Y-BOCS obsessive score. Meanwhile, there was no correlation between consistency parameter values and symptom severity in OCD.
Conclusion: Our detailed analysis of the decision-making deficit in OCD suggests that the most recent outcome has a small influence on the expectancy of prospect valence, as indicated by the lower recency parameter, and is characterized by more impulsive choices, as indicated by the lower consistency parameter.
1. Introduction
Obsessive-compulsive disorder (OCD), according to the Diagnostic and Statistical Manual of Mental Disorders, Fifth Edition (DSM-5), is “characterized by recurrent, intrusive, and distressing thoughts (obsession) and repetitive behaviors or mental acts (compulsions) that are executed to avoid anxiety or neutralize obsessions” (1). Several studies have shown that OCD is associated with cognitive impairment (2, 3). Some domains of cognitive impairment, decision-making, inhibition, and planning have been reported as candidates for the endophenotype of OCD (4, 5). A deficit in decision-making refers to the difficulty in selecting an option from various choices that yield different consequences (6). Obsessive-compulsive symptoms include deficits in decision-making because seeking immediate relief from negative emotions such as anxiety and disgust through compulsive behavior results in long-term functional problems (7–9).
There are two types of decision-making in some situations (10). The first includes decisions under risk, and the second includes decisions under ambiguity (10). The former is a decision situation in which information on the results of diverse choices and their prospects is provided. The latter, which is usually measured by the Iowa Gambling Task (IGT) (11), requires decision-makers to find information by themselves by processing feedback of the previous choice in a situation in which the outcome and probabilities are implied but not directly expressed.
The IGT is a task in which participants select cards to win prizes. It required the participants to select one deck from four decks (A, B, C, and D). Decks A and B are disadvantageous decks that cause greater losses than gains. The other decks (C and D) are advantageous, making more gains than losses. Although normal decision-makers select advantageous decks more often than disadvantageous decks as trials progress, patients with OCD do not show this phenomenon (12–16). Therefore, patients with OCD are considered to have deficits in decision-making (4, 15–18).
IGT consists of complex interactions among motivational, cognitive, and response processes (19). Therefore, deficits in decision-making in specific participant groups may result in different component processes in the IGT (19). Some cognitive models have examined the mechanisms of the psychological processes involved in IGT. Two of these, the expectancy-valence learning model (EVL) (20) and the prospect valence learning model (PVL) (21), were successfully fitted with empirical data (19). The EVL model of IGT performance assumes three processes, namely, motivation, memory/learning, and response consistency (22). The motivational parameter shows how the participant integrates the gains and losses of all previous trials and forms an expected valence for the present trial, which depends on the probability of a subsequent trial (22, 23). The memory/learning parameter indicates that the expectancy of a deck in a given trial is the sum of the expectancy of the deck in a previous trial and the difference between the valence of the present choice and that of the deck in a previous trial (22, 23). Response consistency shows the degree of consistency in making decisions based on the expectation of valence for each deck or whether the participants chose decks randomly (22, 23).
The PVL model, which is a modified EVL model proposed by Ahn et al. (21), has four parameters, namely, feedback sensitivity, loss aversion, recency, and response consistency. The motivation component of the EVL model is separated into two parameters as follows: feedback sensitivity and loss aversion (22). Since the PVL model employs a non-linear utility function and effectively explains the impact of gain–loss frequency on the formation of expectancy for each deck, it would be more effective than the EVL model, which uses a linear function for analyzing IGT performance (21). Feedback sensitivity, indicated by the non-linear model, shows the association between the total of gains and losses and prospect valence. A higher feedback sensitivity score indicates that the subjective estimation is more closely related to the actual amount of gains and losses. The loss aversion parameter indicates the inclination to avoid loss. The recency parameter refers to the shaping or adjustment of a preference for each deck, formed by recent outcomes with gains or losses. A low recency parameter indicates that the value of the most recent card choice has a small effect on the expectancy of the deck, and forgetting is more progressive. The response consistency parameter indicates the consistency of the participants' choice behavior. A low response consistency parameter indicates that the participant chose more randomly.
Several clinical studies have used the PVL model to analyze IGT performance. For example, chronic cannabis use was significantly lower in four parameters, especially in loss aversion and consistency, compared with healthy controls (24). All four parameters were significantly lower in patients with schizophrenia than in healthy controls (25). Patients with anorexia nervosa have a significantly lower recency parameter (22), and female students at high risk for anorexia nervosa have a lower recency parameter and response consistency than healthy controls (23). In patients with a major depressive episode with a suicide attempt, the loss aversion and recency parameters were lower than those in controls (26).
Mathematical models allow the decomposition of IGT performance into distinct components: feedback sensitivity, loss aversion, learning (recency), and response processes. This approach enables a comprehensive analysis of the underlying decision-making processes and characterization of decision-making deficits in individuals with OCD. However, to the best of our knowledge, no study has explored these aspects of the PVL model using IGT among individuals with OCD in comparison with healthy controls (HCs).
This study aimed to explore which parameters of the PVL model in IGT showed differences between patients with OCD and healthy controls and identify whether the parameters of the PVL model were associated with obsessive-compulsive severity. Our hypothesis postulated that IGT performance among individuals with OCD would be poorer than HCs. This can be attributed to reduced feedback sensitivity, recency, and consistency parameters among individuals with OCD. Moreover, we hypothesized that the loss aversion parameter in individuals with OCD would be significantly higher compared with HCs. Several previous studies have indicated that individuals with OCD demonstrate higher avoidance learning and loss aversion (27–30).
2. Materials and methods
2.1. Participants
Forty-seven medication-free patients with OCD (OCD) and 47 HC were recruited. All individuals diagnosed with OCD were recruited from the outpatient unit in the Department of Neuropsychiatry, Kyushu University Hospital, from February 2016 to September 2021. All patients with OCD were diagnosed using the Structured Clinical Interview for Diagnostic and Statistical Manual of Mental Disorders, Fourth Edition (DSM-IV), Axis I disorders (patient version) and fulfilled the DSM-IV criteria. None of the patients had any current comorbid Axis I disorders. We also verified that all patients met the criteria for OCD in the Diagnostic and Statistical Manual of Mental Disorders, Fifth Edition (DSM-5). All OCD participants had not taken any psychiatric medication for at least 4 weeks. These procedures were performed on the same day as the IGT assessment. The HCs were recruited from local communities. They were assessed using the Structured Clinical Interview for DSM-IV (non-patient version). The participants had no history of psychiatric disorders, head injury, or epilepsy.
This study was conducted according to the principles of the Declaration of Helsinki and was approved by the Kyushu University Ethics Committee. All participants provided written informed consent prior to the commencement of the study.
2.2. Clinical assessment
The severity of OCD symptoms was measured by the Japanese version of the Yale–Brown Obsessive-Compulsive Scale (Y-BOCS). The Hamilton Rating Scales of Anxiety (HAM-A) (31) and Depression (HAM-D) (32) were used to assess anxiety and depression, respectively. The Japanese version of the National Adult Reading Test (33) measures the estimated intelligence quotient (IQ).
2.3. Iowa gambling task
We used the Japanese version of the IGT in the present study. Its only difference from the original IGT, which is presented by Bechara (11), is that the play money is in Japanese yen instead of US dollars. The participants chose one card at a time from four decks of cards labeled A, B, C, and D. They were told that they had a loan of JPY 200,000 (fictitious money) available at the start of the task, and they selected cards from any deck at their own pace. Decks A and B incur net losses over time. Although they provide larger immediate rewards (+10,000 yen for every card), they also impose larger penalties (a penalty of −125,000 yen after picking 10 cards). Deck A has five losses per 10 cards and Deck B has one loss per 10 cards. In contrast, decks C and D have total rewards (+5000 yen on every card) that are greater than the penalties (total penalty of −25,000 yen after picking 10 cards; deck C has five losses per 10 cards and deck D has one loss per 10 cards). The participants were not informed about the risks of each deck or the number of selections allowed. One hundred cards were selected to complete the task, which were subsequently divided into five blocks of 20 card selections.
Decision-making was evaluated using the total net score and the net score of each block. The net score was computed by subtracting the number of cards in each block of 20 card selections from the total number of cards. To investigate decision-making changes over time, we calculated the net score of each block and the total blocks.
2.4. Prospect valence learning model
The PVL model is comprised of two models based on the learning model. One is the PVL model with a delta-learning rule (PVL-Delta) which uses the Rescorla-Wagner rule (34), and the other is the PVL model with a decay-reinforcement learning rule (PVL-DecayRI). Several studies reported that the PVL-DecayRI had better post-hoc model fits than the delta rule on the IGT (21, 35, 36); therefore, this study adopted the PVL-DecayRI.
The explanation of the PVL-DecayRI, which is described below, has been cited in previous studies (21–25, 37, 38).
The above calculation shows how a participant's subjective expectancy valence [u (t)] is developed. Here, x (t) is the net gain, which is defined as win (t) – | lose (t)| in trial t, and α determines the feedback sensitivity. It has a value of zero to one and shows the relationship between the actual net gain and expectancy valence. As the value approaches zero, the individual's expectancy valence is not influenced by the actual number of gains or losses. If the value goes to one, the expectancy valence is sensitively influenced by the alternation of the actual amount of gains/losses. In this equation, λ is a loss aversion parameter, which ranges from zero to five and shows a tendency to respond sensitively to losses relative to gains. If λ = 0, the participant does not judge it as a loss at all. If λ = 1, the participant judges the losses and gains are equal, whereas if λ > 1, the participant tends to pay more attention to the losses than gains in the formation.
According to the PVL model, expectancy valence is affected by learning. This implies that the experience of gain or loss in the early trials influenced the expectancy valence of the participants. The recency parameter, which is the learning parameter in PVL-DecayRI, is calculated using the following equation:
In the above calculation, Ej (t) shows the expectancy for deck j on trial t. A is the recency parameter, which determines the earlier expectancy valence of a selected deck j on trial t. Ij (t) is a dummy variable. It is coded 1 if j card is selected in tth trial. If j card is not selected, Ij (t) is coded as zero. The recency parameter (0 < A < 1) indicates the extent to which the expected values of all decks are discounted in each trial. A low value of A means rapid forgetting and a strong recency effect, whereas a high A value indicates good learning/less memory decay.
The response consistency parameter is calculated using the following equation:
D (t +1) is defined as the deck selected in the next trial, t + 1. The probability that deck j is selected for the next trial is given by Pr [D (t+1) = j]. Parameter θ (t) shows the degree of selection of the decks, which is formed by the expectancy valence. The PVL model adapts a trial-dependent choice rule which is showed θ (t) = 3c-1. The response consistency parameter c ranges from zero to five. If c approaches zero, the participant makes a random selection, whereas if c approaches five, the participant makes a consistent deck selection based on expectancy.
2.5. Statistical analysis
Tests were conducted using SPSS version 28.0.1.0. Demographic and clinical data were analyzed using chi-square test, Student's t-test, and Welch's t-test. To examine IGT performance, a two-way repeated measures ANOVA was performed as the between-subject factor and block as the within-subject factor. The Bonferroni correction was used for post-hoc analyses. Spearman's correlation test was used to examine the correlation between the total net score for OCD and the severity of OCD symptoms.
To estimate PVL parameters, a Markov Chain Monte Carlo sampling scheme was used in OpenBUGS, and BRugs was used (39). The PVL parameters were analyzed using a Mann-Whitney U test. Correlations between PVL parameters and the severity of OCD symptoms were analyzed using the Spearman correlation coefficient test.
3. Results
3.1. Demographic characteristics
There were no significant differences in sex [χ2 (1) = 0.05; p = 0.826], age [t (92) = 0.18; p = 0.857], and estimated verbal IQ [t (92) = −1.48; p = 0.143] between the patients with OCD and HCs. However, HAM-D [t (66.74) = 5.15; p = 0.000] and HAM-A [t (52.51); p = 0.000] significantly differed. The mean Y-BOCS score in the OCD group was 23.81 (standard deviation, 5.97). Table 1 shows the mean scores of the demographic characteristics and clinical symptoms.
3.2. IGT performance
The total net score of the OCD group was significantly lower than that of the HC group [t (92) = −3.54, p < 0.001, r = 0.120] (Table 2). We conducted a two-factor repeated measures ANOVA on the conditions, and the results showed a significant difference in the group factor [F(1, 92) = 12.54, p = 0.001, partial η2 = 0.120], block factor [F(4, 368) = 3.11, p = 0.015, partial η2 = 0.033], and interaction effects (F(4, 368) = 2.73, p = 0.029, partial η2 = 0.032]. Figure 1 shows the changes in net scores in the OCD and HC groups. In the main effect of the block factor, the net score of block 5 was significantly higher than that of block 1 (p = 0.019) using Bonferroni corrections. In the interaction, the simple main effects of the group factor showed significant differences: the net score of block 3 (OCD < HC, p = 0.012, partial η2 = 0.067), block 4 (OCD < HC, p < 0.001, partial η2 = 0.120), and block 5 (OCD < HC, p = 0.002, partial η2 = 0.098). In the HC, the simple main effect between blocks was significantly different [F(4, 89) = 4.95, p = 0.001, partial η2 = 0.182]. The post-hoc comparison using Bonferroni corrections in the HC group showed that the net score of block 5 was significantly higher than that of blocks 1 (p = 0.03) and 2 (p = 0.003). Meanwhile, in OCD, there were no significant differences in the simple main effect between each block [F(4, 89) = 0.54, p = 0.709, partial η2 = 0.0249].
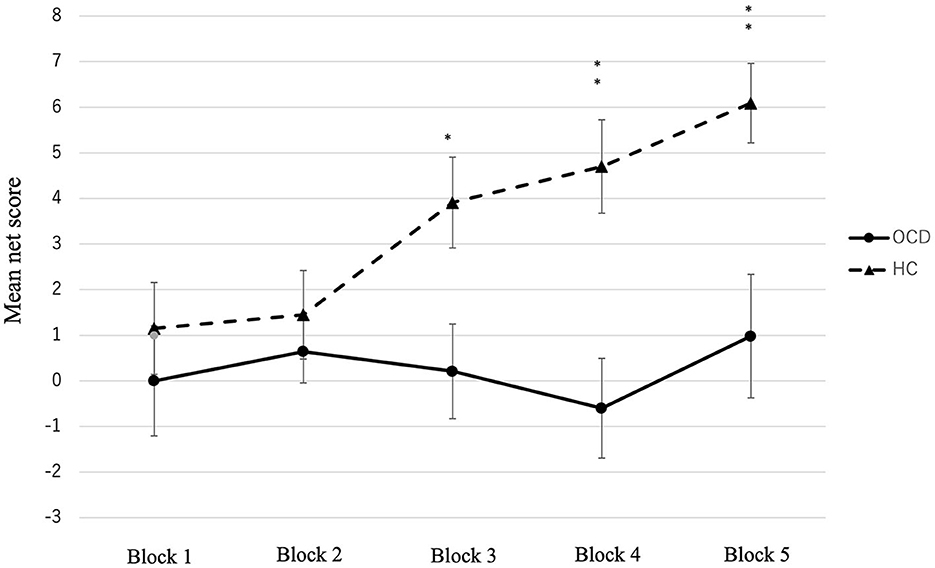
Figure 1. Mean net score of the five blocks in the OCD and HC. Significant differences were observed between the two groups in blocks 3, 4, and 5. *p < 0.05, **p < 0.001, ***p < 0.0001.
There was no significant correlation between the total net score and the obsessive Y-BOCS score (r = 0.160, p = 0.273), compulsive Y-BOCS score (r = 0.226, p = 0.119), or total Y-BOCS score (r = 0.222, p = 0.126).
3.3. PVL model parameters
Patients with OCD showed lower feedback sensitivity (U = 1373.5, p = 0.042, r = 0.21), recency (U = 17770.0, p = 0.000, r = 0.52), and response consistency (U = 1625.0, p = 0.000, r = 0.41) than the HCs. There was no significant difference in loss aversion (U = 1361.5) parameter between the two groups. The mean PVL parameter values in patients with OCD and HCs are shown in Table 3 and Figure 2.
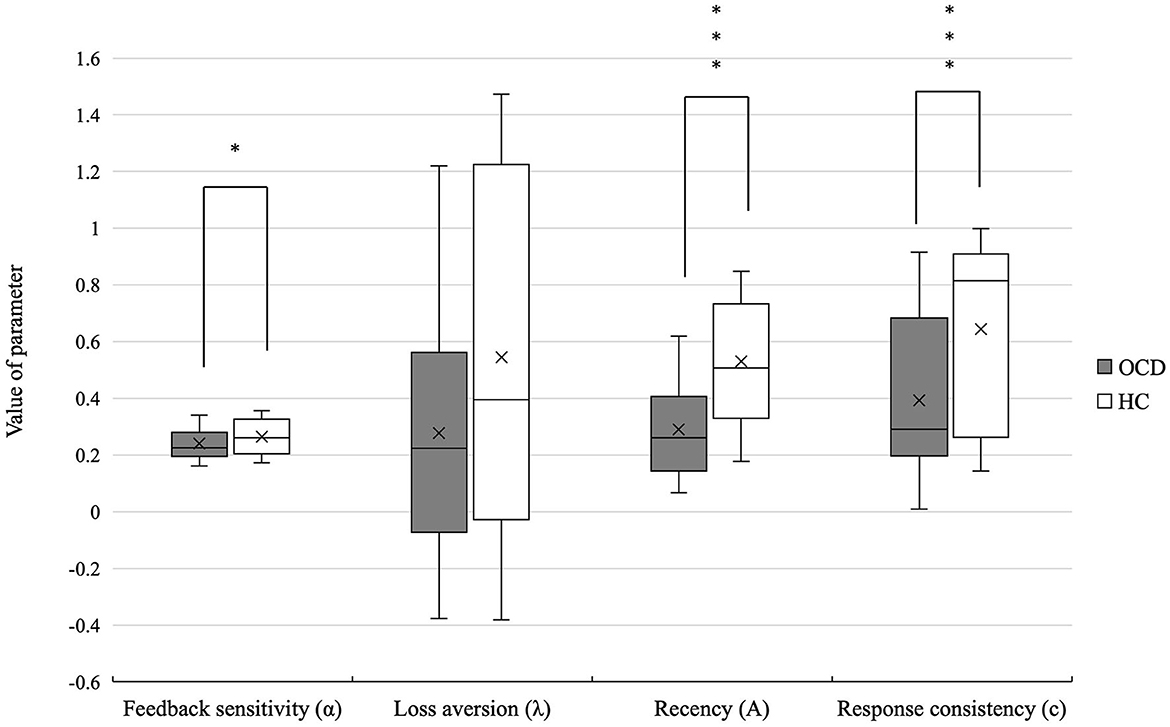
Figure 2. Differences in the PVL-DecayRI model parameter of OCD and HCs. Significant differences were observed between the two groups in feedback sensitivity, recency, and response consistency. *p < 0.05, ***p < 0.001.
Figure 3 shows that there was a positive correlation between the recency parameter and Y-BOCS obsession score (r = 0.334, p = 0.022). No correlations were observed between the other PVL parameters and OCD symptom severity (see Figure 4). The correlation coefficients are presented in Table 4.
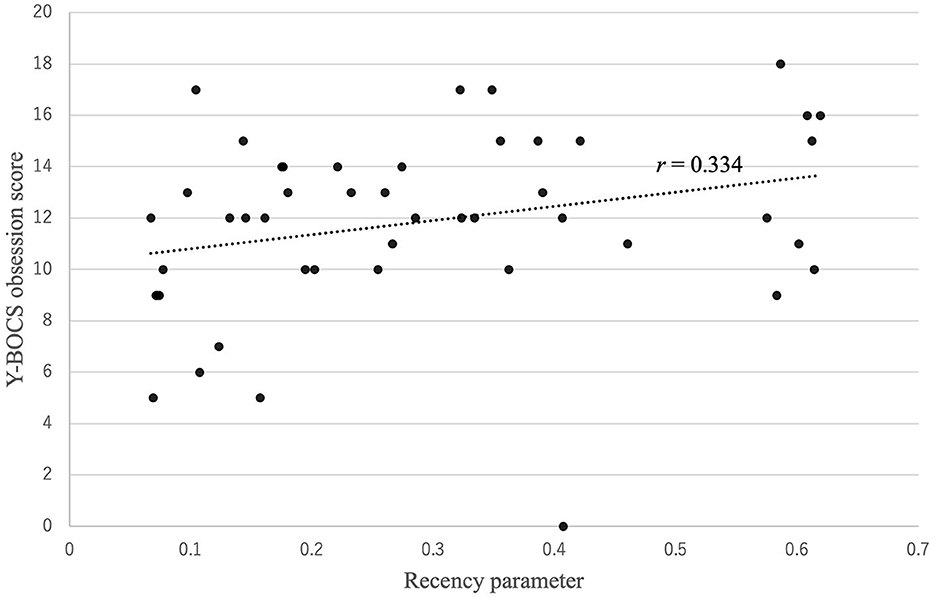
Figure 3. Correlation between the recency parameter and Y-BOCS obsession score. There was a positive correlation between the recency parameter and Y-BOCS obsession score: r (47) = 0.334, p = 0.022.
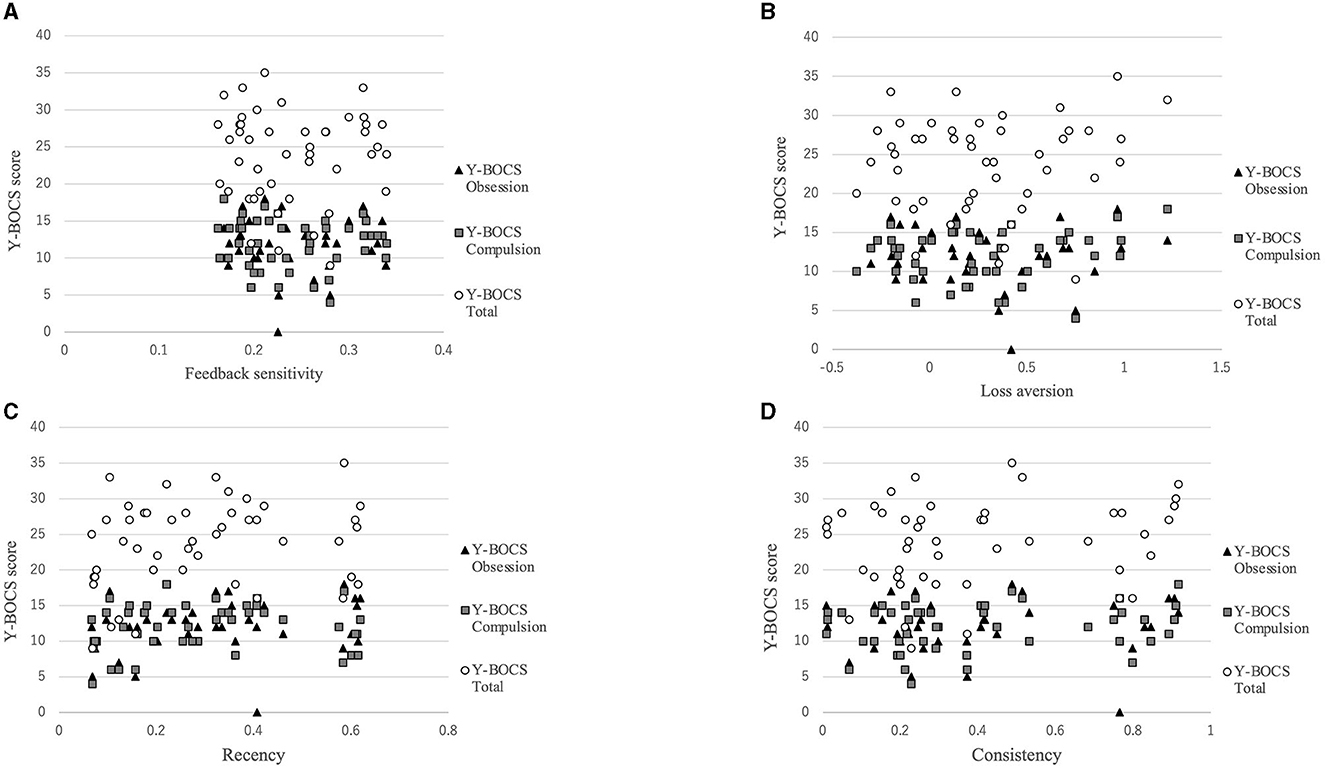
Figure 4. Correlations between symptom severity and each parameter for the PVL-DecayRI. Except for that between the Y-BOCS obsession score and recency parameter, no correlation was observed between the Y-BOCS scores and the other parameters for the PVL-DecayRL. (A) Feedback sensitivity parameter; (B) loss aversion parameter; (C) recency parameter; and (D) consistency parameter.
4. Discussion
We found that patients with OCD had a deficit in decision-making and significantly lower recency and response consistency parameters than those in the HC group. However, the loss aversion parameter was not significantly different between patients with OCD and HC. In line with a previous meta-analysis (15), patients with OCD showed significantly lower total net scores than HC, no increase in net scores as the trials progressed, and no correlation between the total net score and symptom severity. Value-based decision-making, including the IGT, incorporates emotion-based learning and interoception (40, 41). IGT performance encompasses both emotional and cognitive processes, which are facilitated by reward- and punishment-related motivation (42). Individuals with OCD encounter challenges in emotion regulation (43) and deficits in interoception (44, 45) and exhibit impaired reward generalization (46). Therefore, the OCD group exhibited poorer IGT performance compared with the HC group.
The lower recency and response consistency parameters in the OCD group indicate that patients with OCD had difficulty updating the current outcome and selected the cards randomly. Therefore, patients with OCD either have deficits in learning about eventuality-controlling options (25, 47) or experience a rapid decline in previously learned information (25, 48). In addition, patients with OCD may impulsively choose cards throughout the five blocks (25, 48).
These results may be due to structural and functional brain abnormalities associated with OCD. Previous studies have revealed that the brain regions related to IGT performance in IGT are the orbitofrontal cortex (OFC) (49–51), dorsolateral prefrontal cortex (51, 52), ventromedial prefrontal cortex (51–54), anterior cingulate cortex (52), and parietal cortex (52). Additionally, striatal circuits are associated with learning and decision-making (55). Patients with OCD exhibit structural and functional abnormalities (56–61) in these brain regions. Moreover, the working memory (WM) deficits in patients (62–64) might be associated with the results of this study. The WM is the cognitive system used to hold some amount of information as the focus of attention (65, 66) and is associated with IGT performance (66–68). The prefrontal and parietal cortices are involved in WM (69–71), and patients with OCD exhibit dysfunction and structural abnormalities in these regions (56, 61, 72). Thus, these abnormalities in the brain regions may involve lower values of recency and consistency parameters.
The reduced feedback sensitivity observed in the OCD group suggests that the subjective evaluation of deck selection outcomes among OCD patients was not significantly influenced by the actual amount of gains or losses. This could potentially indicate a deficit in the reward processing system, linked to the disruption of frontostriatal circuits in OCD (73, 74). However, the effect size of this difference was very small. Therefore, the results should be interpreted with caution.
Contrary to our initial hypothesis suggesting a higher loss aversion parameter among OCD patients than HCs, the loss aversion parameter in the OCD group was not significantly different from that of HCs. This finding indicates that the lower IGT performance in individuals with OCD does not involve loss aversion. Interestingly, certain addictive disorders associated with compulsive behaviors (75), such as Internet gambling disorder, pathological gambling disorder, and alcohol disorder, demonstrated lower performance on value-based decision-making and lower loss aversion parameters of the prospect theory compared with HCs (76, 77). Although no direct correlation was established between the loss aversion parameter and severity of compulsivity in this study, compulsivity could influence our results. Further research is required to validate our results and hypotheses.
We found no correlation between the total net score and symptom severity in patients with OCD. These results are supported by a previous meta-analysis (4), which proposed that the deficit in decision-making might be an endophenotype of OCD. Interestingly, in this study, the value of the recency parameter in patients with OCD, which was lower than that in HC, was positively correlated with the Y-BOCS obsessive score. In other words, the more severe the obsession OCD patients have, the larger the influence on the expectancy of the most recent outcome. Thus, the lower recency parameter may be a state, but not a trait, of OCD. Conversely, the value of the consistency parameter, which was lower than that of HCs, did not correlate with symptom severity. As mentioned above, the lower consistency parameter in patients with OCD indicates that OCD patients might make impulsive choices under ambiguous decision-making conditions (25, 48). Some studies have found that impulsivity and compulsivity may be involved in common psychological and neurobiological mechanisms (78–81) and have proposed that impulsivity might be a feature of OCD (82, 83). Therefore, the lower consistency parameter in patients with OCD may reflect their trait nature.
This study has some limitations. First, we did not examine variables such as reward sensitivity, approach-avoidance motivational tendencies, or interoception, all of which affect IGT performance. Therefore, our findings could be influenced by these unexplored factors. Second, we did not identify the best-fitting model by comparing it with other cognitive models, such as the Value-Plus Perseverance model (84) and outcome representation learning model (85). However, this study aimed to compare the values of PVL-DecayRI parameters between patients with OCD and HCs. Future studies are needed to develop the best-fitting model for IGT in OCD. Third, we did not employ neuroimaging techniques; nevertheless, we mentioned some brain abnormalities in patients with OCD in the Discussion section. Fourth, we did not examine aspects of OCD heterogeneity such as age at onset (86–88), duration of illness (89), and OCD dimensional symptoms (90, 91). Fifth, we did not investigate other neuropsychological functions associated with IGT, such as working memory, attention, and set-shifting. Despite these limitations, we found new information regarding decision-making in OCD. As mentioned above, some studies have proposed that decision-making may be one of the candidates for the endophenotype of OCD (4). In future, we hope to address the limitations of this study and conduct a study to investigate the parameters within a computational model for individuals who have relatives with OCD to determine whether the same parameters, observed in individuals with OCD, are also involved in their reduced IGT performance (4).
5. Conclusion
Patients with OCD had significantly lower total net scores than HC, and there was no increase in net scores as the trials progressed on the IGT. Application of the PVL-DecayRI model showed that patients with OCD had lower recency and response consistency parameters than the HC group. These findings indicate that a deficit in decision-making in OCD may occur because the most recent outcome has a small effect on expectancy, thereby contributing to a computational decision-making model for patients with OCD.
Data availability statement
The original contributions presented in the study are included in the article/supplementary material, further inquiries can be directed to the corresponding author.
Ethics statement
The studies involving humans were approved by Kyushu University Ethics Committee. The studies were conducted in accordance with the local legislation and institutional requirements. The participants provided their written informed consent to participate in this study.
Author contributions
KM designed the study, collected the data, and wrote the initial draft of the manuscript. HT collected the data, designed the study, and critically reviewed the manuscript. AO, MK, and KS contributed to the data analysis and interpretation. SH, KK, and AM contributed to data collection. TN critically reviewed the manuscript. All authors approved the final version of the manuscript.
Funding
This study was supported by the JSPS KAKENHI, grant numbers JP16K10253, JP19K08076, and JP22K07598.
Acknowledgments
The authors would like to thank Editage (www.editage.com) and Ms. Katherine Ono for English language editing.
Conflict of interest
The authors declare that the research was conducted in the absence of any commercial or financial relationships that could be construed as a potential conflict of interest.
Publisher's note
All claims expressed in this article are solely those of the authors and do not necessarily represent those of their affiliated organizations, or those of the publisher, the editors and the reviewers. Any product that may be evaluated in this article, or claim that may be made by its manufacturer, is not guaranteed or endorsed by the publisher.
References
1. Association AP. Diagnostic and Statistical Manual of Mental Disorders: Fifth Edition (Dsm-5). Washington, DC: APA (2013).
2. Abramovitch A, Abramowitz JS, Mittelman A. The neuropsychology of adult obsessive-compulsive disorder: a meta-analysis. Clin Psychol Rev. (2013) 33:1163–71. doi: 10.1016/j.cpr.2013.09.004
3. Shin NY, Lee TY, Kim E, Kwon JS. Cognitive functioning in obsessive-compulsive disorder: a meta-analysis. Psychol Med. (2014) 44:1121–30. doi: 10.1017/S0033291713001803
4. Bora E. Meta-analysis of neurocognitive deficits in unaffected relatives of obsessive-compulsive disorder (OCD): comparison with healthy controls and patients with OCD. Psychol Med. (2020) 50:1257–66. doi: 10.1017/S0033291720001634
5. Chamberlain SR, Blackwell AD, Fineberg NA, Robbins TW, Sahakian BJ. The neuropsychology of obsessive compulsive disorder: the importance of failures in cognitive and behavioural inhibition as candidate endophenotypic markers. Neurosci Biobehav Rev. (2005) 29:399–419. doi: 10.1016/j.neubiorev.2004.11.006
6. Lee D. Decision making: from neuroscience to psychiatry. Neuron. (2013) 78:233–48. doi: 10.1016/j.neuron.2013.04.008
7. Cavedini P, Gorini A, Bellodi L. Understanding obsessive-compulsive disorder: focus on decision making. Neuropsychol Rev. (2006) 16:3–15. doi: 10.1007/s11065-006-9001-y
8. Sachdev PS, Malhi GS. Obsessive-compulsive behaviour: a disorder of decision-making. Aust N Z J Psychiatry. (2005) 39:757–63. doi: 10.1080/j.1440-1614.2005.01680.x
9. Pushkarskaya H, Tolin D, Ruderman L, Kirshenbaum A, Kelly JM, Pittenger C, et al. Decision-making under uncertainty in obsessive-compulsive disorder. J Psychiatr Res. (2015) 69:166–73. doi: 10.1016/j.jpsychires.2015.08.011
10. Brand M, Labudda K, Markowitsch HJ. Neuropsychological correlates of decision-making in ambiguous and risky situations. Neural Netw. (2006) 19:1266–76. doi: 10.1016/j.neunet.2006.03.001
11. Bechara A, Damasio AR, Damasio H, Anderson SW. Insensitivity to future consequences following damage to human prefrontal cortex. Cognition. (1994) 50:7–15. doi: 10.1016/0010-0277(94)90018-3
12. Cavedini P, Riboldi G, D'Annucci A, Belotti P, Cisima M, Bellodi L. Decision-making heterogeneity in obsessive-compulsive disorder: ventromedial prefrontal cortex function predicts different treatment outcomes. Neuropsychologia. (2002) 40:205–11. doi: 10.1016/S0028-3932(01)00077-X
13. Starcke K, Tuschen-Caffier B, Markowitsch HJ, Brand M. Dissociation of decisions in ambiguous and risky situations in obsessive-compulsive disorder. Psychiatry Res. (2010) 175:114–20. doi: 10.1016/j.psychres.2008.10.022
14. Cavedini P, Zorzi C, Piccinni M, Cavallini MC, Bellodi L. Executive dysfunctions in obsessive-compulsive patients and unaffected relatives: searching for a new intermediate phenotype. Biol Psychiatry. (2010) 67:1178–84. doi: 10.1016/j.biopsych.2010.02.012
15. Nistico V, De Angelis A, Erro R, Demartini B, Ricciardi L. Obsessive-compulsive disorder and decision making under ambiguity: a systematic review with meta-analysis. Brain Sci. (2021) 11:143. doi: 10.3390/brainsci11020143
16. Zhang L, Dong Y, Ji Y, Tao R, Chen X, Ye J, et al. Trait-related decision making impairment in obsessive-compulsive disorder: evidence from decision making under ambiguity but not decision making under risk. Sci Rep. (2015) 5:17312. doi: 10.1038/srep17312
17. Kim HW, Kang JI, Namkoong K, Jhung K, Ha RY, Kim SJ. Further evidence of a dissociation between decision-making under ambiguity and decision-making under risk in obsessive-compulsive disorder. J Affect Disord. (2015) 176:118–24. doi: 10.1016/j.jad.2015.01.060
18. Zhang L, Dong Y, Ji Y, Zhu C, Yu F, Ma H, et al. Dissociation of decision making under ambiguity and decision making under risk: a neurocognitive endophenotype candidate for obsessive-compulsive disorder. Prog Neuropsychopharmacol Biol Psychiatry. (2015) 57:60–8. doi: 10.1016/j.pnpbp.2014.09.005
19. Dai J, Kerestes R, Upton DJ, Busemeyer JR, Stout JC. An improved cognitive model of the Iowa and soochow gambling tasks with regard to model fitting performance and tests of parameter consistency. Front Psychol. (2015) 6:229. doi: 10.3389/fpsyg.2015.00229
20. Busemeyer JR, Stout JC. A contribution of cognitive decision models to clinical assessment: decomposing performance on the bechara gambling task. Psychol Assess. (2002) 14:253–62. doi: 10.1037/1040-3590.14.3.253
21. Ahn WY, Busemeyer JR, Wagenmakers EJ, Stout JC. Comparison of decision learning models using the generalization criterion method. Cogn Sci. (2008) 32:1376–402. doi: 10.1080/03640210802352992
22. Chan TW, Ahn WY, Bates JE, Busemeyer JR, Guillaume S, Redgrave GW, et al. Differential impairments underlying decision making in anorexia nervosa and bulimia nervosa: a cognitive modeling analysis. Int J Eat Disord. (2014) 47:157–67. doi: 10.1002/eat.22223
23. Na E, Kang B, Kim MS. Decision-making deficits are associated with learning impairments in female college students at high risk for anorexia nervosa: Iowa gambling task and prospect valence learning model. Front Psychiatry. (2018) 9:759. doi: 10.3389/fpsyt.2018.00759
24. Fridberg DJ, Queller S, Ahn WY, Kim W, Bishara AJ, Busemeyer JR, et al. Cognitive mechanisms underlying risky decision-making in chronic cannabis users. J Math Psychol. (2010) 54:28–38. doi: 10.1016/j.jmp.2009.10.002
25. Kim MS, Kang BN, Lim JY. Decision-making deficits in patients with chronic schizophrenia: Iowa gambling task and prospect valence learning model. Neuropsychiatr Dis Treat. (2016) 12:1019–27. doi: 10.2147/NDT.S103821
26. Alacreu-Crespo A, Guillaume S, Seneque M, Olie E, Courtet P. Cognitive modelling to assess decision-making impairments in patients with current depression and with/without suicide history. Eur Neuropsychopharmacol. (2020) 36:50–9. doi: 10.1016/j.euroneuro.2020.04.006
27. Sip KE, Gonzalez R, Taylor SF, Stern ER. Increased loss aversion in unmedicated patients with obsessive-compulsive disorder. Front Psychiatry. (2017) 8:309. doi: 10.3389/fpsyt.2017.00309
28. Gillan CM, Morein-Zamir S, Urcelay GP, Sule A, Voon V, Apergis-Schoute AM, et al. Enhanced avoidance habits in obsessive-compulsive disorder. Biol Psychiatry. (2014) 75:631–8. doi: 10.1016/j.biopsych.2013.02.002
29. Endrass T, Kloft L, Kaufmann C, Kathmann N. Approach and avoidance learning in obsessive-compulsive disorder. Depress Anxiety. (2011) 28:166–72. doi: 10.1002/da.20772
30. Kaufmann C, Beucke JC, Preuße F, Endrass T, Schlagenhauf F, Heinz A, et al. Medial prefrontal brain activation to anticipated reward and loss in obsessive-compulsive disorder. Neuroimage Clin. (2013) 2:212–20. doi: 10.1016/j.nicl.2013.01.005
31. Hamilton M. The assessment of anxiety states by rating. Br J Med Psychol. (1959) 32:50–5. doi: 10.1111/j.2044-8341.1959.tb00467.x
32. Hamilton M. A Rating scale for depression. J Neurol Neurosurg Psychiatry. (1960) 23:56–62. doi: 10.1136/jnnp.23.1.56
33. Matsuoka K, Uno M, Kasai K, Koyama K, Kim Y. Estimation of premorbid iq in individuals with alzheimer's disease using japanese ideographic script (kanji) compound words: japanese version of national adult reading test. Psychiatry Clin Neurosci. (2006) 60:332–9. doi: 10.1111/j.1440-1819.2006.01510.x
34. Rescorla RA, Wagner AR. A Theory of Pavlovian Condi- Tioning: Variations in the Effectiveness of Reinforcement and Non- Reinforcement. London: Appleton-Century-Crofts (1972).
35. Yechiam E, Busemeyer JR. Evaluating generalizability and parameter consistency in learning models. Games Econ Behav. (2008) 63:370–94. doi: 10.1016/j.geb.2007.08.011
36. Yechiam E, Busemeyer JR. Comparison of basic assumptions embedded in learning models for experience-based decision making. Psychon Bull Rev. (2005) 12:387–402. doi: 10.3758/BF03193783
37. Steingroever H, Wetzels R, Wagenmakers E-J. A comparison of reinforcement learning models for the Iowa gambling task using parameter space partitioning. J Prob Solv. (2013) 5:1150. doi: 10.7771/1932-6246.1150
38. Ahn WY, Vasilev G, Lee SH, Busemeyer JR, Kruschke JK, Bechara A, et al. Decision-making in stimulant and opiate addicts in protracted abstinence: evidence from computational modeling with pure users. Front Psychol. (2014) 5:849. doi: 10.3389/fpsyg.2014.00849
39. Team RDC. R: A Language and Environment for Statistical Computing. Vienna: R Fundation for Statisitical Computing (2021).
40. Turnbull OH, Bowman CH, Shanker S, Davies JL. Emotion-based learning: insights from the Iowa gambling task. Front Psychol. (2014) 5:162. doi: 10.3389/fpsyg.2014.00162
41. Dunn BD, Galton HC, Morgan R, Evans D, Oliver C, Meyer M, et al. Listening to your heart. How interoception shapes emotion experience and intuitive decision making. Psychol Sci. (2010) 21:1835–44. doi: 10.1177/0956797610389191
42. Singh V. A potential role of reward and punishment in the facilitation of the emotion-cognition dichotomy in the Iowa gambling task. Front Psychol. (2013) 4:944. doi: 10.3389/fpsyg.2013.00944
43. See CCH, Tan JM, Tan VSY, Sundermann O. A systematic review on the links between emotion regulation difficulties and obsessive-compulsive disorder. J Psychiatr Res. (2022) 154:341–53. doi: 10.1016/j.jpsychires.2022.07.023
44. Bragdon LB, Eng GK, Belanger A, Collins KA, Stern ER. Interoception and obsessive-compulsive disorder: a review of current evidence and future directions. Front Psychiatry. (2021) 12:686482. doi: 10.3389/fpsyt.2021.686482
45. Yoris A, Garcia AM, Traiber L, Santamaria-Garcia H, Martorell M, Alifano F, et al. The inner world of overactive monitoring: neural markers of interoception in obsessive-compulsive disorder. Psychol Med. (2017) 47:1957–70. doi: 10.1017/S0033291717000368
46. Rouhani N, Wimmer GE, Schneier FR, Fyer AJ, Shohamy D, Simpson HB. Impaired generalization of reward but not loss in obsessive-compulsive disorder. Dep Anxiety. (2019) 36:121–9. doi: 10.1002/da.22857
47. Brambilla P, Perlini C, Bellani M, Tomelleri L, Ferro A, Cerruti S, et al. Increased salience of gains versus decreased associative learning differentiate bipolar disorder from schizophrenia during incentive decision making. Psychol Med. (2013) 43:571–80. doi: 10.1017/S0033291712001304
48. Vassileva J, Ahn WY, Weber KM, Busemeyer JR, Stout JC, Gonzalez R, et al. Computational modeling reveals distinct effects of hiv and history of drug use on decision-making processes in women. PLoS ONE. (2013) 8:e68962. doi: 10.1371/journal.pone.0068962
49. Li X, Lu ZL, D'Argembeau A, Ng M, Bechara A. The Iowa gambling task in fMRI images. Hum Brain Mapp. (2010) 31:410–23. doi: 10.1002/hbm.20875
50. Zha R, Li P, Liu Y, Alarefi A, Zhang X, Li J. The orbitofrontal cortex represents advantageous choice in the Iowa gambling task. Hum Brain Mapp. (2022) 43:3840–56. doi: 10.1002/hbm.25887
51. Colautti L, Iannello P, Silveri MC, Antonietti A. Decision making in Parkinson's disease: an analysis of the studies using the Iowa gambling task. Eur J Neurosci. (2021) 54:7513–49. doi: 10.1111/ejn.15497
52. Krain AL, Wilson AM, Arbuckle R, Castellanos FX, Milham MP. Distinct neural mechanisms of risk and ambiguity: a meta-analysis of decision-making. Neuroimage. (2006) 32:477–84. doi: 10.1016/j.neuroimage.2006.02.047
53. Northoff G, Grimm S, Boeker H, Schmidt C, Bermpohl F, Heinzel A, et al. Affective judgment and beneficial decision making: ventromedial prefrontal activity correlates with performance in the Iowa gambling task. Hum Brain Mapp. (2006) 27:572–87. doi: 10.1002/hbm.20202
54. Hiser J, Koenigs M. The multifaceted role of the ventromedial prefrontal cortex in emotion, decision making, social cognition, and psychopathology. Biol Psychiatry. (2018) 83:638–47. doi: 10.1016/j.biopsych.2017.10.030
55. Cox J, Witten IB. Striatal circuits for reward learning and decision-making. Nat Rev Neurosci. (2019) 20:482–94. doi: 10.1038/s41583-019-0189-2
56. Piras F, Piras F, Chiapponi C, Girardi P, Caltagirone C, Spalletta G. Widespread structural brain changes in ocd: a systematic review of voxel-based morphometry studies. Cortex. (2015) 62:89–108. doi: 10.1016/j.cortex.2013.01.016
57. Boedhoe PSW, Schmaal L, Abe Y, Alonso P, Ameis SH, Anticevic A, et al. Cortical abnormalities associated with pediatric and adult obsessive-compulsive disorder: findings from the enigma obsessive-compulsive disorder working group. Am J Psychiatry. (2018) 175:453–62. doi: 10.1176/appi.ajp.2017.17050485
58. Park H, Kim M, Kwak YB, Cho KIK, Lee J, Moon SY, et al. Aberrant cortico-striatal white matter connectivity and associated subregional microstructure of the striatum in obsessive-compulsive disorder. Mol Psychiatry. (2022) 27:3460–7. doi: 10.1038/s41380-022-01588-6
59. Shephard E, Stern ER, van den Heuvel OA, Costa DLC, Batistuzzo MC, Godoy PBG, et al. Toward a neurocircuit-based taxonomy to guide treatment of obsessive-compulsive disorder. Mol Psychiatry. (2021) 26:4583–604. doi: 10.1038/s41380-020-01007-8
60. Milad MR, Rauch SL. Obsessive-compulsive disorder: beyond segregated cortico-striatal pathways. Trends Cogn Sci. (2012) 16:43–51. doi: 10.1016/j.tics.2011.11.003
61. Stein DJ, Costa DLC, Lochner C, Miguel EC, Reddy YCJ, Shavitt RG, et al. Obsessive-compulsive disorder. Nat Rev Dis Primers. (2019) 5:52. doi: 10.1038/s41572-019-0102-3
62. Nakao T, Nakagawa A, Nakatani E, Nabeyama M, Sanematsu H, Yoshiura T, et al. Working memory dysfunction in obsessive-compulsive disorder: a neuropsychological and functional MRI study. J Psychiatr Res. (2009) 43:784–91. doi: 10.1016/j.jpsychires.2008.10.013
63. Abramovitch A, McCormack B, Brunner D, Johnson M, Wofford N. The impact of symptom severity on cognitive function in obsessive-compulsive disorder: a meta-analysis. Clin Psychol Rev. (2019) 67:36–44. doi: 10.1016/j.cpr.2018.09.003
64. Heinzel S, Kaufmann C, Grützmann R, Klawohn J, Riesel A, Bey K, et al. Polygenic risk for obsessive-compulsive disorder (OCD) predicts brain response during working memory task in OCD, unaffected relatives, and healthy controls. Sci Rep. (2021) 11:18914. doi: 10.1038/s41598-021-98333-w
65. Smith EE, Jonides J. Storage and executive processes in the frontal lobes. Science. (1999) 283:1657–61. doi: 10.1126/science.283.5408.1657
66. Jameson TL, Hinson JM, Whitney P. Components of working memory and somatic markers in decision making. Psychon Bull Rev. (2004) 11:515–20. doi: 10.3758/BF03196604
67. Bagneux V, Thomassin N, Gonthier C, Roulin JL. Working memory in the processing of the Iowa gambling task: an individual differences approach. PLoS ONE. (2013) 8:e81498. doi: 10.1371/journal.pone.0081498
68. Cui JF, Wang Y, Shi HS, Liu LL, Chen XJ, Chen YH. Effects of working memory load on uncertain decision-making: evidence from the Iowa gambling task. Front Psychol. (2015) 6:162. doi: 10.3389/fpsyg.2015.00162
69. Bahmani Z, Clark K, Merrikhi Y, Mueller A, Pettine W, Isabel Vanegas M, et al. Prefrontal contributions to attention and working memory. Curr Top Behav Neurosci. (2019) 41:129–53. doi: 10.1007/7854_2018_74
70. Rezayat E, Clark K, Dehaqani MA, Noudoost B. Dependence of working memory on coordinated activity across brain areas. Front Syst Neurosci. (2021) 15:787316. doi: 10.3389/fnsys.2021.787316
71. Owen AM, McMillan KM, Laird AR, Bullmore E. N-Back working memory paradigm: a meta-analysis of normative functional neuroimaging studies. Hum Brain Mapp. (2005) 25:46–59. doi: 10.1002/hbm.20131
72. Robbins TW, Vaghi MM, Banca P. Obsessive-compulsive disorder: puzzles and prospects. Neuron. (2019) 102:27–47. doi: 10.1016/j.neuron.2019.01.046
73. Xie C, Ma L, Jiang N, Huang R, Li L, Gong L, et al. Imbalanced functional link between reward circuits and the cognitive control system in patients with obsessive-compulsive disorder. Brain Imaging Behav. (2017) 11:1099–109. doi: 10.1007/s11682-016-9585-7
74. Figee M, Vink M, de Geus F, Vulink N, Veltman DJ, Westenberg H, et al. Dysfunctional reward circuitry in obsessive-compulsive disorder. Biol Psychiatry. (2011) 69:867–74. doi: 10.1016/j.biopsych.2010.12.003
75. van Timmeren T, Daams JG, van Holst RJ, Goudriaan AE. Compulsivity-related neurocognitive performance deficits in gambling disorder: a systematic review and meta-analysis. Neurosci Biobehav Rev. (2018) 84:204–17. doi: 10.1016/j.neubiorev.2017.11.022
76. Hong W, Liang P, Pan Y, Jin J, Luo L, Li Y, et al. Reduced loss aversion in value-based decision-making and edge-centric functional connectivity in patients with internet gaming disorder. J Behav Addict. (2023) 12:458–70. doi: 10.1556/2006.2023.00014
77. Genauck A, Quester S, Wustenberg T, Morsen C, Heinz A, Romanczuk-Seiferth N. Reduced loss aversion in pathological gambling and alcohol dependence is associated with differential alterations in amygdala and prefrontal functioning. Sci Rep. (2017) 7:16306. doi: 10.1038/s41598-017-16433-y
78. Fontenelle LF, Mendlowicz MV, Versiani M. Impulse control disorders in patients with obsessive-compulsive disorder. Psychiatry Clin Neurosci. (2005) 59:30–7. doi: 10.1111/j.1440-1819.2005.01328.x
79. Fontenelle LF, Oostermeijer S, Harrison BJ, Pantelis C, Yücel M. Obsessive-compulsive disorder, impulse control disorders and drug addiction: common features and potential treatments. Drugs. (2011) 71:827–40. doi: 10.2165/11591790-000000000-00000
80. Matsunaga H, Kiriike N, Matsui T, Oya K, Okino K, Stein DJ. Impulsive disorders in Japanese adult patients with obsessive-compulsive disorder. Compr Psychiatry. (2005) 46:43–9. doi: 10.1016/j.comppsych.2004.07.001
81. Kashyap H, Fontenelle LF, Miguel EC, Ferrao YA, Torres AR, Shavitt RG, et al. ‘Impulsive compulsivity' in obsessive-compulsive disorder: a phenotypic marker of patients with poor clinical outcome. J Psychiatr Res. (2012) 46:1146–52. doi: 10.1016/j.jpsychires.2012.04.022
82. Benatti B, Dell'Osso B, Arici C, Hollander E, Altamura AC. Characterizing impulsivity profile in patients with obsessive-compulsive disorder. Int J Psychiatry Clin Pract. (2014) 18:156–60. doi: 10.3109/13651501.2013.855792
83. Ettelt S, Ruhrmann S, Barnow S, Buthz F, Hochrein A, Meyer K, et al. Impulsiveness in obsessive-compulsive disorder: results from a family study. Acta Psychiatr Scand. (2007) 115:41–7. doi: 10.1111/j.1600-0447.2006.00835.x
84. Worthy DA, Pang B, Byrne KA. Decomposing the roles of perseveration and expected value representation in models of the Iowa gambling task. Front Psychol. (2013) 4:640. doi: 10.3389/fpsyg.2013.00640
85. Haines N, Vassileva J, Ahn WY. The outcome-representation learning model: a novel reinforcement learning model of the Iowa gambling task. Cogn Sci. (2018) 42:2534–61. doi: 10.1111/cogs.12688
86. Hwang SH, Kwon JS, Shin YW, Lee KJ, Kim YY, Kim MS. Neuropsychological profiles of patients with obsessive-compulsive disorder: early onset versus late onset. J Int Neuropsychol Soc. (2007) 13:30–7. doi: 10.1017/S1355617707070063
87. Zhang J, Yang X, Yang Q. Neuropsychological dysfunction in adults with early-onset obsessive-compulsive disorder: the search for a cognitive endophenotype. Braz J Psychiatry. (2015) 37:126–32. doi: 10.1590/1516-4446-2014-1518
88. Roth RM, Milovan D, Baribeau J, O'Connor K. Neuropsychological functioning in early- and late-onset obsessive-compulsive disorder. J Neuropsychiatry Clin Neurosci. (2005) 17:208–13. doi: 10.1176/jnp.17.2.208
89. Nakao T, Nakagawa A, Yoshiura T, Nakatani E, Nabeyama M, Sanematsu H, et al. Duration effect of obsessive-compulsive disorder on cognitive function: a functional MRI study. Depress Anxiety. (2009) 26:814–23. doi: 10.1002/da.20484
90. Bragdon LB, Gibb BE, Coles ME. Does neuropsychological performance in OCD relate to different symptoms? A meta-analysis comparing the symmetry and obsessing dimensions. Dep Anxiety. (2018) 35:761–74. doi: 10.1002/da.22785
Keywords: obsessive-compulsive disorder, decision-making, Iowa gambling test, prospective valence learning model, consistency parameter
Citation: Murayama K, Tomiyama H, Ohno A, Kato K, Matsuo A, Hasuzawa S, Sashikata K, Kang M and Nakao T (2023) Decision-making deficits in obsessive-compulsive disorder are associated with abnormality of recency and response consistency parameter in prospect valence learning model. Front. Psychiatry 14:1227057. doi: 10.3389/fpsyt.2023.1227057
Received: 22 May 2023; Accepted: 07 September 2023;
Published: 29 September 2023.
Edited by:
Henry W. Chase, University of Pittsburgh, United StatesReviewed by:
Peter Zhukovsky, University of Toronto, CanadaAbeer Eissa, Ain Shams University, Egypt
Stacie L. Warren, The University of Texas at Dallas, United States
Copyright © 2023 Murayama, Tomiyama, Ohno, Kato, Matsuo, Hasuzawa, Sashikata, Kang and Nakao. This is an open-access article distributed under the terms of the Creative Commons Attribution License (CC BY). The use, distribution or reproduction in other forums is permitted, provided the original author(s) and the copyright owner(s) are credited and that the original publication in this journal is cited, in accordance with accepted academic practice. No use, distribution or reproduction is permitted which does not comply with these terms.
*Correspondence: Keitaro Murayama, bXVyYXlhbWEua2VpdGFyby4wMDNAbS5reXVzaHUtdS5hYy5qcA==