- 1Substance Abuse Research Division, Department of Psychiatry and Behavioral Neurosciences, School of Medicine, Wayne State University, Detroit, MI, United States
- 2Sleep Disorders Center, Henry Ford Health System, Detroit, MI, United States
Background: Benzodiazepine (BZD) misuse is a significant public health problem, particularly in conjunction with opioid use, due to increased risks of overdose and death. One putative mechanism underlying BZD misuse is affective dysregulation, via exaggerated negative affect (e.g., anxiety, depression, stress-reactivity) and/or impaired positive affect (anhedonia). Similar to other misused substances, BZD consumption is sensitive to price and individual differences. Although purchase tasks and demand curve analysis can shed light on determinants of substance use, few studies have examined BZD demand, nor factors related to demand.
Methods: This ongoing study is examining simulated economic demand for alprazolam (among BZD lifetime misusers based on self-report and DSM-5 diagnosis; n = 23 total; 14 male, 9 female) and each participant’s preferred-opioid/route using hypothetical purchase tasks among patients with opioid use disorder (n = 59 total; 38 male, 21 female) who are not clinically stable, i.e., defined as being early in treatment or in treatment longer but with recent substance use. Aims are to determine whether: (1) BZD misusers differ from never-misusers on preferred-opioid economic demand, affective dysregulation (using questionnaire and performance measures), insomnia/behavioral alertness, psychiatric diagnoses or medications, or urinalysis results; and (2) alprazolam demand among BZD misusers is related to affective dysregulation or other measures.
Results: Lifetime BZD misuse is significantly (p < 0.05) related to current major depressive disorder diagnosis, opioid-negative and methadone-negative urinalysis, higher trait anxiety, greater self-reported affective dysregulation, and younger age, but not preferred-opioid demand or insomnia/behavioral alertness. Alprazolam and opioid demand are each significantly positively related to higher anhedonia and, to a lesser extent, depression symptoms but no other measures of negative-affective dysregulation, psychiatric conditions or medications (including opioid agonist therapy or inpatient/outpatient treatment modality), or sleep-related problems.
Conclusion: Anhedonia (positive-affective deficit) robustly predicted increased BZD and opioid demand; these factors could modulate treatment response. Routine assessment and effective treatment of anhedonia in populations with concurrent opioid and sedative use disorder may improve treatment outcomes.
Clinical trial registration: https://clinicaltrials.gov/ct2/show/NCT03696017, identifier NCT03696017.
1. Introduction
Although the opioid overdose epidemic continues to generate unprecedented numbers of deaths, medical, and epidemiological data clearly indicate these adverse outcomes are not solely due to over-consumption of opioids but often involve use of multiple substances (1–6). The Food and Drug Administration recognizes the health dangers of opioid/benzodiazepine (BZD) polysubstance use, and issued labeling changes for prescribing BZDs and opioids (7). However, the impact of such changes is minimal when people take a prescribed drug inconsistent with its labeling or use someone else’s prescription [e.g., (8)].
There has been limited systematic research on mechanisms underlying BZD/opioid polysubstance misuse [for review, (9)]. Although BZDs are often co-prescribed with opioids (10–13), there is substantial co-occurring use and misuse of opioids and BZDs (14–16). Whereas BZD misuse alone can be harmful, when combined with opioids, BZD misuse contributes dose-dependently to health-risk behaviors, poor treatment outcomes, overdoses and deaths (16–26).
Interpreting BZD misuse and consequences, particularly in the context of opioid misuse, is challenging. First, temporal patterns of opioid/BZD consumption are highly variable, ranging from simultaneous use (co-administration) to sequential use (one drug used within several hours before the other) to concurrent use (both drugs consumed during a broader temporal window, e.g., within a few days/weeks of one another). The behavioral mechanisms underlying these different co-use patterns are likely to differ. In fact, persons who co-use BZDs with opioids report several motives including managing anxiety, enhancing the drug “high,” promoting sleep, and suppressing opioid withdrawal (27–30). Second, BZD/opioid polysubstance use rarely occurs in isolation, i.e., persons using BZDs and opioids often use other psychoactive substances such as nicotine, alcohol, cannabis, and psychostimulants. Also, it is important to separate the psychopharmacological effects and consequences of BZD use from those of alcohol use, as these are highly comorbid (5, 31–33) and share similar reinforcing properties (34) and neurochemical mechanisms of action. A third interpretive challenge is that there are demographic differences in BZD/opioid polysubstance use. For example, BZD use and misuse is more common among women than men (26, 35), whites than other racial/ethnic groups (36), and among injection opioid users (26, 37); notably, the latter two factors are correlated, as some prior research has found opioid injectors are more likely to be white than black (38–40). Finally, several types of comorbidities can potentially modulate BZD/opioid use including anxiety-related symptoms/diagnoses (41–43), and sleep problems (9, 44, 45). A common assumption is that exaggerated negative affect plays a pivotal role in motivating BZD use to “self-medicate” anxious or depressive symptoms [i.e., negative reinforcement; (46)], however, this may not be the only functional relationship between psychiatric conditions and the reinforcing effects of BZDs.
Several theories of substance use disorders have outlined a central role of affective dysregulation and stress-reactivity (47–51). The present research builds on a dual-deficit theory of reward deficiency and stress surfeit in addiction (52). Our working hypothesis is that BZD/opioid polysubstance misuse may be perpetuated by a dual-deficit in hedonic regulation (difficulties modulating emotional reactions relative to the context and the person’s long-term goals). From the standpoint of clinical practice (which we emphasize more than etiological issues), we propose that this dual-deficit maintains polysubstance misuse and makes treatment more challenging. Further, we propose this dual-deficit biases motivated behaviors (predominantly guided by negative reinforcement processes), such that polysubstance use acutely blunts aversive states and directs actions away from natural rewards.
Benzodiazepine seeking/consumption, as for other misused substances, is sensitive to economic price. This process can be studied using self-administration (actual consumption) or hypothetical purchase tasks (simulated) and applying demand curve analysis to examine the intensity and elasticity of demand (53, 54), which can also be conceptualized as amplitude and persistence of demand, respectively (55). Alprazolam is a rapid-onset BZD that is frequently misused (56–59). Studies of rhesus monkeys have demonstrated that BZDs are self-administered, however, economic demand for BZDs is complexly related to a compound’s selectivity and intrinsic efficacy at α1 subunit-containing GABAA receptors, as well as the animal’s baseline history of self-administration (60–64). Therefore, it is reasonable to use a standard, often-misused BZD such as alprazolam to investigate individual difference in BZD demand. Recently, it was shown that alprazolam functioned as a reinforcer in three of six monkeys tested. For two of those three alprazolam self-administering animals, alprazolam enhanced self-administration of fentanyl whereas for the other monkey alprazolam self-administration suppressed fentanyl intake (65). These data highlight the importance of individual differences in the reinforcing effects of BZDs and opioids; however, we presently have limited understanding of the reasons underlying these differences.
To our knowledge, only three clinical studies have used hypothetical purchasing tasks to investigate BZD demand, although none specifically with alprazolam. Petry and Bickel (66) studied 40 persons undergoing treatment for heroin use disorder. Among several price and income manipulations, they found that diazepam (the only BZD studied) substituted for heroin, whereas heroin purchases were independent of diazepam prices, suggesting an asymmetrical substitution effect. This indicates that diazepam is reinforcing in persons addicted to heroin but does not specify for what reason(s). In a separate study, Petry (67) also reported that diazepam demand was price-elastic among individuals with DSM-IV alcohol abuse/dependence and a history of polysubstance use. Recently, Schwartz et al. (68) studied 52 persons in outpatient opioid agonist treatment for opioid use disorder at a baseline visit and a 6-month follow-up visit; they found that demand intensity for BZD pills (not specified) increased across time points and was predictive of BZD-positive urine samples.
In summary, we lack data on factors that influence BZD demand, alone and especially in the context of opioid use disorder. Importantly, group factors can be included in demand curve analyses to examine individual difference variables that modulate BZD consumption. Accordingly, the present study aims to investigate: (1) among persons in treatment for opioid use disorder, whether lifetime or past-year BZD misusers differ from never-misusers on measures of simulated opioid demand (co-primary outcome), affective dysregulation (e.g., psychiatric diagnoses, anhedonia, distress tolerance), and insomnia/daytime sleepiness; and (2) in the subgroups of lifetime and past-year BZD misusers, whether simulated BZD demand (co-primary outcome) is specifically associated with affective dysregulation, controlling for other factors.
2. Materials and methods
2.1. Study context
The local IRB approved all research procedures. This ongoing study is being conducted according to the Declaration of Helsinki and is registered at ClinicalTrials.gov (NCT03696017). All participants provided informed consent.
2.2. Participant selection
This study assesses patients currently in treatment (baseline visit) for their opioid and potentially other substance use disorder(s) who are not presently clinically stable, which we defined a priori as early (first 6 months) in treatment or in treatment longer but self-report having used opioids during the past month. As this programmatic research is thematically focused on BZD/opioid polysubstance use, we attempted to recruit a sample enriched with individuals with a history of BZD misuse in addition to their opioid misuse; however, we did not explicitly require a history of, or current, use of BZDs to be enrolled in this study.
First, we defined BZD misuse history based on two lifetime factors, either: (1) any BZD misuse based on a “yes” response to the question, “Have you ever used sedatives/hypnotics not as prescribed intending to get high,” on the Drug History and Use Questionnaire DHUQ (described in Section “2.3.4. Substance use”), or (2) diagnosis of sedative use disorder involving a BZD based on the SCID diagnostic interview (described in Section “2.3.5. Psychopathology and affective dysregulation”). Any participant meeting at least one of these two criteria was classified as a lifetime BZD misuser, and any participant not meeting either criterion was classified as a BZD never-misuser. Importantly, any participant who reported using BZDs as exactly prescribed for them throughout their lifetime, and denied misuse, was classified as a never-misuser. Second, to account for possible temporal variation in the effects of BZD misuse or abstinence, we defined differences in recency of BZD misuse as either (1) more than 1 year ago, or (2) within the past year, relative to the date of the initial screening visit. Participants who reported BZD misuse more than 1 year prior, or met DSM-5 criteria for partially remitted or past sedative use disorder were classified as misusers more than a year ago. Participants who reported BZD misuse within the past year, or met DSM-5 criteria for current (past-year) sedative use disorder involving a BZD, were classified as past-year misusers. Thus, we formed three distinct groups for analyses: (1) never misuse, (2) misuse > 1 year ago, and (3) past-year misuse of BZDs.
All participants are adults, ages 18–70 years old enrolled in a substance use disorder treatment program (outpatient or residential) in the Detroit metropolitan region. Exclusion criteria were estimated IQ < 80, expired breath alcohol > 0.02% breath alcohol concentration, neurological disorders that affect cognition, and current psychosis or suicidality. This study is also approved to re-contact participants (in-person or remotely) for 3-month follow-up assessment; these follow-up data will be reported elsewhere.
2.3. Experimental assessments
2.3.1. Hypothetical opioid and benzodiazepine purchase tasks
A simulated Opioid Purchasing Task is tailored to each participant’s preferred opioid and route of administration (e.g., injected, snorted, oral) based on screening self-report. Of the 59 total participants, 46 reported using heroin (22 snorted, 23 injected, 1 smoked), 1 snorted fentanyl, 10 took oral hydrocodone, and 2 took oral oxycodone. The purchasing task is modeled after extant purchasing tasks for various substances [e.g., (69–71)], but personalizing the task for specific opioids/routes is novel. Participants are asked to imagine a typical day, with no access to other opioids unless they buy the preferred opioid at the listed prices. Participants make purchasing choices based on instructions that the amount purchased at each unit price (independent observations) must be consumed within 24-h (i.e., no saving or stockpiling drug). Prices per morphine 10-mg equivalent dose are $0 (free; no constraint) and 20 non-zero unit prices of $0.01, $0.10, $0.50, $1, $3, $5, $7.50, $10, $12.50, $15, $20, $25, $30, $35, $40, $45, $50, $60, $80, and $100. The participant indicates on a standard form how many unit doses s/he would purchase (dependent variable) at each unit price (independent variable).
A parallel simulated BZD Purchasing Task uses similar instructions and unit prices for alprazolam (0.25-mg equivalent oral dose): $0 (free), and $0.01, $0.10, $0.50, $1, $3, $5, $7.50, $10, $12.50, $15, $20, $25, $30, $35, $40, $45, $50, $60, $80, and $100. The participant indicates on a standard form how many unit doses s/he would purchase at each unit price. Among the 37 BZD misusers in this sample (11 of whom endorsed a prior prescription), 15 reported misuse of two or more BZDs across their lifetime (concurrent past-month misuse of multiple BZDs was infrequent): 25 endorsed ever misusing alprazolam (Xanax™), 13 endorsed misusing diazepam (Valium™), 11 endorsed misusing clonazepam (Klonipin™), and 6 endorsed misusing lorazepam (Ativan™), and 3 (who misused in the past month) did not identify the specific BZD(s) by name. All participants reported misuse of these BZDs only via the oral route of administration (e.g., no snorting or injection). Thus, use of an oral alprazolam purchasing task was appropriate in this participant sample.
2.3.2. Demographics
Information on age, educational level/degree, and self-identified sex, race, and ethnicity are obtained via self-report. Estimated verbal intelligence is obtained by administering the Shipley Institute of Living Scale (72).
2.3.3. Type of treatment
Standardized forms are used to collect information on type of treatment facility (acute or longer-term residential, transitional, day program, or other outpatient), type of medication for opioid use disorder [grouped as agonist therapy (methadone and buprenorphine) vs. no agonist therapy (naltrexone and no medication)], and other non-substance use disorder treatment medications (e.g., for anxiety, depression, sleep, pain).
2.3.4. Substance use
Substance use is evaluated with a comprehensive Drug History and Use Questionnaire developed in our laboratory (available on request); it is used (either via paper/pencil or Qualtrics administration) to assess lifetime substance use (e.g., onset of use, regular use of opioids and BZDs and other substances, adverse consequences of substance use, number of quit attempts). This instrument also is used to determine the relative timeline of opioid and BZD use (prescribed or not), misuse and progression.
Biomarkers of recent substance use include alcohol breath testing and urine drug screening. Participants must provide a supervised alcohol-free breath sample (<0.02% BAC; AlcoSensor Intoximeter). A urine sample is collected into multi-test cups with temperature strips (CLIA Waived; temperature must be 92–96° F). Samples are tested for opioids, methadone, cocaine metabolites, benzodiazepines, amphetamines, barbiturates (negative cutoff < 300 ng/ml), and THC (negative cutoff < 50 ng/ml). After the study began, we initiated fentanyl urinalysis using test strips; however, at this time, too few participants have data for this measure.
2.3.5. Psychopathology and affective dysregulation
The Semi-Structured Clinical Interview for DSM-5 [SCID; (73, 74)] is used to evaluate lifetime and current psychiatric and substance use disorders. The SCID is administered by a trained clinical psychology masters level student, supervised by co-author LHL.
Anhedonia, the reduced experience or anticipation of pleasure (75, 76) linked to dopamine-mediated reward dysfunction and drug craving (77–80), is measured with the validated 14-item Snaith-Hamilton Pleasure Scale [SHAPS; (81, 82)]. Individuals are asked about their agreement with 14 statements; example items include: “I would be able to enjoy my favorite meal” (food/drink), “I would enjoy seeing others’ smiling faces” (social interaction), “I would be able to enjoy a beautiful landscape or view” (sensory experience), and “I would find pleasure in my hobbies and past-times” (interest/past-times), which is consistent with a recent conceptualization of anhedonia as having multiple domains, although these are not yet well understood (83). Each statement receives a score of either 0 (definitely agree or agree) or 1 (definitely disagree or disagree). High scores reflect the participant’s disagreement with the item statement (i.e., inability to experience pleasure from the event). Notably, most healthy individuals score < 2 (low anhedonia), whereas psychiatric patient samples often score 2 or higher.
The Beck Depression Inventory-II (BDI-II) is a gold-standard, 21-item clinical measure of current (past 2-week) depression symptoms validated against the original version (84) and in low-income African-Americans (85) and substance users (86). Guidelines for BDI-II cutoff scores are that: 0–13 indicates no or minimal depression; 14–19 indicates mild to moderate depression; 19–28 indicates moderate to severe depression; and 29–63 indicates severe depression (87).
The State-Trait Anxiety Inventory (STAI) (88) is a well-validated 40-item measure that differentiates symptoms of state anxiety (Y1 scale) from chronic trait anxiety (Y2 scale) by evaluating agreement with each item on a four-point Likert scale.
The 14-item Perceived Stress Scale (PSS) (89) measures the degree to which the subject views past-month life situations as stressful. It is reliable and correlates with self-report and behavioral criteria.
The 36-item Difficulties with Emotion Regulation Scale (DERS) (90) measures six empirically valid constructs related to emotion dysregulation: Non-acceptance of emotional responses, Difficulties in engaging in goal-directed behavior, Impulse control difficulties, Lack of emotional awareness, Limited access to emotion regulation strategies, and Lack of emotional clarity.
The 20-item Alcohol and Drug Use Self-Efficacy Scale (ADUSE) (91) assesses self-efficacy and responses to high-risk situations that can trigger substance use. Items are grouped into negative affect, social positive withdrawal/urges, and physical/other concerns; subjects indicate how “tempted” and “confident” they would be in each situation.
Distress tolerance, defined as the perceived capacity to tolerate distress and interpreted here as the ability to remain drug abstinent in the face of difficulties (92–94) is measured with the Distress Tolerance Scale which has 15 items with good construct validity and reliability (95).
We include two performance measures putatively related to affective dysregulation. The Paced Auditory Serial Addition Task (PASAT) (96) is a mental arithmetic task that measures processing speed and flexibility during which participants must add each new digit to the one presented immediately prior. We used three trial blocks of increasing difficulty such that the presentation rate of numbers that must be held in memory and added increases within each trial block. Participants can quit performing the task during trial block three; performance accuracy and latency to task termination are outcome measures. In the Emotional Stroop Test (97), words presented (in different colors) vary in their affective meaning: neutral, pleasant, negative, aggressive. The participant is instructed to identify (by key-pressing) the color of the printed word; response accuracy and latency (ms) are outcome measures.
2.3.6. Insomnia and behavioral alertness
The 7-item Insomnia Severity Index (ISI) asks about problem severity of sleep-onset, sleep-maintenance, early morning awakening, sleep satisfaction, interference with daily function, perceived impairment, and level of distress from insomnia. It has good internal consistency and concurrent validity (with polysomnography, sleep diaries, and clinician or significant-other reports), making it a valid and reliable measure of perceived sleep disturbance (98, 99).
The 8-item Epworth Sleepiness Scale (ESS) measures “sleep propensity,” i.e., recent likelihood of dozing or falling asleep (rather than just feeling tired) in several situations (100). It is reliable and some items correlate with the gold-standard Multiple Sleep Latency Test.
The Psychomotor Vigilance Task (PVT) (101, 102) is a computerized, adaptive task (reaction time to a visual stimulus presented at random inter-trial intervals) that is used to assess attentional lapses; this objective, validated measure of sleepiness will complement the ISI and ESS measures.
2.4. Data analysis
Economic demand curve analysis is used to estimate the amounts of each participant’s preferred-opioid and, for lifetime BZD misusers, alprazolam consumed across increasing unit prices. Specifically, we measure each participant’s demand intensity (amplitude at low prices) and elasticity (resistance to price increases) based on the number of opioid $10 units purchased/consumed, in relation to opioid unit prices ranging from $0.01–$100.00. For participants with lifetime BZD misuse history, we also measure demand intensity and elasticity for alprazolam 0.25 mg units in relation to alprazolam unit prices, also ranging from $0.01–$100.00.
Hypothetical purchase task data were screened for unsystematic responses. Two curves that were unsystematic (one opioid, one BZD) were removed from analyses; this low proportion of data removal is similar to rates reported in prior studies. Each participant’s hypothetical purchase data were entered into a GraphPad Prism template1. Consumption values were transformed using the inverse hyperbolic sine transform (IHS; Equation 1 below) which is approximately log-equivalent for consumption values > 5 and for values < 5 converges to zero, such that zero consumption values can be included in analyses. Curves were fit with both non-normalized and normalized versions of the zero-bounded exponential model of demand (103):
In this model, Q is consumption, Q0 is consumption at unit price = 0 (demand intensity), x is unit price, and α is a free parameter that indexes the rate of change of the curve slope. This model accounts for these data which included many instances of reported zero consumption, and preserves the log-like scaling that represents relative changes in consumption with relative changes in unit price, i.e., the definition of elasticity.
Each model (opioid and BZD) was first used to estimate intensity of demand (Q0) and demand elasticity (α) and curve fit (r2), separately for each participant, in GraphPad Prism. The model also automatically calculates “essential value” [EV; (54)], which is proportional to the inverse of α [EV = 1/(100 × α)], and easily communicates the rate of change in elasticity, namely, higher EV reflects greater resistance to the (typical consumption-decreasing) effect of increasing unit prices.
As these study participants were in substance use disorder treatment, it is unsurprising that some individuals indicated no demand for opioids (n = 15 of 58) or alprazolam (n = 7 of 23) by providing all-zero consumption values across unit prices (i.e., non-participation). For these curves, Q0 and EV were recoded as 0; in these cases, the α parameter was treated as undefined/missing because it was infinitely high, reflecting low demand (104), and we used the EV parameter instead of α to retain a larger sample size for analysis.
For both opioid and alprazolam purchasing tasks, the binary variable “participation” (i.e., making non-zero vs. all-zero responses) and continuous parameters r2, Q0, α, and EV from demand modeling for each participant were exported into SPSS v27 to examine subgroup differences. Zero-bounded exponential modeling in GraphPad Prism was also used to generate subgroup-average demand curves for plotting (see figure captions for results of group-average curve fits).
For Aim 1, ANOVAs and chi-square tests were used to examine BZD misuse history group (never, >1 year ago, and past-year) differences in demographic, opioid use disorder treatment type, psychiatric diagnoses/medications, urinalysis results, medications, experimental opioid demand, affective dysregulation, and sleep-related measures. For Aim 2, ANOVAs, correlations, and multiple linear regression were used to examine associations of affective dysregulation and other measures with experimental BZD demand metrics.
3. Results
Figure 1 presents the CONSORT diagram for participant flow through the experimental procedures.
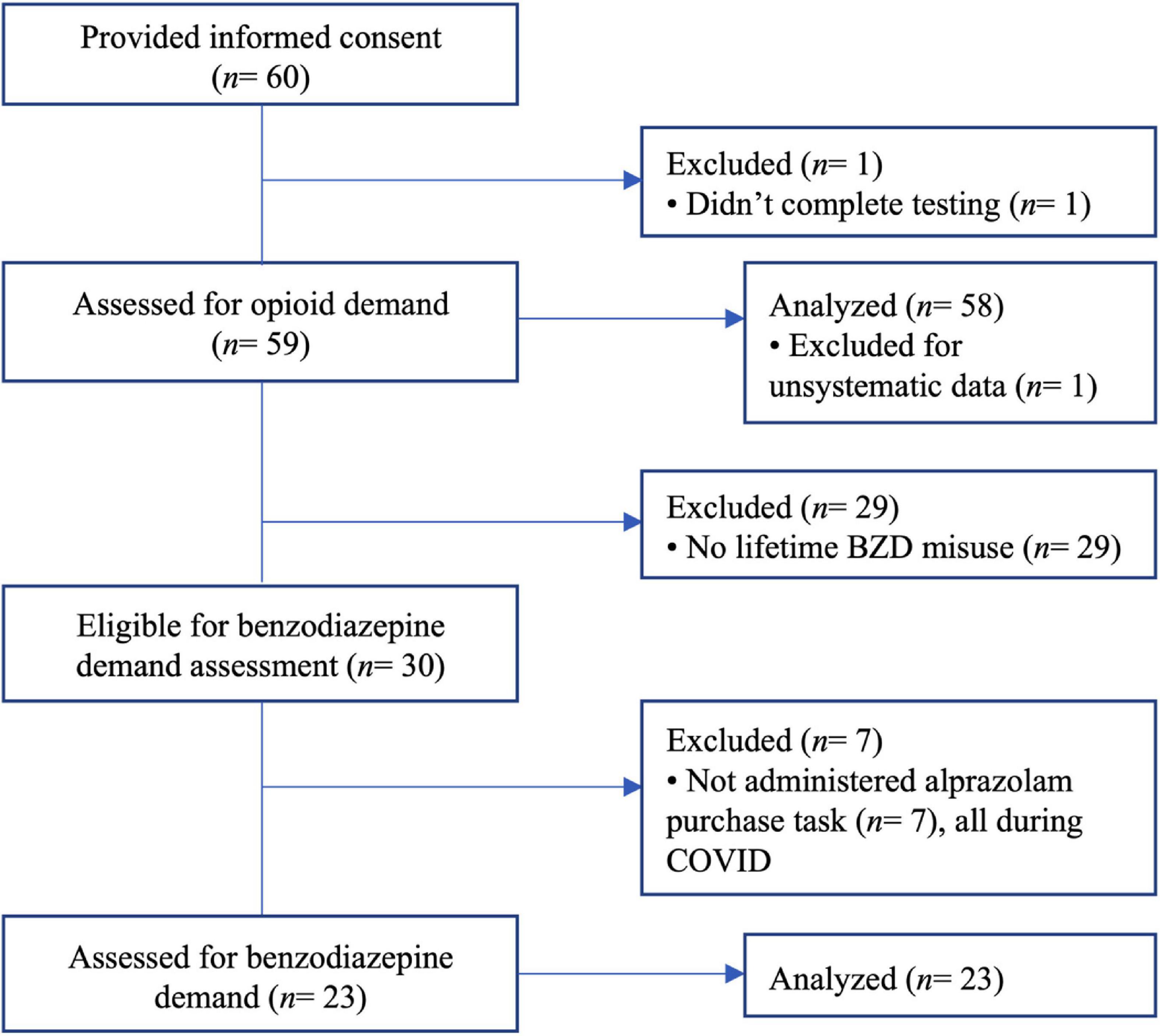
Figure 1. CONSORT diagram illustrating the flow of participants through the experimental procedures.
3.1. Aim 1: Differences between BZD misusers and never-misusers
Table 1 presents characteristics for the overall sample (n = 59) and by subgroups of participants who denied lifetime BZD misuse (n = 22), who misused BZDs > 1 year ago (n = 17) and who misused BZDs within the past year (n = 20), based on self-report from the Drug History and Use Questionnaire and SCID interview-based diagnosis of sedative disorder (see Section “2.2. Participant selection” for details). The subgroups significantly (p < 0.05) differed on several measures. Relative to never-misusers, lifetime BZD misusers (past-year and >1 year groups did not differ) were younger, more likely to be diagnosed with current major depressive disorder (with trends toward more depression symptoms on the BDI-II and likelihood of taking an antidepressant medication), and to present a urine sample that was opioid-negative and methadone-negative (with a trend toward more cocaine-negative samples).
Relative to never-misusers, lifetime BZD misusers reported significantly higher scores for trait anxiety (STAI Y2 scale) and emotion regulation problem (DERS). Unexpectedly, lifetime BZD misusers had more correct responses and were less likely to quit task performance under cognitive duress (PASAT), and had faster response latencies during positive and negative affective interference trials (Emotional Stroop task). However, covariance analyses (ANCOVA) with age–which differed between BZD-misuser and never-misuser groups (see Table 1), found that these group differences in task performance were no longer significant, i.e., older age more parsimoniously explained longer response latencies (Stroop) and less accurate performance and more task quitting (PASAT). There were no other BZD misuse group differences on other measures, and presenting a BZD + urine sample was not associated with these measures.
Opioid demand curve fits were very high: 54 of 58 participants had r2 values > 0.80. Table 1 indicates that, based on the primary SPSS analysis of parameters that were computed from each participant’s demand curve (i.e., units of analysis), opioid demand intensity and essential value did not significantly differ for lifetime BZD misusers (>1 year ago or past-year) vs. never-misusers.
In contrast, higher SHAPS anhedonia scores were significantly positively correlated with higher intensity of opioid demand (Q0, r = 0.59, p < 0.001), but not essential value (r = 0.19, p = 0.160). To refine the interpretation of these effects, participants were stratified into three groups based on SHAPS total scores (0, 1, or 2+), consistent with previous clinical studies and the observed distribution of scores in the present sample. Table 2 (upper section) and Figure 2A illustrate that those participants with SHAPS scores ≥ 2 had significantly higher opioid demand intensity, but not essential value, compared to subgroups with lower SHAPS scores. SHAPS scores and BDI-II scores were significantly correlated in the overall sample (r = 0.56, p < 0.001). Compared to SHAPS scores, BDI-II depression symptom scores showed a similar but weaker positive association with opioid demand intensity (r = 0.39, p = 0.002) and were not significantly associated with essential value (r = 0.20, p = 0.139).
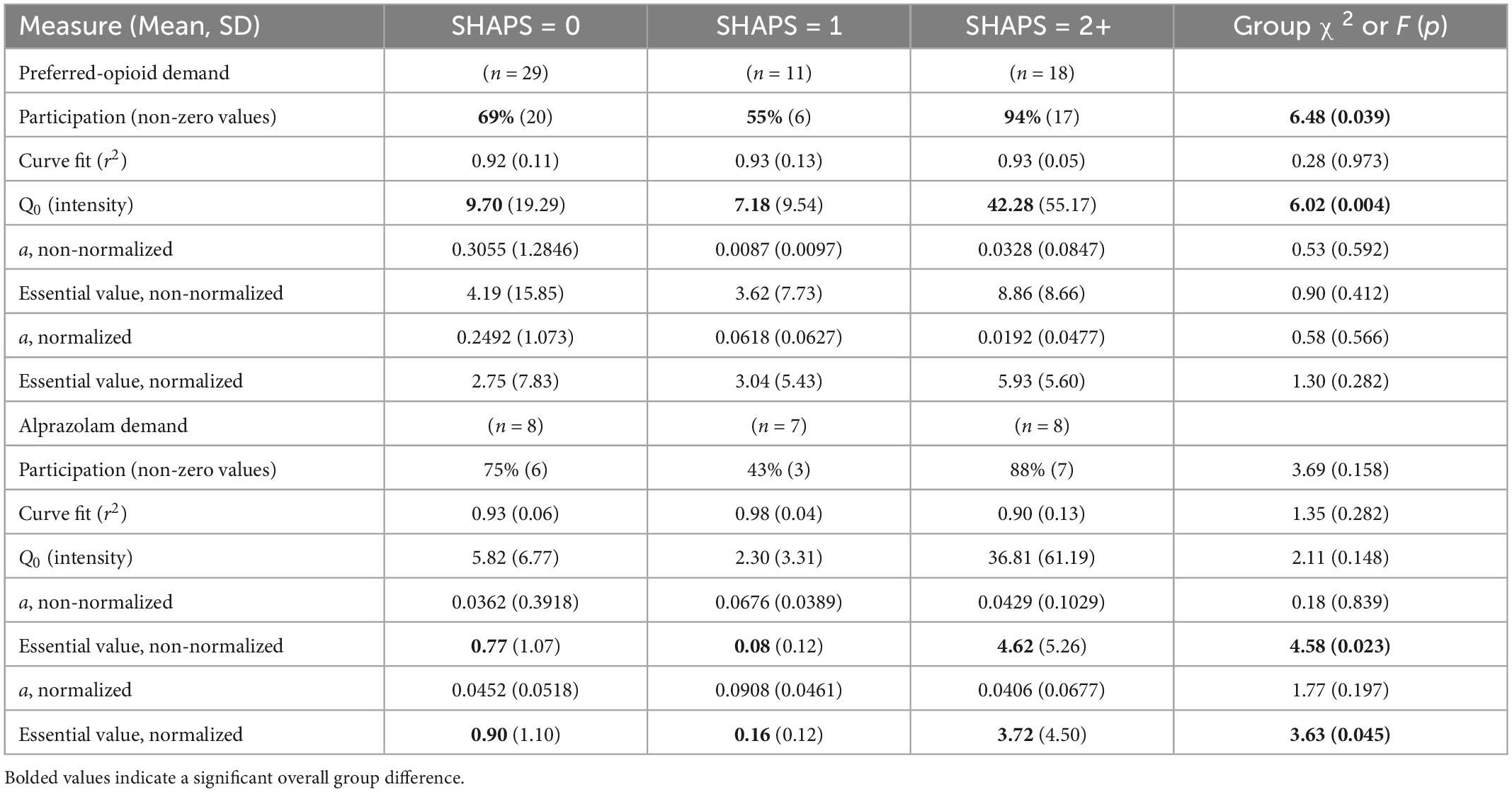
Table 2. Parameter estimates for opioid and alprazolam demand, stratified by Snaith-Hamilton Pleasure Scale (SHAPS) anhedonia total scores.
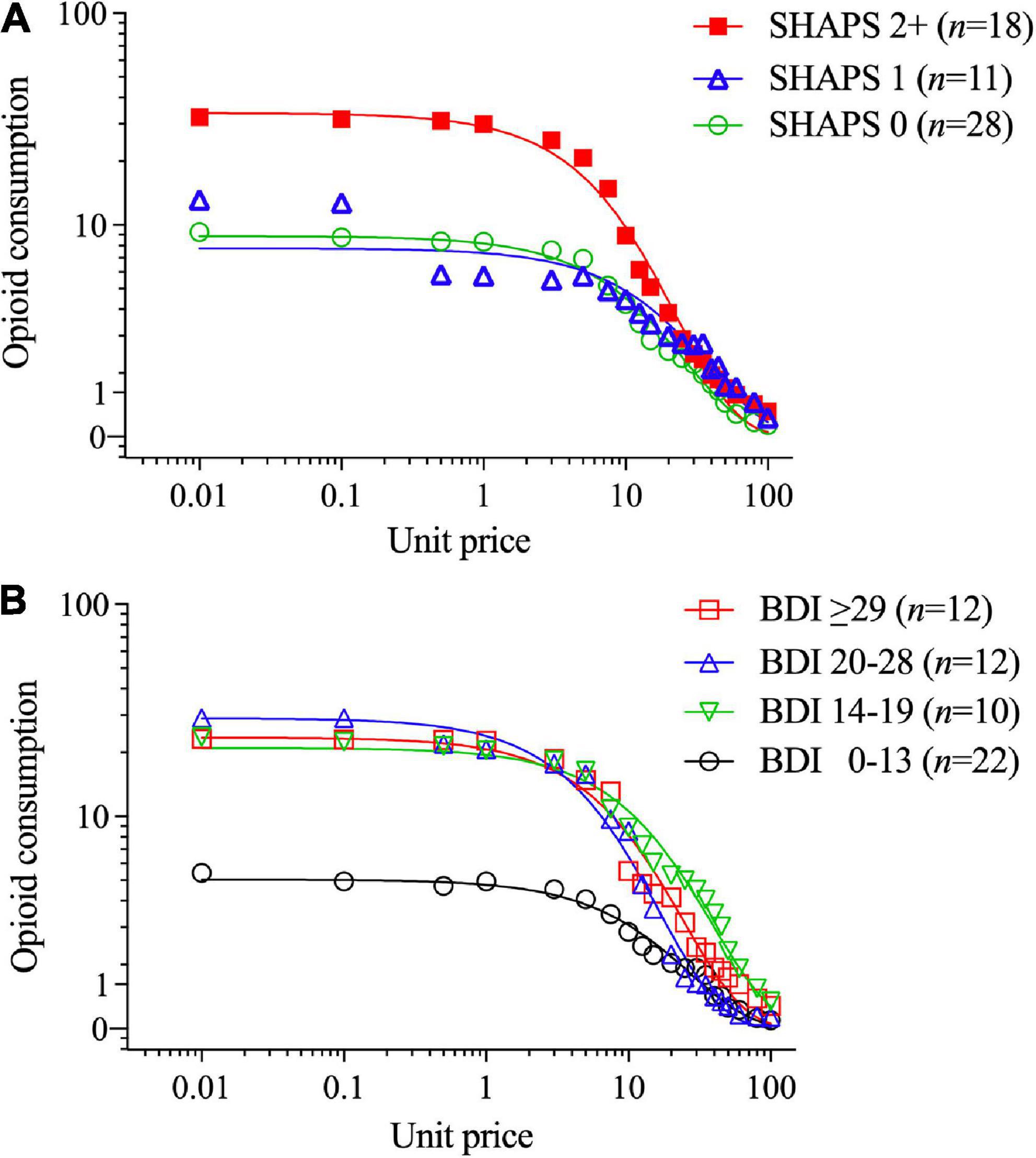
Figure 2. Opioid demand stratified by (A) Snaith-Hamilton Pleasure Scale (SHAPS) anhedonia total scores (0 vs. 1 vs. 2+) and (B) Beck Depression Inventory-II (BDI-II) scores (using clinical cutoff values). The primary SPSS analysis found significant SHAPS anhedonia group differences in opioid demand (see text, Section “3.1. Aim 1: Differences between BZD misusers and never-misusers”), and parameters computed in GraphPad Prism from group-average curves in Figure 1A confirm that the subgroup with SHAPS scores ≥ 2 compared to scores of 1 or 0 had higher opioid demand intensity (Q0 = 33.7 vs. 7.64 vs. 8.75, respectively), and essential value (EV = 7.75 vs. 2.98 vs. 2.36, respectively), F(1,18) = 84.2. The primary SPSS analysis did not find a significant BDI-II group difference in opioid demand intensity or essential value; however, parameters computed in GraphPad Prism from group-average curves in Figure 1B found that BDI-II scores indicating mild or greater depression severity (≥14) were associated with higher opioid demand intensity (Q0 = 4.9, 21.0, 29.0 and 23.5 for groups with scores of 0–13, 14–19, 20–28, and ≥ 29, respectively) and essential value (EV = 1.32, 8.24, 4.91, and 5.75, respectively), F(1,18) = 115.
Figure 2B illustrates that those participants with BDI-II depression symptom scores ≥ 14 (i.e., mild or greater depression severity) exhibited higher levels of opioid demand than those with lower BDI-II scores [≤13 indicates no clinical concern (87)]. SHAPS scores significantly correlated with several other measures of affective dysregulation (Table 3), however, these other measures were not related to opioid demand.
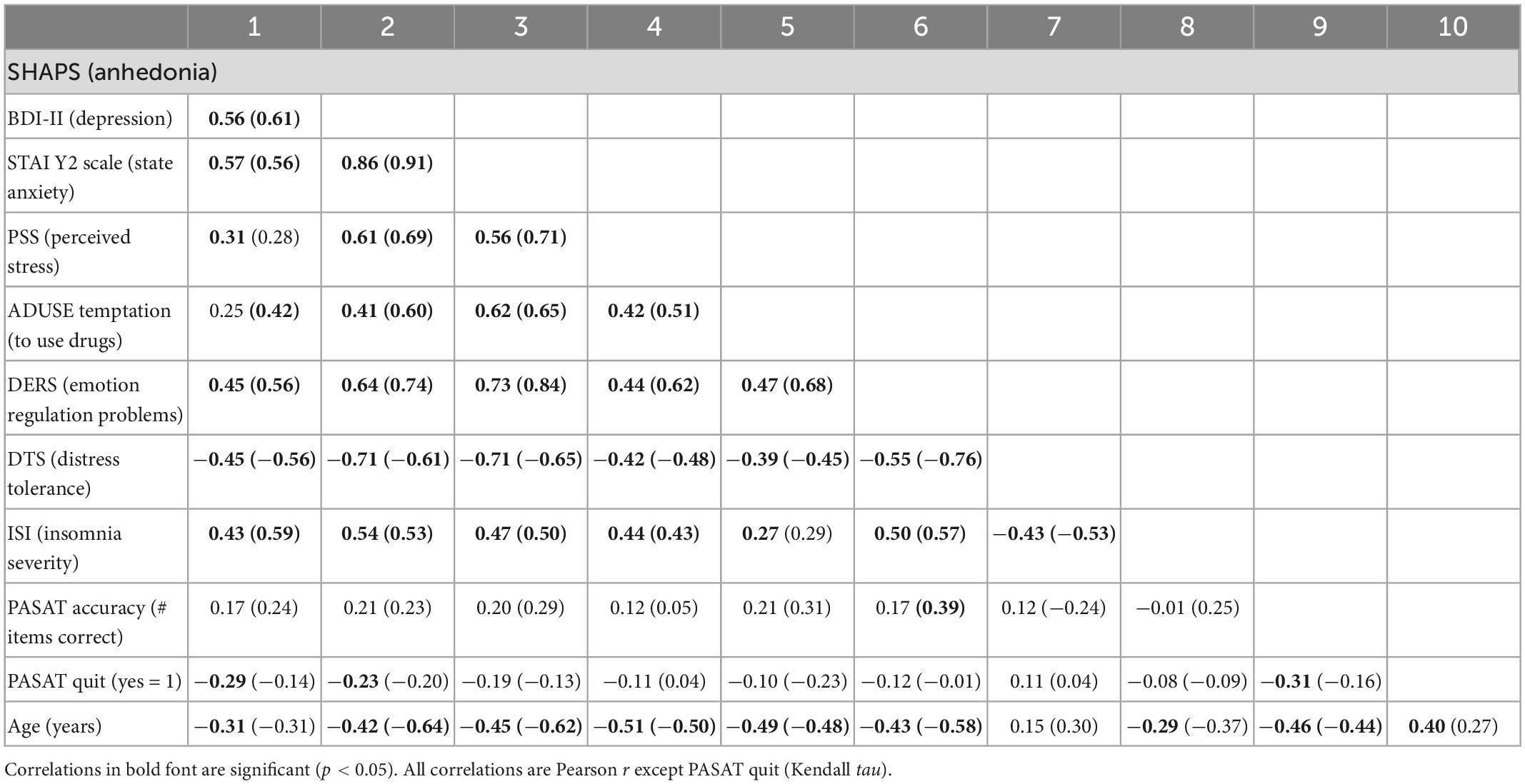
Table 3. Correlations between selected measures of affective dysregulation, insomnia severity and age in the overall sample (N = 59), and in parentheses, the subgroup of lifetime benzodiazepine misusers (n = 23).
A multiple stepwise linear regression model with these two predictors found that only SHAPS anhedonia scores significantly predicted opioid demand intensity (standardized beta = 0.593, t = 5.46, p < 0.001) and explained 34.0% of the variance (adjusted r2), F(1,55) = 29.79, p < 0.001. SHAPS scores significantly correlated with younger age (r = −0.31, p = 0.018) and lower scores on the DTS (r = −0.42, p = 0.001), and with higher scores on STAI Trait Anxiety (r = 0.56, p < 0.001), and DERS (r = 0.45, p < 0.001), ISI (r = 0.45, p < 0.001), and PSS (r = 0.33, p = 0.008). Importantly, SHAPS scores singularly and significantly predicted opioid demand intensity when controlling for all these covariates, although adjusted r2 decreased to 23.3%, standardized beta = 0.497, t = 4.13, p < 0.001, F(1,52) = 17.06, p < 0.001.
In exploratory analyses, opioid demand metrics did not significantly differ when comparing males (n = 36) vs. females (n = 21), opioid injection users (n = 23) vs. non-injection users (n = 34), participants on opioid agonist therapy (methadone or buprenorphine, n = 41) vs. no agonist therapy (naltrexone or no medication, n = 17), participants in outpatient treatment (n = 44) vs. residential treatment (n = 9), nor participants with positive vs. negative urinalysis results, or presence/absence of substance use disorder and mental health diagnoses.
3.2. Aim 2: Differences within lifetime BZD-misusing subgroups
Among lifetime BZD misusers, alprazolam demand curve fits were very high: 22 of 23 participants had r2 values > 0.80. SHAPS anhedonia and BDI-II depression symptom scores, which were correlated in the overall sample, remained significantly correlated in this subgroup (r = 0.61, p < 0.001). In bivariate analyses, alprazolam demand intensity (Q0) significantly correlated with SHAPS scores (r = 0.69, p < 0.001) and marginally with BDI-II scores (r = 0.40, p = 0.058); and alprazolam essential value (EV) significantly correlated with both SHAPS scores (r = 0.46, p = 0.027) and BDI-II scores (r = 0.44, p = 0.034). SHAPS scores significantly correlated with several other measures of affective dysregulation in the subgroup of past-year BZD users (Table 3), however, these other measures were not related to alprazolam demand intensity or essential value. Although participant age was related to measures of affective dysregulation, age was not significantly related to BZD demand intensity or essential value. As we did for opioid demand, the same clinical cut-points were used to form SHAPS and BDI-II subgroups.
Table 2 (lower section) and Figure 3 illustrate that those participants with SHAPS scores ≥ 2 (Figure 3A) and BDI-II scores ≥ 20 (indicating moderate to severe depression levels; Figure 3B) exhibited differences in alprazolam demand. A multiple stepwise linear regression model with these two predictors found that only SHAPS scores significantly predicted alprazolam demand intensity (standardized beta = 0.691, t = 4.38, p < 0.001) and explained 45.2% of the variance (adjusted r2), F(1,21) = 19.14, p < 0.001. A multiple stepwise linear regression model with these two predictors found that only SHAPS scores significantly predicted alprazolam essential value (standardized beta = 0.462, t = 2.39, p < 0.027) and explained 17.6% of the variance (adjusted r2), F(1,21) = 5.70, p < 0.027.
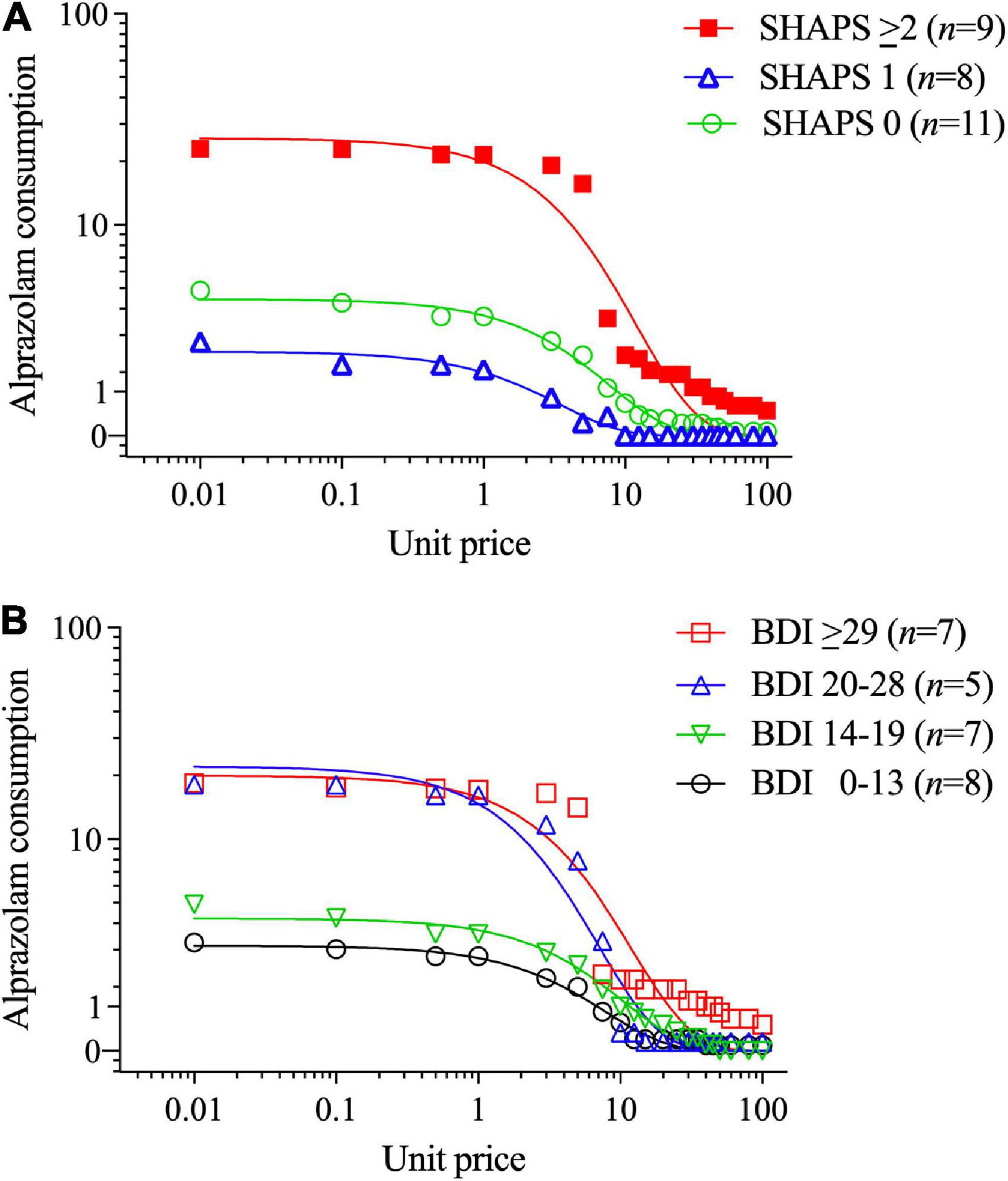
Figure 3. Alprazolam demand stratified by (A) Snaith-Hamilton Pleasure Scale (SHAPS) anhedonia total scores (0 vs. 1 vs. 2+), and (B) Beck Depression Inventory-II (BDI-II) depression total scores (using clinical cutoff values). The primary SPSS analysis found that participants with higher SHAPS anhedonia scores (≥2) and higher BDI-II depression symptom scores had significantly higher alprazolam demand intensity (see Section “3.2. Aim 2: Differences within lifetime BZD-misusing subgroups”). Parameters computed in GraphPad Prism from group-average curves in Figure 2A confirm that the subgroup with SHAPS scores ≥ 2 compared to scores of 1 or 0 had higher alprazolam demand intensity (Q0 = 25.7 vs. 2.11 vs. 4.22, respectively), and essential value (EV = 3.11 vs. 0.074 vs. 0.34, respectively), F(1,18) = 78.9. The primary SPSS analysis did not find a significant BDI-II group difference in alprazolam demand intensity or essential value; however, parameters computed in GraphPad Prism from group-average curves in Figure 2B found that progressively increasing BDI-II scores (0–13, 14–19, 20–28, and ≥ 29) were associated with monotonically increasing alprazolam demand intensity (Q0 = 1.92, 5.98, 15.7 and 19.9, respectively) and essential value (EV = 0.26, 0.37, 1.26, and 2.40, respectively), F(1,18) = 74.7.
Alprazolam demand metrics did not significantly differ when comparing males (n = 14) vs. females (n = 9), injection opioid users (n = 11) vs. non-injection users (n = 12), participants on opioid agonist therapy (n = 13) vs. no agonist therapy (n = 10), those in outpatient treatment (n = 14) vs. residential treatment (n = 8), nor participants with positive vs. negative urinalysis results. Notably, presenting a BZD + urine sample (n = 7), reflecting recent use, was not significantly related to alprazolam demand. Although presence/absence of substance use disorder diagnoses and some mental health diagnoses was unrelated to alprazolam demand, there were two exceptions. First, presence (n = 8) vs. absence (n = 14) of major depressive disorder diagnosis was associated with greater alprazolam essential value (mean EV = 4.04 vs. 0.80), F(1,20) = 4.50, p = 0.047, with a trend toward higher demand intensity (mean Q0 = 36.5 vs. 4.5), F(1,20) = 3.90, p = 0.062, as well as higher symptom scores on SHAPS anhedonia (M = 3.75 vs. 0.57), F(1,20) = 18.70, p < 0.001, and BDI-II depression (M = 29.3 vs. 14.1), F(1,20) = 12.96, p = 0.002. Second, presence (n = 7) vs. absence (n = 15) of PTSD diagnosis was associated with greater alprazolam demand intensity (mean Q0 = 41.8 vs. 4.2), F(1,20) = 5.35, p = 0.032, and higher symptom scores on SHAPS anhedonia (M = 3.43 vs. 0.93), F(1,20) = 7.56, p = 0.012, and BDI-II depression (M = 31.3 vs. 14.2), F(1,20) = 17.84, p < 0.001.
Benzodiazepine and opioid demand intensities were highly positively correlated (r = 0.98, p < 0.001; Figure 4A), as were BZD and opioid essential values (r = 0.86, p < 0.001; Figure 4B) and choice participation (χ2 = 27.00, p < 0.001). Regression slopes within each panel of Figure 4 indicate that opioid demand metrics were proportionally greater for the preferred-opioid than alprazolam. Repeated measures ANOVAs found that demand intensity was non-significantly higher for the preferred-opioid than alprazolam (Q0 = 16.1 vs. 15.5), F(1,22) = 3.98, p = 0.085; whereas, essential value was significantly higher for the preferred-opioid than alprazolam (EV = 4.10 vs. 1.90), F(1,22) = 6.83, p = 0.016. Figure 4C illustrates average demand curves (in the BZD-misusing group) for both the preferred-opioid and alprazolam.
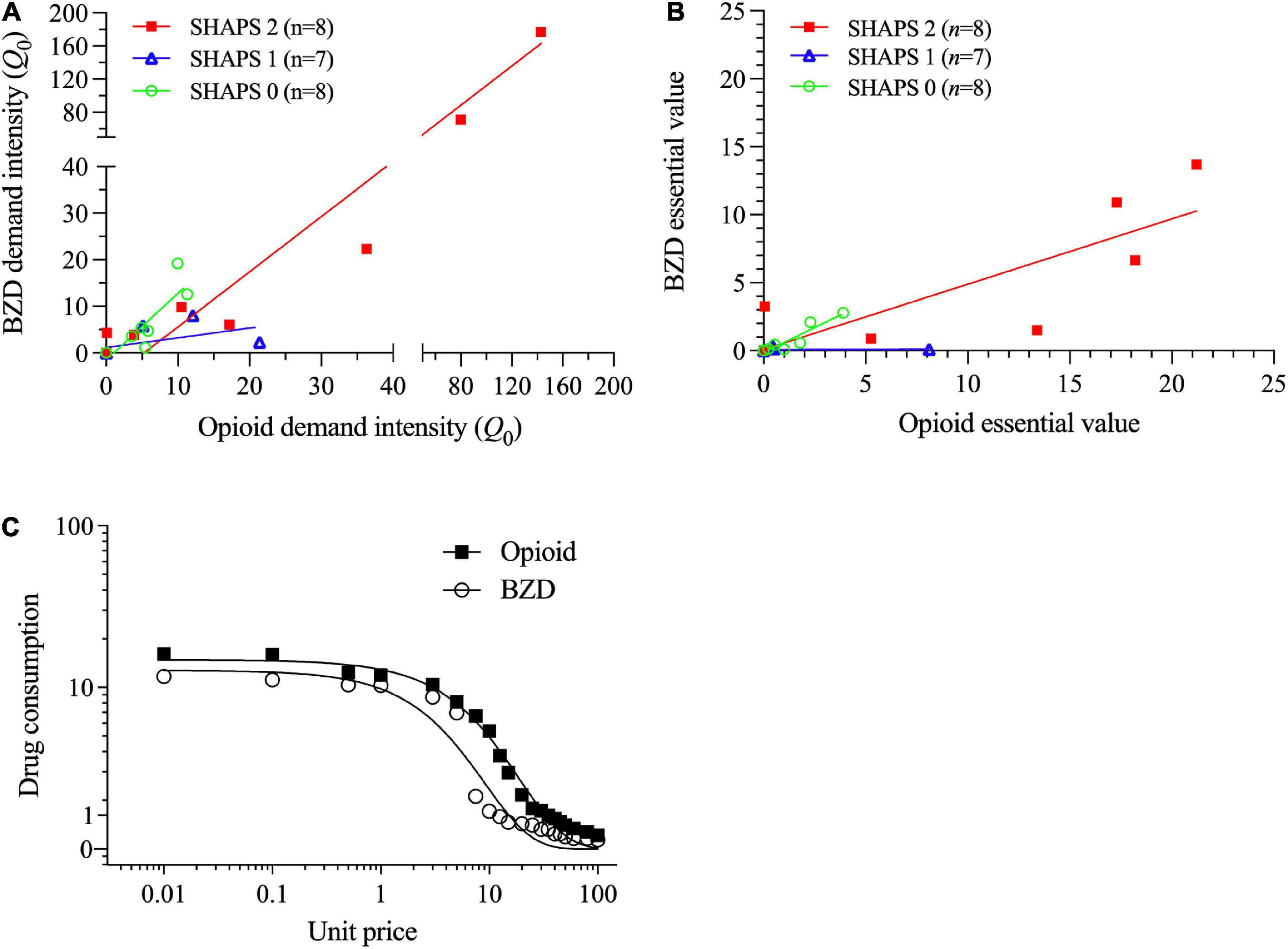
Figure 4. Within the subgroup of lifetime benzodiazepine (BZD) users, correlations of (A) opioid and benzodiazepine demand intensities (split axes enable better data visualization at low values) and (B) opioid and benzodiazepine essential values. Each of these two panels shows values separately for subgroups with 0, 1 or ≥ 2 Snaith-Hamilton Pleasure Scale (SHAPS) anhedonia scores. (C) In the primary SPSS analysis, opioid essential value (but not demand intensity) was significantly greater for the preferred-opioid than alprazolam (see Section “3.2. Aim 2: Differences within lifetime BZD-misusing subgroups”). In the secondary GraphPad Prism analysis, the preferred-opioid and alprazolam curves significantly differed (Q0 = 18.7 vs. 11.6; EV = 4.83 vs. 1.03), F(1,18) = 68.0, p < 0.01.
4. Discussion
This ongoing study of persons being treated for opioid use disorder, and with polysubstance misuse histories, is examining factors that modulate economic demand for a standard BZD that is frequently misused (alprazolam) and each participant’s preferred misused opioid. The primary novel finding from this analysis is that participants who report multiple symptoms of anhedonia–a deficit in the experience and anticipation of pleasure–manifest significantly increased economic demand for both alprazolam and opioid drugs.
The first aim of the study was to determine whether BZD misuse history is related to affective dysregulation, opioid economic demand, and other clinically relevant measures. Based on systematic self-report and psychiatric diagnosis of sedative use disorder (involving a BZD), more than half of the sample (37 of 59 participants) were classified as having misused BZDs during their lifetime and over half of those (20 of 37) misused BZDs during the past year, whereas the remaining participants denied lifetime BZD misuse (22 of 59; comparison group). Relative to never-misusers, BZD misusers (both lifetime and past-year subgroups) were: higher on trait anxiety and emotion regulation problems and more likely to meet criteria for current major depressive disorder (consistent with our hypothesis); more likely to present opioid-negative and methadone-negative urine samples; and younger in age. In general, lifetime and past-year BZD misusers did not significantly differ on any of these measures; the only observed differences were between BZD misusing subgroups and the never-misuser group.
Surprisingly, BZD misusers did not significantly differ from never-misusers on several symptom measures of affective dysregulation including anhedonia (SHAPS), depression (BDI-II), state anxiety (STAI), distress tolerance (DTS), perceived stress (PSS), nor self-efficacy to resist substance use (ADUSE). Also, BZD misusers and never-misusers did not differ on current anxiety disorder, PTSD or bipolar disorder diagnoses (although the latter was infrequent) that are commonly linked to problems of affective dysregulation, nor did the groups differ on current non-sedative substance use disorder diagnoses.
Interestingly, BZD misusers and never-misuser groups did not significantly differ in experimental opioid demand. To our knowledge, this is the first clinical study to examine opioid demand in relation to differences in BZD-misuse history. By comparison, Petry and Bickel (66) examined simulated demand for heroin or the BZD diazepam in persons with a heroin-use history; most reported histories of injection use and all reported polysubstance use. Although all participants were in outpatient treatment and most were maintained on buprenorphine, all were instructed to imagine drug purchases while not receiving medication treatment (whereas such an instruction was not given in the present study). Those authors found that demand for heroin modestly decreased (i.e., was inelastic or relatively insensitive) in response to increases in its experimental price. In a separate assessment, it was found that as heroin price increased, diazepam purchasing increased. Thus, diazepam functioned as an economic substitute for heroin; yet, heroin purchases were found to be independent of diazepam prices, indicating asymmetrical substitution. In a recent study of persons in outpatient opioid agonist treatment for opioid use disorder, Schwartz et al. (68) found at a baseline visit that intensity of demand for BZD pills, which was lower than for heroin or cocaine in their sample, predicted the proportion of BZD-positive urine samples over a 6-month follow-up interval; thus, demand metrics were found to have clinical predictive value, which has also been demonstrated for treatment of tobacco use disorder (105), alcohol use disorder (106), and cocaine use disorder (107). Notably, Schwartz et al. (68) observed that opioid demand intensity (but not essential value) was significantly greater than for BZD pills, whereas the present study found that essential value for opioid vs. alprazolam significantly differed, with a trend for demand intensity. It is possible that (a) differences in the participant samples from the two studies, and/or (b) assessment of demand using the participant’s preferred opioid and route in the present study, played a role in these slightly discrepant findings.
The second aim of the present study was to investigate whether measures of affective dysregulation would modulate BZD demand within the subgroup of past-year BZD users. Anhedonia, which was associated with significantly greater opioid demand, was also found to significantly increase BZD demand. Therefore, in this sample, elevated anhedonia was a common predictor of increased drug demand. Interestingly, current depression symptom levels (BDI-II) showed similar, but slightly weaker, effects than anhedonia on both opioid and BZD demand. This raises an important question of behavioral specificity. Anhedonia symptoms measured with the SHAPS represents a more narrow phenotype (positive hedonic deficit) than depression symptoms measured with the BDI-II. Although the Beck Depression Inventory has three items (#4, loss of pleasure; #12, loss of interest; #21, loss of interest in sex) that have been proposed to measure anhedonia (75) the majority of items focus on negative-affective symptoms and neurovegetative signs of affective disorder. Thus, anhedonia more precisely captures impairment of positive reinforcement. Interestingly, anhedonia but not depression was found to predict cocaine use in a clinical trial (108), in support of its distinct construct and predictive validity.
Anhedonia has been associated with impaired reinforcement learning (82, 109, 110). Thus, for persons with higher (vs. lower) anhedonia, repeated drug use and conditioning may strengthen drug demand to a greater degree so that it becomes more intense (at low prices, Q0) and resistant to price increases (inelastic, or higher essential value). This phenotype maps onto some proposed sub-domains of anhedonia, e.g., approach motivation, reward valuation, effort valuation/willingness to work, and habit formation (111, 112). Although anhedonia might generally increase drug demand (as we found for opioid and alprazolam), it is conceivable that anhedonia might also interact differently across misused drugs [but see (113) for interpretive complexities]. Notably, laboratory animal models of drug self-administration have found that GABAergic agents including BZDs and alcohol can produce anti-conflict effects, i.e., they disinhibit punished behaviors (114–117) and this could enhance the expression of risky behaviors (118). For people who have experienced adverse consequences of opioid and sedative polydrug use (119, 120), i.e., such use has been punished or suppressed, BZDs (and alcohol) may interfere with efforts to abstain. Further research might explore whether this BZD anti-conflict effect could be enhanced in persons with higher anhedonia and may also interact with the behavioral cost of the drug.
The neural substrate for anhedonia is hypothesized to involve disruptions to a cortical/subcortical neural circuit whereby elevated prefrontal cortical excitability leads to decreased striatal dopamine activation (79, 121–123). Interestingly, opioid withdrawal-related anhedonia in rats (increased intracranial electrical self-stimulation) was associated with reduced vulnerability to subsequent morphine self-administration (124). However, in samples of patients with opioid use disorder, anhedonia has been variously found to correlate with recent opioid use during medication treatment but not during long-term abstinence (125, 126) as well as drug-cue or natural reward cue-reactivity during opioid abstinence (127–129) but not in all studies (130); these mixed findings imply that elevated anhedonia may be a dissociable phenotype from opioid or other substance use/abstinence [cf. (131)].
In a preclinical study, rats withdrawn from BZD exposure exhibited reduced preferences for both a cage compartment that had been paired with a sexual odor cue and for a context previously paired with amphetamine–a pattern of attenuated reward-seeking behaviors suggestive of increased anhedonia (132). In humans, there are very few studies of BZDs and anhedonic symptoms. Use of BZDs among patients with major depressive disorder was found to be associated with increased anhedonia but not anxiety or depression symptom levels (perhaps because BZDs mitigated anxiety) and anhedonia was the strongest predictor of BZD use in that study (133). A recent clinical study of repeated ketamine infusions in 42 patients with treatment-resistant major depression found that ketamine (an NMDA receptor antagonist) significantly reduced anhedonia (SHAPS scores) after each infusion but only among the subgroup of patients who did not use BZDs (134). Although the present study was not designed to examine BZD withdrawal and anhedonia, we did not find any significant difference in anhedonia scores between BZD misusers and never-misusers, nor between participants whose urine samples tested BZD-positive vs. BZD-negative. Further research is needed to understand the relationship between BZD use/discontinuation and anhedonia.
Benzodiazepines modulate activity to varying degrees at GABAA receptor subtypes which differentially correlate with their reinforcing, sedative/hypnotic and myorelaxant properties (60, 63, 135, 136). In persons with opioid use disorder, BZDs might also (e.g., via GABA interneurons on mu-opioid receptors) indirectly modulate mu-opioid receptor function (137), potentially leading to altered sensitivity to drug reinforcement (138). In the present study, neither past-year BZD misuse nor BZD-positive urine samples were related to opioid demand; however, only 15% of the overall sample had BZD-positive samples so there is likely insufficient statistical power to detect an effect. Unfortunately, we lack systematic data on the precise temporal pattern of BZD and opioid use (e.g., simultaneous vs. concurrent), which could potentially influence these results.
We also note that several measures were not significantly related to BZD misuse group, anhedonia or depression, nor opioid or BZD demand. These include some demographic factors (e.g., notably, no sex differences), psychiatric comorbidities other than sedative use disorder, and several measures of negative affective dysregulation. Absence of effects of insomnia and negative affective disturbance–which are often related (44, 139)–was surprising. However, we did find that younger age was significantly related to several measures of affective dysregulation (SHAPS, BDI-II, STAI, ADUSE, DTS, DERS, PASAT), both in the overall sample and within the past-year BZD-use group (see Table 3), but age was not significantly related to BZD demand metrics.
This study has several limitations. First, there is a relatively small sample size, although our sample is not smaller than others’ comparable work (66, 68). Notably, our planned enrollment is expected to be up 120 participants, so we will have ample power to examine these and other effects in greater detail. Second, we conducted sensitivity analyses that excluded individuals with zero participation in the purchase task (leading to reduction in group size); these analyses suggest that alprazolam demand metrics should be cautiously interpreted, whereas censoring of participants with zero participation did not significantly alter opioid demand metrics. Third, we are recruiting individuals from various treatment settings/modalities to increase the heterogeneity and population representativeness of the sample with regard to polysubstance use and types of interventions; although this introduces variance that may complicate interpretation of the findings, we believe it can improve the generalizability of findings to treatment settings and prompt new hypotheses for investigation. Fourth, it is presently not feasible to collect reliable data on medication treatment doses, which could affect opioid demand and perhaps BZD demand. Notably, it has been shown in laboratory animal models that acute pretreatment with morphine, buprenorphine or naltrexone can increase fentanyl demand elasticity, i.e., decrease essential value (140). Fifth, unlike Petry and Bickel (66), we did not examine cross-price elasticity between the preferred-opioid and alprazolam in this study; although we designed such a manipulation, this was ultimately excluded due to the length of the overall assessment battery (several additional measures in this battery are not reported here). Finally, consistent with the work by Schwartz et al. (68), we are interested in whether these demand measures can predict longer-term outcomes. In the present study, we are collecting 3-month follow-up measures; however, at this time, these data are too sparse for meaningful analysis. However, it should be noted that purchasing “participation” (i.e., making non-zero drug choices at any price) in an in-treatment population may indicate the presence of a relapse risk. Thus, in future research, it could be useful to include participation as well as demand intensity and essential value metrics when reporting results with samples of patients.
In conclusion, this study identifies increased anhedonia as a shared factor for greater economic demand of opioid and BZD drugs in persons with histories of polysubstance use who are being treated for opioid use disorder. Anhedonia, which has commonly received attention in psychiatric disorders especially major depressive disorder [e.g., (75, 77, 141)], has a biological basis partly independent of depressive symptoms (142–144). Anhedonia has been observed during acute and protracted drug abstinence and may be related to drug craving (78). Thus, anhedonia may play an important predictive role in substance use disorder treatment outcome. Based on our findings that anhedonia can modulate drug demand, along with recent findings that experimental demand can predict treatment outcome in substance use disorders (68), we believe that it could be useful to routinely include assessments of anhedonia and hypothetical drug demand in clinical settings to monitor the progress and recovery of persons with these disorders.
Future directions are to understand the multidimensional nature of affective dysregulation in this population, develop improved biomarkers/phenotypes to predict clinical outcomes and, from this improved understanding, develop behavioral, medication and neuromodulation interventions to reduce anhedonia and improve treatment efficacy (111, 141, 145–147).
Data availability statement
The original contributions presented in this study are included in the article/supplementary material, further inquiries can be directed to the corresponding author.
Ethics statement
The studies involving human participants were reviewed and approved by Human Investigation Committee, Wayne State University. The patients/participants provided their written informed consent to participate in this study.
Author contributions
MG acquired funding, designed the study, oversees data collection, conducted the analyses, and drafted the manuscript. TM, LL, and TR contributed to study design and assessments and edited the manuscript. TM conducted psychiatric interviews and collected data. LL supervised psychiatric assessments. All authors taken responsibility for the content of the manuscript.
Funding
This study was supported by NIH R21/R33 DA044946 (MG), Gertrude Levin Endowed Chair in Addiction and Pain Biology (MG), NIH F30 DA052118 (TM), Michigan Department of Health and Human Services (Helene Lycaki/Joe Young, Sr. Funds), and Detroit Wayne Integrated Health Network.
Acknowledgments
The authors thank Alina Woodford, Hayley Harrison, Lauren Cislo, Klevis Karavidha, Alanna Foulon, Jasmine Hollins, Heidi Aguas, Alyson Renock, and Nareen Sadik for their assistance with participant recruitment, data collection and management; and Ciara Cannoy, Jennifer Ellis, Shaylin Excel, Marina Fodor, Krithika Prakash, Cara Struble, and Halle Thomas for conducting psychiatric interviews.
Conflict of interest
The authors declare that the research was conducted in the absence of any commercial or financial relationships that could be construed as a potential conflict of interest.
Publisher’s note
All claims expressed in this article are solely those of the authors and do not necessarily represent those of their affiliated organizations, or those of the publisher, the editors and the reviewers. Any product that may be evaluated in this article, or claim that may be made by its manufacturer, is not guaranteed or endorsed by the publisher.
Footnotes
References
1. Centers for Disease Control and Prevention,. Web-based Injury Statistics Query and Reporting System (WISQARS). (2014). Available online at: http://www.cdc.gov/injury/wisqars/fatal.html (accessed November 1, 2022).
2. Centers for Disease Control and Prevention. National Vital Statistics System Mortality Data. (2015). Available online at: http://www.cdc.gov/nchs/deaths.htm (accessed November 1, 2022).
3. Cicero TJ, Ellis MS, Kasper ZA. Polysubstance use: a broader understanding of substance use during the opioid crisis. Am J Public Health. (2020) 110:244–50. doi: 10.2105/AJPH.2019.305412
4. Crummy EA, O’Neal TJ, Baskin BM, Ferguson SM. One is not enough: understanding and modeling polysubstance use. Front Neurosci. (2020) 14:569. doi: 10.3389/fnins.2020.00569
5. Jones CM, Paulozzi LJ, Mack KA. Alcohol involvement in opioid pain reliever and benzodiazepine drug abuse-related emergency department visits and drug-related deaths – United States, 2010. MMWR Morb Mortal Wkly Rep. (2014) 63:881–5.
6. Yarborough BJH, Stumbo SP, Janoff SL, Yarborough MT, McCarty D, Chilcoat HD, et al. Understanding opioid overdose characteristics involving prescription and illicit opioids: a mixed methods analysis. Drug Alcohol Depend. (2016) 167:49–56. doi: 10.1016/j.drugalcdep.2016.07.024
7. Dowell D, Haegerich TM, Chou R. CDC guideline for prescribing opioids for chronic pain–United States, 2016. JAMA. (2016) 315:1624–45.
8. McAuley A, Matheson C, Robertson JR. From the clinic to the street: the changing role of benzodiazepines in the Scottish overdose epidemic. Int J Drug Policy. (2022) 100:103512. doi: 10.1016/j.drugpo.2021.103512
9. Jones JD, Mogali S, Comer SD. Polydrug abuse: a review of opioid and benzodiazepine combination use. Drug Alcohol Depend. (2012) 125:8–18.
10. Dobscha SK. Prescription opioids and benzodiazepines: moving beyond “just say no”. Pain Med. (2013) 14:1447–9. doi: 10.1111/pme.12237
11. Kim HS, McCarthy DM, Courtney DM, Lank PM, Lambert BL. Benzodiazepine-opioid co-prescribing in a national probability sample of ED encounters. Am J Emerg Med. (2017) 35:458–64. doi: 10.1016/j.ajem.2016.11.054
12. Larochelle MR, Zhang F, Ross-Degnan D, Wharam JF. Trends in opioid prescribing and co-prescribing of sedative hypnotics for acute and chronic musculoskeletal pain: 2001-2010. Pharmacoepid Drug Saf. (2015) 24:885–92. doi: 10.1002/pds.3776
13. Sullivan MD. What are we treating with opioid and sedative-hypnotic combination therapy? Pharmacoepidem Drug Saf. (2015) 24:893–5.
14. Bernardy NC, Lund BC, Alexander B, Friedman MJ. Increased polysedative use in veterans with posttraumatic stress disorder. Pain Med. (2014) 15:1083–90.
15. Boggis J, Feder K. Trends in and correlates of tranquilizer misuse among adults who misuse opioids in the United States, 2002-2014. Drug Alcohol Depend. (2019) 198:158–61. doi: 10.1016/j.drugalcdep.2019.01.014
16. Lavie E, Fatséas M, Denis C, Auriacombe M. Benzodiazepine use among opiate-dependent subjects in buprenorphine maintenance treatment: correlates of use, abuse and dependence. Drug Alcohol Depend. (2009) 99:338–44. doi: 10.1016/j.drugalcdep.2008.07.017
17. Calcaterra S, Glanz J, Binswanger IA. National trends in pharmaceutical opioid related overdose deaths compared to other substance related overdose deaths: 1999-2009. Drug Alcohol Depend. (2013) 131:263–70. doi: 10.1016/j.drugalcdep.2012.11.018
18. Chen LH, Hedegaard H, Warner M. Drug-poisoning deaths involving opioid analgesics: United States, 1999-2011. NCHS Data Brief. (2014) 166:1–8.
19. Garg RK, Fulton-Kehoe D, Franklin GM. Patterns of opioid use and risk of opioid overdose death among medicaid patients. Med Care. (2017) 55:661–8.
20. Gladden RM, O’Donnell J, Mattson CL, Seth P. Changes in opioid-involved overdose deaths by opioid type and presence of benzodiazepines, cocaine, and methamphetamine – 25 states, July-December 2017 to January-June 2018. Morbid Mortal Weekly Rep. (2019) 68:737–44. doi: 10.15585/mmwr.mm6834a2
21. Jann M, Kennedy WK, Lopez G. Benzodiazepines: a major component in unintentional prescription drug overdoses with opioid analgesics. J Pharm Pract. (2014) 27:5–16. doi: 10.1177/0897190013515001
22. Macleod J, Steer C, Tilling K, Cornish R, Marsden J, Millar T, et al. Prescription of benzodiazepines, z-drugs, and gabapentinoids and mortality risk in people receiving opioid agonist treatment: observational study based on the UK Clinical Practice Research Datalink and Office for National Statistics death records. PLoS Med. (2019) 16:e1002965. doi: 10.1371/journal.pmed.1002965
23. Nielsen S, Dietze P, Lee N, Dunlop A, Taylor D. Concurrent buprenorphine and benzodiazepines use and self-reported opioid toxicity in opioid substitution treatment. Addiction. (2007) 102:616–22. doi: 10.1111/j.1360-0443.2006.01731.x
24. Park TW, Saitz R, Ganoczy D, Ilgen MA, Bohnert ASB. Benzodiazepine prescribing patterns and deaths from drug overdose among US veterans receiving opioid analgesics: case-cohort study. BMJ. (2015) 350:h2698. doi: 10.1136/bmj.h2698
25. Paulozzi LJ, Kilbourne EM, Shah NG, Nolte KB, Desai HA, Landen MG, et al. A history of being prescribed controlled substances and risk of drug overdose death. Pain Med. (2012) 13:87–95.
26. Votaw VR, Geyer R, Rieselbach MM, McHugh RK. The epidemiology of benzodiazepine misuse: a systematic review. Drug Alcohol Depend. (2019) 200:95–114.
27. Fatséas M, Lavie E, Denis C, Auriacombe M. Self-perceived motivation for benzodiazepine use and behavior related to benzodiazepine use among opiate-dependent patients. J Subst Abuse Treat. (2009) 37:407–11. doi: 10.1016/j.jsat.2009.03.006
28. Mateu-Gelabert P, Jessell L, Goodbody E, Kim D, Gile K, Teubl J, et al. High enhancer, downer, withdrawal helper: multifunctional nonmedical benzodiazepine use among young adult opioid users in New York City. Int J Drug Policy. (2017) 46:17–27. doi: 10.1016/j.drugpo.2017.05.016
29. Navaratnam V, Foong K. Adjunctive drug use among opiate addicts. Curr Med Res Opin. (1990) 11:611–9.
30. Stein MD, Kanabar M, Anderson BJ, Lembke A, Bailey GL. Reasons for benzodiazepine use among persons seeking opioid detoxification. J Subst Abuse Treat. (2016) 68:57–61.
31. Gudin JA, Mogali S, Jones JD, Comer SD. Risks, management, and monitoring of combination opioid, benzodiazepines, and/or alcohol use. Postgrad Med. (2013) 125:115–30.
32. Moses TEH, Greenwald MK. History of regular nonmedical sedative and/or alcohol use differentiates substance-use patterns and consequences among chronic heroin users. Addict Behav. (2019) 97:14–9.
33. Ogbu UC, Lotfipour S, Chakravarthy B. Polysubstance abuse: alcohol, opioids and benzodiazepines require coordinated engagement by society, patients, and physicians. West J Emerg Med. (2015) 16:76–9. doi: 10.5811/westjem.2014.11.24720
34. Evans SM, Griffiths RR, de Wit H. Preference for diazepam, but not buspirone, in moderate drinkers. Psychopharmacology (Berl). (1996) 123:154–63.
35. McHugh RK, Geyer RB, Chase AR, Griffin ML, Bogunovic O, Weiss RD. Sex differences in benzodiazepine misuse among adults with substance use disorders. Addict Behav. (2021) 112:106608.
36. Cook B, Creedon T, Wang Y, Lu C, Carson N, Jules P, et al. Examining racial/ethnic differences in patterns of benzodiazepine prescription and misuse. Drug Alcohol Depend. (2018) 187:29–34.
37. Tucker D, Hayashi K, Milloy- M, Nolan S, Dong H, Kerr T, et al. Risk factors associated with benzodiazepine use among people who inject drugs in an urban Canadian setting. Addict Behav. (2016) 52:103–7. doi: 10.1016/j.addbeh.2015.10.002
38. Fuller CM, Borrell LM, Latkin CA, Galea S, Ompad DC, Strathdee SA, et al. Effects of race, neighborhood, and social network on age at initiation of injection drug use. Am J Public Health. (2005) 95:689–95. doi: 10.2105/AJPH.2003.02178
39. Jones CM, Christensen A, Gladden RM. Increases in prescription opioid injection abuse among treatment admissions in the United States, 200-2013. Drug Alcohol Depend. (2017) 176:89–95. doi: 10.1016/j.drugalcdep.2017.03.011
40. Martins SS, Santaella-Tenorio J, Marshall BDL, Maldonado A, Cerdá M. Racial/ethnic differences in trends in heroin use and heroin-related risk behaviors among nonmedical prescription opioid users. Drug Alcohol Depend. (2015) 151:278–83. doi: 10.1016/j.drugalcdep.2015.03.020
41. Ellis JD, Pasman E, Brown S, Lister JJ, Agius E, Resko SM. An examination of correlates of simultaneous opioid and benzodiazepine use among patients in medication treatment for opioid use disorder in a small midwestern community. J Addict Dis. (2022) 40:542–51. doi: 10.1080/10550887.2022.2042152
42. Hassan AN, Le Foll B. Polydrug use disorder in individuals with opioid use disorder. Drug Alcohol Depend. (2019) 198:28–33.
43. McHugh RK, Votaw VR, Bogunovic O, Karakula SL, Griffin ML, Weiss RD. Anxiety sensitivity and nonmedical benzodiazepine use among adults with opioid use disorder. Addict Behav. (2017) 65:283–8.
44. Greenwald MK, Moses TEH, Roehrs TA. At the intersection of sleep deficiency and opioid use: mechanisms and therapeutic opportunities. Transl Res. (2021) 234:58–73. doi: 10.1016/j.trsl.2021.03.006
45. Oliveira C, Filipe R, Meira J, Sampaio L, Teixeira L, Rodrigues J, et al. Benzodiazepine use in opioid maintenance treatment programme: risks and clinical outcomes. Acta Med Port. (2021) 34:209–16.
46. Markou A, Kosten TR, Koob GF. Neurobiological similarities in depression and drug dependence: a self-medication hypothesis. Neuropsychopharmacology. (1998) 18:135–74. doi: 10.1016/S0893-133X(97)00113-9
48. Lijffijt M, Hu K, Swann A. Stress modulates illness-course of substance use disorders: a translational review. Front Psychiatry. (2014) 5:83. doi: 10.3389/fpsyt.2014.00083
49. Nöel X, Brevers D, Bechara A. A triadic neurocognitive approach to addiction for clinical interventions. Front Psychiatry. (2013) 4:179. doi: 10.3389/fpsyt.2013.00179
50. Sinha R. Chronic stress, drug use, and vulnerability to addiction. Ann N Y Acad Sci. (2008) 1141:105–30.
51. Weiss F, Ciccocioppo R, Parsons LH, Katner S, Liu X, Zorrilla EP, et al. Compulsive drug-seeking behavior and relapse: neuroadaptation, stress, and conditioning factors. Ann N Y Acad Sci. (2001) 937:1–26.
52. Koob GF. Addiction is a reward deficit and stress surfeit disorder. Front Psychiatry. (2013) 4:72. doi: 10.3389/fpsyt.2013.00072
53. Greenwald MK. Behavioral economic analysis of drug preference using Multiple choice procedure data. Drug Alcohol Depend. (2008) 93:103–10.
55. Schwartz LP, Toegel F, Devine JK, Holtyn AF, Roma P, Hursh SR. Latent structure of behavioral economic heroin and cocaine demand curves. Exp Clin Psychopharmacol. (2022). doi: 10.1037/pha0000594 [Epub ahead of print].
56. Ait-Daoud N, Hamby AS, Sharma S, Blevins D. A review of alprazolam use, misuse, and withdrawal. J Addict Med. (2018) 12:4–10.
57. Corkery JM, Guirguis A, Chiappini S, Martinotti G, Schifano F. Alprazolam-related deaths in Scotland, 2004-2020. J Psychopharmacol. (2021) 36:1020–35. doi: 10.1177/02698811221104065
58. Sellers EM, Ciraulo DA, DuPont RL, Griffiths RR, Kosten TR, Romach MK, et al. Alprazolam and benzodiazepine dependence. J Clin Psychiatry. (1993) 54(Suppl.):64–75.
59. Verster JC, Volkerts ER. Clinical pharmacology, clinical efficacy, and behavioral toxicity of alprazolam: a review of the literature. CNS Drug Rev. (2004) 10:45–76. doi: 10.1111/j.1527-3458.2004.tb00003.x
60. Licata SC, Rowlett JK. Abuse and dependence liability of benzodiazepine-type drugs: GABAA receptor modulation and beyond. Pharmacol Biochem Behav. (2008) 90:74–89. doi: 10.1016/j.pbb.2008.01.001
61. Licata SC, Rowlett JK. Self-administration of bretazenil under progressive-ratio schedules: behavioral economic analysis of the role intrinsic efficacy plays in the reinforcing effects of benzodiazepines. Drug Alcohol Depend. (2011) 113:157–64. doi: 10.1016/j.drugalcdep.2010.07.019
62. Rowlett JK, Lelas S. Comparison of zolpidem and midazolam self-administration under progressive-ratio schedules: consumer demand and labor supply analyses. Exp Clin Psychopharmacol. (2007) 15:328–37. doi: 10.1037/1064-1297.15.4.328
63. Rowlett JK, Platt DM, Lelas S, Atack JR, Dawson GR. Different GABAA receptor subtypes mediate the anxiolytic, abuse-related, and motor effects of benzodiazepine-like drugs in primates. Proc Natl Acad Sci U.S.A. (2005) 103:915–20. doi: 10.1073/pnas.0405621102
64. Shinday NM, Sawyer EK, Fischer BD, Platt DM, Licata SC, Atack JR, et al. Reinforcing effects of compounds lacking intrinsic efficacy at α1 subunit-containing GABAA receptor subtypes in midazolam- but not cocaine-experienced rhesus monkeys. Neuropsychopharmacology. (2013) 38:1006–14. doi: 10.1038/npp.2012.265
65. Berro LF, Zamarripa A, Rowlett JK. Self-administration of fentanyl-alprazolam combinations by rhesus monkeys responding under a progressive-ratio schedule. J Pharmacol Exp Ther. (2022). doi: 10.1124/jpet.122.001191 [Epub ahead of print].
66. Petry NM, Bickel WK. Polydrug abuse in heroin addicts: a behavioral economic analysis. Addiction. (1998) 93:321–35.
67. Petry NM. A behavioral economic analysis of polysubstance abuse in alcoholics: asymmetrical substitution of alcohol and cocaine. Drug Alcohol Depend. (2001) 62:31–9. doi: 10.1016/j.drugalcdep.2004.04.006
68. Schwartz LP, Blank L, Hursh SR. Behavioral economic demand in opioid treatment: predictive validity of hypothetical purchase tasks for heroin, cocaine, and benzodiazepines. Drug Alcohol Depend. (2021) 221:108562. doi: 10.1016/j.drugalcdep.2021.108562
69. Amlung MT, MacKillop J. Understanding the effects of stress and alcohol cues on motivation for alcohol via behavioral economics. Alcohol Clin Exp Res. (2014) 38:1780–9. doi: 10.1111/acer.12423
70. Bruner NR, Johnson MW. Demand curves for hypothetical cocaine in cocaine-dependent individuals. Psychopharmacology. (2014) 231:889–97. doi: 10.1007/s00213-013-3312-5
71. Greenwald MK, Sarvepalli S, Cohn JA, Lundahl LH. Demand curve analysis of marijuana use among persons living with HIV. Drug Alcohol Depend. (2021) 220:108524. doi: 10.1016/j.drugalcdep.2021.108524
72. Zachary R. Shipley Institute of Living Scale: Revised Manual. Los Angeles, CA: Western Psychological Services (1991).
73. American Psychiatric Association.Diagnostic and Statistical Manual of Mental Disorders. 5th ed. Washington, DC: American Psychiatric Association (2015).
74. First MB, Williams JBW, Karg RS, Spitzer RL. Structured Clinical Interview for DSM–5, Research Version. Arlington, VA: American Psychiatric Association (2015).
75. Joiner TE, Brown JS, Metalsky GI. A test of the tripartite model’s prediction of anhedonia’s specificity to depression: patients with major depression versus patients with schizophrenia. Psychiatry Res. (2003) 119:243–50. doi: 10.1016/s0165-1781(03)00131-8
76. Pizzagalli DA, Jahn AL, O’Shea JP. Toward an objective characterization of an anhedonic phenotype: a signal-detection approach. Biol Psychiatry. (2005) 57:319–27. doi: 10.1016/j.biopsych.2004.11.026
77. Heinz A, Schmidt LG, Reischies FM. Anhedonia in schizophrenic, depressed, or alcohol-dependent patients – neurobiological correlates. Pharmacopsychiatry. (1994) 27(Suppl. 1):7–10.
78. Janiri L, Martinotti G, Dario T, Reina D, Paparello F, Pozzi G, et al. Anhedonia and substance-related symptoms in detoxified substance-dependent patients: a correlation study. Neuropsychobiology. (2005) 52:37–44. doi: 10.1159/000086176
79. Schmidt K, Nolte-Zenker B, Patzer J, Bauer M, Schmidt LG, Heinz A. Psychopathological correlates of reduced dopamine receptor sensitivity in depression, schizophrenia, and opiate and alcohol dependence. Pharmacopsychiatry. (2001) 34:66–72. doi: 10.1055/s-2001-15184
80. Willner P, Hale AS, Argyropoulos S. Dopaminergic mechanism of antidepressant action in depressed patients. J Affect Disord. (2005) 86:37–45.
81. Franken IHA, Rassin E, Muris P. The assessment of anhedonia in clinical and non-clinical populations: further validation of the Snaith-Hamilton Pleasure Scale (SHAPS). J Affect Disord. (2007) 99:83–9.
82. Huys QJM, Pizzagalli DA, Bogdan R, Dayan P. Mapping anhedonia onto reinforcement learning: a behavioural meta-analysis. Biol Mood Anxiety Disord. (2013) 3:12. doi: 10.1186/2045-5380-3-12
83. Case JAC, Sullivan-Toole H, Mattoni M, Jacobucci R, Forbes EE. Evaluating the item-level factor structure of anhedonia. J Affect Disord. (2022) 299:215–22.
84. Beck AT, Steer RA, Ball R, Ranieri W. Comparison of beck depression inventories –IA and –II in psychiatric outpatients. J Pers Assess. (1996) 67:588–97. doi: 10.1207/s15327752jpa6703_13
85. Grothe KB, Dutton GR, Jones GN, Bodenlos J, Ancona M, Brantley PJ. Validation of the Beck Depression Inventory-II in a low-income African American sample of medical outpatients. Psychol Assess. (2005) 17:110–4. doi: 10.1037/1040-3590.17.1.110
86. Buckley TC, Parker JD, Heggie J. A psychometric evaluation of the BDI-II in treatment-seeking substance abusers. J Subst Abuse Treat. (2001) 20:197–204. doi: 10.1016/s0740-5472(00)00169-0
87. Wang Y-P, Gorenstein C. The beck depression inventory: uses and applications. In: Martin CR, Hunter, L-A, Patel VB, Preedy VR, Rajendran R (Eds). The Neuroscience of Depression: Features, Diagnosis and Treatment. New York, NY: Academic Press (2021). p. 165–74. doi: 10.1016/B978-0-12-817933-8.00020-7
88. Spielberger CD, Gorsuch RL, Lushene RE. State Trait Anxiety Inventory manual. Palo Alto, CA: Consulting Psychologist Press (1970). p. 23–49.
89. Cohen S, Kamarck T, Mermelstein R. A global measure of perceived stress. J Health Soc Behav. (1983) 24:385–96.
90. Gratz KL, Roemer L. Multidimensional assessment of emotion regulation and dysregulation: development, factor structure, and initial validation of the difficulties in emotion regulation scale. J Psychopathol Behav Assess. (2004) 26:41–54.
91. Brown TG, Seraganian P, Tremblay J, Annis H. Process and outcome changes with relapse prevention versus 12-step aftercare programs for substance abusers. Addiction. (2002) 97:677–89. doi: 10.1046/j.1360-0443.2002.00101.x
92. Brandon TH, Herzog TA, Juliano LM, Irvin JE, Lazev AB, Simmons NV. Pretreatment task persistence predicts smoking cessation outcome. J Abn Psychol. (2003) 111:180–5.
93. Brown RA, Lejuez CW, Kahler CW, Strong DR. Distress tolerance and duration of past smoking cessation attempts. J Abnorm Psychol. (2002) 111:180–5.
94. Daughters SB, Lejuez CW, Kahler CW, Strong DR, Brown RA. Psychological distress tolerance and duration of most recent abstinence attempt among residential treatment-seeking substance abusers. Psychol Addict Behav. (2005) 19:208–11. doi: 10.1037/0893-164X.19.2.208
95. Simons JS, Gaher RM. The distress tolerance scale: development and validation of a self-report measure. Motiv Emot. (2005) 29:83–102.
96. Lejuez CW, Kahler CW, Brown RA. A modified computer version of the Paced Auditory Serial Learning Task (PASAT) as a laboratory-based stressor. Behav Therap. (2003) 26:290–3. doi: 10.1016/j.jbtep.2005.06.002
97. Williams JMG, Mathews A, MacLeod C. The emotional stroop task and psychopathology. Psychol Bull. (1996) 120:3–24.
98. Bastien CH, Vallieres A, Morin CM. Validation of the insomnia severity index as an outcome measure for insomnia research. Sleep Med. (2001) 2:297–307.
100. Johns MW. Sleepiness in different situations measured by the Epworth sleepiness scale. Sleep. (1994) 17:703–10.
101. Basner M, Dinges DF. An adaptive-duration version of the PVT accurately tracks changes in psychomotor vigilance induced by sleep restriction. Sleep. (2012) 35:193–202. doi: 10.5665/sleep.1620
102. Dinges DI, Powell JW. Microcomputer analysis of performance on a portable, simple visual RT task sustained operations. Behav Res Methods Instr Comp. (1985) 17:652–5.
103. Gilroy SP, Kaplan BA, Schwartz LP, Reed DD, Hursh SR. A zero-bounded model of operant demand. J Exp Anal Behav. (2021) 115:729–46. doi: 10.1002/jeab.679
104. Heckman BW, Cummings MK, Nahas GJ, Willemsen MC, O’Connor RJ, Borland R, et al. Behavioral economic purchase tasks to estimate demand for novel nicotine/tobacco products and prospectively predict future use: evidence from the Netherlands. Nicotine Tob Res. (2019) 21:784–91. doi: 10.1093/ntr/nty042
105. Secades-Villa R, Pericot-Valverde I, Weidberg S. Relative reinforcing efficacy of cigarettes as a predictor of smoking abstinence among treatment-seeking smokers. Psychopharmacology (Berl.). (2016) 233:3103–12. doi: 10.1007/s00213-016-4350-6
106. Murphy JG, Dennhardt AA, Yurasek AM, Skidmore JR, Martens MP, MacKillop J, et al. Behavioral economic predictors of brief alcohol intervention outcomes. J Consult Clin Psychol. (2015) 83:1033–43.
107. Yoon JH, Suchting R, McKay SA, San Miguel GG, Vujanovic AA, Sotts AL, et al. Baseline cocaine demand predicts contingency management treatment outcomes for cocaine-use disorder. Psychol Addict Behav. (2020) 34:164–74. doi: 10.1037/adb0000475
108. Crits-Cristoph P, Wadden S, Gaines A, Rieger A, Gallop R, McKay JR, et al. Symptoms of anhedonia, not depression, predict the outcome of treatment of cocaine dependence. J Subst Abuse Treat. (2018) 92:46–50.
109. Chen C, Takahashi T, Nakagawa S, Inoue T, Kusumi I. Reinforcement learning in depression: a review of computational research. Neurosci Biobehav Rev. (2015) 55:247–67.
110. Rothkirch M, Tonn J, Köhler S, Sterzer P. Neural mechanisms of reinforcement learning in unmedicated patients with major depressive disorder. Brain. (2017) 140:1147–57. doi: 10.1093/brain/awx025
111. Craske MG, Meuret AE, Ritz T, Treanor M, Dour HJ. Treatment for anhedonia: a neuroscience driven approach. Depress Anxiety. (2016) 33:927–38.
112. Zald DH, Treadway MT. Reward processing, neuroeconomics, and psychopathology. Annu Rev Clin Psychol. (2017) 13:471–95.
113. Salamone JD, Cousins MS, Snyder BJ. Behavioral functions of nucleus accumbens dopamine: empirical and conceptual problems with the anhedonia hypothesis. Neurosci Biobehav Rev. (1997) 21:341–59. doi: 10.1016/s0149-7634(96)00017-6
114. Aguilar de Arcos F, Verdejo-García A, Ceverino A, Montanez-Pareja M, López-Juárez E, Sánchez-Barrerra M, et al. Dysregulation of emotional response in current and abstinent heroin users: negative heightening and positive blunting. Psychopharmacology. (2008) 198:159–66. doi: 10.1007/s00213-008-1110-2
115. Liljequist S, Engel JA. The effects of GABA and benzodiazepine receptor antagonists on the anti-conflict actions of diazepam and ethanol. Pharmacol Biochem Behav. (1984) 21:521–5. doi: 10.1016/s0091-3057(84)80033-7
116. Panlilio LV, Thorndike EB, Schindler CW. Lorazepam reinstates punishment-suppressed remifentanil self-administration in rats. Psychopharmacology. (2005) 179:374–82. doi: 10.1007/s00213-004-2040-2
117. Rowlett JK, Lelas S, Tornatzky W, Licata SC. Anti-conflict effects of benzodiazepines in rhesus monkeys: relationship with therapeutic doses in humans and role of GABAA receptors. Psychopharmacology. (2006) 184:201–11.
118. Lane SD, Tcheremissine OV, Lieving LM, Nouvion S, Cherek DR. Acute effects of alprazolam on risky decision making in humans. Psychopharmacology. (2005) 181:364–73.
119. Moses TEH, Lundahl LH, Greenwald MK. Factors associated with sedative use and misuse among regular heroin users. Drug Alcohol Depend. (2018) 185:10–6. doi: 10.1016/j.drugalcdep.2017.11.035
120. Moses TEH, Woodcock EA, Lister JJ, Lundahl LH, Greenwald MK. Developing a scale of domains of negative consequences of chronic heroin use. Addict Behav. (2019) 77:260–6. doi: 10.1016/j.addbeh.2017.07.027
121. Ferenczi EA, Zalocusky KA, Liston C, Grosenick L, Warden MR, Amatya D, et al. Prefrontal cortical regulation of brainwide circuit dynamics and reward-related behavior. Science. (2016) 351:aac9698. doi: 10.1126/science.aac9698
122. Nusslock R, Alloy RB. Reward processing and mood-related symptoms: an RDoC and translational neuroscience perspective. J Affect Disord. (2017) 216:3–16. doi: 10.1016/j.jad.2017.02.001
123. Russo SJ, Nestler EJ. The brain reward circuitry in mood disorders. Nat Rev Neurosci. (2013) 14:609–25.
124. Swain Y, Muelken P, Skansberg A, Lanzdorf D, Haave Z, LeSage MG, et al. Higher anhedonia during withdrawal from initial opioid exposure is protective against subsequent opioid self-administration in rats. Psychopharmacology. (2020) 237:2279–91. doi: 10.1007/s00213-020-05532-w
125. Garfield JBB, Cotton SM, Allen NB, Cheetham A, Kras M, Yücel M, et al. Evidence that anhedonia is a symptom of opioid dependence associated with recent use. Drug Alcohol Depend. (2017) 177:29–38.
126. Lubman DI, Garfield JBB, Gwini SM, Cheetham A, Cotton SM, Yücel M, et al. Dynamic associations between opioid use and anhedonia: a longitudinal study in opioid dependence. J Psychopharmacol. (2018) 32:957–64. doi: 10.1177/0269881118791741
127. Huhn AS, Meyer RE, Harris JD, Ayaz H, Deneke E, Stankowski DM, et al. Evidence of anhedonia and differential reward processing in prefrontal cortex among post-withdrawal patients with prescription opiate dependence. Brain Res Bull. (2016) 123:102–9. doi: 10.1016/j.brainresbull.2015.12.004
128. Petrie DJ, Knapp KS, Freet CS, Deneke E, Brick TR, Cleveland HH, et al. Prefrontal cortical response to natural rewards and self-reported anhedonia are associated with greater craving among recently withdrawn patients in residential treatment for opioid use disorder. Brain Res Bull. (2022) 190:32–41. doi: 10.1016/j.brainresbull.2022.09.012
129. Zijlstra F, Veltman DJ, Booij J, van den Brink W, Franken IHA. Neurobiological substrates of cue-elicited craving and anhedonia in recently abstinent opioid-dependent males. Drug Alcohol Depend. (2009) 99:183–92. doi: 10.1016/j.drugalcdep.2008.07.012
130. Stull SW, Bertz JW, Panlilio LV, Kowalczyk WJ, Phillips KA, Moran LM, et al. I feel good? Anhedonia might not mean “without pleasure” for people treated for opioid use disorder. J Abnorm Psychol. (2021) 130:537–49.
131. Pozzi G, Martinotti G, Reina D, Dario T, Frustaci A, Janiri L, et al. The assessment of post-detoxification anhedonia: influence of clinical and psychosocial variables. Subst Use Misuse. (2008) 43:22–32. doi: 10.1080/00952990701202954
132. Lacerra C, Martijena ID, Bustos SG, Molina VA. Benzodiazepine withdrawal facilitates the subsequent onset of escape failures and anhedonia: influence of different antidepressant drugs. Brain Res. (1999) 819:40–7. doi: 10.1016/s0006-8993(98)01341-9
133. Rizvi SJ, Sproule BA, Gallaugher L, McIntyre RS, Kennedy SH. Correlates of benzodiazepine use in major depressive disorder: the effect of anhedonia. J Affect Disord. (2015) 187:101–5.
134. Wilkowska A, Wiglusz MS, Galuszko-Wegielnik M, Wlodarczyk A, Cubala WJ. Antianhedonic effect of repeated ketamine infusions in patients with treatment resistant depression. Front Psychiatry. (2021) 12:704330. doi: 10.3389/fpsyt.2021.704330
135. Cheng T, Wallace DM, Ponteri B, Tuli M. Valium without dependence? Individual GABAA receptor subtype contribution toward benzodiazepine addiction, tolerance, and therapeutic effects. Neuropsychiatr Dis Treat. (2018) 14:1351–61. doi: 10.2147/NDT.S164307
136. Tan KR, Rudolph U, Lüscher C. Hooked on benzodiazepines: GABAA receptor subtypes and addiction. Trends Neurosci. (2011) 34:188–97. doi: 10.1016/j.tins.2011.01.004
137. Poisnel G, Dhilly M, Le Boisselier R, Barre L, Debruyne D. Comparison of five benzodiazepine-receptor agonists on buprenorphine-induced μ-opioid receptor regulation. J Pharmacol Sci. (2009) 110:36–46. doi: 10.1254/jphs.08249fp
138. Walker BM, Ettenberg A. Benzodiazepine modulation of opiate reward. Exp Clin Psychopharmacol. (2001) 9:191–7.
139. Fairholme CP, Nosen EL, Nillni YI, Schumacher JA, Tull MT, Coffey SF. Sleep disturbance and emotion dysregulation as transdiagnostic processes in a comorbid sample. Behav Res Ther. (2013) 51:540–6. doi: 10.1016/j.brat.2013.05.014
140. Hammerslag LR, Hofford RS, Kang Q, Kryscio RJ, Beckmann JS, Bardo MT. Changes in fentanyl demand following naltrexone, morphine, and buprenorphine in male rats. Drug Alcohol Depend. (2020) 207:107804. doi: 10.1016/j.drugalcdep.2019.107804
141. Höflich A, Michenthaler P, Kasper S, Lanzenberger R. Circuit mechanisms of reward, anhedonia, and depression. Int J Neuropsychopharmacology. (2019) 22:105–18.
142. Der-Avakian A, Markou A. The neurobiology of anhedonia and other reward-related deficits. Trends Neurosci. (2012) 35:68–77.
143. Rizvi SJ, Pizzagalli DA, Sproule BA, Kennedy SH. Assessing anhedonia in depression: potentials and pitfalls. Neurosci Biobehav Rev. (2016) 65:21–35. doi: 10.1016/j.neubiorev.2016.03.004
144. Treadway MT, Zald DH. Reconsidering anhedonia in depression: lessons from translational neuroscience. Neurosci Biobehav Rev. (2011) 35:537–55. doi: 10.1016/j.neubiorev.2010.06.006
145. Hatziagiakoumis DS, Martinotti G, Di Giannantonio M, Janiri L. Anhedonia and substance dependence: clinical correlates and treatment options. Front Psychiatry. (2011) 2:10. doi: 10.3389/fpsyt.2011.00010
146. Pisoni A, Davis SW, Smoski M. Neural signatures of saliency-mapping in anhedonia: a narrative review. Psychiatry Res. (2021) 304:114123. doi: 10.1016/j.psychres.2021.114123
Keywords: benzodiazepine, demand, anhedonia, affective dysregulation, opioid use disorder
Citation: Greenwald MK, Moses TEH, Lundahl LH and Roehrs TA (2023) Anhedonia modulates benzodiazepine and opioid demand among persons in treatment for opioid use disorder. Front. Psychiatry 14:1103739. doi: 10.3389/fpsyt.2023.1103739
Received: 20 November 2022; Accepted: 02 January 2023;
Published: 19 January 2023.
Edited by:
James K. Rowlett, University of Mississippi Medical Center, United StatesReviewed by:
Richard De La Garza, University of California, Los Angeles, United StatesCarolina Haass-Koffler, Brown University, United States
Sally Huskinson, University of Mississippi Medical Center, United States
Copyright © 2023 Greenwald, Moses, Lundahl and Roehrs. This is an open-access article distributed under the terms of the Creative Commons Attribution License (CC BY). The use, distribution or reproduction in other forums is permitted, provided the original author(s) and the copyright owner(s) are credited and that the original publication in this journal is cited, in accordance with accepted academic practice. No use, distribution or reproduction is permitted which does not comply with these terms.
*Correspondence: Mark K. Greenwald, bWdyZWVuQG1lZC53YXluZS5lZHU=