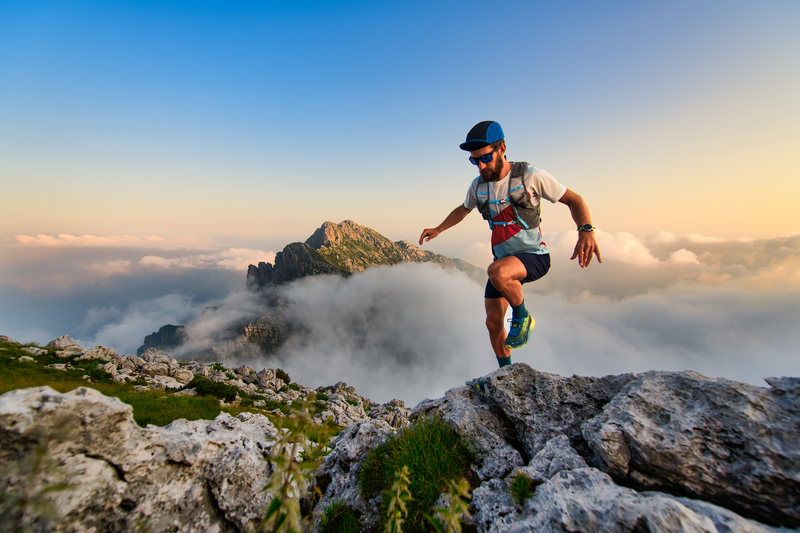
94% of researchers rate our articles as excellent or good
Learn more about the work of our research integrity team to safeguard the quality of each article we publish.
Find out more
ORIGINAL RESEARCH article
Front. Psychiatry , 28 June 2022
Sec. Public Mental Health
Volume 13 - 2022 | https://doi.org/10.3389/fpsyt.2022.919892
This article is part of the Research Topic Adaption to Change and Coping Strategies: New Resources for Mental Health View all 29 articles
Objective: Prior studies have shown that greenness can reduce the burden of depressive disorders. However, most were focused on local-scale analyses while limited evaluated globally. We aimed to investigate the association between greenness and the burden of depressive disorders using data from 183 countries worldwide.
Methods: We used the normalized difference vegetation index (NDVI) to estimate greenness. Country-level disability-adjusted life year (DALY) loss due to depressive disorders was used to represent depressive disorder burdens. A generalized linear mixed model was applied to assess the relationship between greenness and depressive disorders after controlling for covariates. Stratified analyses were conducted to determine the effects of greenness across several socio-demographic levels.
Results: The findings showed a significant negative association between greenness and the health burden of depressive disorders with a coefficient of −0.196 (95% CI: −0.356, −0.035) in the DALY changes per interquartile unit increment of NDVI. The stratified analyses suggested beneficial effects of greenness on depressive disorders across sex, various age groups especially for those aged <49 years, with low-income and/or those living in highly urbanized countries.
Conclusions: Our study noted that greenness exposure was significant negative association with the burden of depressive disorders. The findings should be viewed as recommendations for relevant authorities in supporting environmental greenness enhancement to reduce the mental burdens.
Mental illness is one of the most widespread and devastating global problems (1). The impact of mental illness is multiplied because of its ability to trigger other health burdens, such as chronic diseases (2). Even worse, mental illness on its own and in combination with other chronic health conditions is often considered a contributing factor to suicide (3). One of the most common and debilitating mental health burdens, on society is depressive disorders. According to the latest report from World Health Organization (WHO), at a global level, over 264 million people suffer from depressive disorders (4). According to the Global Burden of Disease (GBD) study in 2015, depressive disorders are the second leading causes of global age-specific disability-adjusted life years (DALY) for people aged 20–24 years with the change rate of 0.08 to 0.15 (%), the third for people aged 15–19 years with the change rate of 0.01–0.08 (%), and the fourth also fifth leading cause for people aged 25–44 years (5).
Over time, related issues in the economy, education, and wealth have made socioeconomic status an important focus leading to individual mental health problems, such as depression (6). Previous studies also have documented that the elevated extent of urbanization worldwide is one of the risk factors associated with mental illness. It is estimated that around 60% of the global population will live in cities by 2030 (7). This increasing urbanization will create cities that are more densely populated, further increasing the scarcity of the natural environment in these locations, and subsequently increasing the risk for mental burdens such as depression. The main scarcity of natural environments that often occurs is the limited amount of green space. Previous studies showed that limited green space can increase the concentration of air pollution, which in turn leaves populations more vulnerable to mental illness (8, 9). Numerous studies also reported how green environments could help reduce the burden of depressive disorders (10, 11). In relation to epidemiological studies, estimation of exposure to greenness by using remote sensing data such as normalized difference vegetation index (NDVI) is widely used (12–14). For example, by using multi-temporal NDVI data from MODIS, a study in Korea showed that exposure to greenness could alleviate depressive symptoms in in the seven major cities in Korea (15). Roe et al. also confirmed that exposure to greenness is associated with reductions in stress (16). Residential proximity to green space is often linked with reduced levels of disease burden from both physical and psychological conditions, such as diabetes, stroke, cardiovascular disease, and stress (17, 18). By considering that time spent in green space could possibly affect neural mechanisms, previous studies have also investigated the potential pathways by which exposure to greenness benefits mental health and vitality related to cognitive function (19, 20), as well as the extent to which differences in age and sex can influence these outcomes (21). Furthermore, the psycho-evolutionary theory or stress reduction theory, indicating frequent nature contact could evoke positive stimuli, which in turn promotes a reduction in physiological activation and blocks negative thoughts (22).
Although previous studies have investigated the positive effect of greenness on depressive disorders such as major depressive disorder and dysthymia, most were only focused on local-scale analyses while limited available studies have evaluated the benefits of greenness globally. Knowing that depressive disorders is a pioneer for global psychological burden, in this ecological study, we proposed a novel concept to investigate the association between greenness and the burden of depressive disorders in 183 countries worldwide. The main aimed was to respond to scientific gaps related to the relationship between green exposure and mental health burden, which is mostly only carried out in developed countries. By evaluating the association using multinational data, this study proposed to cover the shortfall in evaluating the relationship between greenness exposure and the burden of depression globally, which includes developed and developing countries. We hypothesized that exposure to greenness is inversely associated with the health burden due to depressive disorders globally. We also hypothesized that the beneficial effects of greenness on depressive disorders varies by age, sex, economic status, and urbanization level. Finally, for this study we focused on population-based proxies, therefore the related findings could serve as a baseline for global studies that support environmental development to improve the quality of health and wellbeing.
We used the data for disability-adjusted life years (DALY) loss to represent the country-level disease burden due to depressive disorders. This data was provided by the World Health Organization (WHO) from the Global Burden of Disease (GBD) study database. Country-level annual estimation data were available for the four follow-up years (2000, 2010, 2015, and 2016) (www.who.int/healthinfo/global_burden_disease/estimates/en/index1.html). This data is an estimated value and calculated from a combination of medical data, epidemiology, survey, and meta-regression modeling for each country. In brief, DALY is a summary metric of population health burden due to specific disease. It includes two components: years lived with disability (YLD) and years of life lost due to premature mortality (YLL). This data used a new normative standard life table to compute YLL and adjusted for comorbidity to calculate YLD (23). The DALY data is available for the entire population and is grouped by various factors, such as sex and age group (5–14, 15–29, 30–49, 50–59, 60–69, and ≥70 years). According to the database used, we defined depressive disorders using International Classification of Diseases 10th revision codes (F32–F33, F34.1) for non-communicable diseases. In total, 183 WHO member countries across five continents with available data on the health burden of depressive disorders were analyzed. Figure 1 shows the spatial distribution of DALY loss due to depressive disorders. The mental health burden due to depressive disorders was selected as the primary outcome of this study because of the high global burden due to depressive disorders and because previous studies showed that this health burden could trigger an increase in the risk of other health burdens (2, 3).
Figure 1. Spatial distribution of DALY loss due to depressive disorders across countries based on the average value from four study periods (2000, 2010, 2015, 2016).
Could cover all regions of the world, the Normalized Difference Vegetation Index (NDVI) measured by a Terra Moderate Resolution Imaging Spectroradiometer (Terra-MODIS) sensor with 1x1 km spatial resolution was used to estimate the presence of greenness in each country (24). The data provided by the National Aeronautics and Space Administration (NASA) includes the monitoring and measuring of vegetation, plants, and biomass production, as well as components of greenness including chlorophyll, canopy structure, and leaf (25). The monthly NDVI used in this study was MOD13A3 version 6 and for a given pixel has a range of values from −1.0 to +1.0. Positive values represent greener vegetation and negative values indicate limit vegetation (26). Since recent studies have indicated an association between negative NDVI values and the proximity to water (27), pixels with negative values were excluded to avoid misclassification bias due to the effects of water. In our analysis, satellite-images with an acquisition date closer to mid-season were collected for January, April, July, and October; the month settings for the data collection take into consideration countries with two and/or four seasons. In total, 292 MODIS NDVI images were used to assess the greenness of the global area covering the 183 selected countries. For image integration, we generated a monthly global greenness map by combining the 292 images. Next, we established similar procedures to assess greenness for the four selected months. Finally, monthly greenness concentrations were calculated to estimate the annual average values of greenness for each country.
In all, this process integrated a total of 4,672 images across the four follow-up years (2000, 2010, 2015, and 2016). The spatial distribution of greenness in each country based on the NDVI estimations is shown in Figure 2.
Figure 2. Spatial distribution of global greenness based on average of NDVI (crude) assessment from four study periods (2000, 2010, 2015, 2016).
Referring to previous literature, several potential variables assessed in each country were considered for model adjustments (Supplementary Table 1). We controlled for demographic covariates including population density, age, and sex provided by United Nations because these factors are often linked with depressive disorders (28, 29). The proportion/ percentage of the educated population on a country-level data obtained from World Bank Group was considered as a protective effect against depressive disorders (30). Because disparity in socioeconomic status is medical health care for each population (31–33), income level data from World Bank Group, as well as the proportion of urban areas in each country, were incorporated into the model. We also considered country-level healthcare expenditure data from World Bank Group (34). Further, sociocultural elements such as religion data provided by United Nations, were also taken into account because they play an important role in helping people adapt to physiological burdens (35). We examined the divorce rate data from United Nations as a social burden since it has been previously linked to depressive disorders (36, 37). Next, we treated blood pressure data from WHO and lifestyle behaviors including smoking and alcohol consumption as covariates obtained from World Bank Group, as suggested by previous studies (38–41). In this study, we also considered exposure to air pollution such as fine particulate matter (PM2.5) estimation with spatial resolution 1 × 1 km2 provided by The Atmosphere Composition Analysis Group, Dalhousie University (in 2000, 2010, 2015, 2016). This is because recent studies confirmed the negative effects of PM2.5 on mental health (42, 43). As a part of covariate adjusted in the association model, country-level PM2.5 data is a daily total column of aerosol optical depth retrievals from satellites that was coupled with the GEOS-Chem transport model and geographically weighted regression model (44). Finally, since a prior study confirmed the link between mental disorders and temperature (45), thus, this study also took into account this meteorological factor in the model adjustment.
Descriptive statistics were provided to present the country-level characteristics of all covariates examined in this study, including DALY loss of depressive disorders, environmental exposures (greenness and PM2.5), demographic factors (population density, sex, and age), economic status, the prevalence rate of education level, urbanization level, the prevalence rates of populations without religion, divorce rate, lifestyle behaviors (alcohol consumption and smoking), blood pressure, healthcare expenditure, and meteorological factors (i.e., temperature).
The main model with adjustment for the above-listed covariates was developed using the Generalized Linear Mixed Model (GLMM) with a penalized quasi-likelihood (PQL) algorithm to determine the relationship between exposure to greenness and the health burden of depressive disorders. The GLMMPQL accounts for both fixed and random effects and provides a flexible approach for analyzing health outcomes (46). In the case where spatial data are available from different areas, GLMMPQL can adjust the overall fixed effects while the structure of correlation is nested within areas, enabling the adjustment of regional differences in e.g., autocorrelation distances, and considering spatial autocorrelation only between observations in the same region (47). Given the values for DALY loss due to depressive disorders clustered in some countries, we added an additional term of “continent” as fixed effect in the GLMMPQL calculation to minimize the biases due to the spatial autocorrelation issue. Then, a Spatial Autocorrelation (Global Moran's I) was performed to examine whether a spatial autocorrelation (SAC) could be detected in the association model (46). By using residual estimation value from the main model, Moran's I Index and both z-score and p-value were calculated. An insignificant Moran's I with an index close to 0 and p > 0.05 supports the evidence that there is no explicit spatial autocorrelation problem in the developed model. Furthermore, generalized variance-inflation factors (GVIFs) were applied to examine the multicollinearity problem across covariates (48, 49). The GVIFs value was < 4 for all covariates, thus all variables were included in our model adjustment (Supplementary Table 2).
To evaluate the robustness of our main association model, a sensitivity test was applied. We included different covariates in six separate models adjusted by population density, age, sex, and year in order to discern the change in coefficient estimation and significance. Specifically, Model 1 only includes greenness exposure; Model 2 includes exposure to greenness and PM2.5 exposure; Model 3 adds education and economic status in addition to exposure to greenness and PM2.5 exposure; Model 4 adds urbanization level and the prevalence rate of the population without religion; Model 5 adds behavior factors; and Model 6 considered blood pressure as a risk factor and divorce rates for each country. In this sensitivity analysis, we assumed that there is no significant change in the estimation coefficient or the significance indicator (p-value) which indicates the robustness of the main relationship model.
Subsequently, this study conducted stratified analysis based on the level of greenness and socio-demographic factors. First, by using quartile of NDVI (Q1–Q4), the association between greenness in different exposure levels and the burden of depressive disorders were examined. In this case, Q1 represented countries with lower exposure to greenness (as reference), and Q4 represented countries with higher exposure to greenness, quartile 1 (NDVI: 0.085–0.389); quartile 2 (NDVI: 0.390–0.524), quartile 3 (NDVI: 0.525–0.622), and quartile 4 (NDVI: 0.623–0.808). This stratified analysis was considered, given that not all countries have the same green exposure. By completing this analysis, it can be seen whether there is a difference in the effect of the level of green exposure on the outcome. Second, the association between greenness and depressive disorder burdens was examined in different level of socio-demographic factors (50). In this part, we stratified the data by sexes (male and female), six age groups (5–14, 15–29, 30–49, 50–59, 60–69, and ≥70 years), economic statuses (low-income, middle-income, and high-income countries), and urbanization level (low-urban, middle-urban, and high-urban countries).
As a part of validity analysis, positive-negative control variables were used to check the strength of a causal inference of an exposure-outcome association when unobserved factors were thought to be present. Two approaches were used in this study, including a positive-negative outcome control and a positive-negative exposure control. A positive-negative outcome control aimed to identify whether using the same exposure (greenness) and replacing health burden (i.e., DALY loss due to depressive disorders) with other disease burdens from the same dataset could yield consistent results. For positive outcome control, we evaluated the linkage between greenness and the burden of disease due to cardiovascular diseases and for negative outcome control we evaluated Human Immunodeficiency Virus or HIV. Cardiovascular diseases were chosen as a positive outcome control because Yeager's study showed that greenness has a beneficial impact on reducing cardiovascular risk (51). HIV was chosen as a negative outcome control since no studies focused on this issue. In contrast, in the positive-negative exposure control analysis, this study identified whether using studied health burden (i.e., depressive disorders) and replacing greenness exposure could generate a consistent finding. For the positive exposure control, we examined the association between PM2.5 exposure and burden of IHD. A prior study confirmed that PM2.5 was correlated with an increased risk of depressive disorder burdens (52). Then, wind speed was used for the negative exposure control with the assumption that no association between wind speed and depressive disorder burdens.
All of the spatial and statistical analyses were performed using ArcGIS 10.7.1 (Esri Inc., Redlands, California, United States) and R version 3.6.3 (The R packages Foundation for Statistical Computing, Vienna, Austria). Coefficient estimates with 95% confidence intervals (CI) were reported and p < 0.05 were considered statistically significant. Further, to avoid false discovery rate for independent test statistics, adjusted p-value (Adj. p-value) was then calculated using the more powerful adjustment method proposed by Benjamini and Hochberg (53) and Jafari and Ansari-Pour (54).
Table 1 presents the descriptive statistics of each variable examined in this study. The average global burden due to depressive disorders was 5.62 years [standard deviation (SD): 1.44 years] for the four study periods (2000, 2010, 2015, and 2016). The average amount of greenness—NDVI was 0.49 (SD: 0.21) and estimated per interquartile (IQR = 0.309), the average value was 1.59 (SD: 1.37); average value of PM2.5 exposure was 19.45 μg/m3 (SD: 16.10 μg/m3); the population density of all selected countries was about 163 population per km2 (mean ± SD: 163.37 ± 722.47 persons); and almost 50.0% of the global population measured was female.
Table 1. Descriptive statistics of variables examined in this study, (A) continuous variables and (B) categorical variables.
A significant negative association between global greenness and depressive disorder burdens were observed (Table 2), with a coefficient of the main model was −0.635 (95% CI: −1.155, −0.115) for the DALY changes per increase in NDVI from 0 to 1 or coefficient estimate was −0.193 (95% CI: −0.356, −0.035) in the DALY changes per interquartile unit increment of NDVI (IQR = 0.309). This finding indicates greenness may have a beneficial effect in dealing with the health burden due to depressive disorders. We consistently found significant negative associations between greenness and health outcome from the first six sensitivity test models.
Table 2. Association models between greenness and depressive disorders in the DALY changes per unit increment of NDVI, supported by stratified analysis.
In a detailed assessment, the coefficient estimates for all associated risk factors adjusted in the models were presented in Supplementary Table 3. In the main model for the relationship between greenness and DALY loss due to depressive disorders, several variables other than greenness were identified as significant, such as sex (female) and divorce rate with estimated coefficients (95% CI) were 3.253 and 0.264, respectively. Moreover, after controlled for all risk factors, the findings also suggested that greenness had a significant negative relationship with the health burden due to depressive disorders in countries with the highest exposure to greenness (quartile 4); coefficient estimate was −0.510 (95% CI: −1.017, −0.003), compared to those with the lowest exposure (quartile 1) (Table 3). We also assessed the spatial-autocorrelation effects in the model. As shown in Supplementary Table 4, no statistically significant clustering effects (p > 0.05) were found in the developed models.
Table 3. Coefficient estimations of greenness by quartile attributed to depressive disorders in multivariable adjusted models.
After adjusting for potential risk factors, the stratified analyses by sex, age group, economic status, and urbanization level were assessed (Figure 3). We found a significant beneficial effect of greenness on depressive disorders for both females and males with coefficient estimate were −0.109 and −0.104 (95% CI: −0.213, −0.005 and 95% CI: −0.178, −0.003), respectively, in the DALY changes per interquartile unit increment of NDVI. This finding suggesting no sex-based inequality in relation to the impact of greenness on depressive disorder burdens. We found that the burden due to depressive disorders had a negative association with greenness in several age groups, especially the group aged 5–49 years rose to significance. The results from the stratified analysis also showed that significant negative associations of greenness with the health burden of depressive disorders were found in low-income countries and countries with high urbanization levels, coefficient estimate was −0.216 and −0.394 (95% CI), respectively, in the DALY changes per interquartile increment of NDVI.
Figure 3. Stratified analysis by sex, age groups, economic status, and urbanization levels in relation to depressive disorders in the DALY changes based on (A) crude NDVI; increase in NDVI from 0 to 1, (B) interquartile increment unit of NDVI (IQR = 0.309).
The results of the associations between positive or negative exposures and depressive disorder burdens were shown in Supplementary Table 5. First, a significant positive association was observed between fine particulate matter (PM2.5), a positive exposure control, and depressive disorders. Second, the relationship between the health burden of depressive disorders and wind speed, a negative exposure control, was not significant. We also examined associations between exposure to greenness and positive or negative outcomes. A significant relationship between greenness and cardiovascular diseases, a positive outcome control, was found, whereas a non-significant relationship between greenness and HIV, a negative outcome control, was also observed.
To our knowledge, this is the first global ecological study to investigate the association between greenness and the health burden of depressive disorders across multiple countries. Consistent with our hypotheses, our findings showed a significant negative association between greenness and depressive disorder burdens. In various sensitivity analyses, we consistently obtained similar results and supportive evidence that the higher the greenness exposure, the lower the health burden due to depressive disorders. The significant negative association of exposure to greenness with the burden of depressive disorders remained after accounting for potential country-level risk factors.
Our findings were reinforced by previous local studies. For example, Sarkar et al. observed a protective effect of greenness on depressive disorders, with 4.0% lower odds of major depressive disorder per interquartile increment in NDVI (odds ratio 0.96, 95% CI 0.93–0.99; p = 0.004) (50). In America, a study by Bezold et al. also reported that there was a 6% lower incidence rate of high depressive symptoms related to an interquartile range increase in greenness exposure (55). Taking children as the subject, a cohort study in the United States showed that increased exposure to greenness represented by NDVI was associated with a reduced risk of mental disorders such as depression (56). Moreover, the benefit of living in a green environment on mental health related to a lower incidence of depression was also found in South Africa (57). Applying the waitlist-control randomized controlled trial design, a study in Singapore that evaluated the effects of horticultural therapy on Asian adults showed the effectiveness of this therapy in improving mental wellbeing (58). Further, Takayama's study also reported that both people with and without depressive tendencies, forest bathing have a significant positive effect on improving profile of mood states (59). Supportive findings regarding how greenness exposure is associated with a lower risk of depression were also demonstrated by other previous studies (60–68). The beneficial impact of greenness on reduction in the health burden was also identified for physical health diseases such as cardiovascular mortality (51); malignant neoplasms (69, 70); diabetes mellitus (71, 72); respiratory diseases (73, 74); and on the body's metabolic system (75). In general, this study differed from those previous investigations, where the health burden of depressive disorder was represented by the DALY loss metric (considering year loss due to morbidity and mortality) rather than incident or prevalence rate data.
How the presence of greenness could have a significant negative association with the burden of depressive disorders was explained by several plausible reasons. Ulrich (76) emphasized that there are four pieces of evidence indicating the benefits of greenness on health, including (1) a sense of control: green space could provide a sense of control by allowing people a temporary means of physical or mental escape from a stressful conditions; (2) social support: how green space is used to increase social support; (3) physical movement and exercise; and (4) natural positive distractions in mitigating stress, pain, and frightening. In 2008, Ulrich et al. also reported relationships between environmental interventions and health outcomes in two main categories—reduce pain dan reduce stress (77). Furthermore, beneficial pathways of greenness on mental condition confirmed by Kaplan and Kaplan (78) who introduced attention restoration theory (ART). They stated that certain environments including nature are particularly effective at fostering recovery and dealing with fatigue.
We did not find a sex difference in the association between greenness and the burden of depressive disorders. In support of our findings, a systematic review by Bolte et al. showed that no consistent sex differences have been shown related to the impact of greenness on health (79). We did find that the association between greenness and the health burden of depressive disorders extended across various age groups and was significant particularly in those aged 5–49 years. Our findings are supported by several studies suggesting that greenness can reduce the risk of mental health burden among children and adults (80–90), even during pregnancy (91). Contrary to the previous findings on the impact of greenness on the elderly population (13, 92), we did not find significant association effects after age 49 years. Reasonable explanations may be due to changes in socioeconomic roles and decreased mobility to access green spaces at this age, as has also been suggested by Gilroy (93). In the subsequent analyses, we found a significant negative association between exposure to greenness and depressive disorder burdens in low-income and highly urbanized countries, respectively. Similarly, Tomita et al. also proposed the benefits of greenness for mental wellbeing in sub-Saharan Africa which has been experiencing rapid urbanization and economic transition (57). Moreover, Hoffimann's study also confirmed that exposure to greenness has the potential to mitigate health inequalities associated with socioeconomic deprivation (94).
Several strengths were noted in this study. This is the first global ecological study to investigate the association between greenness and the health burden of depressive disorders across 183 countries worldwide. Thus, our findings can serve as a global baseline for future environmental development research studies. Our methodological considerations were sound and included the use of various spatial-statistical approaches to deal with the spatial autocorrelation issue that may be caused by clustering patterns. Moreover, we adjusted our analytical models for several potential confounders with influence on the effectiveness of greenness in minimizing the burden of depressive disorders.
Some limitations also need to be acknowledged. Recognizing that the DALY data provided by WHO is only available at the country-level, not available at the state and/or city levels. A distinct analysis for big countries within-country variability cannot be done. Coming studies could examine the effects of greenness benefits at local communities in the case a finer-resolution DALY data available. In addition, the health burden data represented by DALY obtained from the Global Burden of Disease (GBD) study is multinational and multitemporal data that can be updated. Knowing this issue, considering the latest DALY data is recommended. Although we used greenness data for the same period as the main outcome, due to the limited accessibility of vegetation species data for each country, we did not adjust for the effects of biodiversity in the models. We only used NDVI value to represent total greenness exposure and assumed that all types of greenery had a positive impact on health. In the case of land use or land cover data showing the diversity of greenness is available on a global scale or other green index derived from satellite could estimate spatial greenness exposure, perhaps this could be a better consideration for future studies. Some related factors are unavailable in the global datasets, such as genetic or hereditary disease, health care quality index and ethnicity and/ or race. It will be important to explore the effects of these important factors on depressive disorders in future studies. This study also used an ecological study design, and the measure of exposure was only proxy-based on the average of the whole population. We recognize that the lack of individual data might have some impact on the strength of evidence provided by this study. Finally, the results of this global analysis cannot be directly compared to observational studies and the results should be interpreted with caution.
Our findings demonstrate a significant negative association between exposure to greenness and the burden of depressive disorders, particularly in low-income and high-urbanized countries. This study is the first to provide evidence for the link between greenness and depressive disorder burdens on a global scale. The findings from this study should serve as a call to policymakers and communities to deploy environmental interventions in terms of helping to deal with the global health burden of depressive disorders, with the potential for positive repercussions for the world.
The original contributions presented in the study are included in the article/Supplementary Material, further inquiries can be directed to the corresponding author.
AKA, S-CCL, H-JS, C-DW, and JDS: conceptualization. AA, H-JT, W-CP, YLG, and C-DW: methodology. AKA and W-CP: formal analysis. AKA, H-JT, and C-DW: writing—original draft preparation. AKA, H-JT, W-CP, YLG, C-PY, C-SW, H-JS, S-CCL, C-DW, and JDS: writing—review and editing. C-DW, S-CCL, and H-JS: supervision and funding acquisition. All authors contributed to the article and approved the submitted version.
This study was funded by Academia Sinica, Taiwan, under Trans-disciplinary PM2.5 Exposure Research in Urban Areas for Health-oriented Preventive Strategies (II) (Project No.: AS-SS-110-02).
The authors declare that the research was conducted in the absence of any commercial or financial relationships that could be construed as a potential conflict of interest.
All claims expressed in this article are solely those of the authors and do not necessarily represent those of their affiliated organizations, or those of the publisher, the editors and the reviewers. Any product that may be evaluated in this article, or claim that may be made by its manufacturer, is not guaranteed or endorsed by the publisher.
We would like to acknowledge the funding support from Academia Sinica, Taiwan, under “Trans-disciplinary PM2.5 Exposure Research in Urban Areas for Health-oriented Preventive Strategies (II)”. This study was then supported by the World Health Organization (WHO), which provided the metrics, i.e. disability-adjusted life years (DALY) database to estimate the global health burden of depressive disorders; the National Aeronautics and Space Administration (NASA), which provided the global greenness – NDVI data (MOD13A3); the Atmospheric Composition Analysis Group, which provided global PM2.5 data; the United Nations Agency, which provided demographic data; and the World Bank Group, which provided the provisions of economic status, the prevalence rate of smoking, alcohol consumption and risk factor data at a country-level. We thank Tami R. Bartell at Mary Ann & J. Milburn Smith Child Health Research, Outreach, and Advocacy Center, Stanley Manne Children's Research Institute, Ann & Robert H. Lurie Children's Hospital of Chicago for English editing.
The Supplementary Material for this article can be found online at: https://www.frontiersin.org/articles/10.3389/fpsyt.2022.919892/full#supplementary-material
1. WHO. Depression and Other Common Mental Disorders: Global Health Estimates. Geneva: World Health Organization (2017). Retrieved from WHO/MSD/MER/2017.2
2. Piane GM, Smith TC. Building an evidence base for the co-occurrence of chronic disease and psychiatric distress and impairment. Prev Chronic Dis. (2014) 11:188. doi: 10.5888/pcd11.140211
3. Brådvik L. Suicide risk and mental disorders. Int J Environ Res Public Health. (2018) 15:2028. doi: 10.3390/ijerph15092028
4. WHO. Newsroom: Depression. World Health Organization (2020). Retrieved from https://www.who.int/news-room/fact-sheets/detail/depression (accessed October 12, 2020).
5. Kassebaum NJ, Arora M, Barber RM, Bhutta ZA, Brown J, Carter A, et al. Global, regional, and national disability-adjusted life-years (DALYs) for 315 diseases and injuries and healthy life expectancy (HALE), 1990–2015: a systematic analysis for the global burden of disease study (2015). Lancet. (2016) 388:1603–58. doi: 10.1016/S0140-6736(16)31460-X
6. Krieger N, Williams DR, Moss NE. Measuring social class in US public health research: concepts, methodologies, and guidelines. Annu Rev Public Health. (1997) 18:341–78. doi: 10.1146/annurev.publhealth.18.1.341
7. United Nations. World Population Prospects: The 2017 Revision. Population Divisions, United Nations (2016). Retrieved from https://population.un.org/wpp/
8. Kruizse H, van der Vliet N, Staatsen B, Bell R, Chiabai A, Muiños G, et al. Urban green space: creating a triple win for environmental sustainability, health, and health equity through behavior change. Int J Environ Res Public Health. (2019) 16:4403. doi: 10.3390/ijerph16224403
9. van den Berg AE, Maas J, Verheij RA, Groenewegen PP. Green space as a buffer between stressful life events and health. Soc Sci Med. (2010) 70:1203–10. doi: 10.1016/j.socscimed.2010.01.002
10. Beyer KMM, Kaltenbach A, Szabo A, Bogar S, Nieto FJ, Malecki KM. Exposure to neighborhood green space and mental health: evidence from the survey of the health of Wisconsin. Int J Environ Res Public Health. (2014) 11:3453–72. doi: 10.3390/ijerph110303453
11. Kuo M. How might contact with nature promote human health? Promising mechanisms and a possible central pathway. Front Psychol. (2015) 6:1093. doi: 10.3389/fpsyg.2015.01093
12. Asri AK, Yu CP, Pan WC, Guo YL, Su HJ, Lung SC, et al. Global greenness in relation to reducing the burden of cardiovascular diseases: ischemic heart disease and stroke. Environ Res Lett. (2020) 15:124003. doi: 10.1088/1748-9326/abbbaf
13. Banay RF, James P, Hart JE, Kubzansky LD, Spiegelman D, Okereke OI, et al. Greenness and depression incidence among older women. Environ Health Perspect. (2019) 127:27001. doi: 10.1289/EHP1229
14. Kim S, Kim H, Lee JT. Interactions between ambient air particles and greenness on cause-specific mortality in seven Korean metropolitan cities, 2008-2016. Int J Environ Res Public Health. (2019) 16:1866. doi: 10.3390/ijerph16101866
15. Song H, Lane KJ, Kim H, Kim H, Byun G, Le M, et al. Association between urban greenness and depressive symptoms: evaluation of greenness using various indicators. Int J Environ Res Public Health. (2019) 16:173. doi: 10.3390/ijerph16020173
16. Roe JJ, Thompson WC, Aspinall AP, Brewer JM, Duff IE, Miller D, et al. Green space and stress: evidence from cortisol measures in deprived urban communities. Int J Environ Res Public Health. (2013) 10:4086–103. doi: 10.3390/ijerph10094086
17. Dadvand P, Bartoll X, Basagaña X, Dalmau-Bueno A, Martinez D, Ambros A, et al. Green spaces and general health: roles of mental health status, social support, physical activity. Environ Int. (2016) 91:161–7. doi: 10.1016/j.envint.2016.02.029
18. James P, Banay RF, Hart JE, Laden F. A review of the health benefits of greenness. Curr Epidemiol Rep. (2015) 2:131–42. doi: 10.1007/s40471-015-0043-7
19. Berman MG, Kross E, Krpan KM, Askren MK, Burson A, Deldin PJ, et al. Interacting with nature improves cognition and affect for individuals with depression. J Affect Disord. (2012) 140:300–5. doi: 10.1016/j.jad.2012.03.012
20. Bratman GN, Hamilton JP, Daily GC. The impacts of nature experience on human cognitive function and mental health. Ann N Y Acad Sci. (2012) 1249:118–36. doi: 10.1111/j.1749-6632.2011.06400.x
21. van den berg M, Van Poppel M, Van Kamp I, Andrusaityte S, Balseviciene B, Cirach M, et al. Visiting green space is associated with mental health and vitality: a cross-sectional study in four European cities. Health Place. (2016) 38:8–15. doi: 10.1016/j.healthplace.2016.01.003
22. Markevych I, Schoierer J, Hartig T, Chudnovsky A, Hystad P, Dzhambov AM, et al. Exploring pathways linking greenspace to health: theoretical and methodological guidance. Environ Res. (2017) 158:301–17. doi: 10.1016/j.envres.2017.06.028
23. WHO. WHO Methods and Data Sources for Global Burden of Disease Estimates 2000-2016: Global Health estimates Technical Paper WHO/HIS/IER/GEH/2018.4. Geneva: WHO (2018).
24. NASA. Find Data—LAADS DAAC. National Aeronautics and Space Administration (2018). Retrieved from https://ladsweb.modaps.eosdis.nasa.gov/search/order/1/MOD11A1–6
25. Gascon M, Cirach M, Martínez D, Dadvand P, Valentín A, Plasència A, et al. Normalized difference vegetation index (NDVI) as a marker of surrounding greenness in epidemiological studies: the case of Barcelona city. Urban For Urban Green. (2016) 19:88–94. doi: 10.1016/j.ufug.2016.07.001
26. Wu CD, Chen YC, Pan WC, Zeng YT, Chen MJ, Guo YL, et al. Land-use regression with long-term satellite-based greenness index and culture-specific sources to model PM2.5 spatial-temporal variability. Environ Pollut. (2017) 224:148–57. doi: 10.1016/j.envpol.2017.01.074
27. Wheeler BW, White M, Stahl-Timmins W, Depledge MH. Does living by the coast improve health and wellbeing? Health Place. (2012) 18:1198–201. doi: 10.1016/j.healthplace.2012.06.015
28. Ferrari AJ, Charlson FJ, Norman RE, Patten SB, Freedman G, Murray CJL, et al. Burden of depressive disorders by country, sex, age, and year: findings from the global burden of disease study 2010. PLOS Med. (2013) 10:e1001547. doi: 10.1371/journal.pmed.1001547
29. Kessler RC, Bromet EJ. The epidemiology of depression across cultures. Annu Rev Public Health. (2013) 34:119–38. doi: 10.1146/annurev-publhealth-031912-114409
30. Bauldry S. Variation in the protective effect of higher education against depression. Soc Ment Health. (2015) 5:145–61. doi: 10.1177/2156869314564399
31. Freeman A, Tyrovolas S, Koyanagi A, Chatterji S, Leonardi M, Ayuso-Mateos JL, et al. The role of socio-economic status in depression: results from the COURAGE (aging survey in Europe). BMC Public Health. (2016) 16:1098. doi: 10.1186/s12889-016-3638-0
32. Lorant V, Deliège D, Eaton W, Robert A, Philippot P, Ansseau M. Socioeconomic inequalities in depression: a meta-analysis. Am J Epidemiol. (2003) 157:98–112. doi: 10.1093/aje/kwf182
33. Sampson L, Ettman CK, Galea S. Urbanization, urbanicity, and depression: a review of the recent global literature. Curr Opin Psychiatry. (2020) 33:233–44. doi: 10.1097/YCO.0000000000000588
34. Moitra M, Santomauro D, Collins PY, Vos T, Whiteford H, Saxena S, et al. The global gap in treatment coverage for major depressive disorder in 84 countries from 2000–2019: a systematic review and Bayesian meta-regression analysis. PLOS Med. (2022) 19:e1003901. doi: 10.1371/journal.pmed.1003901
35. Bonelli R, Dew RE, Koenig HG, Rosmarin DH, Vasegh S. Religious and spiritual factors in depression: review and integration of the research. Depress Res Treat. (2012) 2012:962860. doi: 10.1155/2012/962860
36. Li Z, Wei A, Palanivel V, Jackson JC. A data-driven analysis of sociocultural, ecological, and economic correlates of depression across nations. J Cross Cult Psychol. (2021) 52:822–43. doi: 10.1177/00220221211040243
37. Vousoura E, Verdeli H, Warner V, Wickramaratne P, Baily CDR. Parental divorce, familial risk for depression, and psychopathology in offspring: a three-generation study. J Child Fam Stud. (2012) 21:718–25. doi: 10.1007/s10826-011-9523-7
38. Abdoli N, Salari N, Darvishi N, Jafarpour S, Solaymani M, Mohammadi M, et al. The global prevalence of major depressive disorder (MDD) among the elderly: a systematic review and meta-analysis. Neurosci Biobehav Rev. (2022) 132:1067–73. doi: 10.1016/j.neubiorev.2021.10.041
39. Hildrum B, Mykletun A, Stordal E, Bjelland I, Dahl AA, Holmen J. Association of low blood pressure with anxiety and depression: the nord-trøndelag health study. J Epidemiol Community Health. (2007) 61:53–8. doi: 10.1136/jech.2005.044966
40. Kuria MW, Ndetei DM, Obot IS, Khasakhala LI, Bagaka BM, Mbugua MN, et al. The association between alcohol dependence and depression before and after treatment for alcohol dependence. ISRN Psychiatry. (2012) 2012:482802. doi: 10.5402/2012/482802
41. Morse DE, Psoter WJ, Baek LS, Eisenberg E, Cohen D, Cleveland D, et al. Smoking and drinking in relation to depressive symptoms among persons with oral cancer or oral epithelial dysplasia. Head Neck. (2010) 32:578–87. doi: 10.1002/hed.21227
42. Ali NA, Khoja A. Growing evidence for the impact of air pollution on depression. Ochsner J. (2019) 19:4. doi: 10.31486/toj.19.0011
43. Vert C, Sánchez-Benavides G, Martinez D, Gotsens X, Gramunt N, Cirach M, et al. Effect of air pollution on anxiety and depression in adults: a cross-sectional study. J Transp Health. (2017) 5:S95. doi: 10.1016/j.jth.2017.05.252
44. van Donkelaar A, Martin RV, Brauer M, Hsu NC, Kahn RA, Levy RC, et al. Global estimates of fine particulate matter using a combined geophysical-statistical method with information from satellites, models, and monitors. Environ Sci Technol. (2016) 50:3762–72. doi: 10.1021/acs.est.5b05833
45. Chan E, Lam H, So S, Goggins WB, Ho JY, Liu S, et al. Association between ambient temperatures and mental disorder hospitalizations in a subtropical city: a time-series study of Hong Kong special administrative region. Int J Environ Res Public Health. (2018) 15:754. doi: 10.3390/ijerph15040754
46. Leung WT, Tam TY, Pan WC, Wu CD, Lung SC, Spengler JD. How is environmental greenness related to students' academic performance in english and mathematics? Landsc Urban Plan. (2019) 181:118–24. doi: 10.1016/j.landurbplan.2018.09.021
47. Dormann CF, McPherson JM, Araújo MB, Bivand R, Bolliger J, Carl GJ, et al. Methods to account for spatial autocorrelation in the analysis of species distributional data: a review. Ecography. (2007) 30:609–28. doi: 10.1111/j.2007.0906-7590.05171.x
48. Fox J. Third Edition—Applied Regression Analysis and Generalized Linear Models. 3rd ed. Thousand Oaks, CA: SAGE Publication (2015).
49. Helbich M, de Beurs D, Kwan MP, O'Connor RC, Groenewegen PP. Natural environments and suicide mortality in the Netherlands: a cross-sectional, ecological study. Lancet Planet Heal. (2018) 2:134–9. doi: 10.1016/S2542-5196(18)30033-0
50. Sarkar C, Webster C, Gallacher J. Residential greenness and prevalence of major depressive disorders: a cross-sectional, observational, associational study of 94 879 adult UK Biobank participants. Lancet Planet Heal. (2018) 2:162–73. doi: 10.1016/S2542-5196(18)30051-2
51. Yeager R, Riggs DW, DeJarnett N, Srivastava S, Lorkiewicz P, Xie Z, et al. Association between residential greenness and exposure to volatile organic compounds. Sci Total Environ. (2020) 707:135435. doi: 10.1016/j.scitotenv.2019.135435
52. Xue T, Guan T, Zheng Y, Geng G, Zhang Q, Yao Y, et al. Long-term PM2.5 exposure and depressive symptoms in China: a quasi-experimental study. Lancet Reg Health. (2021) 6:100079. doi: 10.1016/j.lanwpc.2020.100079
53. Benjamini Y, Hochberg Y. Controlling the false discovery rate: a practical and powerful approach to multiple testing. J R Stat Soc Ser B. (1995) 57:289–300. doi: 10.1111/j.2517-6161.1995.tb02031.x
54. Jafari M, Ansari-Pour N. Why, when and how to adjust your P values? Cell J. (2019) 20:604–7. doi: 10.22074/cellj.2019.5992
55. Bezold CP, Banay RF, Coull BA, Hart JE, James P, Kubzansky LD, et al. The relationship between surrounding greenness in childhood and adolescence and depressive symptoms in adolescence and early adulthood. Ann Epidemiol. (2018) 28:213–9. doi: 10.1016/j.annepidem.2018.01.009
56. Madzia J, Ryan P, Yolton K, Percy Z, Newman N, LeMasters G, et al. Residential greenspace association with childhood behavioral outcomes. J Pediatr. (2019) 207:233–40. doi: 10.1016/j.jpeds.2018.10.061
57. Tomita A, Vandormael AM, Cuadros D, Di Minin E, Heikinheimo V, Tanser F, et al. Green environment and incident depression in South Africa: a geospatial analysis and mental health implications in a resource-limited setting. Lancet Planet Heal. (2017) 1:152–62. doi: 10.1016/S2542-5196(17)30063-3
58. Ng KST, Sia A, Ng MKW, Tan CTY, Chan HY, Tan CH, et al. Effects of horticultural therapy on asian older adults: a randomized controlled trial. Int J Environ Res Public Health. (2018) 15:1705. doi: 10.3390/ijerph15081705
59. Furuyashiki A, Tabuchi K, Norikoshi K, Kobayashi T, Oriyama S. A comparative study of the physiological and psychological effects of forest bathing (Shinrin-yoku) on working age people with and without depressive tendencies. Environ Health Prevent Med. (2019) 24:46. doi: 10.1186/s12199-019-0800-1
60. Brown SC, Perrino T, Lombard J, Wang K, Toro M, Rundek T, et al. Health disparities in the relationship of neighborhood greenness to mental health outcomes in 249,405 U.S. medicare beneficiaries. Int J Environ Res Public Health. (2018) 15:430. doi: 10.3390/ijerph15030430
61. Cohen-Cline H, Turkheimer E, Duncan GE. Access to green space, physical activity and mental health: a twin study. J Epidemiol Community Health. (2015) 69:523–9. doi: 10.1136/jech-2014-204667
62. Nesbitt L, Meitner MJ, Girling C, Sheppard SRJ, Lu Y. Who has access to urban vegetation? A spatial analysis of distributional green equity in 10 US cities. Landsc Urban Plan. (2019) 181:51–79. doi: 10.1016/j.landurbplan.2018.08.007
63. Pun VC, Manjourides J, Suh HH. Association of neighborhood greenness with self-perceived stress, depression, and anxiety symptoms in older US adults. Environ Health. (2018) 17:39. doi: 10.1186/s12940-018-0381-2
64. Meadows M, Hoffman M. The nature, extent and causes of land degradation in South Africa: legacy of the past, lessons for the future? Area. (2002) 34:428–37. doi: 10.1111/1475-4762.00100
65. FAO. APFC Session Theme: Forests for Peace and Well-Being. Asia-Pacific Forestry Commission (2019). Retrieved from http://www.fao.org/3/ca4880en/ca4880en.pdf
66. Fraser EDG. Urban ecology in Bangkok, Thailand: community participation, urban agriculture and forestry: Environments- A Journal of Interdisciplinary Studies [Environments]. (2002) 30:37–49. Retrieved from: https://www.researchgate.net/publication/228822471_Urban_ecology_in_Bangkok_Thailand_Community_participation_urban_agriculture_and_forestry (accessed January 2022).
67. Said I, Mansor M. Green infrastructure in cities and towns in Southeast Asian countries: quest for research. In: Second International Seminar on Sustainable Development. Jakarta (2011). Retrieved from http://medinanet.org/index.php/articles/uplanning/211-green-infrastructure-in-cities-and-towns-insoutheastasian-countries-quest-for-research
68. Yuen B. Use and experience of neighborhood parks in Singapore. J Leis Res. (1996) 28:293–311. doi: 10.1080/00222216.1996.11949777
69. Demoury C, Thierry B, Richard H, Sigler B, Kestens Y, Parent ME. Residential greenness and risk of prostate cancer: a case-control study in Montreal, Canada. Environ Int. (2017) 98:129–36. doi: 10.1016/j.envint.2016.10.024
70. Porcherie M, Lejeune M, Gaudel M, Pommier J, Faure E, Heritage Z, et al. Urban green spaces and cancer: a protocol for a scoping review. BMJ Open. (2018) 8:e018851. doi: 10.1136/bmjopen-2017-018851
71. Bodicoat DH, O'Donovan G, Dalton AM, Gray LJ, Yates T, Edwardson C, et al. The association between neighborhood greenspace and type 2 diabetes in a large cross-sectional study. BMJ Open. (2014) 4:e006076. doi: 10.1136/bmjopen-2014-006076
72. Fan S, Xue Z, Yuan J, Zhou Z, Wang Y, Yang Z, et al. Associations of residential greenness with diabetes mellitus in Chinese Uyghur adults. Int J Environ Res Public Health. (2019) 16:5131. doi: 10.3390/ijerph16245131
73. Eldeirawi K, Kunzweiler C, Zenk S, Finn P, Nyenhuis S, Rosenberg N, et al. Associations of urban greenness with asthma and respiratory symptoms in Mexican American children. Ann Allergy Asthma Immunol. (2019) 122:289–95. doi: 10.1016/j.anai.2018.12.009
74. Squillacioti G, Bellisario V, Levra S, Piccioni P, Bono R. Greenness availability and respiratory health in a population of urbanized children in North-Western Italy. Int J Environ Res Public Health. (2020) 17:108. doi: 10.3390/ijerph17010108
75. Yang BY, Liu KK, Markevych I, Knibbs LD, Bloom MS, Dharmage SC, et al. Association between residential greenness and metabolic syndrome in Chinese adults. Environ Int. (2020) 135:105388. doi: 10.1016/j.envint.2019.105388
76. Ulrich RS. Effects of gardens on health outcomes: theory and research. In: Cooper Marcus C, Barnes M, editors. Healing Gardens: Therapeutic Benefits and Design Recommendations. New York, NY: John Wiley (1999). p. 27–86.
77. Ulrich RS, Zimring C, Zhu X, DuBose J, Seo HB, Choi YS, et al. A review of the research literature on evidence-based healthcare design. HERD. (2008) 1:61–125. doi: 10.1177/193758670800100306
78. Kaplan R, Kaplan S. The experience of nature: a psychological perspective. In: The Experience of Nature: A Psychological Perspective. Cambridge; New York, NY: Cambridge University Press (1989).
79. Bolte G, Nanninga S, Dandolo L. Sex/gender differences in the association between residential green space and self-rated health—a sex/gender-focused systematic review. Int J Environ Res Public Health. (2019) 16:4818. doi: 10.3390/ijerph16234818
80. Andrusaityte S, Grazuleviciene R, Dedele A, Balseviciene B. The effect of residential greenness and city park visiting habits on preschool children's mental and general health in Lithuania: a cross-sectional study. Int J Hyg Environ Health. (2020) 223:142–50. doi: 10.1016/j.ijheh.2019.09.009
81. Bowler DE, Buyung-Ali LM, Knight TM, Pullin AS. A systematic review of evidence for the added benefits to health of exposure to natural environments. BMC Public Health. (2010) 10:456. doi: 10.1186/1471-2458-10-456
82. de Vries S, Verheij RA, Groenewegen PP, Spreeuwenberg P. Natural environments—healthy environments? An exploratory analysis of the relationship between greenspace and health. Environ Plan A Econ. (2003) 35:1717–31. doi: 10.1068/a35111
83. Han, K.-T. Influence of limitedly visible leafy indoor plants on the psychology, behavior, and health of students at a junior high school in Taiwan. Environ Behav. (2009) 41:658–92. doi: 10.1177/0013916508314476
84. Huynh Q, Craig W, Janssen I, Pickett W. Exposure to public natural space as a protective factor for emotional well-being among young people in Canada. BMC Public Health. (2013) 13:407. doi: 10.1186/1471-2458-13-407
85. Keniger LE, Gaston KJ, Irvine KN, Fuller RA. What are the benefits of interacting with nature? Int J Environ Res Public Health. (2013) 10:913–35. doi: 10.3390/ijerph10030913
86. Pretty J, Peacock J, Hine R, Sellens M, South N, Griffin M. Green exercise in the UK countryside: effects on health and psychological well-being, and implications for policy and planning. J Environ Plan Manag. (2007) 50:211–31. doi: 10.1080/09640560601156466
87. Strife S, Downey L. Childhood development and access to nature: a new direction for environmental inequality research. Organ Environ. (2009) 22:99–122. doi: 10.1177/1086026609333340
88. Sugiyama T, Leslie E, Giles-Corti B, Owen N. Associations of neighborhood greenness with physical and mental health: do walking, social coherence and local social interaction explain the relationships? J Epidemiol Community Health. (2008) 62:e9. doi: 10.1136/jech.2007.064287
89. Taylor AF, Kuo FE, Sullivan WC. Coping with add: the surprising connection to green play settings. Environ Behav. (2001) 33:54–77. doi: 10.1177/00139160121972864
91. Zhan Y, Liu J, Lu Z, Yue H, Zhang J, Jiang Y. Influence of residential greenness on adverse pregnancy outcomes: a systematic review and dose-response meta-analysis. Sci Total Environ. (2020) 718:137420. doi: 10.1016/j.scitotenv.2020.137420
92. Perrino T, Lombard J, Rundek T, Wang K, Dong C, Gutierrez CM, et al. Neighborhood greenness and depression among older adults. Br J Psychiatry. (2019) 215:476–80. doi: 10.1192/bjp.2019.129
93. Gilroy R. Places that support human flourishing: lessons from later life. Plan Theory Pract. (2008) 9:145–63. doi: 10.1080/14649350802041548
Keywords: country-level, DALY loss, depressive disorders, global analysis, greenness
Citation: Asri AK, Tsai H-J, Pan W-C, Guo YL, Yu C-P, Wu C-S, Su H-J, Lung S-CC, Wu C-D and Spengler JD (2022) Exploring the Potential Relationship Between Global Greenness and DALY Loss Due to Depressive Disorders. Front. Psychiatry 13:919892. doi: 10.3389/fpsyt.2022.919892
Received: 14 April 2022; Accepted: 07 June 2022;
Published: 28 June 2022.
Edited by:
María del Mar Molero Jurado, University of Almeria, SpainReviewed by:
Sitong Chen, Victoria University, AustraliaCopyright © 2022 Asri, Tsai, Pan, Guo, Yu, Wu, Su, Lung, Wu and Spengler. This is an open-access article distributed under the terms of the Creative Commons Attribution License (CC BY). The use, distribution or reproduction in other forums is permitted, provided the original author(s) and the copyright owner(s) are credited and that the original publication in this journal is cited, in accordance with accepted academic practice. No use, distribution or reproduction is permitted which does not comply with these terms.
*Correspondence: Chih-Da Wu, Y2hpZGF3dUBtYWlsLm5ja3UuZWR1LnR3
Disclaimer: All claims expressed in this article are solely those of the authors and do not necessarily represent those of their affiliated organizations, or those of the publisher, the editors and the reviewers. Any product that may be evaluated in this article or claim that may be made by its manufacturer is not guaranteed or endorsed by the publisher.
Research integrity at Frontiers
Learn more about the work of our research integrity team to safeguard the quality of each article we publish.