- 1Department of Radiology, Huazhong University of Science and Technology Union Shenzhen Hospital, Shenzhen, China
- 2Guangdong Provincial Key Laboratory of Genome Stability and Disease Prevention and Regional Immunity and Diseases, Department of Pathology, Shenzhen University School of Medicine, Shenzhen, Guangdong, China
- 3Department of Depressive Disorder, Shenzhen Kangning Hospital, Shenzhen Mental Health Center, Shenzhen, Guangdong, China
- 4Department of Radiology, Shenzhen Kangning Hospital, Shenzhen Mental Health Center, Shenzhen, China
Objectives: To explore the suicide risk-specific disruption of cortical hierarchy in major depressive disorder (MDD) patients with diverse suicide risks.
Methods: Ninety-two MDD patients with diverse suicide risks and 38 matched controls underwent resting-state functional MRI. Connectome gradient analysis and stepwise functional connectivity (SFC) analysis were used to characterize the suicide risk-specific alterations of cortical hierarchy in MDD patients.
Results: Relative to controls, patients with suicide attempts (SA) had a prominent compression from the sensorimotor system; patients with suicide ideations (SI) had a prominent compression from the higher-level systems; non-suicide patients had a compression from both the sensorimotor system and higher-level systems, although it was less prominent relative to SA and SI patients. SFC analysis further validated this depolarization phenomenon.
Conclusion: This study revealed MDD patients had suicide risk-specific disruptions of cortical hierarchy, which advance our understanding of the neuromechanisms of suicidality in MDD patients.
1. Introduction
Major depressive disorder (MDD) is a common, debilitating, and potentially lethal disorder (1). Patients with MDD usually have high suicide risk levels (2), which aggravate public health crises around the world (3). Previous studies offered a three-step theory of suicide [suicidal ideations (SIs), suicidal attempts (SAs), and suicidal death (SD)] and emphasized the progression from SI to SA (4, 5). It was reported that SI is an important precondition for SA (4–6), while SA is a powerful predictor of completed suicide (7). This progressive relationship indicates that SI and SA may be important intervention points for predicting and preventing suicide. Currently, psychological scales and observed clinical characteristics are the most commonly used methods to evaluate the risk levels of suicide in MDD patients (8, 9). However, these methods cannot identify patients with SI and SA objectively and accurately, particularly for those unwilling to disclose their thinking or commit suicidal acts (5, 8). Therefore, it is urgent to find the objective biomarker to discriminate and identify MDD patients with diverse suicide risk.
Resting-state functional MRI (rs-fMRI) had been widely used to investigate MDD patients with diverse suicidal risks from the perspective of regional brain activity (10) and the brain connectome (9), which has presented inspiring results (11, 12). Wagner et al. found SA had lower regional brain function involved in the fronto-parietal regions and the occipital regions relative to health control and MDD patients without SA by using the amplitude of low-frequency fluctuation index (13). Kang et al. reported SA had higher resting-state functional connectivity (RSFC) of the amygdala and the insula and of the superior orbitofrontal area and the middle temporal area than non-suicide attempters; thus they concluded that the RSFC of the amygdala may reflect suicide risk levels (14). From the perspective of intra-and inter-network interaction, Ho et al. found that lower intra-network coherence of all default subnetworks and insula salience network were associated with the higher past-month SI in depressed adolescents (15). These studies present inspiring results regarding the potential linkage between brain activity or connectivity and suicidality in MDD patients. However, most previous studies focused on a single subgroup (SA or SI) (9–11, 14, 15); thus, there is still a lack of comprehensive, translatable understanding for identifying MDD patients with diverse suicide risks, which warrants further research with new approaches.
Recent research in neuroscience has advanced our understanding that depression is associated with dysfunction of cortical hierarchy (16) as well as disruption of information flow from unimodal sensorimotor networks to the transmodal default network (17), rather than of individual systems alone (17, 18). More recently, Xia et al. demonstrated that MDD patients presented dysfunction of the core connectome hierarchy, which had close linkage with gene expression profiles (16). However, the suicide risk-specific alterations of cortical hierarchy as well as the intact of hierarchical information propagation in MDD patients are still uncovered. The stepwise functional connectivity (SFC) analysis, as a complementary simulation approach for gradient analysis (19), has shown that cortical hierarchy can also be understood as a sequence of steps in connectivity space specifically from unimodal sensorimotor networks to the transmodal default network (19–21). Thus, the combination of two approaches in MDD patients with diverse suicide risks, enable us to understand the specific disruption of the unimodal to transmodal processing as well as their sequence of steps in the connectivity space in MDD patients with diverse suicide risk.
To address these gaps, this study aimed to characterize: (i) the specific macroscale unimodal-to-transmodal gradient alterations in MDD with diverse suicide risks and (ii) the sequence impairment of integration from the unimodal sensory networks to the transmodal networks in MDD with diverse suicide risks.
2. Materials and methods
2.1. Subjects
Ninety-two adult patients with a diagnosis of MDD (average age: 32.79 ± 12.21 years old; 34 males and 58 females) according to the DSM-IV criteria (22–25) and 38 healthy adults (average age: 37.12 ± 12.61 years old, 13 males and 25 females) with no history of neurological or psychiatric illness participated in this study. Details of inclusion criteria were provided in Supplementary material.
MDD patients were further divided into three subgroups according to their levels of suicide risk, which were estimated by the third item on the HAMD-17 (13, 26, 27). The low suicide risk group [not suicidal (NS) group, n = 30] consisted of patients with scores ≤1, indicating the absence of suicidal ideation or suicide attempts within last 2 weeks; the high suicide risk group (n = 62) consisted of patients with scores of 2, 3, or 4. The high suicide risk group was then divided into the suicide ideation (SI, n = 30) group (with scores of 2 or 3), indicating the presence of suicidal ideation but no suicide attempts within last 2 weeks and the suicide attempt (SA, n = 32) group (with scores of 4), indicating a history of suicide attempts within last 2 weeks.
The local Research Ethics Committee approved this study, and written informed consent was obtained from each participant before the study.
2.2. Data acquisition and data preprocessing
All of the structural and rs-fMRI images were acquired on a 3.0 Tesla MR scanner (GE Discovery 750) with an eight-channel head coil. Data were preprocessed using DPABI v5.11 (28) following previous studies (20, 29). Detailed imaging parameters and data preprocessing were provided in Supplementary materials.
2.3. Connectome gradient analysis
Connectome gradient analysis had been developed to analyze the cortical hierarchy at the systems level, which anchors the unimodal sensory networks on the one end and the transmodal network on the other end (30, 31) and supports the transition from perception to more abstract cognitive functions (31, 32). Gradient mapping provides us a simplified representation in terms of main dimensions to characterize the macroscale cortical hierarchy (16, 31). The connectome gradient analysis was processed using the BrainSpace toolbox 2 (33). More details can be found in the Supplementary materials and a previous study (20).
2.4. Stepwise connectivity estimation
Stepwise functional connectivity (SFC) analysis is a graph theory-based method that counts the number of defined seeds to other brain regions in a given length of connectivity distance (19–21). More details for stepwise connectivity analysis can be found in the Supplementary materials.
3. Statistical analysis
One-way ANOVA and the Chi-square test were performed to detect the differences in demographic and clinical data among the four groups using IBM SPSS v.25.0 (34). Post hoc tests were conducted using the Turkey method and Bonferroni method. The significance level was set at p < 0.05.
A one-sample t-test was performed for SFC to explore the spatial transition patterns of each group. The significance level was set at p < 0.0001, FDR corrected.
General linear models implemented in the DPABI toolbox were performed to determine differences between any two groups (HC vs. MDD, MDD-NS vs. MDD-SI, MDD-NS vs. MDD-SA and MDD-SI vs. MDD-SA), including normalized gradient scores and SFC degree at each link-step distance. Sex, age, and education years were set as covariates. Duration of depressive episodes and HAMD score were set as additional covariates in the comparison of patients. The Gaussian random field (GRF) was used for multiple comparison correction (voxel level of p < 0.01, cluster level of p < 0.05).
4. Results
4.1. Demographic and clinical characteristics
No significant differences were found in education among healthy controls and MDD subgroups (one-way ANOVA, F value = 1.75, p = 0.160). However, sex and age differed significantly among them (sex, Chi-square test, χ2 value =11.56, p < 0.05; age, one-way ANOVA, F value = 4.45, p < 0.05). Post hoc analysis revealed that the SA group had a higher proportion of women than the NS group and SI group (p < 0.05), while the HC group and NS group were older on average than the SI group and SA group (p < 0.05). Among MDD patients, the NS group had a longer duration of depressive episodes than the SI group and SA group (p < 0.05), while the SA group had a higher HAMD score than the NS group and SI group (p < 0.05). The demographic and clinical characteristics information is provided in Table 1.
4.2. Gradient alterations in MDD with diverse suicide risks
To maximize interpretability, we only retained principal gradients explaining more than 10% of connectome variance in the dataset following previous studies (20, 21). The first and second principal gradients explained 11.33 and 10.18% of the connectome variance in our dataset, respectively (Figure 1A). The connectome gradient maps for four groups are shown in Figure 1B. The spatial patterns of connectome gradient maps were outstandingly similar among healthy controls and the three MDD subgroups, with the unimodal sensory networks (VIS, AUD, SMN) on the one end and the transmodal network [default mode network, (DMN)] on the other end.
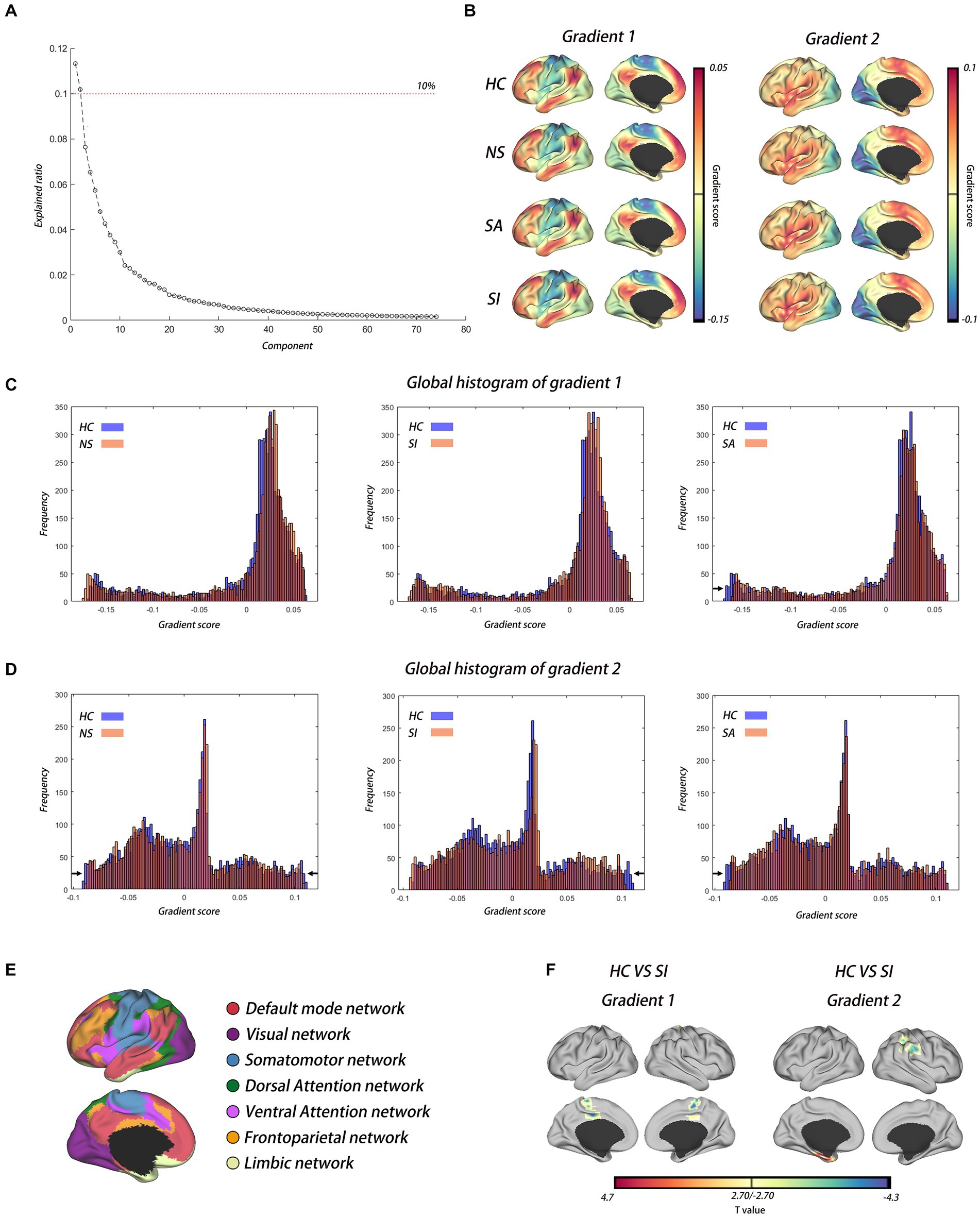
Figure 1. The cortical hierarchy dysfunction in MDD with diverse suicide risks. (A) Variance explained by gradient; the dotted curve shows gradients that explained more than 10% of connectome variance in the dataset. (B) The first and second functional gradients’ spatial pattern in HC and MDD with diverse suicide risks. (C,D) Global histograms showed the depolarization phenomenon in MDD patients with diverse suicide risks compared with HC in gradient 1 and gradient 2, respectively. (E) Seven networks template estimated by Yeo. (F) Voxel-wise statistical comparisons between HC and MDD patients with SI in gradient 1 and gradient 2, respectively (GRF corrected, voxel level of p < 0.01, cluster level of p < 0.05). HC, health controls; NS, not suicidal group; SI, suicide ideation group; SA, suicide attempt group.
Visual inspection of the global histogram of the principal gradients indicated that the extremes of the unimodal-to-transmodal gradient were contracted uniquely in MDD patients with diverse suicide risks compared with healthy controls. The SA group had a prominent compression from the sensorimotor system of the cortical hierarchy (gradient 1 and gradient 2), the SI group had a prominent compression from higher-level systems such as the frontoparietal network (FPN) and DMN (gradient 2), and the NS group had a compression from both the sensorimotor system and higher-level systems (gradient 2), although it was less prominent than that of patients with SI or SA (Figures 1C,D and Supplementary Figures S1A,B). Group comparison indicated that SI had a higher gradient score in the SMN (e.g., Cingulum_Mid_L) than in the HC (Gradient 1), while SI had a higher gradient score in the DMN (e.g., SupraMarginal_R) and a lower gradient score in the limbic network (e.g., ParaHippocampal_L) than in the HC (Gradient 2) (Figure 1F and Table 2). Compared to NS, SI had a higher gradient score in the VIS (e.g., cuneus), and SA had a lower gradient score in the DMN (e.g., medial frontal gyrus, precuneus) (Supplementary Figure S1D and Table 2). Of note, the whole-brain network allocations were based on the seven networks template by Yeo et al. (35) (Figure 1E).
4.3. Integration impaired in MDD with diverse suicide risks
Similar spatial transition patterns of stepwise connectivity were observed among healthy controls and the three MDD subgroups (Figure 2). Unimodal sensory-driven networks reached and stabilized at the transmodal networks (DMN) through seven steps (Figure 2).
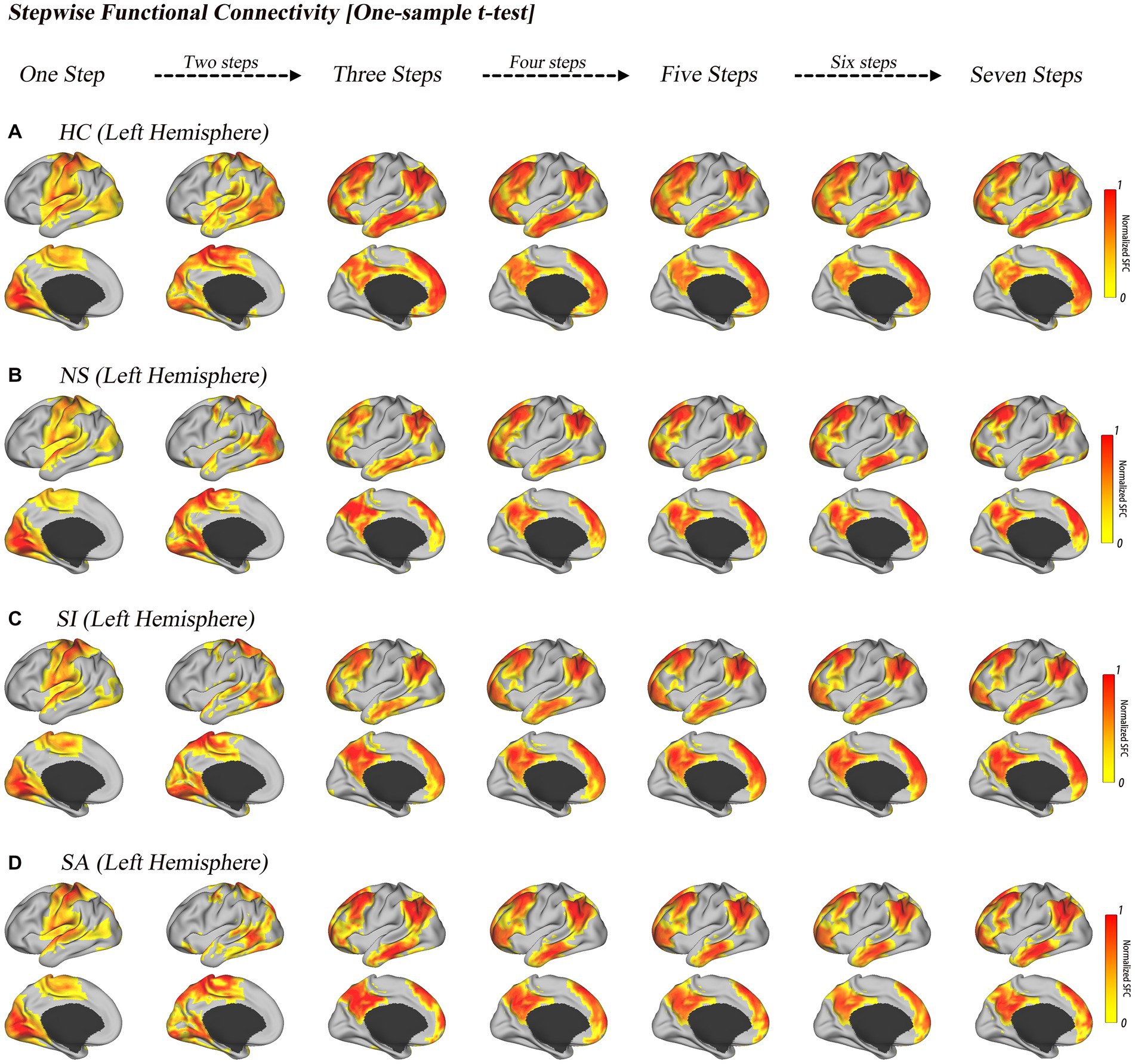
Figure 2. Stepwise functional connectivity pattern in HC and MDD with diverse suicide risks. Surface projections of the one-sample t-test results of the unimodal-to-transmodal link-step distances for HC (A), NS (B), SI (C), and SA (D). All of the results are displayed after multiple comparison correction (p < 0.0001, FDR corrected). The color bar represents the normalized color scale, 0 represents non-significant results, and 1 is the maximum value corresponding to the smallest p value.
Relative to HC, NS patients had an increased SFC degree of the DMN (e.g., precuneus, SupraMarginal_R) at steps 1–3 and increased SFC degree of VIS (e.g., lingual gyrus) at steps 4–7; SI patients had a decreased SFC degree of the limbic network (inferior frontal gyrus and SupraMarginal_R) as well as increased SFC degree of the DMN (precuneus); SA patients had an increased SFC degree of the VIS (e.g., cuneus, Calcarine_L) at steps 3–7, which also extended to the DMN (e.g., precuneus); a transient decrease in the SFC degree of the limbic network (e.g., inferior frontal gyrus) was also observed in step 2. More details are provided in Figure 3 and Table 3.
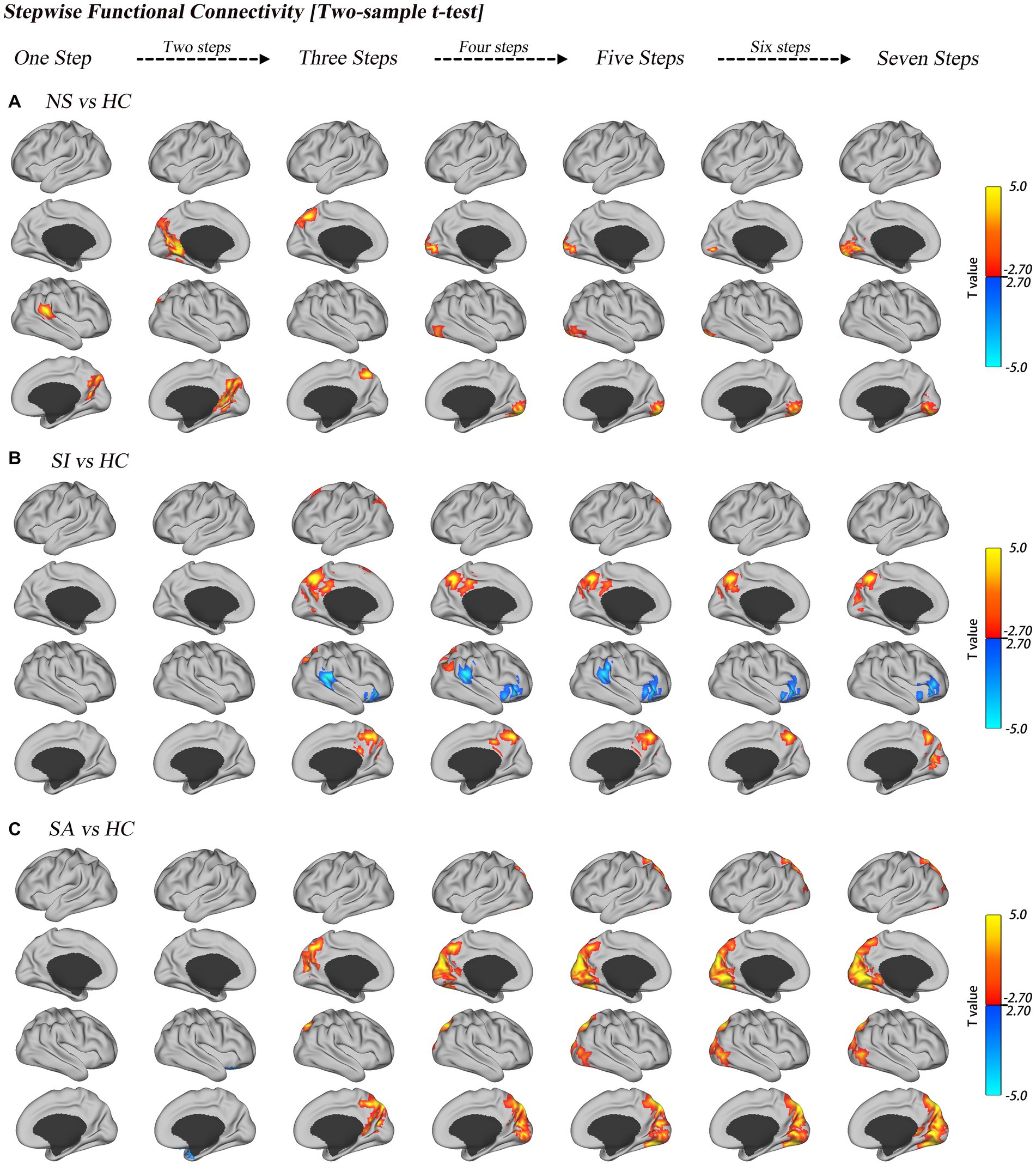
Figure 3. Group comparisons of link-step distance between HC and MDD with diverse suicide risks. Surface projections of the two-sample t-test results of the unimodal-to-transmodal link-step distances for NS vs. HC (A), SI vs. HC (B), and SA vs. HC (C). All of the results are displayed after multiple comparison correction (GRF correction, voxel level of p < 0.01, cluster level of p < 0.05).
Pairwise comparison of patients with diverse suicide risks revealed that NS patients had increased SFC degree of the VIS (e.g., Lingual_L, Lingual_R) and decreased SFC degree of the SMN (e.g., Postcentral_L, Postcentral_R) at step 1 when compared to SI patients. NS had decreased SFC degree of the SMN (e.g., Postcentral_L) at step 1 when compared to SA patients. SI patients had increased SFC degree of the DMN (e.g., Frontal_Sup_Medial_L) at step 5 when compared to SA patients. More details are provided in Supplementary Figure S2 and Table 3.
4.4. Control analyses
In order to investigate the potential influences of global signal regression (GSR) on gradient analysis and SFC analysis. We further conducted a control analysis without GSR in the functional MRI pre-processing. Furthermore, in order to minimize the risk of Type-I errors or false positives, we re-analyzed the data using a multiple comparison correction threshold of voxel-level p < 0.01 and cluster-level p < 0.008. Similar results were found between the validation analysis and primary analysis. More details are provided in Supplementary Figures S3–S9 and Supplementary Tables S1, S2.
5. Discussion
This is the first study, to our knowledge, to investigate the cortical hierarchy and the sequence of steps in the connectivity space in MDD with diverse suicide risks using connectome gradient and SFC analysis. We demonstrated that a suicide risk-specific disruption of unimodal sensorimotor networks to the transmodal default network existed in MDD patients. Specifically, relative to HC, SA patients had a prominent compression from the sensorimotor system of the cortical hierarchy; SI patients had a prominent compression from the higher-level systems such as the FPN and DMN; NS patients had a compression from both the sensorimotor system and higher-level systems, although it was less prominent than that of SI and SA. The SFC analysis further validated this depolarization phenomenon related to atypical functional transitions from unimodal to multimodal cortical areas in MDD patients with diverse suicide risk levels. Cortical hierarchy disorganization and the sequence of steps in connectivity space abnormalities observed in this study might further improve our understanding of the neural substrates underlying the diverse suicidality in MDD patients.
Hierarchical architecture is one of the recent advances in neuroscience for understanding the organizational principles in the brain (31). First, the unimodal sensory networks (VIS, AUD, SMN) are involved in receiving and abstracting stimulation (36–38). Then, the DMN integrates abstract information with personal emotion, self-cognition, and theoretical ideas and finally guides action in the outside world (39–42). In line with a recent connectome gradient study on MDD (16), this study also observed that MDD patients had compressed global gradient score compared to HC (Supplementary Figure S1A). Moreover, we found the contracted pattern exhibited suicide risk-specific patterns in MDD patients. SA mainly involves the unimodal sensorimotor networks (VIS), the SI mainly involves the transmodal default network (DMN), and NS involves both the unimodal and transmodal networks but less prominently compared to SI and SA (Supplementary Figures S1A,B).
5.1. MDD patients with SA exhibited abnormal cortical hierarchy organization mainly in the primary network (VIS)
Compared to HC, MDD patients with SA exhibited gradient compression mainly in the primary network (visual network) in both the first and second gradient. Furthermore, the SFC analysis revealed information transition dysfunction across all of the link-step distances (steps 2–7), which also mainly involved the visual networks, as well as the DMN. The visual network plays important roles in information feedforward and feedback (43, 44). The contact visual network is helpful for resisting disturbance and making a correct response during tasks (43, 44), while a compromised visual network can lead to misconceptions and incorrect decisions in MDD patients with SA (45, 46). Consistent with present results, Holmes et al. indicated that suicide-related imagery, which resulted from the disruption of the visual network, was an essential factor causing suicidal behavior (47, 48). Wagner et al. found SA exhibited attenuated regional brain function as well as decreased functional connectivity involved in the occipital regions (visual related network), although they also revealed other brain regions abnormalities in SA group when compared to health control and MDD patients without SA (13, 49). Stumps et al. found the connection between the visual network and amygdala to be significantly altered in posttraumatic stress disorder patients with SA but not in the psychiatric control group, and they concluded that the disruption of visual network was an important factor causing SA (9). A prior meta-analysis also demonstrated that the hypoactivation of the visual network (e.g., left superior occipital gyrus) was closely related to suicidal thoughts and behaviors (50). Our findings complement previous literature with that MDD with SA possessed abnormal cortical hierarchy organization within the visual network, this disorganization would lead to SA be more concentrated in negative imagery and be more sensitive with negative imagery than HC (48), which would directly influence the emotional system (51) and finally lead to suicidal behavior (52).
5.2. MDD with SI exhibited abnormal cortical hierarchy organization mainly in the transmodal network (DMN)
Compared to HC, MDD with SI exhibited gradient compression mainly in the positive pole, where the transmodal network (DMN) is located. The SFC analysis revealed similar findings, which indicated that the information transition dysfunction was also mainly involved in the DMN. The DMN plays a critical role in ruminative processes (53) and eliminating negative information (54); the abnormal cortical hierarchy organization of the DMN observed in SI patients may be responsible for the failure to eliminate negative information (54). Once depressed patients are deeply embedded in negative emotions, they are more likely to have suicidal thoughts (55). The DMN had also been widely recognized as an important node correlating with the severity of SI (46, 56, 57). Schreiner et al. found that an increased connection between the precuneus and primary sensorimotor regions as well as a decreased connection between the posterior cingulate cortex (PCC) and visual attention regions was associated with greater severity of SI in MDD adolescents (58). In MDD adults with SI, the severity of SI was closely correlated with an ongoing low-frequency BOLD signal in the dorsal PCC, ventral PCC, and dorsal anterior cingulate cortex, as well as the decreased connections among these three regions (59). Thus, it is plausible that abnormal cortical hierarchy organization of the DMN may be an important feature for MDD patients with SI.
5.3. MDD with NS exhibited abnormal cortical hierarchy organization in both the unimodal and transmodal networks
Compared to HC, MDD with NS exhibited gradient compression in both the unimodal and transmodal networks, although it was less severe than that of MDD with high suicide risk levels (Supplementary Figures S1A,B). SFC analysis revealed that MDD patients with NS had unique disruption during information transition from unimodal to transmodal networks, and the dysfunction of transmodal networks (DMN) occurred at the early link-step distances, while the dysfunction of the visual network occurred at medium–late link-step distances. Although the significance of dysfunction from unimodal to transmodal networks is not clear, our findings were in line with those of recent studies. Using a large cohort of 2,414 participants (1,276 patients with MDD and 1,138 healthy controls), Xia et al. also demonstrated that patients with MDD exhibited abnormal global topography of the principal primary-to-transmodal gradient, as indicated by a reduced explanation ratio, gradient range, and gradient variation (16). Moreover, they found that focal alterations of gradient scores were mostly in the primary systems involved in sensory processing and in the transmodal systems implicated in high-order cognition (16). Our results also agreed with a recent effective connectivity study by Ray et al. using dynamic causal modeling, also demonstrating that depressed patients had hierarchy organization disruption, including compromises in both the backward connections (high-level network to primary network) and forward connections (primary network to high-level network), which were correlated with depressive symptomatology (17). This widespread dysfunction from the unimodal to transmodal networks would lead to failure in the processing of abstract representations (60) and information integration (61), which are characteristics of MDD (17).
6. Limitations
Several limitations should be acknowledged. First, the effect of antidepressant therapy was not included in our study, similar to other clinical imaging studies in the field (14, 62). A longitudinal study with detailed experimental and antidepressant profiling of a cohort of treatment-naive MDD patients is needed in the future. Second, the age, gender, and HAMD score were imbalanced among subgroups of MDD patients. Although we regressed them as covariates to reduce the potential influences, we could not eliminate these effects. In the future, we should recruit matched patients and design a more rigorous experiment to exclude such potential influences (63–65). Third, the sample size of the MDD subgroups was relatively small. Thus, these preliminary results should be validated in the future with large sample. Fourth, we only measured the current suicidal thoughts and behaviors (STBs, within last 2 weeks) of MDD patients. We acknowledge the lifetime history of STBs in the NS group could potentially affect the functional brain networks in MDD patients (66, 67). Therefore, it is important to record the lifetime history of STBs in future study.
7. Conclusion
In summary, the present work revealed suicide risk-specific disruptions of cortical hierarchy in MDD patients, which advance our understanding of the neuromechanisms of suicidality in MDD patients.
Data availability statement
The raw data supporting the conclusions of this article will be made available by the authors, without undue reservation.
Ethics statement
The studies involving human participants were reviewed and approved by Research Ethics Committee of Kangning Hospital. The patients/participants provided their written informed consent to participate in this study.
Author contributions
LS, ZX, ZY, CS, LX, XZ, and QY: drafting or revision of the manuscript for content, including medical writing for content, study concept or design, analysis or interpretation of data.
HG: major role in the acquisition of data, study concept and design. All authors contributed to the article and approved the submitted version.
Funding
This study has received funding by Natural Scientific Foundation of China (grant numbers: 81560283 and 81201084) and Natural Science Foundation of Guangdong (grant numbers: 2020A1515011332, and 2022A1515012503), the Shenzhen Key Medical Discipline Construction Fund (no. SZXK041), and the Shenzhen Fund for Guangdong Provincial High-level Clinical Key Specialties (no. SZGSP013).
Conflict of interest
The authors declare that the research was conducted in the absence of any commercial or financial relationships that could be construed as a potential conflict of interest.
Publisher’s note
All claims expressed in this article are solely those of the authors and do not necessarily represent those of their affiliated organizations, or those of the publisher, the editors and the reviewers. Any product that may be evaluated in this article, or claim that may be made by its manufacturer, is not guaranteed or endorsed by the publisher.
Supplementary material
The Supplementary material for this article can be found online at: https://www.frontiersin.org/articles/10.3389/fpsyt.2023.1140915/full#supplementary-material
Footnotes
References
1. Stringaris, A. Editorial: what is depression? J Child Psychol Psychiatry. (2017) 58:1287–9. doi: 10.1111/jcpp.12844
2. Wang, L, Zhao, Y, Edmiston, EK, Womer, FY, Zhang, R, Zhao, P, et al. Structural and functional abnormities of amygdala and prefrontal cortex in major depressive disorder with suicide attempts. Front Psych. (2020) 10:923–3. doi: 10.3389/fpsyt.2019.00923
3. World Health, O. Public Health Action for the Prevention of Suicide: A framework. Geneva: World Health Organization (2012).
4. Joiner, TEJr, and Silva, C. “Why people die by suicide: Further development and tests of the interpersonal-psychological theory of suicidal behavior,” In: Meaning, mortality, and choice: The social psychology of existential concerns, eds. PR Shaver and M Mikulincer American Psychological Association (2012) 325–336.
5. Klonsky, ED, May, AM, and Saffer, BY. Suicide, suicide attempts, and suicidal ideation. Annu Rev Clin Psychol. (2016) 12:307–30. doi: 10.1146/annurev-clinpsy-021815-093204
6. DeJong, TM, Overholser, JC, and Stockmeier, CA. Apples to oranges?: a direct comparison between suicide attempters and suicide completers. J Affect Disord. (2010) 124:90–7. doi: 10.1016/j.jad.2009.10.020
7. Suominen, K, Isometsä, E, Suokas, J, Haukka, J, Achte, K, and Lönnqvist, J. Completed suicide after a suicide attempt: a 37-year follow-up study. Am J Psychiatry. (2004) 161:562–3. doi: 10.1176/appi.ajp.161.3.562
8. Franklin, JC, Ribeiro, JD, Fox, KR, Bentley, KH, Kleiman, EM, Huang, X, et al. Risk factors for suicidal thoughts and behaviors: a meta-analysis of 50 years of research. Psychol Bull. (2017) 143:187–232. doi: 10.1037/bul0000084
9. Stumps, A, Jagger-Rickels, A, Rothlein, D, Amick, M, Park, H, Evans, T, et al. Connectome-based functional connectivity markers of suicide attempt. J Affect Disord. (2021) 283:430–40. doi: 10.1016/j.jad.2020.11.061
10. Jollant, F, Lawrence, NS, Giampietro, V, Brammer, MJ, Fullana, MA, Drapier, D, et al. Orbitofrontal cortex response to angry faces in men with histories of suicide attempts. Am J Psychiatry. (2008) 165:740–8. doi: 10.1176/appi.ajp.2008.07081239
11. Schmaal, L, van Harmelen, A-L, Chatzi, V, Lippard, ETC, Toenders, YJ, Averill, LA, et al. Imaging suicidal thoughts and behaviors: a comprehensive review of 2 decades of neuroimaging studies. Mol Psychiatry. (2020) 25:408–27. doi: 10.1038/s41380-019-0587-x
12. Thompson, M. F., Ghahramanlou-Holloway, M., Murphy, M. A., Perera, K. U., Benca-Bachman, C., Palmer, R. H. C., et al. (2022). Resting-state network analysis of suicide attempt history in the UK biobank. bioRxiv [Epub ahead of preprint]. doi: 10.1101/2022.01.04.474947
13. Wagner, G, Li, M, Sacchet, MD, Richard-Devantoy, S, Turecki, G, Bär, KJ, et al. Functional network alterations differently associated with suicidal ideas and acts in depressed patients: an indirect support to the transition model. Transl Psychiatry. (2021) 11:100. doi: 10.1038/s41398-021-01232-x
14. Kang, S-G, Na, K-S, Choi, J-W, Kim, J-H, Son, Y-D, and Lee, YJ. Resting-state functional connectivity of the amygdala in suicide attempters with major depressive disorder. Prog Neuro-Psychopharmacol Biol Psychiatry. (2017) 77:222–7. doi: 10.1016/j.pnpbp.2017.04.029
15. Ho, TC, Walker, JC, Teresi, GI, Kulla, A, Kirshenbaum, JS, Gifuni, AJ, et al. Default mode and salience network alterations in suicidal and non-suicidal self-injurious thoughts and behaviors in adolescents with depression. Transl Psychiatry. (2021) 11:38. doi: 10.1038/s41398-020-01103-x
16. Xia, M., Liu, J., Mechelli, A., Sun, X., Ma, Q., Wang, X., et al. Connectome gradient dysfunction in major depression and its association with gene expression profiles and treatment outcomes. Mol. Psychiat.. (2022) 27:1384–93. doi: 10.1038/s41380-022-01519-5
17. Ray, D, Bezmaternykh, D, Mel'nikov, M, Friston, KJ, and Das, M. Altered effective connectivity in sensorimotor cortices is a signature of severity and clinical course in depression. Proc Natl Acad Sci U S A. (2021) 118:e2105730118. doi: 10.1073/pnas.2105730118
18. Li, BJ, Friston, K, Mody, M, Wang, HN, Lu, HB, and Hu, DW. A brain network model for depression: from symptom understanding to disease intervention. CNS Neurosci Ther. (2018) 24:1004–19. doi: 10.1111/cns.12998
19. Sepulcre, J, Sabuncu, MR, Yeo, TB, Liu, H, and Johnson, KA. Stepwise connectivity of the modal cortex reveals the multimodal organization of the human brain. J Neurosci. (2012) 32:10649–61. doi: 10.1523/JNEUROSCI.0759-12.2012
20. Dong, D, Yao, D, Wang, Y, Hong, SJ, Genon, S, Xin, F, et al. Compressed sensorimotor-to-transmodal hierarchical organization in schizophrenia. Psychol Med. (2021) 53:771–84. doi: 10.1017/s0033291721002129
21. Hong, S-J, Vos de Wael, R, Bethlehem, RAI, Lariviere, S, Paquola, C, Valk, SL, et al. Atypical functional connectome hierarchy in autism. Nat Commun. (2019) 10:1022–2. doi: 10.1038/s41467-019-08944-1
22. Grunebaum, MF, Ellis, SP, Duan, N, Burke, AK, Oquendo, MA, and John Mann, J. Pilot randomized clinical trial of an SSRI vs bupropion: effects on suicidal behavior, ideation, and mood in major depression. Neuropsychopharmacology. (2012) 37:697–706. doi: 10.1038/npp.2011.247
23. Lan, X, Zhou, Y, Zheng, W, Zhan, Y, Liu, W, Wang, C, et al. Association between cognition and suicidal ideation in patients with major depressive disorder: a longitudinal study. J Affect Disord. (2020) 272:146–51. doi: 10.1016/j.jad.2020.03.141
24. Szanto, K, Mulsant, BH, Houck, PR, Dew, MA, Dombrovski, A, Pollock, BG, et al. Emergence, persistence, and resolution of suicidal ideation during treatment of depression in old age. J Affect Disord. (2007) 98:153–61. doi: 10.1016/j.jad.2006.07.015
25. Vuorilehto, M, Valtonen, HM, Melartin, T, Sokero, P, Suominen, K, and Isometsä, ET. Method of assessment determines prevalence of suicidal ideation among patients with depression. Eur Psychiatry. (2014) 29:338–44. doi: 10.1016/j.eurpsy.2013.08.005
26. Cha, C. B., and Franz, P. J., E, M. G, Glenn, C. R., Kleiman, E. M., and Nock, M. K. (2018). Annual research review: suicide among youth - epidemiology, (potential) etiology, and treatment. J Child Psychol Psychiatry, 59, 460–482. doi: 10.1111/jcpp.12831
27. McCauley, E, Berk, MS, Asarnow, JR, Adrian, M, Cohen, J, Korslund, K, et al. Efficacy of dialectical behavior therapy for adolescents at high risk for suicide: a randomized clinical trial. JAMA Psychiat. (2018) 75:777–85. doi: 10.1001/jamapsychiatry.2018.1109
28. Yan, CG, Wang, XD, Zuo, XN, and Zang, YF. DPABI: Data Processing & Analysis for (resting-state) brain imaging. Neuroinformatics. (2016) 14:339–51. doi: 10.1007/s12021-016-9299-4
29. Martínez, K, Martínez-García, M, Marcos-Vidal, L, Janssen, J, Castellanos, FX, Pretus, C, et al. Sensory-to-cognitive systems integration is associated with clinical severity in autism Spectrum disorder. J Am Acad Child Adolesc Psychiatry. (2020) 59:422–33. doi: 10.1016/j.jaac.2019.05.033
30. Huntenburg, JM, Bazin, PL, and Margulies, DS. Large-scale gradients in human cortical organization. Trends Cogn Sci. (2018) 22:21–31. doi: 10.1016/j.tics.2017.11.002
31. Margulies, DS, Ghosh, SS, Goulas, A, Falkiewicz, M, Huntenburg, JM, Langs, G, et al. Situating the default-mode network along a principal gradient of macroscale cortical organization. Proc Natl Acad Sci U S A. (2016) 113:12574–9. doi: 10.1073/pnas.1608282113
32. Mesulam, MM. From sensation to cognition. Brain. (1998) 121:1013–52. doi: 10.1093/brain/121.6.1013
33. Vos de Wael, R, Benkarim, O, Paquola, C, Lariviere, S, Royer, J, Tavakol, S, et al. Brain space: a toolbox for the analysis of macroscale gradients in neuroimaging and connectomics datasets. Commun Biol. (2020) 3:103. doi: 10.1038/s42003-020-0794-7
34. Vergara, VM, Liu, J, Claus, ED, Hutchison, K, and Calhoun, V. Alterations of resting state functional network connectivity in the brain of nicotine and alcohol users. NeuroImage. (2017) 151:45–54. doi: 10.1016/j.neuroimage.2016.11.012
35. Yeo, BT, Krienen, FM, Sepulcre, J, Sabuncu, MR, Lashkari, D, Hollinshead, M, et al. The organization of the human cerebral cortex estimated by intrinsic functional connectivity. J Neurophysiol. (2011) 106:1125–65. doi: 10.1152/jn.00338.2011
36. Goodale, MA, and Milner, AD. Separate visual pathways for perception and action. Trends Neurosci. (1992) 15:20–5. doi: 10.1016/0166-2236(92)90344-8
37. Mishkin, M, and Ungerleider, LG. Contribution of striate inputs to the visuospatial functions of parieto-preoccipital cortex in monkeys. Behav Brain Res. (1982) 6:57–77. doi: 10.1016/0166-4328(82)90081-x
38. Patterson, K, Nestor, PJ, and Rogers, TT. Where do you know what you know? The representation of semantic knowledge in the human brain. Nat Rev Neurosci. (2007) 8:976–87. doi: 10.1038/nrn2277
39. Jbabdi, S, Sotiropoulos, SN, and Behrens, TE. The topographic connectome. Curr Opin Neurobiol. (2013) 23:207–15. doi: 10.1016/j.conb.2012.12.004
40. Konishi, M, McLaren, DG, Engen, H, and Smallwood, J. Shaped by the past: the default mode network supports cognition that is independent of immediate perceptual input. PLoS One. (2015) 10:e0132209. doi: 10.1371/journal.pone.0132209
41. Spreng, RN, DuPre, E, Selarka, D, Garcia, J, Gojkovic, S, Mildner, J, et al. Goal-congruent default network activity facilitates cognitive control. J Neurosci. (2014) 34:14108–14. doi: 10.1523/jneurosci.2815-14.2014
42. Vatansever, D, Menon, DK, Manktelow, AE, Sahakian, BJ, and Stamatakis, EA. Default mode dynamics for global functional integration. J Neurosci Off J Soc Neurosci. (2015) 35:15254–62. doi: 10.1523/JNEUROSCI.2135-15.2015
43. Hocking, J, and Price, CJ. The role of the posterior superior temporal sulcus in audiovisual processing. Cereb Cortex. (2008) 18:2439–49. doi: 10.1093/cercor/bhn007
44. Wilson, SM, Bautista, A, and McCarron, A. Convergence of spoken and written language processing in the superior temporal sulcus. NeuroImage. (2018) 171:62–74. doi: 10.1016/j.neuroimage.2017.12.068
45. Bubl, E, Kern, E, Ebert, D, Bach, M, and Tebartz van Elst, L. Seeing gray when feeling blue? Depression can be measured in the eye of the diseased. Biol Psychiatry. (2010) 68:205–8. doi: 10.1016/j.biopsych.2010.02.009
46. Cao, J, Chen, JM, Kuang, L, Ai, M, Fang, WD, Gan, Y, et al. Abnormal regional homogeneity in young adult suicide attempters with no diagnosable psychiatric disorder: a resting state functional magnetic imaging study. Psychiatry Res. (2015) 231:95–102. doi: 10.1016/j.pscychresns.2014.10.011
47. Holmes, EA, Crane, C, Fennell, MJ, and Williams, JM. Imagery about suicide in depression--"flash-forwards"? J Behav Ther Exp Psychiatry. (2007) 38:423–34. doi: 10.1016/j.jbtep.2007.10.004
48. Holmes, EA, and Mathews, A. Mental imagery in emotion and emotional disorders. Clin Psychol Rev. (2010) 30:349–62. doi: 10.1016/j.cpr.2010.01.001
49. Wagner, G, de la Cruz, F, Köhler, S, Pereira, F, Richard-Devantoy, S, Turecki, G, et al. Connectomics-based functional network alterations in both depressed patients with suicidal behavior and healthy relatives of suicide victims. Sci Rep. (2019) 9:14330. doi: 10.1038/s41598-019-50881-y
50. Huang, X, Rootes-Murdy, K, Bastidas, DM, Nee, DE, and Franklin, JC. Brain differences associated with self-injurious thoughts and behaviors: a meta-analysis of neuroimaging studies. Sci Rep. (2020) 10:2404. doi: 10.1038/s41598-020-59490-6
51. Selby, EA, Kranzler, A, Panza, E, and Fehling, KB. Bidirectional-compounding effects of rumination and negative emotion in predicting impulsive behavior: implications for emotional cascades. J Pers. (2016) 84:139–53. doi: 10.1111/jopy.12147
52. Law, KC, and Tucker, RP. Repetitive negative thinking and suicide: a burgeoning literature with need for further exploration. Curr Opin Psychol. (2018) 22:68–72. doi: 10.1016/j.copsyc.2017.08.027
53. Zhou, H-X, Chen, X, Shen, Y-Q, Li, L, Chen, N-X, Zhu, Z-C, et al. Rumination and the default mode network: meta-analysis of brain imaging studies and implications for depression. NeuroImage. (2020) 206:116287. doi: 10.1016/j.neuroimage.2019.116287
54. Joormann, J. “Inhibition, rumination, and mood regulation in depression,” In: Cognitive limitations in aging and psychopathology, eds. RW Engle, G Sedek, U von Hecker and DN McIntosh Cambridge University Press (2005) 275–312. doi: 10.1017/CBO9780511720413.012
55. Rogers, ML, and Joiner, TE. Suicide-specific rumination relates to lifetime suicide attempts above and beyond a variety of other suicide risk factors. J Psychiatr Res. (2018) 98:78–86. doi: 10.1016/j.jpsychires.2017.12.017
56. Auerbach, RP, Pagliaccio, D, Allison, GO, Alqueza, KL, and Alonso, MF. Neural correlates associated with suicide and nonsuicidal self-injury in youth. Biol Psychiatry. (2021) 89:119–33. doi: 10.1016/j.biopsych.2020.06.002
57. Ordaz, SJ, Goyer, MS, Ho, TC, Singh, MK, and Gotlib, IH. Network basis of suicidal ideation in depressed adolescents. J Affect Disord. (2018) 226:92–9. doi: 10.1016/j.jad.2017.09.021
58. Schreiner, MW, Klimes-Dougan, B, and Cullen, KR. Neural correlates of suicidality in adolescents with major depression: resting-state functional connectivity of the Precuneus and posterior cingulate cortex. Suicide Life Threat Behav. (2019) 49:899–913. doi: 10.1111/sltb.12471
59. Chase, HW, Segreti, AM, Keller, TA, Cherkassky, VL, Just, MA, Pan, LA, et al. Alterations of functional connectivity and intrinsic activity within the cingulate cortex of suicidal ideators. J Affect Disord. (2017) 212:78–85. doi: 10.1016/j.jad.2017.01.013
60. Taylor, P, Hobbs, JN, Burroni, J, and Siegelmann, HT. The global landscape of cognition: hierarchical aggregation as an organizational principle of human cortical networks and functions. Sci Rep. (2015) 5:18112. doi: 10.1038/srep18112
61. Kendler, KS. The origin of our modern concept of depression-the history of melancholia from 1780-1880: a review. JAMA Psychiat. (2020) 77:863–8. doi: 10.1001/jamapsychiatry.2019.4709
62. Hong, S, Liu, YS, Cao, B, Cao, J, Ai, M, Chen, J, et al. Identification of suicidality in adolescent major depressive disorder patients using sMRI: a machine learning approach. J Affect Disord. (2021) 280:72–6. doi: 10.1016/j.jad.2020.10.077
63. Nenning, KH, Xu, T, Schwartz, E, Arroyo, J, Woehrer, A, Franco, AR, et al. Joint embedding: a scalable alignment to compare individuals in a connectivity space. NeuroImage. (2020) 222:117232. doi: 10.1016/j.neuroimage.2020.117232
64. Setton, R, Mwilambwe-Tshilobo, L, Girn, M, Lockrow, AW, Baracchini, G, Hughes, C, et al. Age differences in the functional architecture of the human brain. Cerebral Cortex (2022) 33:114–34. doi: 10.1093/cercor/bhac056
65. Xue, K, Guo, L, Zhu, W, Liang, S, Xu, Q, Ma, L, et al. Transcriptional signatures of the cortical morphometric similarity network gradient in first-episode, treatment-naive major depressive disorder. Neuropsychopharmacology. (2023) 48:518–28. doi: 10.1038/s41386-022-01474-3
66. Jung, J, Choi, S, Han, KM, Kim, A, Kang, W, Paik, JW, et al. Alterations in functional brain networks in depressed patients with a suicide attempt history. Neuropsychopharmacology. (2020) 45:964–74. doi: 10.1038/s41386-019-0560-z
Keywords: major depressive disorder, suicide, resting-state fMRI, connectome gradient, stepwise connectivity
Citation: Shiwei L, Xiaojing Z, Yingli Z, Shengli C, Xiaoshan L, Ziyun X, Gangqiang H and Yingwei Q (2023) Cortical hierarchy disorganization in major depressive disorder and its association with suicidality. Front. Psychiatry. 14:1140915. doi: 10.3389/fpsyt.2023.1140915
Edited by:
Ali Saffet Gonul, Ege University, TürkiyeCopyright © 2023 Shiwei, Xiaojing, Yingli, Shengli, Xiaoshan, Ziyun, Gangqiang and Yingwei. This is an open-access article distributed under the terms of the Creative Commons Attribution License (CC BY). The use, distribution or reproduction in other forums is permitted, provided the original author(s) and the copyright owner(s) are credited and that the original publication in this journal is cited, in accordance with accepted academic practice. No use, distribution or reproduction is permitted which does not comply with these terms.
*Correspondence: Hou Gangqiang, bmloYW9oZ3FAMTYzLmNvbQ==; Qiu Yingwei, cWl1eXcxMjAxQGdtYWlsLmNvbQ==
†These authors have contributed equally to this work