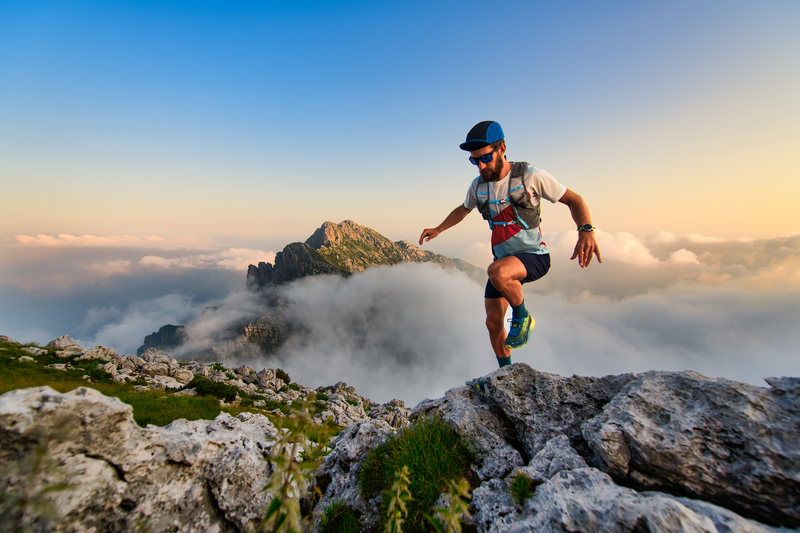
95% of researchers rate our articles as excellent or good
Learn more about the work of our research integrity team to safeguard the quality of each article we publish.
Find out more
EDITORIAL article
Front. Psychiatry , 21 February 2023
Sec. Computational Psychiatry
Volume 14 - 2023 | https://doi.org/10.3389/fpsyt.2023.1133072
This article is part of the Research Topic Machine Learning and Psychosis: Diagnosis, Prognosis and Treatment View all 5 articles
Editorial on the Research Topic
Machine learning and psychosis: Diagnosis, prognosis and treatment
Psychosis is a severe condition associated with considerable disability that affects patients and their families, causing substantial economic burdens in terms of lost productivity and direct costs (medications, hospitalizations, etc.) (1, 2). Individuals affected by psychosis still suffer from a low recovery rate and have a high likelihood of relapse. Moreover, patients affected by psychosis suffer from premature mortality due to cardio-metabolic complications and a higher risk for suicide (3–5).
For these reasons, psychosis prevention and early treatment are public health priorities. However, to date, psychosis still presents many unresolved challenges in diagnosis, identification of specific biomarkers, and treatments, especially in its early stages. Diagnoses are often blurred at presentation, and subgroups of individuals do not achieve a symptomatic and functional recovery, despite receiving specialized care. An increasing amount of evidence supports implementing early detection and intervention in psychotic disorders (6), with benefits in terms of a lower rate of relapse and overall better quality of life and functioning (7).
A precise definition of outcomes and response to treatments assessed readily on presentation would certainly impact patient management. However, so far, studies have struggled to identify valid biomarkers of psychosis trajectories and treatment response (8). Within the many strategies to improve the detection and outcomes of psychosis, machine learning (ML) techniques have seen tremendous development as they are resourceful in handling complex problems. ML are data-driven methods that deal with multivariate patterns by going beyond group-level analyses (9). These techniques have the potential to learn subtle data attributes, analyze massive data sets and combine different modalities (e.g., neuroimaging, clinical data, genetics, etc.) with the final aim of informing clinical decisions associated with diagnosis, prognosis, and treatment in psychiatry. ML can be employed to uncover patterns of risk and biomarkers, helping in early detection, understanding neurobiological heterogeneity, defining subgroups and endophenotypes, and predicting disease trajectory (10, 11).
Therefore, this Research Topic examines how modern computational techniques could contribute to clinical practice in addressing the unmet needs of patients with psychosis in terms of differential diagnoses, treatment, and outcome prediction. The qualified papers assessed the topic from different points of view, from genes to clinical and imaging data, proving how multifaced and adaptable ML techniques are to multiple contexts.
One of the significant advantages of ML tools is the possibility of handling huge amounts of data, such as those obtained from electronic health records (EHRs). EHRs represent a great source of information but often present a lack of standardized instruments, poor quality of the data, and a volume of information difficult to analyze. In their novel paper, Lee et al. assessed the possibility of using EHRs data to predict a 1-year relapse of psychosis. Moreover, they enriched their prediction, including natural language processing (NLP) data from clinical notes and psychological tests. NLP is another exponentially growing field within the bigger ML domain, with the possibility to handle data from clinical notes and patients' records (12). Finally, Lee et al. tested their prediction on an out-of-distribution sample from another hospital. In ML model creation, it is paramount to bear in mind the risk of overfitting the data, creating a model that works very well in the tested population but poorly generalizes to other samples. In the quest to create a tool to implement in clinical practice, overfitting should be avoided. One of the most valuable ways to test the generalizability of a model is by using a completely distinct sample with different characteristics (9), as done by Lee et al..
As our knowledge of the genetic base of psychiatry disorders grows (13), it also increases the need to put these genes in the proper context, clustering them in biological pathways that can help us better understand the pathophysiology of psychosis. From this perspective, Liu et al. performed ML analyses to assess differential gene expression in individuals with schizophrenia and healthy controls, using RNA-seq data from the dorsolateral prefrontal cortex and amygdala. This approach led to an expansion of established schizophrenia-associated gene networks involved in the neurodevelopmental processes implicated in the neurobiology of schizophrenia, including glutamatergic, serotonergic, and dopaminergic synapse pathways, neuroactive ligand-receptor interaction networks, and cAMP signaling pathways.
As for genetics, imaging studies have taken great advantage from ML and strategies for automatic diagnostics in recent years (14). Not only classification (15) but also subtyping of psychotic disorders and prognostic studies (10) have been performed. Haas et al. used structural neuroimaging data to compute the brain age of patients with early-stage schizophrenia and healthy individuals and then clustered patients using multidomain cognitive data. In this way, they distinguished a cluster of cognitively impaired patients from cognitively spared individuals. Interestingly, both local and global brain age were significantly higher in the cognitively impaired population, suggesting that subjects with more severe symptoms also present a steeper decline through the years.
Finally, we are pleased to include articles discussing the challenges and pitfalls of these techniques, such as the perspective article by Smucny et al., who review methods to overcome problems with deep learning tools. Typically, deep learning methods need large datasets and are considered “black boxes,” obscuring their use in medical imaging applications. The authors describe three novel approaches that may help accelerate the incorporation of deep learning into the clinic. They first introduce two methods that can reduce the amount of training data required to develop accurate models: transfer learning and data augmentation. Next, they assess the complex topic of explainable artificial intelligence, tools that reveal what features (and in what combinations) deep learners use to make decisions. Such techniques might help resolve the “black box” problem, a critical step to validating ML tools in clinical practice.
Taken together, the papers included in this Research Topic illustrate how machine learning techniques have the potential not only to improve our understanding of the neurobiology of psychosis but also to contribute to crucial aspects of the clinical practice, such as diagnosis, prognosis, and treatment of this disorder.
All authors listed have made a substantial, direct, and intellectual contribution to the work and approved it for publication.
We acknowledge all the authors that contributed in the Research Topic.
The authors declare that the research was conducted in the absence of any commercial or financial relationships that could be construed as a potential conflict of interest.
All claims expressed in this article are solely those of the authors and do not necessarily represent those of their affiliated organizations, or those of the publisher, the editors and the reviewers. Any product that may be evaluated in this article, or claim that may be made by its manufacturer, is not guaranteed or endorsed by the publisher.
1. Jauhar S, Johnstone M, McKenna PJ. Schizophrenia. Lancet. (2022) 399:473–86. doi: 10.1016/S0140-6736(21)01730-X
2. Charlson FJ, Ferrari AJ, Santomauro DF, Diminic S, Stockings E, Scott JG, et al. Global epidemiology and burden of schizophrenia: findings from the global burden of disease study 2016. Schizophr Bull. (2018) 44:1195–203. doi: 10.1093/schbul/sby058
3. Pillinger T, D'Ambrosio E, McCutcheon R, Howes OD. Is psychosis a multisystem disorder? A meta-review of central nervous system, immune, cardiometabolic, and endocrine alterations in first-episode psychosis and perspective on potential models. Mol Psychiatry. (2019) 24:776–94. doi: 10.1038/s41380-018-0058-9
4. Nordentoft M, Wahlbeck K, Hällgren J, Westman J, Osby U, Alinaghizadeh H, et al. Excess mortality, causes of death and life expectancy in 270,770 patients with recent onset of mental disorders in Denmark, Finland and Sweden. PLoS ONE. (2013) 8:e55176. doi: 10.1371/journal.pone.0055176
5. Girgis RR. The neurobiology of suicide in psychosis: a systematic review. J Psychopharmacol. (2020) 34:811–9. doi: 10.1177/0269881120936919
6. Fusar-Poli P, Salazar de Pablo G, Correll CU, Meyer-Lindenberg A, Millan MJ, Borgwardt S, et al. Prevention of psychosis: advances in detection, prognosis, and intervention. JAMA Psychiatry. (2020) 77:755–65. doi: 10.1001/jamapsychiatry.2019.4779
7. Lieberman JA, Small SA, Girgis RR. Early detection and preventive intervention in schizophrenia: from fantasy to reality. AJP. (2019) 176:794–810. doi: 10.1176/appi.ajp.2019.19080865
8. Suvisaari J, Mantere O, Keinänen J, Mäntylä T, Rikandi E, Lindgren M, et al. Is it possible to predict the future in first-episode psychosis? Front Psychiatry. (2018) 9:580. doi: 10.3389/fpsyt.2018.00580
9. Dwyer DB, Falkai P, Koutsouleris N. Machine learning approaches for clinical psychology and psychiatry. Annu Rev Clin Psychol. (2018) 14:91–118. doi: 10.1146/annurev-clinpsy-032816-045037
10. Chand GB, Dwyer DB, Erus G, Sotiras A, Varol E, Srinivasan D, et al. Two distinct neuroanatomical subtypes of schizophrenia revealed using machine learning. Brain. (2020) 143:1027–38. doi: 10.1093/brain/awaa025
11. Pigoni A, Dwyer D, Squarcina L, Borgwardt S, Crespo-Facorro B, Dazzan P, et al. Classification of first-episode psychosis using cortical thickness: a large multicenter MRI study. Eur Neuropsychopharmacol. (2021) 47:34–47. doi: 10.1016/j.euroneuro.2021.04.002
12. Cohen AS, Rodriguez Z, Warren KK, Cowan T, Masucci MD, Edvard Granrud O, et al. Natural language processing and psychosis: on the need for comprehensive psychometric evaluation. Schizophr Bull. (2022) 48:939–48. doi: 10.1093/schbul/sbac051
13. Trubetskoy V, Pardiñas AF, Qi T, Panagiotaropoulou G, Awasthi S, Bigdeli TB, et al. Mapping genomic loci implicates genes and synaptic biology in schizophrenia. Nature. (2022) 604:502–8. doi: 10.1038/s41586-022-04434-5
14. Bassett DS, Sporns O. Network neuroscience. Nat Neurosci. (2017) 20:353–64. doi: 10.1038/nn.4502
Keywords: machine learning (ML), psychosis, schizophrenia, electronic health records, genetics
Citation: D'Ambrosio E, Abrol A and Pigoni A (2023) Editorial: Machine learning and psychosis: Diagnosis, prognosis and treatment. Front. Psychiatry 14:1133072. doi: 10.3389/fpsyt.2023.1133072
Received: 28 December 2022; Accepted: 06 February 2023;
Published: 21 February 2023.
Edited and reviewed by: Francisco Rodrigues, University of São Paulo, Brazil
Copyright © 2023 D'Ambrosio, Abrol and Pigoni. This is an open-access article distributed under the terms of the Creative Commons Attribution License (CC BY). The use, distribution or reproduction in other forums is permitted, provided the original author(s) and the copyright owner(s) are credited and that the original publication in this journal is cited, in accordance with accepted academic practice. No use, distribution or reproduction is permitted which does not comply with these terms.
*Correspondence: Alessandro Pigoni, YWxlLnBpZ29uaUBnbWFpbC5jb20=
Disclaimer: All claims expressed in this article are solely those of the authors and do not necessarily represent those of their affiliated organizations, or those of the publisher, the editors and the reviewers. Any product that may be evaluated in this article or claim that may be made by its manufacturer is not guaranteed or endorsed by the publisher.
Research integrity at Frontiers
Learn more about the work of our research integrity team to safeguard the quality of each article we publish.