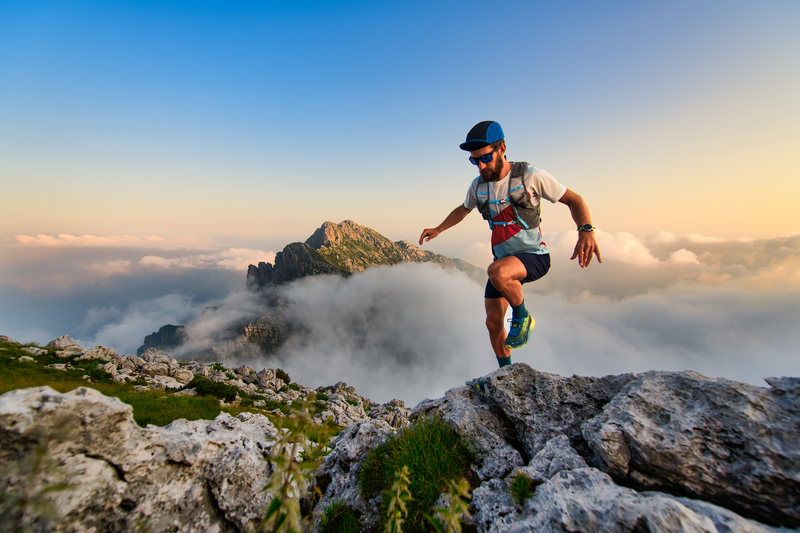
94% of researchers rate our articles as excellent or good
Learn more about the work of our research integrity team to safeguard the quality of each article we publish.
Find out more
ORIGINAL RESEARCH article
Front. Psychiatry , 05 August 2022
Sec. Autism
Volume 13 - 2022 | https://doi.org/10.3389/fpsyt.2022.959763
Individuals with sub-threshold autism spectrum disorder (ASD) are those who have social communication difficulties but do not meet the full ASD diagnostic criteria. ASD is associated with an atypical brain network; however, no studies have focused on sub-threshold ASD. Here, we used the graph approach to investigate alterations in the brain networks of children with sub-threshold ASD, independent of a clinical diagnosis. Graph theory is an effective approach for characterizing the properties of complex networks on a large scale. Forty-six children with ASD and 31 typically developing children were divided into three groups (i.e., ASD-Unlikely, ASD-Possible, and ASD-Probable groups) according to their Social Responsiveness Scale scores. We quantified magnetoencephalographic signals using a graph-theoretic index, the phase lag index, for every frequency band. Resultantly, the ASD-Probable group had significantly lower small-worldness (SW) in the delta, theta, and beta bands than the ASD-Unlikely group. Notably, the ASD-Possible group exhibited significantly higher SW than the ASD-Probable group and significantly lower SW than the ASD-Unlikely group in the delta band only. To our knowledge, this was the first report of the atypical brain network associated with sub-threshold ASD. Our findings indicate that magnetoencephalographic signals using graph theory may be useful in detecting sub-threshold ASD.
Autism spectrum disorder (ASD) is a neurodevelopmental disorder characterized by deficits in social cognition and communication and repetitive/restrictive behaviors (1). These autistic traits are distributed on a continuum from the typical developmental range to the diagnostic range (2). As a result, the wide variety of clinical presentations blurs the lines between autistic and typically developing children (3, 4). As one might infer, there are children who do not meet the full diagnostic criteria but still experience difficulties related to autistic traits. Since these problems can still significantly impair an individual’s daily activities, they are referred to as “sub-threshold ASD” (5). Evidence suggests that sub-threshold ASD tends to involve difficulty in reciprocal communication, which could result in maladjustment and increased risk of psychiatric disorders such as depression and anxiety (6). In fact, Moriwaki and Kamio (7) revealed that children with a higher level of autistic traits have a greater risk of additional mental health problems even if they do not meet the full diagnostic criteria of ASD (7). Therefore, early detection and appropriate support for children who have a certain level of autistic traits (i.e., sub-threshold ASD), but do not fulfill the diagnostic criteria of ASD, is necessary to prevent secondary disorders such as depression and anxiety. However, no reliable biological marker currently exists for detecting sub-threshold ASD.
Evidence suggests that ASD is a disorder of “brain connectivity.” In terms of pair-wise brain connectivity, a popular hypothesis is that ASD is characterized by long-range underconnectivity combined with local overconnectivity [in magnetoencephalography (MEG) or electroencephalography (EEG), connectivity usually refers to how electromagnetic signals from different brain regions are similar]. We know that interactions between pairs of regions combine to make a single, large network of the brain. In this context, a novel way to study the brain is to analyze the macroscopic behavior of the brain network as a whole instead of focusing on the respective pair-wise connectivity. Graph theory effectively describes the properties of complex networks on a large scale (8). Using graph theory, we can express geometric features of a given network in terms of numbers referred to as “graph metrics.” Among these, “small-worldness” (SW) is considered an index of optimal balance between integrated and segregated information processing in the brain network. SW has been well-reported for comparisons of brain networks of children with typical development (TD) and ASD, and these studies have almost consistently reported lower SW in children with ASD. Particularly, the brain network of children with ASD shows significantly lower SW than that of children with TD in the delta (9), theta combined with alpha (10), and beta (11) bands and every (i.e., delta, theta, alpha, beta, and gamma) frequency band (12) [one exception is a higher SW in the gamma band reported by (9)]. It is noteworthy that lower small worldness in the beta band is reported to linearly correlate with severer social impairment in children with ASD (11). Given the relation between SW and ASD symptomatology, one might infer that it would be possible to detect children with sub-threshold ASD in early developmental stages using SW. However, no graph-theoretical studies have focused on “sub-threshold ASD.”
For sub-threshold ASD, whether one fulfills the diagnostic criteria of ASD would be less meaningful, as the definition of sub-threshold ASD borderlines between ASD and typically developing children. As such, children with a similar degree of difficulty in communication could accidentally be diagnosed or not with ASD. In this study, we recruited children, with ASD and TD and classified them into three groups (ASD-Unlikely, ASD-Possible, and ASD-Probable) in terms of difficulties both in communication and repetitive restricted behaviors, rather than them being formally diagnosed with ASD. We combined MEG and graph theory to investigate the brain network of these three groups. We hypothesized that children in the ASD-Possible group would have atypical brain networks differing from those in the ASD-Unlikely or ASD-Probable group. Specifically, we hypothesized that there may be differences in SW in relation to the degree of autistic traits.
We enrolled 54 Japanese children with ASD (36 boys, 18 girls, aged 38–92 months) and 31 children with TD (26 boys, 5 girls, aged 53–89 months) from the Kanazawa University hospital and affiliated hospitals. ASD was diagnosed following the Diagnostic and Statistical Manual of Mental Disorders (DSM) IV (1) using the Diagnostic Interview for Social and Communication Disorders (13) or the Autism Diagnostic Observation Schedule-Generic (14) and the Autism Diagnostic Observation Schedule 2 (15). We excluded participants with blindness, deafness, other neuropsychiatric disorders, and those receiving an ongoing medication regimen. We assessed the intelligence of the participants using the Japanese version of the Kaufman Assessment Battery for Children (K-ABC) (16) and excluded eight participants who scored <70 on the Mental Processing Scale. Consequently, 46 children with ASD (31 boys, 15 girls, aged 38–92 months) and 31 with TD (26 boys, 5 girls, aged 53–89 months) remained for analysis. The Social Responsiveness Scale (SRS) (17) was used to assess the participants’ autistic traits (a detailed explanation of the SRS is available in the Supplementary Data). We separated the participants into three groups in terms of the T-score of the SRS according to a previous study (18); (i) ASD-Unlikely group (children in the normal range, T-scores less than 59), (ii) ASD-Possible group (children with less obvious autistic traits corresponding to sub-threshold ASD, T-scores between 60 and 75), and (iii) ASD-Probable group (children with obvious autistic traits, T-scores greater than 76). The study was approved by the Ethics Committee of Kanazawa University Hospital and conducted following the Declaration of Helsinki. Written informed consent was obtained from all parents.
Magnetoencephalography data were recorded using a 151-channel Superconducting Quantum Interference Device whole-head coaxial gradiometer MEG system for children (PQ 1151 R; Yokogawa/KIT, Kanazawa, Japan) in a magnetically shielded room (Daido Steel, Nagoya, Japan) installed at the MEG Center of Ricoh Company, Ltd. (Kanazawa, Japan). The band-pass-filtered MEG data (0.16–200 Hz) were collected at a sampling rate of 2,000 Hz. We ensured that each child was motionless during the recording (a detailed description of the recording procedure is available in the Supplementary Data).
A total of 25 participants were unable to remain motionless during the magnetic resonance image (MRI) recordings. We employed a suitable MRI brain template for each such participant based on the individual head surface shapes using an algorithm that we had developed for previous studies (see details in Supplementary Data). To use a brain template, we attached three coils at each bilateral mastoid process and the nasion. The other 21 children underwent complete MRI. For those, to use their own MRI image, we attached four coils at each of the bilateral mastoid processes, midline frontal, and vertex. In either case, in reference to the specific magnetic field generated by the coils, we could ascertain the child’s head position within the helmet. A detailed description of the procedure is available in Supplementary Data.
A newly developed silent mode MRI and a sequence allowed us to capture the participants’ brain structure in less than 30 s (19). Consequently, with the help of an MRI-compatible video presentation system and an MRI-compatible headphone system, we were able to conduct MRI without sedation for the other 21 children. The following methods for MR image acquisition have been described before (11). A 1.5-T MRI scanner (SIGNA Explorer; GE Healthcare, United States) was used to collect structural brain images from all participants. The three-dimensional high-resolution T1-weighted gradient-echo and Silenz pulse sequence images were used as an anatomical reference. The imaging parameters were as follows: TR = 435.68 ms, TE = 0.024 ms, flip angle = 7°, FOV = 220 mm, matrix size = 256 × 256 pixels, slice thickness = 1.7 mm, total of 130 transaxial images.
For these 21 participants, we co-registered the MEG and their own MR images following the marker locations. The markers for MEG and MRI were the frontal midline, parietal, and bilateral mastoid processes. For the MEG, we used four coils to generate a magnetic field. For MRI, we used four pieces of lipid capsule as markers. Furthermore, we identified points on the mastoid processes, nasion, and skull surface visually on MRI. Approximately 15–25 points were depicted for each participant.
The MEG analyses were performed using Brainstorm.1 We preprocessed the MEG data according to recommendations of the Organization for Human Brain Mapping (20). Then, we estimated the brain signal sources using an anatomically constrained MEG approach. The signal sources were grouped into 68 regions represented in the Desikan–Killiany atlas using principal component analyses. The data were segmented into 5-s epochs; then, each epoch was band-pass filtered for the used frequency bands: delta (2–4 Hz), theta (4–8 Hz), alpha (8–13 Hz), beta (13–30 Hz), and gamma (30–60 Hz). This procedure was identical to those used in our earlier studies (11, 21). The details regarding the procedure (i.e., preprocessing, atlas-guided source reconstruction, and segmenting) are available in the Supplementary Data.
The phase lag index (PLI) was used to measure the functional connectivity between brain regions (a detailed explanation is available in Supplementary Data). We chose the PLI as it is insensitive to the field spread and has been widely used to describe atypical brain networks in children with ASD (9–12).
The basic topography of a network is represented by a graph consisting of “nodes” and “edges” connecting pairs of nodes. In this study, nodes corresponded to 68 brain regions of the Desikan–Killiany atlas (22). We weighted the edges based on the PLI values between pairs of brain regions. For each epoch, we constructed an undirected weighted functional connectivity matrix (68 × 68) based on the PLI for each frequency band (i.e., delta, theta, alpha, beta, and gamma). We averaged the matrices of all epochs for each participant. For those averaged matrices, weak connectivity may represent spurious connections. To remove possible spurious connections, we binarized those graphs through the application of a proportional weight threshold. We set proportional threshold κ, the proportion of total connections retained, as 0.2 (a κ of 0.2 indicates that the strongest 20% of the connections were selected) in accordance with earlier studies (6, 8). At present, however, there is no formal consensus regarding the selection of a weight threshold. To verify the stability of the results, we also investigated a range of proportional thresholds κ from 0.1 to 0.3 at 0.02 increments.
In this manner, a complex brain network is expressed as graphs in terms of binary matrices. The information included in those graphs can be further summarized via various measures. Well-established measures include the mean clustering coefficient (C), average shortest path length (L), and SW. C represents how clustered a graph’s nodes are and is a commonly used measure of functional segregation. L is the average shortest path length between all node pairs in the network and is the most commonly used measure of functional integration. Notably, human brain networks have higher C and shorter L, which purportedly represents an optimal balance between integration and segregation. Such a network is called a small-world network. In this context, SW, another graph metric, is the ratio of normalized C and normalized L. As such, a graph with a high SW is a network significantly more clustered than a random network yet has approximately the same characteristic path length (a detailed explanation is available in Supplementary Data). In this study, we calculated each participant’s SW, C, and L on each frequency band. In addition to these network-level properties, we also investigated node-level properties to locate regions with atypical properties. In particular, the clustering coefficient and average shortest path length were calculated at each node.
All statistical analyses were performed using Stata (ver. 16.1; Stata Corp., College Station, TX, United States). We used Student’s t-test to compare differences in age and intelligence quotient (IQ: the Mental Processing Scale scores in the K-ABC) between children with ASD and those with TD. Sex differences were examined via a chi-square test.
We used analysis of variance to compare the three groups (ASD-Unlikely, ASD-Possible, and ASD-Probable groups) in terms of age, sex, scores in the Mental Processing Scale and the Achievement Scale of the K-ABC, and SRS total T-score).
Subsequently, to test the differences in network-level graph metrics (C, L, and SW) among the three groups, we fitted a generalized linear model with a gamma error distribution with a log link (i.e., a gamma regression model). Particularly, we predicted log-transformed graph metrics based on the groups (treated as a categorical variable), age, sex, and Mental Processing Scale of the K-ABC. As the test was repeated three times for each frequency band (ASD-Unlikely vs. ASD-Probable, ASD-Unlikely vs. ASD-Possible, ASD-Probable vs. ASD-Possible), adjusted p-values were obtained using a false discovery rate (FDR) approach (23) for three comparisons, and significance was inferred for adjusted p-values of <0.05.
To test the differences in node-level graph metrics (C and L) among the three groups, we similarly predicted log-transformed graph metrics based on the groups, age, sex, and Mental Processing Scale of the K-ABC. As the test was repeated 3 (ASD-Unlikely vs. ASD-Probable, ASD-Unlikely vs. ASD-Possible, ASD-Probable vs. ASD-Possible) * 68 (the number of nodes) = 204 times for each frequency band, FDR-adjusted p-values (23) were calculated for 204 comparisons, and significance was inferred for adjusted p-values of <0.05.
As our primary focus was SW, as exploratory analysis, we investigated linear relations between SW in each frequency band and the SRS scores. In particular, we predicted SW metrics based on the SRS total T-scores.
There were no significant differences in ages, sexes, and K-ABC scores between children with ASD and TD. The participant and group characteristics are shown in Tables 1, 2.
We first investigated the network-level properties in each frequency band setting κ at 0.2. Hereafter, adjusted p-values are presented because statistical significance was inferred based on the adjusted p-values.
Gamma regression models revealed that the ASD-Probable group had significantly lower SW than the ASD-Unlikely group in all frequency bands except for the alpha band. For the delta band, significant differences were observed in all three comparisons. In particular, the ASD-Probable group had significantly lower SW in the delta band than the ASD-Unlikely group (coefficient = −0.32, 95%CI: −0.49, −0.16, p = 0.0001); they also had a significantly lower SW than the ASD-Possible group (coefficient = −0.18, 95%CI: −0.34, −0.01, p = 0.044). In addition, the ASD-Possible group had a significantly lower SW in the delta band than the ASD-Unlikely group (coefficient = −0.15, 95%CI: −0.29, −0.00, p = 0.044). For the theta band, the ASD-Probable group had significantly lower SW than the ASD-Unlikely group (coefficient = −0.26, 95%CI: −0.43, −0.08, p = 0.011). For the beta band, the ASD-Probable group had significantly lower SW than the ASD-Unlikely group (coefficient = −0.25, 95%CI: −0.45, −0.06, p = 0.031). For the gamma band, the ASD-Probable group had an almost significantly lower SW than the ASD-Unlikely group (coefficient = −0.23, 95%CI: −0.41, −0.04, p = 0.05). On the other hand, there were no significant differences in C and L based on the groups. Only models predicting SW had a significant main effect on group. Figure 1 and Table 3 summarize the differences in graph metrics among the groups. FDR-corrected p-values are presented in Table 3. The effect of other variables (i.e., age, sex, and scores on the Mental Processing scale of the K-ABC) are provided in Supplementary Table 1.
Figure 1. Boxplot of small-worldness (SW) in each frequency band. The panels present the phase lag index values of SW among the three groups for each frequency band (mean ± SD). Post-hoc comparisons between the autism spectrum disorder (ASD)-Probable and ASD-Unlikely, ASD-Probable and ASD-Possible, and ASD-Possible and ASD-Unlikely groups: *p < 0.05, **p < 0.000.
Similar patterns were observed for the other κ values. The ASD-Unlikely group showed higher SW than the ASD-Probable group, and the values for the ASD-Possible group lied somewhere in between. The differences in SW between the ASD-Unlikely and ASD-Probable groups were significant for the delta, theta, and beta bands (the differences in the theta and delta bands were significant for every κ from 0.10 to 0.30, and the differences in the beta band were significant when κ was set at 0.10–0.22). Supplementary Figure 1 and Supplementary Table 2 present the relevant results.
We first investigated the node-level properties in each frequency band setting κ at 0.2. After multiple-testing correction, node-level C was significantly different in the nodes corresponding to the right fusiform, right superior parietal (in the alpha band), right supramarginal (in the theta band), and right transverse temporal (in the beta band) areas. These node-level significant differences were observed in the comparison between ASD-Possible and ASD-Probable individuals, except for the right superior parietal where the difference was significant between the ASD-Unlikely and ASD-Probable groups. These results highlight that the difference between the ASD-Unlikely and ASD-Possible groups could not have been detectable if we had only focused on the node-level properties.
Afterward, we investigated the node-level properties for the other κ values. Among the above regions, only the difference in the right transverse temporal (in the beta band) remained significant for the other κ values (when κ was set at 0.22–0.26). These results indicate that the difference in node-level properties per se could not explain the observed difference in network-level properties. Supplementary Table 3 presents the relevant results.
Subsequently, we investigated the relationship of SW with the SRS total T-scores using linear regression models. The regression models showed that lower SW in the delta, theta, beta, and gamma bands was significantly associated with higher SRS total T-scores (t(75) = −4.02, p = 0.0001, t(75) = −3.04, p = 0.003, t(75) = −2.57, p = 0.012, t(75) = −2.50, p = 0.015, respectively). The relevant results are presented in Supplementary Figure 2 and Supplementary Table 4.
To our knowledge, this is the first graph-theoretical study focusing on “sub-threshold ASD.” Our results revealed that each group—ASD-Unlikely, ASD-Possible, and ASD-Probable—as determined based on the SRS, has peculiar brain networks in terms of SW, a measure of optimal balance between functional integration and segregation. Particularly, the ASD-Probable group showed significantly lower SW than the ASD-Unlikely group in the delta, theta, and beta bands. These results suggest that the brain network of ASD-Probable individuals deviates from an unaffected brain’s small-world properties, and the brain network of ASD-Possible individuals falls somewhere in between those of the ASD-Probable and the ASD-Unlikely groups. The differences between the ASD-Possible group and the other two groups were significant even in the delta band.
Among the previous graph-theoretical studies of children with ASD, lower SW was almost consistently reported in various frequency bands [delta (9), theta combined with alpha (10), beta (11), and all frequency bands (12)]. We used the same connectivity measure (i.e., the PLI) as those studies (9–12) and confirmed the lower SW in ASD-Probable individuals. Considering the substantial methodological differences, such as the varying properties of the graphs [weighted (10, 12) vs. binary graphs (9, 11)], electromagnetic field measures [EEG (10, 12) vs. MEG (9, 11)], analytic spaces [sensor space (9, 10, 12) vs. source space (11)], and recording conditions [e.g., the presence of visual stimulation (9, 10), fixation cross (11), or natural environment (12)], it is surprising that lower SW is almost consistently reported. Notably, studies with relatively small sample sizes [i.e., the number of children with ASD vs. those with TD was 24 vs. 24 in (9), 12 vs. 19 in (10), and 20 vs. 25 in (11)] have reported inconsistent results across the frequency bands, whereas studies with larger sample sizes [80 vs. 106 in (12) and 46 vs. 31 in this study] have reported lower SWs for ASD/ASD-Probable children across the frequency bands. Combining the existing evidence, the ASD brain may be considered to exhibit lower SW in every frequency band, but the difference is not as large as those reported by studies with small sample sizes. In this context, our study’s results reinforce the notion that there is atypical information processing in the autistic brain and further support the usefulness and robustness of graph theory to describe the differences in network structures. In particular, using a combination of MEG/EEG and the graph theoretical approach, one would be able to effectively detect a characteristic of the ASD brain (e.g., lower SW in the delta band) regardless of some methodological differences (e.g., measures for the electromagnetic field and recording conditions).
This study was the first to show that an atypical brain network (i.e., lower SW in the delta band) is detectable even in individuals with less evident autistic traits (i.e., ASD-Possible individuals). It is noteworthy that the difference in node-level properties could not by itself explain this network-level difference. More importantly, we could not find a significant difference between the ASD-Possible and ASD-Unlikely groups in terms of node-level properties. These findings suggest that (i) the less evident autistic traits might not arise from atypical local information processing and may rather reflect atypical information processing at the network level and (ii) node-level properties might be insufficient to distinguish individuals with less evident autistic traits from the general population. In this sense, this study highlights the possible usefulness of graph theory for the detection of individuals with sub-threshold ASD, which could not be achieved using traditional approaches such as area by area comparisons. This particular application may be attractive from a clinical perspective because sub-threshold ASD is often overlooked, although the affected individuals tend to experience difficulties in social adaptation, and thus need support, as do children who meet the diagnostic criteria for ASD.
Finally, in the exploratory analysis of the linear relation between SW and the SRS scores, we found significant associations between these two metrics in all frequency bands except alpha. In this context, we reconfirmed the previously reported association for the beta band (11) and extended the previous findings in that the relation between SW and autistic symptoms could be observable in the other frequency bands.
This study had several limitations. First, the sample size was too small. Studies with a larger sample are needed to verify the neural bases of the brain networks in sub-threshold ASD. Second, all participants were young children. It is necessary to include adolescents and adult individuals with sub-threshold ASD to generalize the results. Third, the children remained motionless in the MEG system with the aid of visual attention (e.g., showing a video program). Because of this, the MEG data were recorded with eyes open under visual stimulation. As a result, the observed brain activity needs to be clearly distinguished from “resting” brain activity. Studies under controlled conditions of attention may provide more reliable evidence; however, these conditions would be difficult to apply to young children.
In conclusion, our results revealed varying atypical neural network properties according to the degree of autistic traits. Our findings highlight a theory of altered brain connectivity in ASD, which focuses on atypical SW network properties assessed using graph theory. Our results indicate that SW derived from graph theory, when applied to a MEG signal, may help characterize the neural basis of sub-threshold ASD.
The raw data supporting the conclusions of this article will be made available by the authors, without undue reservation.
This study was approved by the Ethics Committee of Kanazawa University Hospital and conducted following the Declaration of Helsinki. Written informed consent was obtained from the minor(s)’ legal guardian/next of kin for the publication of any potentially identifiable images or data included in this article.
YS: conceptualization and writing – original draft. YS and TH: methodology and project administration. YS, DS, TH, and MaK: software. YS, DS, and TH: formal analysis and data curation. YY, ST, CH, and KY: investigation. YY, ST, CH, KY, and SI: resources. YS, TH, and MiK: funding acquisition. MiK and SY: supervision. DS, TH, and MiK: writing – review and editing. All authors contributed to the article and approved the submitted version.
This study was supported by the Center of Innovation Program of the Japan Science and Technology Agency, JST, Grant-in-Aid for JSPS Fellows (Grant Number: 22J14602), and the Center of Developmental Education and Research Award.
We thank Satoshi Miyata and Tomoko Nishimura for supporting the statistical analysis. We are grateful to all study participants and our colleagues at the Bambi Plan for their support. We also thank Editage (www.editage.com) for English language editing.
The authors declare that the research was conducted in the absence of any commercial or financial relationships that could be construed as a potential conflict of interest.
All claims expressed in this article are solely those of the authors and do not necessarily represent those of their affiliated organizations, or those of the publisher, the editors and the reviewers. Any product that may be evaluated in this article, or claim that may be made by its manufacturer, is not guaranteed or endorsed by the publisher.
The Supplementary Material for this article can be found online at: https://www.frontiersin.org/articles/10.3389/fpsyt.2022.959763/full#supplementary-material
1. American Psychiatric Association. Diagnostic and Statistical Manual of Mental Disorders: DSM-IV-TR (4th ed.), Text Version. Washington, DC: American Psychiatric Association (2000).
2. Constantino JN, Todd RD. Autistic traits in the general population: a twin study. Arch Gen Psychiatry. (2003) 60:524–30. doi: 10.1001/archpsyc.60.5.524
3. Skuse DH, Mandy W, Steer C, Miller LL, Goodman R, Lawrence K, et al. Social communication competence and functional adaptation in a general population of children: preliminary evidence for sex-by-verbal IQ differential risk. J Am Acad Child Adolesc Psychiatry. (2009) 48:128–37. doi: 10.1097/CHI.0b013e31819176b8.37
4. Masi A, DeMayo MM, Glozier N, Guastella AJ. An overview of autism spectrum disorder, heterogeneity and treatment options. Neurosci Bull. (2017) 33:183. doi: 10.1007/s12264-017-0100-y.93
5. Gökçen E, Petrides KV, Hudry K, Frederickson N, Smillie LD. Sub-threshold autism traits: the role of trait emotional intelligence and cognitive flexibility. Br J Psychol. (2014) 105:187–99. doi: 10.1111/bjop.12033
6. Saito A, Stickley A, Haraguchi H, Takahashi H, Ishitobi M, Kamio Y. Association between autistic traits in preschool children and later emotional/behavioral outcomes. J Autism Dev Disord. (2017) 47:3333–46. doi: 10.1007/s10803-017-3245-7
7. Moriwaki A, Kamio Y. Associations between autistic traits and psychiatric issues and Japanese school children and adolescents. Japanese J Autistic Spectr. (2013) 10:11–7.
8. De Vico Fallani F, Richiardi J, Chavez M, Achard S. Graph analysis of functional brain networks: practical issues in translational neuroscience. Philos Trans R Soc Lond B Biol Sci. (2014) 369:20130521. doi: 10.1098/rstb.2013.0521
9. Takahashi T, Yamanishi T, Nobukawa S, Kasakawa S, Yoshimura Y, Hiraishi H, et al. Band-specific atypical functional connectivity pattern in childhood autism spectrum disorder. Clin Neurophysiol. (2017) 128:1457–65. doi: 10.1016/j.clinph.2017.05.010
10. Boersma M, Kemner C, de Reus MA, Collin G, Snijders TM, Hofman D, et al. Disrupted functional brain networks in autistic toddlers. Brain Connect. (2013) 3:41–9. doi: 10.1089/brain.2012.0127
11. Soma D, Hirosawa T, Hasegawa C, An KM, Kameya M, Hino S, et al. Atypical resting state functional neural network in children with autism spectrum disorder: graph theory approach. Front Psychiatry. (2021) 12:790234. doi: 10.3389/fpsyt.2021.790234
12. Han J, Zeng K, Kang J, Tong Z, Cai E, Chen H, et al. Development of brain network in children with autism from early childhood to late childhood. Neuroscience. (2017) 367:134–46. doi: 10.1016/j.neuroscience.2017.10.015
13. Wing L, Leekam SR, Libby SJ, Gould J, Larcombe M. The diagnostic interview for social and communication disorders: background, inter-rater reliability and clinical use. J Child Psychol Psychiatry. (2002) 43:307–25. doi: 10.1111/1469-7610.00023
14. Lord C, Risi S, Lambrecht L, Cook EH Jr., Leventhal BL, DiLavore PC, et al. The autism diagnostic observation schedule-generic: a standard measure of social and communication deficits associated with the spectrum of autism. J Autism Dev Disord. (2000) 30:205–23. doi: 10.1037/t17256-000
15. Lord C, Rutter M, DiLavore PC, Risi S, Gotham K, Bishop S. Autism Diagnostic Observation Schedule, Second Edition. Torrence: Western Psychological Services (2012).
16. Kaufman AS, Kaufman NL. Kaufman Assessment Battery for Children (K-ABC) Administration and Scoring Manual. Circle Pines: American Guidance Service (1983). doi: 10.1037/t27677-000
17. Constantino JN, Gruber CP. Social Responsiveness Scale: Manual. Los Angeles, CA: Western Psychological Services (2005).
18. Oka T, Ishikawa SI, Saito A, Maruo K, Stickley A, Watanabe N, et al. Changes in self-efficacy in Japanese school-age children with and without high autistic traits after the Universal Unified Prevention Program: a single-group pilot study. Child Adolesc Psychiatry Ment Health. (2021) 15:42. doi: 10.1186/s13034-021-00398-y
19. Huang MX, Mosher JC, Leahy RM. A sensor-weighted overlapping-sphere head model and exhaustive head model comparison for MEG. Phys Med Biol. (1999) 44:423–40. doi: 10.1088/0031-9155/44/2/010
20. Pernet C, Garrido MI, Gramfort A, Maurits N, Michel CM, Pang E, et al. Issues and recommendations from the OHBM COBIDAS MEEG committee for reproducible EEG and MEG research. Nat Neurosci. (2020) 23:1473–83. doi: 10.1038/s41593-020-00709-0
21. Hirosawa T, An KM, Soma D, Shiota Y, Sano M, Kameya M, et al. Epileptiform discharges relate to altered functional brain networks in autism spectrum disorders. Brain Commun. (2021) 3:fcab184. doi: 10.1093/braincomms/fcab184
22. Desikan RS, Ségonne F, Fischl B, Quinn BT, Dickerson BC, Blacker D, et al. An automated labeling system for subdividing the human cerebral cortex on MRI scans into gyral based regions of interest. Neuroimage. (2006) 31:968–80. doi: 10.1016/j.neuroimage.2006.01.021
Keywords: sub-threshold autism spectrum disorder, magnetoencephalography (MEG), social responsiveness scale (SRS), graph theory, small-worldness
Citation: Shiota Y, Soma D, Hirosawa T, Yoshimura Y, Tanaka S, Hasegawa C, Yaoi K, Iwasaki S, Kameya M, Yokoyama S and Kikuchi M (2022) Alterations in brain networks in children with sub-threshold autism spectrum disorder: A magnetoencephalography study. Front. Psychiatry 13:959763. doi: 10.3389/fpsyt.2022.959763
Received: 02 June 2022; Accepted: 15 July 2022;
Published: 05 August 2022.
Edited by:
Shaoqiang Han, First Affiliated Hospital of Zhengzhou University, ChinaReviewed by:
Masamichi Yokokura, Hamamatsu University School of Medicine, JapanCopyright © 2022 Shiota, Soma, Hirosawa, Yoshimura, Tanaka, Hasegawa, Yaoi, Iwasaki, Kameya, Yokoyama and Kikuchi. This is an open-access article distributed under the terms of the Creative Commons Attribution License (CC BY). The use, distribution or reproduction in other forums is permitted, provided the original author(s) and the copyright owner(s) are credited and that the original publication in this journal is cited, in accordance with accepted academic practice. No use, distribution or reproduction is permitted which does not comply with these terms.
*Correspondence: Tetsu Hirosawa, aGlyb3Nhd2F0ZXRzdTE5ODJAeWFob28uY28uanA=
†These authors have contributed equally to this work
Disclaimer: All claims expressed in this article are solely those of the authors and do not necessarily represent those of their affiliated organizations, or those of the publisher, the editors and the reviewers. Any product that may be evaluated in this article or claim that may be made by its manufacturer is not guaranteed or endorsed by the publisher.
Research integrity at Frontiers
Learn more about the work of our research integrity team to safeguard the quality of each article we publish.