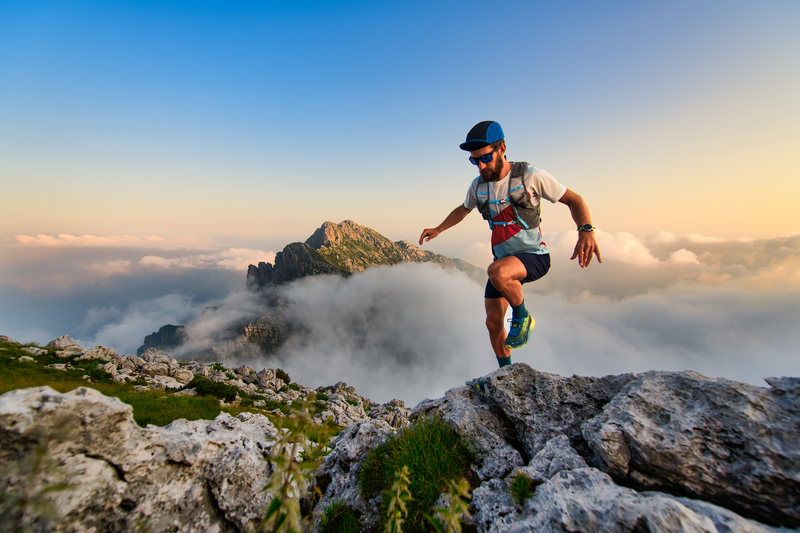
95% of researchers rate our articles as excellent or good
Learn more about the work of our research integrity team to safeguard the quality of each article we publish.
Find out more
ORIGINAL RESEARCH article
Front. Psychiatry , 09 March 2023
Sec. Computational Psychiatry
Volume 14 - 2023 | https://doi.org/10.3389/fpsyt.2023.1102811
Background: A greatly growing body of literature has revealed the mediating role of DNA methylation in the influence path from childhood maltreatment to psychiatric disorders such as post-traumatic stress disorder (PTSD) in adult. However, the statistical method is challenging and powerful mediation analyses regarding this issue are lacking.
Methods: To study how the maltreatment in childhood alters long-lasting DNA methylation changes which further affect PTSD in adult, we here carried out a gene-based mediation analysis from a perspective of composite null hypothesis in the Grady Trauma Project (352 participants and 16,565 genes) with childhood maltreatment as exposure, multiple DNA methylation sites as mediators, and PTSD or its relevant scores as outcome. We effectively addressed the challenging issue of gene-based mediation analysis by taking its composite null hypothesis testing nature into consideration and fitting a weighted test statistic.
Results: We discovered that childhood maltreatment could substantially affected PTSD or PTSD-related scores, and that childhood maltreatment was associated with DNA methylation which further had significant roles in PTSD and these scores. Furthermore, using the proposed mediation method, we identified multiple genes within which DNA methylation sites exhibited mediating roles in the influence path from childhood maltreatment to PTSD-relevant scores in adult, with 13 for Beck Depression Inventory and 6 for modified PTSD Symptom Scale, respectively.
Conclusion: Our results have the potential to confer meaningful insights into the biological mechanism for the impact of early adverse experience on adult diseases; and our proposed mediation methods can be applied to other similar analysis settings.
A greatly growing body of literature has revealed that adverse childhood experience is a leading neurodevelopmental risk factor, which could ultimately exhibit a long-lasting impact on many complex human disorders and diseases in adulthood such as cardiovascular dysregulation (1, 2), sleep disturbance (3), lung cancer (4), chronic metabolic diseases (5), especially neurological and psychiatric disorders (6–8). Adverse childhood event has a very generalized definition which includes any experience with the potential to be harmful to children, such as famine, sexual, and physical abuse as well as emotional and physical neglect (9). Among these, maltreatment in childhood has been attracted particular attention because it remains a major public health and social welfare problem worldwide (10, 11). For example, it is reported that about 4∼16% of children are physically abused and approximately 10% are neglected or psychologically abused in every year in high income countries and developed regions (11).
The negative consequence of childhood maltreatment not only involves significantly high morbidity of adult disorders and diseases, but also includes substantially increased risk of premature mortality. Indeed, it has been demonstrated that adults who ever had severe maltreatment experience in childhood would suffer from approximately 20 years shorter life expectancy on average compared to those without early adversity (11). Furthermore, childhood maltreatment is not only a well-established predictor of lifetime diseases such as diverse psychiatric disorders, linking with a higher occurrence of psychiatric health problems per se, but also associating with an earlier onset age, greater severe symptom and poorer response to psychotherapy or pharmacotherapy (12). In addition, early maltreatment experience has also been shown to have a lasting negative effect on many other important aspects of individual functions persisting from childhood into adulthood by compromising relationship quality, educational attainment, employment prospects and earnings, and physical health (12, 13). Therefore, understanding the biological mechanism of childhood maltreatment on becomes increasingly critical for developing innovative preventive and therapeutic strategies to improve health conditions.
When exploring the connection between childhood maltreatment and adult diseases, a natural question arises that why maltreatment in childhood can lead to an increased risk for negative conditions (e.g., diseases/disorders) in adulthood even many years after such an exposure itself has ceased (12). There are several possibly reasonable interpretations to answer this question and to explain the enduring influence of childhood maltreatment on adult diseases, among which the significant role of epigenetics has emerged as a promising mechanism underlying the biological sequelae of maltreatment in childhood on adverse outcome in adult (9, 14–18). Biologically, epigenetic regulation enables the body to respond to the influence of environmental factors by altering gene expression level through chemical modifications which modulate chromatin structure and/or DNA accessibility without inducing alteration to the DNA sequence. In the literature, DNA methylation is one of the most frequently investigated epigenetic regulation processes and involves the addition of a methyl group at sites in the DNA where a cytosine nucleotide occurs next to a guanine nucleotide (CpG dinucleotides).
Previous studies have established the relationship between childhood maltreatment, DNA methylation and various complex diseases/disorders. For example, it was shown that early life stress was significantly associated with DNA methylation in rodents and that low levels of maternal care (licking, grooming, and arched-back nursing) were related to greater level of methylation of the glucocorticoid receptor gene in offspring (14, 15). Lutz and Turecki showed that early life experience (e.g., childhood abuse) can modify lifelong stressful activities (e.g., psychopathological disorders) through DNA methylation regulation (19). Klengel et al. (20) found that exposure to stress would cause long-term changes in DNA methylation, which may be in turn related to the pathophysiology of psychiatric disorders such as post-traumatic stress disorder (PTSD) and depression. Several recent systematic reviews offered a comprehensive description about the connection between early maltreatment experience, altered patterns of DNA methylation and complex human diseases (9, 12, 21). Statistically, the evidence above suggests that an intermediary model can help understand how childhood maltreatment alters long-lasting changes of DNA methylation, which further affects psychiatric disorders (22–27).
However, the mechanisms regarding how childhood maltreatment possibly influences diseases remain largely elusive. Methodologically, this motivates us to consider DNA methylation as a critical causal mediator of childhood maltreatment on the development of adult diseases using mediation analysis models. As a result, the essential requirement of mediation analysis arises when we are interested in potential mechanism between an exposure (e.g., childhood maltreatment) and an outcome (e.g., psychiatric disorder) and long to acquire in-depth insight into such understanding. Formally, a mediating variable, also known as mediator, is defined as an intermediate variable (e.g., DNA methylation) in the causal sequence that connects the exposure with the outcome (28). From a statistical perspective, mediation analysis can be applied to investigate the interplay between childhood maltreatment, DNA methylation and disorder (28–35).
Traditionally, single CpG site mediation analysis is conducted to identify methylations with mediating role; however, as the number of CpG sites can be much larger than sample size, mediation analysis focusing on one mediator at a time has become increasingly challenging and often infeasible to directly apply them towards the large and complex data collected in an epigenetic study (35, 36). Furthermore, the following three facts motivate us to implement gene-centric mediation analysis in the present work. First, methylation signals within a gene are often correlated with each other, sometimes quite strongly. For example, methylations on proximal CpG sites are generally similar to each other and genes in the same pathway often show coordinated co-expression pattern (37). Such correlation, if not correctly handled, would result in false discoveries in the traditional single CpG site mediation analysis. Second, as genes are important functional units in living organisms, gene-based mediation analysis offers more biologically meaningful results. Third, a gene likely contains several mediation signals, single CpG site mediation analysis is thus conservative and does not fare well for large-scale multiple testing tasks (38–41); in contrast, analyzing the mediation effect of the whole gene rather than focusing on individual methylation site is generally more powerful (34).
In this study, with relevant data available from the Grady Trauma Project (GTP) as an illustrative example (8, 42), we intend to carry out a mediation analysis using composite null hypothesis to examine the mediating role of DNA methylation on the signaling pathway from childhood maltreatment to psychiatric disorders in adult, where the two path association tests could be implemented through an equivalent manner of variance-component based score tests under the context of mixed models. More specifically, we would implement the gene-centric mediation analysis to simultaneously model a group of DNA methylation CpG sites as mediators and explore their mediating role in the impact of childhood maltreatment on psychiatric disorders (Figure 1).
Figure 1. Statistical framework of the gene-centric mediation analysis with one exposure X (e.g., childhood maltreatment), multiple DNA methylation mediators M, and one outcome Y (e.g., PTSD). Here, α = (α1, …, αK) is the vector of effect sizes of the exposure on K mediators in the exposure-mediator model, β = (β1, …, βK) is the vector of effect sizes of the K mediators on the outcome in the mediator-outcome model, c′ is the direct effect conditional on the mediators, and c is the total exposure on outcome effect. There are two types of effects from X to Y: the direct effect of X on Y (i.e., c′) and the indirect effect of X on Y via the intermediate variables M. The indirect effect, also known as mediation effect denoted by αβ, represents the amount of mediation coming from two sources: the effect from X to M (i.e., α) and the effect from M to Y (i.e., β).
To effectively detect the existence of mediation effect with high-dimensional mediators, we first employ the variance component-based score test in a linear mixed model via an inverse transformation to assess the association between childhood maltreatment and a group of DNA methylation CpG sites, and then test for the effect of DNA methylation on the disorder of focus while adjusting for the direct influence of childhood maltreatment within the framework of linear mixed-effects model (43–46). We finally determine whether the mediation effect of DNA methylation exists by following the main idea proposed in Liu et al. (41), which can lead to desirable type I error rate control and higher power compared to existing mediation effect test methods.
The GTP was a cross-sectional study dedicated to investigate the role of environmental and genetic risk factors in the development of stress-related psychiatric disorders, such as PTSD, depressive disorder, and anxiety disorder, that confer a heavy burden on public health worldwide (8, 47). We downloaded datasets of various types of depression/stress scores (e.g., current stress score and cumulative life stress score), baseline features (e.g., sex and age) and DNA methylation for African-American participants with PTSD from GTP (the GEO accession number: GSE72680).
The exposure of our interest is childhood maltreatment including emotional abuse, physical abuse, sexual abuse, emotional neglect, and physical neglect, which was measured using the Childhood Trauma Questionnaire (48) and further dichotomized in terms of the moderate to extreme criteria. Current PTSD symptoms and depression symptoms were assessed with the modified PTSD Symptom Scale (PSS) (49) and the Beck Depression Inventory (BDI) (50), respectively. Following prior work (51), in our analysis we defined PTSD cases with comorbid current PTSD and depression symptoms (PTSD&Dep) as having PSS score ≥14 and BDI score ≥14, and defined controls with no current PTSD and no depressive symptoms as having PSS score ≤7 and BDI score ≤7 despite being exposed to trauma. Other continuous outcomes of interest include distinct types of depression or stress scores (Table 1).
For quality control we filtered out those already receiving PTSD-relevant treatments (such as treatments for depression, bipolar disorder, PTSD, and anxiety disorder) since the treatments might affect DNA methylation changes which can complicate the mediation effect. More details about the GTP study can be found elsewhere (8, 42).
DNA methylation levels of these GTP subjects were measured in peripheral blood using the Illumina Infinium Human Methylation 450K BeadChip. Batch effects and other potential latent sources of variation [e.g., environmental variables (52) and genetic variation (53, 54)] were removed by using R package “sva” (version 3.35.2) (55). We removed non-CpG sites (probes with ch labels) and CpG sites located on sex chromosomes, and excluded cross-reactive probes as suggested in Chen et al. (56). After the data processing, 264,564 DNA methylation CpG sites were retained. Furthermore, the beta value for each DNA methylation at a CpG site was logit-transformed to generate the M-value for each locus because, unlike the beta value, the M-value is not bounded between zero and one and is thus more valid for statistical modeling (57).
We adjusted for the confounding effects of age, sex, body mass index (BMI) when examining the association between childhood maltreatment and DNA methylation as well as the association between DNA methylation and PTSD or other score outcomes. In addition, cell type was the single most important known factor in determining DNA methylation profiles (58) and some differences in cell types might significantly confound results from DNA methylation analyses (59, 60); we therefore included blood cell type (i.e., CD4T, CD8T, Mono, B cell, and NK) proportions as covariates, which was directly obtained by the laboratory file of the data.
We treat childhood maltreatment as the exposure and PTSD (or score) as the outcome, and apply an epigenetic mediation framework to investigate how the maltreatment in childhood alters long-lasting DNA methylation changes, which in turn affects adult psychiatric disorders such as PTSD (Figure 1). More specifically, we would carry out a gene-centric mediation analysis (34, 35, 40), where each mediation effect test includes one exposure X, multiple methylation mediators M which are per se likely high-dimensional, and one outcome Y that may be continuous or binary for n individuals. According to the gene annotation mapping file we define the set of DNA methylation CpG sites for each gene as those located within the entire gene body and 500 bps upstream of the transcription start site (TSS) so that the promoter can be included (61, 62). A total of 16,295 genes were analyzed, with the median of the number of DNA methylation CpG sites per gene equal to 16.
In the mediation analysis the influence of the exposure X on the outcome Y stands for the direct effect (denoted by c′ in Figure 1), and the influence of X on M and subsequently M on Y for the indirect effect which is also called the mediation effect (denoted by αβ in Figure 1). If X affects Y only through M (i.e., c′ is zero), such association is referred to as full mediation; otherwise, it is referred to as partial mediation (i.e., c′ is non-zero) (35, 63). Our objective is to assess whether the causal effect of X on Y is mediated via M. Note that, traditionally, the presence of a significant total effect (i.e., c ≠ 0 in the outcome-exposure model) is the prerequisite for the mediation effect test (29). However, in practice the situation in which the total effect is nonsignificant but a substantial mediation effect remains is not uncommon; for example, the direct effect is opposite in sign to the indirect effect and has a similar size in magnitude, which is referred to as suppression or inconsistent mediation (64–68). Therefore, following prior studies (66, 67), in the present work we always carry out our mediation effect test regardless of whether there exists a substantial total effect of the exposure on.
For each gene under analysis, we first apply an inverse regression as done in Djordjilović et al. (69) and treat the impacts of CpG sites (mediators) on childhood maltreatment (exposure) as random effects; thus the associations between childhood maltreatment and methylation in the exposure-mediator model can be derived by applying a variance component test in the linear mixed model. In the next step, we use the same strategy to utilize a mixed model and assess the association between methylation and PTSD (or scores) in the mediator-outcome model by Davies’ method via the SKAT package (43, 70). Finally, a joint significance test is conducted to evaluate the significance of the mediation effects using a Benjamini–Hochberg false discovery rate (FDR) correction for multiple testing. To his aim, we here follow the main idea of divide-aggregate composite-null test (DACT) proposed in Liu et al. (41); to formally obtain the P-value of mediation effect, we rely on a modified test statistic and utilize the Efron empirical null framework to estimate the proportions of the three sub-null hypotheses across whole genome mediators. The pipeline of this mediation analysis is described in detail in Supplementary material.
A total of 191 cases with the symptom of comorbid current PTSD/depression and 161 controls were finally reserved for further analysis. Between-group differences in clinical features of all the remaining participants are summarized in Table 1. The position of DNA methylation CpG sites of all the 352 participants were determined by gene symbols, and a total of 16,565 unique genes were obtained.
We first examined the association between childhood maltreatment and PTSD or other PTSD-related score outcomes (i.e., H0: c = 0). The results for the total effect are shown in Table 2. It was observed that the maltreatment in childhood substantially affected the development of PTSD or increased PTSD-relevant scores after adjusting for the multiple-test issue at the FDR level of 0.05. For example, the experience of extreme maltreatment in childhood would significantly result in higher risk [odds ratio = 2.16, 95% confidence intervals (CIs) 1.35∼3.46) of occurring PTSD in adult (P = 1.65 × 10–3), lead to an increase of 6.55 units (95% CIs 3.76∼9.33) in the BDI total score (P = 7.25 × 10–6) or an elevation of 1.86 units (95% CIs 1.19∼2.52) in the current stress score (P = 1.07 × 10–7).
Table 2. Results of the gene-centric mediation analysis with childhood maltreatment as the exposure, DNA methylation CpG sites located within a gene as mediators, and PTSD and six PTSD-relevant scores as outcomes.
We next evaluated the association between childhood maltreatment and a group of DNA methylation CpG sites (i.e., H0: α = 0) and the association between a set of DNA methylation and PTSD (and various score outcomes) for each gene (i.e., H0: β = 0). As a result, it was shown that the maltreatment in childhood possibly affected many promising DNA methylation CpG sites across these PTSD-relevant outcomes, with the number of significant associations ranging from 670 for the BDI total score to 1,392 for the network life stress score (P < 0.05). On the other hand, after the adjustment of the direct effect of maltreatment, we identified many DNA methylation CpG sites associated with PTSD or other PTSD-related score outcomes, with the number of promising association signals ranging from 349 for the network life stress score to 1,996 for PTSD (P < 0.05).
Finally, we applied DACT to detect DNA methylation CpG sites that may exert mediating effects on the path from the maltreatment in childhood to PTSD-relevant outcomes in adult. We found that there were 13 and 6 genes with methylation CpG sites having mediation effects for the BDI total score and the current stress score (FDR <0.05), respectively. In addition, although methylation CpG sites in these genes exhibited mediating roles, only a few sites were significantly differentially methylated between the cases and controls (FDR <0.05). We show more detailed information regarding these DNA methylation CpG sites in Table 3.
Table 3. Located genes of DNA methylation CpG sites with mediation effects for the Beck depression inventory total score and the current stress score.
A great deal of literature has revealed the mediating role of epigenetic alteration such as DNA methylation standing on the path from early adverse experience (e.g., childhood maltreatment) to adult psychotic disorders (9, 12, 14–18). However, to our knowledge, there are currently only few studies which formally investigate this important issue under the hypothesis testing framework of composite null hypothesis mediation analysis. The present work is exactly among the first to explore whether DNA methylation CpG sites have a substantial mediating effect from a contemporary mediation perspective of composite null hypothesis testing. Previous work has already demonstrated that considering the composite null nature in mediation effect test holds the key to improve power for identifying active mediators in mediation analysis (34, 35, 38–41, 71).
In the present study, we applied a recently proposed novel method called DACT to determinate which DNA methylation CpG sites may play the mediating role in the connection between childhood maltreatment and PTSD or PTSD-related scores. The advantage of DACT is that it only takes two sets of P-values as input, can thus be scalable to studies with large scale sample size in mediation analysis (41). In addition, DACT can borrow the useful information of all DNA methylation mediators across the whole genome to estimate some necessary parameters. Note that, it has been shown that, compared with other composite-null based mediation effect test methods such as the JS-comp which circumvents estimating the proportion parameters of composite null sub-hypotheses under some strong modeling assumptions (40, 72), considering study-specific scenarios and estimating these proportion parameters are crucial to generate well-calibrated type I error control in mediation analysis (34, 35, 41).
In addition, unlike prior work which considered a single DNA methylation CpG site in each time (36), we instead analyzed a group of DNA methylation CpG sites located within their nearby gene jointly when detecting active methylation mediators. As demonstrated in previous work (34), the number of DNA methylation CpG sites can be much greater than sample size; thus, focusing on one mediator at a time is not efficiently powerful enough to handle thousands of mediators. The strength of this gene-centric mediation analysis is that it has the potential to further enhance the power by aggravating multiple weak association signals. In the meantime, this gene-centric mediation effect method also challenges the traditional single mediator mediation analysis (40). We effectively addressed this challenging task by leveraging a random-effect model in which we assumed the effects of the exposure on the mediators and the effects of multiple mediators on the outcome followed a normal distribution with an unknown variance parameter. Therefore, the two path association tests in our mediation analysis can be implemented by an equivalent manner of variance-component based score tests under the context of mixed models. It has been demonstrated that the variance component test is often more powerful compared to its counterpart such as multivariate Wald test (43, 44, 46, 73–76).
As a result, using DACT with two P-values available from variance component tests, we discovered a set of DNA methylation within some genes having promising mediation effect. Many of these genes were previously demonstrated to be associated with psychiatric disorders such as stress, depression, schizophrenia, and PTSD. Note that, psychiatric disorders are highly correlated in genetic foundation and clinical manifestation (77–82). For example, PPM1L, encoding protein phosphatase, was detected to be responsible for the regulation of stress-activated protein kinase signaling cascade and apoptosis (83, 84). It is worth noting that this gene also identified by a previous study (33). CNRIP1, encoding the cannabinoid receptor interacting protein 1, is an intracellular protein that interacts with the C-terminal tail of CB1R and regulates its intrinsic activity. Aberrant CNRIP1 DNA promoter methylation was observed in post-mortem in human patients with schizophrenia (85), and decreased methylation of the CNRIP1 DNA promoter was discovered in the ventral hippocampus of a rodent model of schizophrenia susceptibility (86). Recent work showed that ventral hippocampal overexpression of CNRIP1 would lead to a schizophrenia-like phenotype in the rat (87). In addition, CNRIP1 was a regulator involved in the neural development (88). SLC25A23 encodes the human APC2 (ATP-Mg/Pi carrier), which is a part of Ca2+ sensitive mitochondrial carriers; and SLC25A23 mediates the influx of Ca2+ and regulates ATP levels in neurons (89). The altered SLC25 family gene expression was revealed to be a marker of mitochondrial dysfunction in brain regions under experimental mixed anxiety/depression-like disorder (90). Moreover, SLC25A23 was identified as a candidate biomarker gene that can improve low mood state of major depressive disorder (MDD) (91); a recent study further confirmed this finding and demonstrated that up-regulation of SLC25A23 might be associated with MDD (92). As another example, a genome-wide association study showed that a genetic variant mapping to the first intron of TLL1 was strongly related to PTSD, implying that TLL1 might be a susceptibility gene for this disorder (93). Additionally, SNAP91, encoding the synaptosome-associated protein 91, was identified to be a risk gene of schizophrenia (94, 95).
Besides the direct evidence described above, prior studies also offered indirect evidence supporting the association for some of these gene with brain-related diseases such as neurodegenerative diseases. For instance, mutations of MYO7A were found to be responsible for Usher syndrome, a brain-related disease (96). ABAT, a key enzyme responsible for catabolism of principal inhibitory neurotransmitter γ-aminobutyric acid (GABA), exhibited the connection between GABA metabolism and nucleoside metabolism and played a key role in developing neurometabolic disorders such as mtDNA depletion syndrome (97). In addition, APOC1 encodes a member of the apolipoprotein C1 family, which plays a well-established role in the transport and metabolism of lipids within the central nervous system and is critical for healthy brain function. The importance of apolipoproteins in brain physiology is also highlighted by many genetic studies, where apolipoprotein gene polymorphisms have been identified as risk factors for several neurological diseases (98). In addition, it is shown that the APOC1 insertion allele, in combination with APOE ε4, likely served as a potential risk factor for developing Alzheimer’s disease (99).
Finally, our study is not without limitations. First, we assumed that all DNA methylation CpG sites were affected by childhood maltreatment and had influences on PTSD-relevant outcomes. This assumption might be not true as it was likely only a fraction of methylation with substantial effects. Consequently, under this scenario a sparse relationship was more suitable. Second, because PTSD is a brain-relevant disorder and it was impossible and ethically unreasonable for the availability of brain tissues from individuals alive, in this work the analyzed DNA methylation had to be measured in peripheral blood, which might not fully reflect DNA methylation alteration in brain tissues. Third, although they might be considerably important, some other covariates (e.g., tobacco smoking, alcohol drinking, cocaine use, heroin use, and marijuana use) were simply ignored in accordance with previous study (36, 100) as these variables had relatively high missing values. Variation in DNA methylation is strongly associated with lifestyle factors such as smoking behavior (101–103); one possible solution to covariates with high missing values is to develop a methylation score to efficiently predict smoking status (104–107). However, if we attempt to apply this predictive approach to impute missing data, to avoid model overfitting we must look at a random split of the data into two non-overlapping samples—one for prediction and the other for mediation analysis, which would certainly lead to reduced power in mediation effect test. Fourth, only one PTSD dataset was analyzed, no external datasets were used for validating our discovered methylation sites with mediating effects. In addition, further longitudinal data that can provide time-span facts for discovered DNA methylation CpG sites is particularly warranted.
In conclusion, by leveraging novel statistical methods we identified multiple promising DNA methylation sites that had mediating roles in the influence path from childhood maltreatment to PTSD-relevant outcomes in adult. Our results have the potential to confer meaningful insights into the biological mechanism for the impact of early adverse experience on adult diseases; and our proposed mediation methods can be applied to other similar analysis settings.
The data presented in the study are deposited in the GTP repository, accession number GSE72680. The data can be found at: https://www.ncbi.nlm.nih.gov/geo/query/acc.cgi?acc=GSE72680.
PZ and HX contributed to conception of the study. PZ and ZS obtained the data, cleared up the datasets, and performed the data analyses. PZ, SZ, HX, XL, and ZS interpreted the results of the data analyses, and drafted and revised the manuscript. All authors contributed to the article and approved the submitted version.
This research of PZ was supported in part by the National Natural Science Foundation of China (82173630 and 81402765), the Youth Foundation of Humanity and Social Science Funded by Ministry of Education of China (18YJC910002), the Natural Science Foundation of Jiangsu Province of China (BK20181472), the China Postdoctoral Science Foundation (2018M630607 and 2019T120465), the Qinglan Research Project of Jiangsu Province for Young and Middle-Aged Academic Leaders, the Six-Talent Peaks Project in Jiangsu Province of China (WSN-087), the Training Project for Youth Teams of Science and Technology Innovation at Xuzhou Medical University (TD202008), the Postdoctoral Science Foundation of Xuzhou Medical University, and the Statistical Science Research Project from National Bureau of Statistics of China (2014LY112). This research of HX was also supported by the Industry-University Collaborative Education Project of the Higher Education Department of MOE (202101085008), the Special Subject Key Project Jiangsu Higher Education of Jiangsu Higher Education Association (2022JSGJKT008), and Xuzhou Social Development Key R&D Plan (KC21306). This research of SZ was supported by Postgraduate Research and Practice Innovation Program of Jiangsu Province (KYCX22_2960). The funders had no role in study design, data collection and analysis, decision to publish, or preparation of the manuscript.
We express our gratitude to the Grady Trauma Project for making its datasets publicly available and are indebted to all the investigators and participants contributed to this study. The data analyses in the present study were carried out with the high-performance computing cluster that was supported by the special central finance project of local universities for Xuzhou Medical University. We are very grateful to the editor and four reviewers for their constructive comments, which have resulted in a dramatic improvement of the earlier version of this manuscript.
The authors declare that the research was conducted in the absence of any commercial or financial relationships that could be construed as a potential conflict of interest.
All claims expressed in this article are solely those of the authors and do not necessarily represent those of their affiliated organizations, or those of the publisher, the editors and the reviewers. Any product that may be evaluated in this article, or claim that may be made by its manufacturer, is not guaranteed or endorsed by the publisher.
The Supplementary Material for this article can be found online at: https://www.frontiersin.org/articles/10.3389/fpsyt.2023.1102811/full#supplementary-material
1. Pretty C, O’Leary DD, Cairney J, Wade TJ. Adverse childhood experiences and the cardiovascular health of children: a cross-sectional study. BMC Pediatr. (2013) 13:208. doi: 10.1186/1471-2431-13-208
2. Allen H, Wright BJ, Vartanian K, Dulacki K, Li HF. Examining the prevalence of adverse childhood experiences and associated cardiovascular disease risk factors among low-income uninsured adults. Circulation. (2019) 12:e004391. doi: 10.1161/CIRCOUTCOMES.117.004391
3. Chapman DP, Wheaton AG, Anda RF, Croft JB, Edwards VJ, Liu Y, et al. Adverse childhood experiences and sleep disturbances in adults. Sleep Med. (2011) 12:773–9.
4. Brown DW, Anda RF, Felitti VJ, Edwards VJ, Malarcher AM, Croft JB, et al. Adverse childhood experiences are associated with the risk of lung cancer: a prospective cohort study. BMC Public Health. (2010) 10:20. doi: 10.1186/1471-2458-10-20
5. Zimmet P, Shi Z, El-Osta A, Ji L. Epidemic T2DM, early development and epigenetics: implications of the Chinese famine. Nat Rev Endocrinol. (2018) 14:738–46. doi: 10.1038/s41574-018-0106-1
6. Arnow B. Relationships between childhood maltreatment, adult health and psychiatric outcomes, and medical utilization. J Clin Psychiatry. (2004) 65:10–5.
7. Chapman DP, Whitfield CL, Felitti VJ, Dube SR, Edwards VJ, Anda RF. Adverse childhood experiences and the risk of depressive disorders in adulthood. J Affect Disord. (2004) 82:217–25. doi: 10.1016/j.jad.2003.12.013
8. Gillespie CF, Bradley B, Mercer K, Smith AK, Conneely K, Gapen M, et al. Trauma exposure and stress-related disorders in inner city primary care patients. Gen Hosp Psychiatry. (2009) 31:505–14. doi: 10.1016/j.genhosppsych.2009.05.003
9. Parade SH, Huffhines L, Daniels TE, Stroud LR, Nugent NR, Tyrka AR. A systematic review of childhood maltreatment and DNA methylation: candidate gene and epigenome-wide approaches. Transl Psychiatry. (2021) 11:134. doi: 10.1038/s41398-021-01207-y
10. Brown DW, Anda RF, Tiemeier H, Felitti VJ, Edwards VJ, Croft JB, et al. Adverse childhood experiences and the risk of premature mortality. Am J Prev Med. (2009) 37:389–96. doi: 10.1016/j.amepre.2009.06.021
11. Gilbert R, Widom CS, Browne K, Fergusson D, Webb E, Janson S. Burden and consequences of child maltreatment in high-income countries. Lancet. (2009) 373:68–81. doi: 10.1016/s0140-6736(08)61706-7
12. Cecil CAM, Zhang Y, Nolte T. Childhood maltreatment and DNA methylation: a systematic review. Neurosci Biobehav Rev. (2020) 112:392–409. doi: 10.1016/j.neubiorev.2020.02.019
13. Danese A, Moffitt TE, Harrington H, Milne BJ, Polanczyk G, Pariante CM, et al. Adverse childhood experiences and adult risk factors for age-related disease: depression, inflammation, and clustering of metabolic risk markers. Arch Pediatr Adolesc Med. (2009) 163:1135–43. doi: 10.1001/archpediatrics.2009.214
14. Weaver ICG, Cervoni N, Champagne FA, D’Alessio AC, Sharma S, Seckl JR, et al. Epigenetic programming by maternal behavior. Nat Neurosci. (2004) 7:847–54.
15. Weaver ICG, Champagne FA, Brown SE, Dymov S, Sharma S, Meaney MJ, et al. Reversal of maternal programming of stress responses in adult offspring through methyl supplementation: altering epigenetic marking later in life. J Neurosci. (2005) 25:11045–54. doi: 10.1523/JNEUROSCI.3652-05.2005
16. Kim JK, Samaranayake M, Pradhan S. Epigenetic mechanisms in mammals. Cell Mol Life Sci. (2009) 66:596–612. doi: 10.1007/s00018-008-8432-4
17. Tyrka AR, Ridout KK, Parade SH. Childhood adversity and epigenetic regulation of glucocorticoid signaling genes: associations in children and adults. Dev Psychopathol. (2016) 28:1319–31. doi: 10.1017/S0954579416000870
18. Parent J, Parade SH, Laumann LE, Ridout KK, Yang B-Z, Marsit CJ, et al. Dynamic stress-related epigenetic regulation of the glucocorticoid receptor gene promoter during early development: the role of child maltreatment. Dev Psychopathol. (2017) 29:1635–48. doi: 10.1017/s0954579417001298
19. Lutz PE, Turecki G. DNA methylation and childhood maltreatment: from animal models to human studies. Neuroscience. (2014) 264:142–56. doi: 10.1016/j.neuroscience.2013.07.069
20. Klengel T, Pape J, Binder EB, Mehta D. The role of DNA methylation in stress-related psychiatric disorders. Neuropharmacology. (2014) 80:115–32. doi: 10.1016/j.neuropharm.2014.01.013
21. Misra P, Liu S, Meng X. What DNA methylation modifications and/or genetic variations interact with childhood maltreatment in the development of depression: a systematic review. J Affect Disord. (2019) 252:325–33. doi: 10.1016/j.jad.2019.04.049
22. Mahgoub M, Monteggia LM. Epigenetics and psychiatry. Neurotherapeutics. (2013) 10:734–41. doi: 10.1007/s13311-013-0213-6
23. Kundakovic M. Chapter 19—epigenetics of psychiatric disorders. In: Tollefsbol TO editor. Medical Epigenetics. (Boston, MA: Academic Press) (2016). p. 335–50.
24. Kular L, Kular S. Epigenetics applied to psychiatry: clinical opportunities and future challenges. Psychiatry Clin Neurosci. (2018) 72:195–211. doi: 10.1111/pcn.12634
25. Blacker CJ, Frye MA, Morava E, Kozicz T, Veldic M. A review of epigenetics of PTSD in comorbid psychiatric conditions. Genes. (2019) 10:140. doi: 10.3390/genes10020140
26. Lin E, Tsai SJ. Epigenetics and depression: an update. Psychiatry Investig. (2019) 16:654–61. doi: 10.30773/pi.2019.07.17.2
27. Smigielski L, Jagannath V, Rössler W, Walitza S, Grünblatt E. Epigenetic mechanisms in schizophrenia and other psychotic disorders: a systematic review of empirical human findings. Mol Psychiatry. (2020) 25:1718–48. doi: 10.1038/s41380-019-0601-3
29. Baron RM, Kenny DA. The moderator-mediator variable distinction in social psychological research: conceptual, strategic, and statistical considerations. J Pers Soc Psychol. (1986) 51:1173–82.
30. Imai K, Keele L, Tingley D. A general approach to causal mediation analysis. Psychol Methods. (2010) 15:309.
32. VanderWeele T. Explanation in Causal Inference: Methods for Mediation and Interaction. Oxford: Oxford University Press (2015).
33. Fang R, Yang H, Gao Y, Cao H, Goode EL, Cui Y. Gene-based mediation analysis in epigenetic studies. Brief Bioinform. (2021) 22:bbaa113. doi: 10.1093/bib/bbaa113
34. Shao Z, Wang T, Zhang M, Jiang Z, Huang S, Zeng P. IUSMMT: survival mediation analysis of gene expression with multiple DNA methylation exposures and its application to cancers of TCGA. PLoS Comput Biol. (2021) 17:e1009250. doi: 10.1371/journal.pcbi.1009250
35. Zeng P, Shao Z, Zhou X. Statistical methods for mediation analysis in the era of high-throughput genomics: current successes and future challenges. Comput Struct Biotechnol J. (2021) 19:3209–24. doi: 10.1093/hmg/ddab056
36. Gao Y, Yang H, Fang R, Zhang Y, Goode EL, Cui Y. Testing mediation effects in high-dimensional epigenetic studies. Front Genet. (2019) 10:1195. doi: 10.3389/fgene.2019.01195
37. Shang L, Smith JA, Zhou X. Leveraging gene co-expression patterns to infer trait-relevant tissues in genome-wide association studies. PLoS Genet. (2020) 16:e1008734. doi: 10.1371/journal.pgen.1008734
38. Barfield R, Shen J, Just AC, Vokonas PS, Schwartz J, Baccarelli AA, et al. Testing for the indirect effect under the null for genome-wide mediation analyses. Genet Epidemiol. (2017) 41:824–33. doi: 10.1002/gepi.22084
39. Huang YT. Genome-wide analyses of sparse mediation effects under composite null hypotheses. Ann Appl Stat. (2019) 13:60–84. doi: 10.1214/18-aoas1181
40. Huang YT. Variance component tests of multivariate mediation effects under composite null hypotheses. Biometrics. (2019) 75:1191–204. doi: 10.1111/biom.13073
41. Liu Z, Shen J, Barfield R, Schwartz J, Baccarelli AA, Lin X. Large-scale hypothesis testing for causal mediation effects with applications in genome-wide epigenetic studies. J Am Stat Assoc. (2021) 117:67–81. doi: 10.1080/01621459.2021.1914634
42. Binder EB, Bradley RG, Liu W, Epstein MP, Deveau TC, Mercer KB, et al. Association of FKBP5 polymorphisms and childhood abuse with risk of posttraumatic stress disorder symptoms in adults. JAMA. (2008) 299:1291–305. doi: 10.1001/jama.299.11.1291
43. Wu MC, Lee S, Cai T, Li Y, Boehnke M, Lin X. Rare-variant association testing for sequencing data with the sequence kernel association test. Am J Hum Genet. (2011) 89:82–93. doi: 10.1016/j.ajhg.2011.05.029
44. Zeng P, Zhao Y, Liu J, Liu L, Zhang L, Wang T, et al. Likelihood ratio tests in rare variant detection for continuous phenotypes. Ann Hum Genet. (2014) 78:320–32. doi: 10.1111/ahg.12071
45. Zeng P, Zhao Y, Li H, Wang T, Chen F. Permutation-based variance component test in generalized linear mixed model with application to multilocus genetic association study. BMC Med Res Methodol. (2015) 15:37. doi: 10.1186/s12874-015-0030-1
46. Qiao J, Shao Z, Wu Y, Zeng P, Wang T. Detecting associated genes for complex traits shared across East Asian and Europeanpopulations under the framework of composite null hypothesis testing. J Transl Med. (2022) 20:424. doi: 10.1186/s12967-022-03637-8
47. Uddin M, Ratanatharathorn A, Armstrong D, Kuan PF, Aiello AE, Bromet EJ, et al. Epigenetic meta-analysis across three civilian cohorts identifies NRG1 and HGS as blood-based biomarkers for post-traumatic stress disorder. Epigenomics. (2018) 10:1585–601. doi: 10.2217/epi-2018-0049
48. Bernstein DP, Ahluvalia T, Pogge D, Handelsman L. Validity of the childhood trauma questionnaire in an adolescent psychiatric population. J Am Acad Child Adolesc Psychiatry. (1997) 36:340–8. doi: 10.1097/00004583-199703000-00012
49. Foa EB, Riggs DS, Dancu CV, Rothbaum BO. Reliability and validity of a brief instrument for assessing post-traumatic stress disorder. J Trauma Stress. (1993) 6:459–73. doi: 10.1002/jts.2490060405
50. Beck AT, Ward CH, Mendelson M, Mock J, Erbaugh J. An inventory for measuring depression. Arch Gen Psychiatry. (1961) 4:561–71. doi: 10.1001/archpsyc.1961.01710120031004
51. Wingo AP, Velasco ER, Florido A, Lori A, Choi DC, Jovanovic T, et al. Expression of the PPM1F gene is regulated by stress and associated with anxiety and depression. Biol Psychiatry. (2018) 83:284–95. doi: 10.1016/j.biopsych.2017.08.013
52. Gibson G. The environmental contribution to gene expression profiles. Nat Rev Genet. (2008) 9:575–81. doi: 10.1038/nrg2383
53. Brem RB, Yvert G, Clinton R, Kruglyak L. Genetic dissection of transcriptional regulation in budding yeast. Science. (2002) 296:752–5. doi: 10.1126/science.1069516
54. Schadt EE, Monks SA, Drake TA, Lusis AJ, Che N, Colinayo V, et al. Genetics of gene expression surveyed in maize, mouse and man. Nature. (2003) 422:297–302. doi: 10.1038/nature01434
55. Leek JT, Johnson WE, Parker HS, Jaffe AE, Storey JD. The sva package for removing batch effects and other unwanted variation in high-throughput experiments. Bioinformatics. (2012) 28:882–3. doi: 10.1093/bioinformatics/bts034
56. Chen Y, Lemire M, Choufani S, Butcher D, Grafodatskaya D, Zanke B, et al. Discovery of cross-reactive probes and polymorphic CpGs in the Illumina Infinium HumanMethylation450 microarray. Epigenetics. (2013) 8:203–9. doi: 10.4161/epi.23470
57. Du P, Zhang X, Huang C-C, Jafari N, Kibbe WA, Hou L, et al. Comparison of beta-value and M-value methods for quantifying methylation levels by microarray analysis. BMC Bioinformatics. (2010) 11:587. doi: 10.1186/1471-2105-11-587
58. Jones MJ, Islam SA, Edgar RD, Kobor MS. Adjusting for cell type composition in DNA methylation data using a regression-based approach. In: Haggarty P, Harrison K editors. Population Epigenetics: Methods and Protocols. (New York, NY: Springer New York) (2017). p. 99–106. doi: 10.1007/7651_2015_262
59. Reinius LE, Acevedo N, Joerink M, Pershagen G, Dahlén SE, Greco D, et al. Differential DNA methylation in purified human blood cells: implications for cell lineage and studies on disease susceptibility. PLoS One. (2012) 7:e41361. doi: 10.1371/journal.pone.0041361
60. Jaffe AE, Irizarry RA. Accounting for cellular heterogeneity is critical in epigenome-wide association studies. Genome Biol. (2014) 15:R31. doi: 10.1186/gb-2014-15-2-r31
61. Liu L, Zeng P, Yang S, Yuan Z. Leveraging methylation to identify the potential causal genes associated with survival in lung adenocarcinoma and lung squamous cell carcinoma. Oncol Lett. (2020) 20:193–200. doi: 10.3892/ol.2020.11564
62. Zhang J, Lu H, Zhang S, Wang T, Zhao H, Guan F, et al. How can gene-expression information improve prognostic prediction in TCGA cancers: an empirical comparison study on regularization and mixed effect survival models. Front Genet. (2021) 11:920. doi: 10.3389/fgene.2020.00920
63. VanderWeele TJ. Introduction to statistical mediation analysis by Mackinnon, D. P. Biometrics. (2009) 65:998–1000. doi: 10.1111/j.1541-0420.2009.01315_12.x
64. MacKinnon DP, Krull JL, Lockwood CM. Equivalence of the mediation, confounding and suppression effect. Prev Sci. (2000) 1:173–81. doi: 10.1023/a:1026595011371
65. MacKinnon DP, Lockwood CM, Hoffman JM, West SG, Sheets V. A comparison of methods to test mediation and other intervening variable effects. Psychol Methods. (2002) 7:83–104.
66. Shrout PE, Bolger N. Mediation in experimental and nonexperimental studies: new procedures and recommendations. Psychol Methods. (2002) 7:422–45.
67. Valeri L, VanderWeele TJ. Mediation analysis allowing for exposure–mediator interactions and causal interpretation: theoretical assumptions and implementation with SAS and SPSS macros. Psychol Methods. (2013) 18:137. doi: 10.1037/a0031034
68. Fairchild AJ, McDaniel HL. Best (but oft-forgotten) practices: mediation analysis. Am J Clin Nutr. (2017) 105:1259–71. doi: 10.3945/ajcn.117.152546
69. Djordjilović V, Page C, Gran J, Nøst T, Sandanger T, Veierød M, et al. Global test for high-dimensional mediation: testing groups of potential mediators. Stat Med. (2019) 38:3346–60. doi: 10.1002/sim.8199
70. Davies RB. Algorithm AS 155: the distribution of a linear combination of chi-2 random variables. Appl Stat. (1980) 29:323–33. doi: 10.2307/2346911
71. Dai JY, Stanford JL, LeBlanc M. A multiple-testing procedure for high-dimensional mediation hypotheses. J Am Stat Assoc. (2022) 117:198–213. doi: 10.1080/01621459.2020.1765785
72. Huang YT, Pan WC. Hypothesis test of mediation effect in causal mediation model with high-dimensional continuous mediators. Biometrics. (2016) 72:402–13. doi: 10.1111/biom.12421
73. Lin X, Cai T, Wu MC, Zhou Q, Liu G, Christiani DC, et al. Kernel machine SNP-set analysis for censored survival outcomes in genome-wide association studies. Genet Epidemiol. (2011) 35:620–31. doi: 10.1002/gepi.20610
74. Schifano ED, Epstein MP, Bielak LF, Jhun MA, Kardia SLR, Peyser PA, et al. SNP set association analysis for familial data. Genet Epidemiol. (2012) 36:797–810. doi: 10.1002/gepi.21676
75. Huang YT, Lin X. Gene set analysis using variance component tests. BMC Bioinformatics. (2013) 14:210. doi: 10.1186/1471-2105-14-210
76. Ionita-Laza I, Lee S, Makarov V, Buxbaum JD, Lin X. Sequence kernel association tests for the combined effect of rare and common variants. Am J Hum Genet. (2013) 92:841–53. doi: 10.1016/j.ajhg.2013.04.015
77. Sullivan PF, Daly MJ, O’Donovan M. Genetic architectures of psychiatric disorders: the emerging picture and its implications. Nat Rev Genet. (2012) 13:537–51. doi: 10.1038/nrg3240
78. Cross-Disorder Group of the Psychiatric Genomics Consortium. Genetic relationship between five psychiatric disorders estimated from genome-wide SNPs. Nat Genet. (2013) 45:984–94.
79. Smoller JW, Craddock N, Kendler K, Lee PH, Neale BM, Nurnberger JI. Identification of risk loci with shared effects on five major psychiatric disorders: a genome-wide analysis. Lancet. (2013) 381:1371–9. doi: 10.1016/S0140-6736(12)62129-1
80. Lee PH, Anttila V, Won H, Feng YCA, Rosenthal J, Zhu Z, et al. Genomic relationships, novel loci, and pleiotropic mechanisms across eight psychiatric disorders. Cell. (2019) 179:1469–1482.e11. doi: 10.1016/j.cell.2019.11.020
81. Lu H, Qiao J, Shao Z, Wang T, Huang S, Zeng P. A comprehensive gene-centric pleiotropic association analysis for 14 psychiatric disorders with GWAS summary statistics. BMC Med. (2021) 19:314. doi: 10.1186/s12916-021-02186-z
82. Wang T, Lu H, Zeng P. Identifying pleiotropic genes for complex phenotypes with summary statistics from a perspective of composite null hypothesis testing. Brief Bioinform. (2022) 23:bbab389. doi: 10.1093/bib/bbab389
83. Saito J-I, Toriumi S, Awano K, Ichijo H, Sasaki K, Kobayashi T, et al. Regulation of apoptosis signal-regulating kinase 1 by protein phosphatase 2Cepsilon. Biochem J. (2007) 405:591–6. doi: 10.1042/BJ20070231
84. Hafner M, Landthaler M, Burger L, Khorshid M, Hausser J, Berninger P, et al. Transcriptome-wide identification of RNA-binding protein and microRNA target sites by PAR-CLIP. Cell. (2010) 141:129–41. doi: 10.1016/j.cell.2010.03.009
85. Wockner LF, Noble EP, Lawford BR, Young RM, Morris CP, Whitehall VLJ, et al. Genome-wide DNA methylation analysis of human brain tissue from schizophrenia patients. Transl Psychiatry. (2014) 4:e339. doi: 10.1038/tp.2013.111
86. Perez SM, Aguilar DD, Neary JL, Carless MA, Giuffrida A, Lodge DJ. Schizophrenia-like phenotype inherited by the F2 generation of a gestational disruption model of schizophrenia. Neuropsychopharmacology. (2016) 41:477–86. doi: 10.1038/npp.2015.169
87. Perez SM, Donegan JJ, Boley AM, Aguilar DD, Giuffrida A, Lodge DJ. Ventral hippocampal overexpression of cannabinoid receptor interacting protein 1 (CNRIP1) produces a schizophrenia-like phenotype in the rat. Schizophr Res. (2019) 206:263–70. doi: 10.1016/j.schres.2018.11.006
88. Zheng X, Suzuki T, Takahashi C, Nishida E, Kusakabe M. cnrip1 is a regulator of eye and neural development in Xenopus laevis. Genes Cells. (2015) 20:324–39. doi: 10.1111/gtc.12225
89. Rueda CB, Traba J, Amigo I, Llorente-Folch I, González-Sánchez P, Pardo B, et al. Mitochondrial ATP-Mg/Pi carrier SCaMC-3/Slc25a23 counteracts PARP-1-dependent fall in mitochondrial ATP caused by excitotoxic insults in neurons. J Neurosci. (2015) 35:3566–81. doi: 10.1523/jneurosci.2702-14.2015
90. Babenko VN, Smagin DA, Galyamina AG, Kovalenko IL, Kudryavtseva NN. Altered Slc25 family gene expression as markers of mitochondrial dysfunction in brain regions under experimental mixed anxiety/depression-like disorder. BMC Neurosci. (2018) 19:79. doi: 10.1186/s12868-018-0480-6
91. Le-Niculescu H, Kurian SM, Yehyawi N, Dike C, Patel SD, Edenberg HJ, et al. Identifying blood biomarkers for mood disorders using convergent functional genomics. Mol Psychiatry. (2009) 14:156–74. doi: 10.1038/mp.2008.11
92. Wang Q, Dwivedi Y. Transcriptional profiling of mitochondria associated genes in prefrontal cortex of subjects with major depressive disorder. World J Biol Psychiatry. (2017) 18:592–603. doi: 10.1080/15622975.2016.1197423
93. Xie P, Kranzler HR, Yang C, Zhao H, Farrer LA, Gelernter J. Genome-wide association study identifies new susceptibility loci for posttraumatic stress disorder. Biol Psychiatry. (2013) 74:656–63. doi: 10.1016/j.biopsych.2013.04.013
94. Fromer M, Roussos P, Sieberts SK, Johnson JS, Kavanagh DH, Perumal TM, et al. Gene expression elucidates functional impact of polygenic risk for schizophrenia. Nat Neurosci. (2016) 19:1442–53. doi: 10.1038/nn.4399
95. Hernandez LM, Kim M, Hoftman GD, Haney JR, de la Torre-Ubieta L, Pasaniuc B, et al. Transcriptomic insight into the polygenic mechanisms underlying psychiatric disorders. Biol Psychiatry. (2021) 89:54–64. doi: 10.1016/j.biopsych.2020.06.005
96. Le Quesne Stabej P, Saihan Z, Rangesh N, Steele-Stallard HB, Ambrose J, Coffey A, et al. Comprehensive sequence analysis of nine usher syndrome genes in the UK national collaborative usher study. J Med Genet. (2012) 49:27–36. doi: 10.1136/jmedgenet-2011-100468
97. Besse A, Wu P, Bruni F, Donti T, Graham BH, Craigen WJ, et al. The GABA transaminase, ABAT, is essential for mitochondrial nucleoside metabolism. Cell Metab. (2015) 21:417–27. doi: 10.1016/j.cmet.2015.02.008
98. Elliott DA, Weickert CS, Garner B. Apolipoproteins in the brain: implications for neurological and psychiatric disorders. Clin Lipidol. (2010) 5:555–73. doi: 10.2217/clp.10.37
99. Zhou Q, Zhao F, Lv Z-P, Zheng C-G, Zheng W-D, Sun L, et al. Association between APOC1 polymorphism and Alzheimer’s disease: a case-control study and meta-analysis. PLoS One. (2014) 9:e87017. doi: 10.1371/journal.pone.0087017
100. Smith AK, Ratanatharathorn A, Maihofer AX, Naviaux RK, Aiello AE, Amstadter AB, et al. Epigenome-wide meta-analysis of PTSD across 10 military and civilian cohorts identifies methylation changes in AHRR. Nat Commun. (2020) 11:5965. doi: 10.1038/s41467-020-19615-x
101. Alexandrov LB, Ju YS, Haase K, Van Loo P, Martincorena I, Nik-Zainal S, et al. Mutational signatures associated with tobacco smoking in human cancer. Science. (2016) 354:618–22. doi: 10.1126/science.aag0299
102. Braillon A. Recurrence in early-stage hepatocellular carcinoma: CpG methylation and smoking. J Clin Oncol. (2017) 35:2097–8. doi: 10.1200/jco.2017.72.3932
103. London SJ. Methylation, smoking, and reduced lung function. Eur Respir J. (2019) 54:1900920. doi: 10.1183/13993003.00920-2019
104. Reese SE, Zhao S, Wu MC, Joubert BR, Parr CL, Håberg SE, et al. DNA methylation score as a biomarker in newborns for sustained maternal smoking during pregnancy. Environ Health Perspect. (2017) 125:760–6. doi: 10.1289/ehp333
105. McCartney DL, Hillary RF, Stevenson AJ, Ritchie SJ, Walker RM, Zhang Q, et al. Epigenetic prediction of complex traits and death. Genome Biol. (2018) 19:136. doi: 10.1186/s13059-018-1514-1
106. Barbu MC, Shen X, Walker RM, Howard DM, Evans KL, Whalley HC, et al. Epigenetic prediction of major depressive disorder. Mol Psychiatry. (2021) 26:5112–23. doi: 10.1038/s41380-020-0808-3
Keywords: DNA methylation, divide-aggregate composite-null hypothesis test, gene-based mediation analysis, childhood maltreatment, psychiatric disorder
Citation: Xu H, Shao Z, Zhang S, Liu X and Zeng P (2023) How can childhood maltreatment affect post-traumatic stress disorder in adult: Results from a composite null hypothesis perspective of mediation analysis. Front. Psychiatry 14:1102811. doi: 10.3389/fpsyt.2023.1102811
Received: 19 November 2022; Accepted: 20 February 2023;
Published: 09 March 2023.
Edited by:
Hugo Schnack, Utrecht University, NetherlandsReviewed by:
Manqi Cai, University of Pittsburgh, United StatesCopyright © 2023 Xu, Shao, Zhang, Liu and Zeng. This is an open-access article distributed under the terms of the Creative Commons Attribution License (CC BY). The use, distribution or reproduction in other forums is permitted, provided the original author(s) and the copyright owner(s) are credited and that the original publication in this journal is cited, in accordance with accepted academic practice. No use, distribution or reproduction is permitted which does not comply with these terms.
*Correspondence: Haibo Xu, eGhiQHh6aG11LmVkdS5jbg==; Ping Zeng, enBzdGF0QHh6aG11LmVkdS5jbg==
†These authors have contributed equally to this work and share first authorship
Disclaimer: All claims expressed in this article are solely those of the authors and do not necessarily represent those of their affiliated organizations, or those of the publisher, the editors and the reviewers. Any product that may be evaluated in this article or claim that may be made by its manufacturer is not guaranteed or endorsed by the publisher.
Research integrity at Frontiers
Learn more about the work of our research integrity team to safeguard the quality of each article we publish.