- 1Division of Newborn Medicine, Boston Children’s Hospital, Boston, MA, United States
- 2Fetal-Neonatal Neuroimaging and Developmental Science Center, Boston Children’s Hospital, Boston, MA, United States
- 3Department of Pediatrics, Harvard Medical School, Boston, MA, United States
- 4Abbott, Columbus, OH, United States
- 5Department of Radiology, Boston Children’s Hospital, Boston, MA, United States
Multimodal brain magnetic resonance imaging (MRI) can provide biomarkers of early influences on neurodevelopment such as nutrition, environmental and genetic factors. As the exposure to early influences can be separated from neurodevelopmental outcomes by many months or years, MRI markers can serve as an important intermediate outcome in multivariate analyses of neurodevelopmental determinants. Key to the success of such work are recent advances in data science as well as the growth of relevant data resources. Multimodal MRI assessment of neurodevelopment can be supplemented with other biomarkers of neurodevelopment such as electroencephalograms, magnetoencephalogram, and non-imaging biomarkers. This review focuses on how maternal nutrition impacts infant brain development, with three purposes: (1) to summarize the current knowledge about how nutrition in stages of pregnancy and breastfeeding impact infant brain development; (2) to discuss multimodal MRI and other measures of early neurodevelopment; and (3) to discuss potential opportunities for data science and artificial intelligence to advance precision nutrition. We hope this review can facilitate the collaborative march toward precision nutrition during pregnancy and the first year of life.
Introduction
Optimal nutrition early in life is essential to support the rapid growth and development during infancy, especially among infants born preterm or with severe medical conditions. A critical period, from the 3rd trimester of pregnancy through the first 1,000 days of life, encompasses multiple simultaneous neurodevelopment processes that lay the foundation for increasing neural complexity and subsequent refinement (1, 2). The extended developmental timeline of the human brain provides a large risk surface for environmental factors to affect the developmental trajectory, either by increasing plasticity or vulnerability. While plasticity can provide an increased ability to repair injury, resilience factors can be superseded by damaging factors (3). Impaired brain development during this critical period can result in lifelong functional deficits and an increased risk of neurological disorders (4).
Nutrition plays a key role in supporting proper neurodevelopment. Adequate supply of both macronutrients and micronutrients is imperative to support the complex processes underlying brain development. Myelination accelerates during infancy (5) and is affected by intake of nutrients such as long-chain fatty acids, choline, iron, sphingomyelins and folic acid (6). At 2 years of age, synaptic density peaks are 50% higher than in adulthood (7). Tremendous energy in the form of macronutrients is required to fuel such extensive development. In fact, brain glucose consumption at 2 years of age is already equivalent to that of the adult and continues to rise during childhood, accounting for 50% of the basal metabolic rate by age 10 (8). Malnutrition during the prenatal and postnatal periods inflicts a tremendous cost on the welfare and lifetime achievement of the individual, and therefore to society as well. Indeed, if the three most common micronutrient deficiencies (zinc, iron and vitamin A) were corrected, it is estimated that global IQ would increase by 10 points (9).
Advances in multimodal brain magnetic resonance imaging (MRI) is enabling precision nutrition – optimizing nutrition for individuals – which has the potential to provide high-impact nutritional interventions (10). Multimodal MRI provides insights on the nutritional requirements of specific developmental stages, and progress to the ability to provide individual recommendations. Several nutrients have been specifically studied for early life impacts on neurodevelopment (Table 1) when supplemented after birth. Currently, we have limited knowledge regarding how nutrients interact with other nutrients and non-nutritional factors to modify health outcomes. Understanding these interactions will improve recommendations for populations and individuals. This paper surveys mother-infant nutritional studies, focusing on emerging data science opportunities toward precision nutrition in term infants. Part I reviews current knowledge on the effects of factors (nutritional, genetic and environmental) on infant brain development. Part II discusses data science opportunities in multimodal MRI and marker studies for nutrition. Part III lists major databases, trials and resources relevant to data-driven precision nutrition.
Maternal Factors Influencing Infant Brain Development
Maternal diet plays a crucial role in nutritional status before and during pregnancy, and in breastmilk nutrition during lactation (1, 11–19). These factors, along with parental, environmental, genetic, socioeconomic, and other variables, influence infant brain development and neurocognitive functions later in life (20–22; Figure 1). The process is multifactorial and complex. For decades, multidisciplinary research has aimed to identify key dietary influences during pre-conception, pregnancy, and lactation that can be the basis for efforts to improve infant health and development (Table 1; 23–26). Likewise, researchers have studied developmental differences between breastfed and formula-fed infants driven by extremely complex nutritional differences between breast milk and infant formula and also inherent non-nutritional differences between those populations. There are many societal factors that can facilitate or hinder breastfeeding, so differences between breastfeeding and formula feeding could also result from these non-nutritional influences. While those studies have been successful in determining the essential role of many nutrients such as folate and iodine that have significant consequences when in deficit, new analytical approaches open the door to understanding the complex interactions between dietary nutrients and designing individualized dietary recommendations that account for subtle effects and complex interactions between dietary nutrient and non-nutritional factors. Further, existing studies on early nutritional influences mostly focus on preterm infants (23, 27–33), due to the high risk of nutritional deficiencies and neurodevelopmental concerns in this vulnerable population. Term-born infants, on the other hand, face different nutritional needs (34, 35), and warrant specific studies.
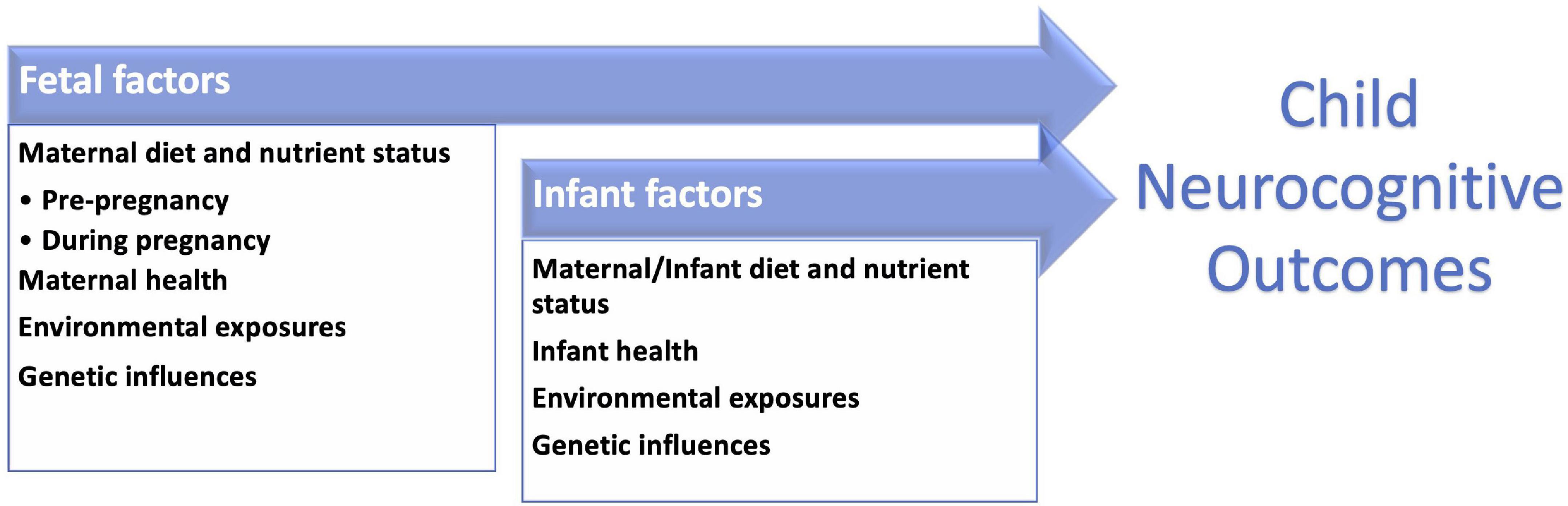
Figure 1. Neurodevelopmental outcomes in childhood are influenced by multiple factors including those present in the prenatal period and infant stages.
Pre-conception
The maternal diet prior to conception affects pregnancy outcomes and infant neurodevelopment (14, 16, 17, 36). Prenatal deficiency in micronutrients such as folate, iron, and iodine have been shown to impact infant outcomes. For example, maternal pre-conception folate supplementation was found to reduce neural tube defects (17). Offspring of mothers that received a pre-conception supplement of folic acid and iron displayed improved fine motor scores at 24 months of age compared to children of mothers that received folic acid alone (37). Iodine deficiency can negatively impact the development of the cerebral cortex and axonal connections, as well as the myelination of the central nervous system (38). Low pre-conception maternal intake of iodine is associated with lower IQ in school-aged children aged 6-7 years, emphasizing the long-lasting impact of pre-conception nutrition deficiencies (39). In addition to specific nutrients, the activity of metabolic pathways can be measured pre-conception. Higher maternal homocysteine levels, reflective of sub-optimal one-carbon metabolism, were associated with an elevated risk of anxiety, depression, and social problems at school age (40). However, our current knowledge is very general and does not incorporate individual patient nutritional needs. Future work to understand interactions between micronutrients, as well as differences in individual responsiveness to nutrient deficiency or excess, is needed to effectively assess the value of and potential targets for nutritional intervention in the pre-conceptual period.
During Pregnancy
During pregnancy, maternal intake of nutrients such as folate, iron, iodine, zinc, choline and polyunsaturated fatty acids can promote the development of the brain and central nervous system in infants (41). Folic acid supplementation is associated with increased brain volume as well as increased performance in language, cognitive (42) and visuospatial domains (43). However, questions remain about how the accumulation of unmetabolized folic acid from high intake may impact fetal development (44). Intrapartum maternal iron deficiency leads to decreased fetal iron stores (45), which can persist for many months after birth (46). Infants with iron deficiency at birth have lower language and fine motor scores at 5 years of age (47). Iron deficiency during infancy leads to impaired inhibitory control in later childhood (48) and poorer cognition and socio-emotional function through adolescence and young adulthood (49, 50). Iodine deficiency is estimated to affect as many as 2 billion people worldwide and is the leading cause of preventable impaired mental functioning (51). Fetal iodine deficiency is associated with irreversible visual and cognitive impairment, as well as worse gross motor development (52). Gestational zinc deficiency has been associated with decreased novelty preference (53), though clinical trials have failed to demonstrate positive effects of maternal zinc supplementation upon child neurodevelopment (54). Choline is essential for neurodevelopment, and maternal choline intake is positively associated with child neurodevelopmental outcomes, including processing speed, attention, inhibitory control, and spatial memory (55). Inadequate gestational supply of nutrients such as folate and polyunsaturated fatty acids (docosahexaenoic & linolenic acid) can impair brain development (56). Given the growing evidence base for the role of maternal intrapartum nutrition on infant brain development, it is an ideal time to apply precision medicine approaches in future studies.
Lactation
During early lactation, an infant is dependent solely on the nutrients from maternal breast milk for nutrition and calories (21). Proper nutrition remains critical in the first years of life to support brain development, including intake of iodine, choline, polyunsaturated fatty acids and other nutrients (1, 3). Key nutrients such as iodine continue to be important during lactation. Maternal supplementation of iodine in populations with high rates of deficiency has been shown to lower infant mortality rates improve cognitive function (57). Additionally, maternal supplementation with iodine during lactation improved psychomotor development (58). A recent systematic review concluded that preclinical and clinical evidence indicates choline supplementation of maternal and/or child diets during the first 1,000 days of life supports normal brain development and increases resilience to developmental insults (59). Other studies are beginning to clarify the role of omega-3 fatty acids in early development. A study of docosahexaenoic acid (DHA) supplementation during infancy demonstrated higher gesture scores at 12 and 18 months (60). Recently, multivariate analysis identified positive associations between maternal intake of omega-3 fatty acids and sub-regional volumes in the frontal cortex and corpus callosum at 1 month of age among a cohort of 92 term-born breastfeeding mother-infant dyads (61). One ongoing application of precision nutrition during lactation is the targeted supplementation of fat and protein using milk analysis for hospitalized infants (62). With advances in the availability of milk analysis, individualized supplementation of expressed human milk could improve growth and developmental outcomes among high-risk infants.
Breastfeeding
A common question is how the relative exposure to breastmilk and formula influences infant neurodevelopment. Breastfeeding exposure has consistently correlated with neurodevelopmental differences in school-aged children. Neurocognitive studies found significantly lower IQ scores as measured by Kaufman Brief Intelligence Test and Wechsler Abbreviated Scale of Intelligence among school-aged children who were fed formula as infants (63–65). These studies, together with others, quantified a neurodevelopmental benefit to breastfeeding during infancy but did not identify any specific nutrients responsible for the association.
Multimodal brain MRI correlates of these neurodevelopmental outcomes are a first step toward defining potential biomarkers. Diffusion MRI studies found lower fractional anisotropy values (i.e., more diffuse water flow) in left hemisphere white matter regions among 18 formula-fed male children compared to 10 breastfed male children at 8 years of age (66). As fractional anisotropy is sensitive to white matter integrity, and could be an early biomarker of the impacts of breastfeeding on brain development. Emphasizing the role of non-nutritional influences, there was no observed effect among female children. Structural MRI studies at the same age also demonstrated lower gray matter volume in the left inferior temporal and superior parietal lobes of 10 male infants who were fed formula compared to 10 male infants who were breastfed (67). Two larger studies extended these findings, identifying lower volumes across the whole brain, total gray matter, cortical gray matter, and subcortical gray matter among ∼10-year-old children who were fed formula during infancy (N = 148) (65); and thinner cortical surfaces in the superior and inferior parietal lobes at 12-18 years of age among 301 children who were fed formula as infants compared to 270 breastfed infants (64). Besides, specialized brain MRI approaches to quantify myelination (68) identified earlier and more rapid myelination in the frontal and temporal white matter, peripheral aspects of the internal capsule and corticospinal tracts, superior longitudinal fasciculus and superior occipital-frontal fasciculus from 10 months to 4 years of age in breastfed infants (69). These studies highlight potential neuroanatomical biomarkers for previously observed neurocognitive associations with breastfeeding (70). However, currently identified markers often have weak effect size and limited ability to predict outcomes. But group-level information about brain structure can be an unparalleled tool to understand the dynamic, temporal nature of nutrient effects on brain development and confounding factors.
While the above studies were cross-sectional, longitudinal data can determine how nutritional exposure alters the trajectory of neurodevelopment. One study of term infants included brain MRI measures at 6, 12, 18, 24, 36, 48, and 60 months, totaling 231 MRI scans from 39 breastfed infants and 221 MRI scans from 54 formula-fed infants (6). Improved overall myelination in the white matter and corpus callosum was found in breastfed children, and the difference increased in magnitude from birth to about 2 years of age then plateaued thereafter. Neurocognitive scores as measured by Wechsler Abbreviated Scale of Intelligence were significantly higher in general, verbal, and non-verbal cognitive domains among breastfed infants. Also, when the formula-fed cohort was divided by formula composition, the formula-based intake of sphingomyelin, phosphatidylcholine, folic acid, iron, choline, vitamin B12, and two omega-3 fatty acids (DHA and arachidonic acid) were associated with myelination differences in focal brain areas. Future studies need to address how breastfeeding alters development during 0-6 months of age, when the brain develops most rapidly.
Non-nutritional Factors
Environmental influences on human neurodevelopment, both positive and negative, begin prenatally (12, 22, 71, 72). Preconception, non- nutritional influence from both parents can shape infant development (73). Maternal adverse psychosocial exposures before conception can influence child development (13, 74–76). Potential mechanisms for the transfer of maternal stress responses to the fetus include transplacental passage of molecular factors, alterations to the uterine environment, and epigenetic changes (73, 77, 78). Postnatally, adverse experiences during childhood are associated with altered brain architecture as well as adverse behavioral and educational outcomes (79–81). For example, childhood poverty is associated with decreased executive function skills; further, childhood cortisol levels were correlated with poverty and measures of executive function (80). Other environmental exposures including toxins, can negatively affect neurodevelopment (82): exposures to endocrine-disrupting chemicals can increase the risk of adverse outcomes such as neurobehavioral disorders (78). Finally, nutritional influences on neurodevelopment are modified by exposures to inflammation, both related to adverse experiences as well as systemic illness or changes in the microbiome (15, 83, 84). For example, iron is sequestered as part of the inflammatory response, with chronic inflammation resulting in iron deficiency anemia in vulnerable populations (85, 86).
Maternal health also influences long-term child neurodevelopment. There are critical windows such as the first trimester, when maternal endocrinological conditions, such as abnormal thyroid hormone levels, can affect brain development (87). A cohort of school-aged children born to women with untreated hypothyroidism had lower neuropsychiatric testing scores across several domains including language and visual-motor performance, compared to peers without maternal hypothyroidism (88). Further, maternal conditions such as obesity and pre-eclampsia alter the placental transfer and fetal accumulation of omega-3 fatty acids (89). Specific dietary factors may be most beneficial among infants affected by maternal conditions.
Genetic predispositions can also affect infant neurodevelopmental outcomes, and modifies the effect of other exposures (90). For example, the presence of a maternal polymorphism in peroxisome proliferator-activated receptor gamma (PPARG), which leads to decreased protein activity, was associated with lower scores in cognitive, language, and motor realms in offspring at 18 months of age (91). The child’s PPARG allele status was not correlated with these outcomes, indicating the lasting influence of the intrauterine environment on long-term development in childhood. Exposure to toxins, in utero and/or postnatally, can negatively impact neuropsychiatric development. For example, in a Taiwanese cohort of 181 children, higher cord blood mercury concentrations were associated with lower cognitive scores at 2 years of age, most significantly among children with a high-risk allele of Apolipoprotein E (92). Similarly, the relationship between child motor development at 20 months of age and maternal blood mercury concentrations were affected by common variants in glutathione-related genes (93). Common variants in glutathione metabolism genes also increased the negative effect of intrapartum maternal exposure to secondhand smoke on cognitive performance, as measured by the Bayley Scales of Infant Development at 2 years of age (94). With such an array of potentially influential factors on child development, a nuanced approach that customizes positive factors such as nutrition may be most effective if personalized based on co-existing risk factors.
Data Science Opportunities Toward Precision Nutrition
The rise of data science, including the application of artificial intelligence to big data, offers unique opportunities for nutritional science. We can generate new hypotheses that can drive intervention studies testing combinations of nutrients and other factors. In addition, machine learning can also enable the analysis and interpretation of large-scale clinical studies. While much interventional nutrition research focuses on one or a few nutrients (95), modern data science allows us to consider multiple nutrients together and quantify their complementary or competing contributions to outcomes. Nutritional intervention studies typically involve only one or a few nutrients, while retrospective correlation studies can examine multiple nutrients, these nutrients are often analyzed separately. Further, only a limited set of non-dietary factors (96, 97) (environmental, genetic, socioeconomic, etc.) have been typically included in models of nutritional effects. In contrast, machine learning approaches can jointly consider all these factors to identify biomarkers of important outcomes, or to determine influential dietary and/or nutritional supplements given other features of an individual mother-infant dyad such as genetic risk, environmental exposures, or previous nutritional interventions. Big data in this context could refer to large cohorts, large number of variables per participant, or both. Such approaches fit well with the concept of precision nutrition (98) – nutrition that can be optimized for individuals, which is elaborated in the 2020-2030 Strategic Plan for National Institute of Health (NIH) Nutrition Research as released in May 2020 (99).
A challenge of the traditional approach to nutrition studies of neurodevelopment is that, in addition to measuring the efficacy of a particular nutrient dose, the temporal window for optimal influence must also be assessed for each nutrient or combination of nutrients. In many cases, a nutritional deficiency may exist, even in healthy and term-born infants, but the effects of the deficiency may not be evident given the long time between exposure and measurable outcome, requiring highly sensitive and specific biomarkers to be identified (100–102). Traditionally, the impact of infant intake of a single nutrient on neurodevelopmental outcomes, or a biomarker of that outcome, is followed over time, typically in school age, childhood, or adolescence (28, 50, 63, 67, 103). This long interval between exposure and assessment is necessary because many neurodevelopmental outcomes cannot be measured until school age or adolescence. For example, the varying impact of supplementation with DHA on neurodevelopment (104–107) may be clarified using machine learning to improve sensitivity by accounting for confounding factors. The need for early intervention fuels studies of blood biomarkers or non-invasive imaging. Often, nutrition studies require highly sensitive and specific biomarkers (100–102) because the effects of suboptimal nutritional status are only detectable after a long post-exposure latency. Such markers could enable the optimization of nutrition at the earliest time possible for mothers and infants. Finally, combining longitudinal data using machine learning could develop new hypotheses on nutrient combinations that impact markers or outcomes.
Multimodal Brain MRI
Neuroimaging such as multimodal brain MRI and magnetoencephalography can non-invasively and quantitatively measure localized effects of specific nutrient on brain structure and function (26, 32, 61), identify neuroanatomic mechanisms linking nutritional status with brain development outcomes, and evaluate the effects of nutritional interventions (29, 100–102). Neuroimaging provide objective and quantitative outcomes that compliment other nutrition biomarkers such as reported diet. Disadvantages include logistical hurdles and expense of image acquisition, and the requirement of advanced analytics. At least two recent advancements are facilitating the use of brain MRI in nutritional studies. The first is the rise of brain MRI databases that span the human lifespan (100–102). Hundreds to tens of thousands of brain MRI data from healthy controls are now available publicly, many with nutrition, parental, socioeconomic, behavior, neurocognitive development, environment, and other types of comprehensive data. The other advancement, as elaborated later, is the quantification of normal brain development in space across different anatomic locations, in time across ages, and across modalities that alternatively assess structural, diffusion, metabolic, or functional data (108). These increase our ability to pinpoint the subtle and complex patterns associated with specific nutrients or malnutrition.
Multi-modal brain MRI offers comprehensive tools to evaluate the effect of nutrition on the brain structure and function. Structural, or anatomic, brain MRIs such as T1- and T2-weighted sequences help reveal nutritional effects on regional volumes (61, 109–112) as well as cortical surface thickness, area, curvature and folding patterns (113–115). T2* and T2’ sequences, in particular, have shown potential to directly quantify iron levels in the brain (116, 117), as has quantitative susceptibility mapping (117–119). Diffusion-weighted and diffusion-tensor brain MRIs offer a lens to quantify white matter water diffusion. Diffusion-tensor-based parameterization maps such as fractional anisotropy, mean diffusivity, apparent diffusion coefficient, radial diffusivity, and axial diffusivity images measure water diffusion magnitude and directionality. Diffusion-tensor-reconstructed tractography quantifies the structural connectivity along white matter tracts (32, 109, 120, 121). Functional MRI of the brain, on the other hand, measures the brain circuits/connectivity based on blood flows, and have been used to probe the neural substrate of nutrition on neurodevelopmental outcomes (122–125). Magnetic resonance spectroscopy reveals the metabolism in the brain that can be related to nutrition (126–129). The choice and the optimal combination of various brain MRI sequence remain an open topic, though, and are largely dependent on the cohort, specific nutrients, and study aims (100, 102).
Neurophysiology Tools
Brain activity and its developmental trajectory can be monitored non-invasively using scalp electroencephalography (EEG) or magnetoencephalography (MEG). EEG/MEG recordings are non-invasive procedures that can be performed in subjects of any age or clinical status. This makes them suitable tools for testing the short-term and long-term neurophysiological effects of malnutrition or nutritional interventions.
EEG is sensitive to the neural correlates of cognitive dysfunction associated with early childhood malnutrition (130). Childhood malnutrition, before the age of two years, is shown to lead to irreversible shifts in the cerebral activity organization that are measurable through EEG (131–134). Quantitative EEG biomarkers of the malnutrition effects may help stratify children at risk of adverse neurodevelopmental effects and inform targeted interventions. An EEG-based age-adjusted classifier (mainly driven by the EEG alpha activity in the lingual gyrus) was also recently developed, which distinguished infants with histories of protein-energy malnutrition from healthy controls with 82% accuracy (134). This encourages the use of EEG as a mediator in disease progression models to optimize cost-effective interventions.
A demonstrated association was also shown between infant diet and early postnatal development of gamma EEG activity, which is sensitive to the development of the GABAergic system (135). EEG activity during infancy was also shown to differ between infants who are fed through breastfeeding versus milk or soy formula, possibly suggesting that EEG measures may reflect environmental- or diet-related influences on the development of brain function that could lead to different neurodevelopmental trajectories (136). Nutritional exposures outside of infancy also lead to differences in EEG traits. Resting-state EEG activity is altered by the content of omega-3 and omega-6 fatty acids diets in infants, children, and adults (100).
With a coordinated effort, large EEG datasets could become available as EEG technology is widely used in hospital settings and can be recorded at the bedside. The use of high-resolution EEG techniques or even MEG, as well as the use of recent advanced computational methods for data analysis, can potentially enhance the development of neurophysiological markers of nutritional interventions or disorders. To our best knowledge, no MEG studies exist investigating these aspects. Nevertheless, the increasing availability of MEG systems worldwide will likely bring to wider, multi-center collection of MEG data in this direction.
Non-imaging Biomarkers for Early Nutritional Interventions
As the time window for nutritional interventions to optimize infant neurodevelopment is likely to precede measurable outcomes, proximal biomarkers of neonatal nutritional status are necessary. Potential markers of infant nutritional status include weight, head circumference, body composition, and macular pigment density (137–145). Body composition measurements determine the relative amounts of fat and fat-free mass, the latter of which includes total body water, bone mineral, and muscle mass (146). Body composition can be measured by multiple approaches including anthropometric measurements, isotope dilution, dual energy x-ray absorptiometry, bioelectrical impedance analysis, MRI, and air displacement plethysmography (147). Each measure has varying measurement types, degrees of accuracy, measurement time, expense, and associated exposures. Differences in body composition among preterm infants have been associated with speed of processing at preschool age (148), indicating that body composition has promise as a proximal biomarker of nutrient effects on neurodevelopment. In addition, regional bone mass (mineral and density) such the head region measured with dual energy X-ray absorptiometry (DEXA) which correlates very well with head circumference measures may potentially serve as a non-invasive marker for brain development.
Macular pigment optical density (MPOD) often reflects lutein and zeaxanthin intake in the adult population (137, 149, 150). It is quantitative and non-invasive measure that can be followed serially over time. Studies have demonstrated the feasibility of measurement among preterm infants (151) and a positive correlation between maternal serum zeaxanthin levels and infant MPOD measurements (152). Breastfed infants have higher MPOD values than formula-fed infants (153, 154), though the exact milk components responsible for this difference remain to be determined. Childhood MPOD measures have been associated with academic performance, supporting its potential role as a biomarker of neurodevelopment (155). However, open questions include (a) whether MPOD remains stable during lactation, and the lack of longitudinal studies in a large cohort for the dynamics of MPOD from pre-conception, pregnancy, and postpartum; (b) what exact nutrients or nutrient combinations in these three stages do MPOD reflect in maternal nutrition – because existing evidence that MPOD reflects lutein and zeaxanthin intake has been from non-pregnant populations. This association may or may not replicate in mothers before, during, and after pregnancy as dietary carotenoids are also transferred to breastmilk (153, 155) and therefore are likely to have a different relationship with MPOD (145).
Measuring Nutritional Intake
Ascertainment of the effects of maternal nutrient intake on infant outcomes can be achieved through targeted supplementation of single or multiple nutrients, or by passive assessment of nutrient intake. Estimating nutrient intake from consumption of infant formulas can include significant error unless bottles are weighed before and after feedings. A formalized, quantitative method to profile maternal nutrient intake is food frequency questionnaire (FFQ) (156, 157). FFQs collect the portion size and frequency of consumption among a list of foods, and can be self-administered or completed by interview (158). This food data is then converted into daily nutrient intake values (159, 160). Advantages to FFQs include scalability, ease of administration, and opportunity for serial measurement. Disadvantages include concerns about reproducibility and systematic errors and biases associated with self-reported data, memory-based measurements, inability to verify or falsify data (161), or objective infant brain measurement (61), and data processing assumptions.
Multivariate Analysis of Macro/MicroNutrient Interactions
The majority of existing studies focus on the effects of a single nutrient, or multiple nutrients examined independently. However, nutrients interact with each other to produce biological effects. For example, choline and DHA interact during eye and brain development (162); vitamin B12, iron and folate all affect red blood cell development (163); and lutein, vitamin E, arachidonic acid and DHA interact during brain development (164–166). Defining additional subsets of nutrients that jointly impact brain development and general health is an essential step toward precision nutrition (167). Multivariate analysis in machine learning and data science is appropriate for the task. One obvious challenge, though, is the need for big data including a full spectrum of nutrient, biomarker and outcome data for ideally thousands or more individuals.
Pathway Connecting Maternal Diet, Breastmilk, Infant Brain Development and Later Neurocognition
Current mother-infant dyad studies focus on associations between nutrient intake and infant outcomes, but causality is not addressed by linking maternal dietary factors to changes in breastmilk composition. For instance, breastmilk components that influence infant brain health may be constant and not significantly modified by maternal intake of nutrients. Alternatively, the maternal nutrients associated with infant brain health may not be related to the quantity of breastmilk components but could instead vary based on the bioavailability or activity of nutrients. Furthermore, nutrient bioavailability and activity may vary during pre-conception, pregnancy, and lactation periods. One way to move toward a causality study is to find a pathway linking maternal diet, breastmilk components, and infant development (either neurocognitive measurements or biomarkers such as brain MRI measures, or both). Establishing a pathway can generate a specific hypothesis regarding nutrients or supplements that may impact breastmilk components and subsequently improve infant brain health. These nutrients could then be studied in randomized trials, the modification of which may show the maximum impact in infant brain development. Recent advancements in multivariate analysis, artificial neural network analysis, and graph theory set the stage for such a pathway study.
Moving From Population Science to Precision Nutrition
Precision nutrition can produce tailored nutritional intake recommendations to optimize health and outcomes (168). By contrast, most nutritional studies focus on population-level associations. Whereas individuals differ by dietary habits, genetics, environmental exposures, parental factors, health status, microbiome, metabolism, socioeconomics, physical activity, psychosocial characteristics, and other factors, precision nutrition aims to create individual nutrition recommendations that consider these complex influences (169–171). Precision nutrition is an important constituent of precision medicine (98). The 2020-2030 NIH Strategic Plan for Nutrition Research articulated four strategic goals: (1) discover what we eat and how it affects us; (2) investigate what and when we should eat; (3) define how nutrition promotes health across our lifespan; and (4) understand how to modify diets to improve health, or “Food as Medicine” (99). The prenatal period, as reviewed in this paper, marks the earliest time that precision nutrition can make an impact, and arguably the time precision nutrition can make the largest lifelong impact.
Existing Resources to Power Precision Nutrition
Birth Cohorts
Some large birth cohort studies and national registries have been collecting data since the 1990s or earlier. These databases aim to provide information that advances our understanding of how various factors act together to impact psychical, socio-emotional, cognitive and behavior development of participants across infancy, adolescents, adulthood, and subsequent generations (172, 173). A typical birth cohort contains thousands to tens of thousands of participants, who were followed up for years or decades for these comprehensive sets of data (Table 2). In all these birth cohort studies, nutritional data is collected alongside other measures that are potential biomarkers of development. Some studies include brain MRI measurements, sometimes longitudinally collected. Examples of such MRI-containing birth cohorts are Dutch Generation R, FinnBrain, Norwegian MoBa, Singapore GUSTO cohorts. Merging data across cohorts, a big data opportunity not yet explored, may face problems such as missing or differently-formatted data. A subset of the datasets may share sufficient data characteristics to be tangible for meta-analysis.
Quantification of Typical Brain Development Over the Lifespan
Healthy brain MRIs in the public domains have grown from ∼8000 in 2014 (174) to now more than 40,000, and including many that represent timepoints now across human lifespan (174–176). Ongoing projects include the HEALthy Brain and Child Development (HBCD) initiative, which is collecting comprehensive birth cohort data from thousands of mother-infant dyads in the next decade in more than 20 sites across the United States (177). Datasets that depict typical neonatal brain development through early childhood are increasingly available at a 1 mm x 1 mm x 1 mm spatial resolution and high temporal resolution (bi-weekly, monthly, quarterly and annual intervals) (178–181). Fetal brain atlases are now available at a weekly interval between 19 and 39 weeks of age (182). These valuable brain MRI data during typical development provide baseline measures for comparison in future cohorts, though the applicability of each reference dataset should have careful consideration of potential confounding exposures (183, 184). Computer-constructed atlas characterizes structural, diffusion and functional neurodevelopment at high spatial and temporal granularity (185). Machine learning-driven age prediction captures subtle deviation compared to a subject’s chronological age, reflecting accelerated or slowed changes associated with aging (186–188). These datasets and sensitive algorithms equip nutrition researchers with a better understanding of brain development in early life, and a series of tools to explore the relationship between maternal nutrition and infant brain health. Opportunities also emerge for brain MRI to identify subtle nutrition deficiencies and suggest nutritional supplements for individual mother-infant dyads, as well as to assess the influence of additional factors relevant to precision nutrition on sensitive MRI biomarkers of development.
Ongoing Clinical Trials
While there are many clinical nutritional intervention studies, few collect the comprehensive data necessary for precision nutrition. At clinicaltrials.gov (accessed on 01/09/2022), there are 202 clinical studies currently enrolling participants that are identified by the keyword ‘nutrition’ and participant age of 0 years, 103 of which are interventional. There were an additional 82 active clinical studies, 49 of which are interventional. Ten active interventional trials involved dietary supplements, mostly focused on preterm infants (7 of 10). An additional 792 completed clinical trials were identified using the same search terms, of which 549 were interventional. One hundred thirty-two were focused on infants, but again only those born preterm, while only 2 included neurodevelopmental outcomes or utilized brain magnetic resonance imaging. Thus, research motivation for studying nutritional interventions for infants is high, but few studies focus on term infants, neurodevelopment or MRI analysis of brain structure or connectivity.
National Institutes of Health Nutritional Task Force
The NIH Nutrition Research Task Force, formed in October 2016 (189), released a 10-year (2020-2030) strategic plan to accelerate nutrition research into precision nutrition in May 2020 (99, 190). To achieve the third of four NIH-wide strategic goals – “to define the role of nutrition across the lifespan” – three specific objectives were developed to focus on nutrition in the pre-conception, pregnancy and infancy stages. These objectives are: examine the role of peri-conceptional and prenatal nutrition in development and disease outcomes (Objective 3-1); enhance knowledge of human milk composition and the translational roles of its components (Objective 3-2); and access the influence of diet and nutritional status on infant developmental and health outcomes (Objective 3-3). Precision nutrition approaches will provide the most comprehensive and personalized answers to these objectives when high-quality data from relevant stages are analyzed using multivariate techniques.
Conclusion
Precision nutrition is an emerging branch of precision medicine. Among the many factors that influence early brain development, nutrition is a relatively modifiable factor. Tremendous opportunities are available to improve neurodevelopmental outcomes by optimizing the very origin of brain development (between conception to infancy), through modifying maternal nutrition (during pre-conception, pregnancy and lactation periods) and infant nutrition. We reviewed the current knowledge in this field, discussed opportunities from data science perspectives, and provided a catalog of major resources. We hope this survey and perspective study helps push nutritional studies for early life to a new height.
Author Contributions
BL, ET, RV, MS, IL, JL, and MK performed the literature reviews and contributed to the draft. SM, PG, and YO directed the review, acquired funding, and wrote the manuscript with input from all authors. All authors contributed to the article and approved the submitted version.
Funding
This study was supported by the Massachusetts Life Science Center Bits to Bytes Data Science Grant (SM, PG, and YO).
Conflict of Interest
JL and MK employees of Abbott Nutrition.
The remaining authors declare that the research was conducted in the absence of any commercial or financial relationships that could be construed as a potential conflict of interest.
Publisher’s Note
All claims expressed in this article are solely those of the authors and do not necessarily represent those of their affiliated organizations, or those of the publisher, the editors and the reviewers. Any product that may be evaluated in this article, or claim that may be made by its manufacturer, is not guaranteed or endorsed by the publisher.
Acknowledgments
We thank Ryan Larsen for his helpful discussion.
References
1. Cusick SE, Georgieff MK. The role of nutrition in brain development: the golden opportunity of the “First 1000 Days.”. J Pediatr. (2016) 175:16–21. doi: 10.1016/j.jpeds.2016.05.013
2. Silbereis JC, Pochareddy S, Zhu Y, Li M, Sestan N. The Cellular and Molecular Landscapes of the Developing Human Central Nervous System. Neuron. (2016) 89:248. doi: 10.1016/j.neuron.2015.12.008
3. Georgieff MK, Ramel SE, Cusick SE. Nutritional influences on brain development. Acta Paediatr Int J Paediatr. (2018) 107:1310–21. doi: 10.1111/apa.14287
4. National Academies of Sciences Engineering and Medicine, Health and Medicine Division, Board on Population Health and Public Health Practice. Brain Health Across the Life Span: Proceedings of a Workshop. Washington, DC: National Academies Press (2020). doi: 10.17226/25703
5. Tau GZ, Peterson BS. Normal development of brain circuits. Neuropsychopharmacology. (2010) 35:147–68. doi: 10.1038/npp.2009.115
6. Deoni S, Dean D, Joelson S, O’Regan J, Schneider N. Early nutrition influences developmental myelination and cognition in infants and young children. Neuroimage. (2018) 178:649–59. doi: 10.1016/j.neuroimage.2017.12.056
7. Peter RH. Synaptic density in human frontal cortex - Developmental changes and effects of aging. Brain Res. (1979) 163:195–205. doi: 10.1016/0006-8993(79)90349-4
8. Goyal MS, Iannotti LL, Raichle ME. Brain nutrition: a life span approach. Annu Rev Nutr. (2018) 38:381–99. doi: 10.1146/annurev-nutr-082117-051652
9. Morris SS, Cogill B, Uauy R. Effective international action against undernutrition: why has it proven so difficult and what can be done to accelerate progress? Lancet. (2008) 371:608–21. doi: 10.1016/S0140-6736(07)61695-X
10. The Lancet. Advancing Early Childhood Development: from Science to Scale An Executive Summary for The Lancet’s Series. (2016). Available online at: www.thelancet.com (Accessed December 2, 2020).
11. Abu-Saad K, Fraser D. Maternal nutrition and birth outcomes. Epidemiol Rev. (2010) 32:5–25. doi: 10.1093/epirev/mxq001
12. Graf WD, Kekatpure MV, Kosofsky BE. Prenatal-Onset Neurodevelopmental Disorders Secondary To Toxins, Nutritional Deficiencies, And Maternal Illness. 1st ed. Amsterdam: Elsevier (2013). doi: 10.1016/B978-0-444-52891-9.00014-2
13. Marques AH, O’Connor TG, Roth C, Susser E, Bjørke-Monsen AL. The influence of maternal prenatal and early childhood nutrition and maternal prenatal stress on offspring immune system development and neurodevelopmental disorders. Front Neurosci. (2013) 7:120. doi: 10.3389/fnins.2013.00120
14. Grieger JA, Grzeskowiak LE, Clifton VL. Preconception dietary patterns in human pregnancies are associated with preterm delivery. J Nutr. (2014) 144:1075–80. doi: 10.3945/jn.114.190686
15. Indrio F, Martini S, Francavilla R, Corvaglia L, Cristofori F, Mastrolia SA, et al. Epigenetic matters: the link between early nutrition, microbiome, and long-term health development. Front Pediatr. (2017) 5:178. doi: 10.3389/fped.2017.00178
16. Vohr BR, Davis EP, Wanke CA, Krebs NF. Neurodevelopment: the impact of nutrition and inflammation during preconception and pregnancy in low-resource settings. Pediatrics. (2017) 139:S38–49. doi: 10.1542/peds.2016-2828F
17. Stephenson J, Heslehurst N, Hall J, Schoenaker DAJM, Hutchinson J, Cade JE, et al. Before the beginning: nutrition and lifestyle in the preconception period and its importance for future health. Lancet. (2018) 391:1830–41. doi: 10.1016/S0140-6736(18)30311-8
18. Cheatham CL. Nutritional Factors in Fetal and Infant Brain Development. Ann Nutr Metab. (2020) 75:20–32. doi: 10.1159/000508052
19. Krebs NF, Lozoff B, Georgieff MK. Neurodevelopment?: the impact of nutrition and inflammation during infancy in low-resource settings. Pediatrics. (2020) 139:S50–8. doi: 10.1542/peds.2016-2828G
20. Fox SE, Levitt P, Nelson CA. How the timing and quality of early experiences influence the development of brain architecture. Child Dev. (2010) 81:28–40. doi: 10.1111/j.1467-8624.2009.01380.x
21. Schwarzenberg SJ, Georgieff MK. Advocacy for improving nutrition in the first 1000 days to support childhood development and adult health. Pediatrics. (2018) 141:e20173716. doi: 10.1542/peds.2017-3716
22. Barrero-Castillero A, Morton SU, Nelson CA, Smith VC. Psychosocial stress and adversity: effects from the perinatal period to adulthood. Neoreviews. (2019) 20:e686–96. doi: 10.1542/neo.20-12-e686
23. Belfort MB, Anderson PJ, Nowak VA, Lee KJ, Molesworth C, Thompson DK, et al. Breast milk feeding, brain development, and neurocognitive outcomes: A 7-year longitudinal study in infants born at less than 30 weeks’ gestation. J Pediatr. (2016) 177:133–9.e1. doi: 10.1016/j.jpeds.2016.06.045
24. Gill BD, Indyk HE, Woollard DC. Current methods for the analysis of selected novel nutrients in infant formulas and adult nutritionals. J AOAC Int. (2016) 99:30–41. doi: 10.5740/jaoacint.15-0247
25. Sullivan D. Modernization of AOAC nutrient methods by stakeholder panel on infant formula and adult nutritionals. J AOAC Int. (2016) 99:3–6. doi: 10.5740/jaoacint.15-0248
26. Cormack BE, Harding JE, Miller SP, Bloomfield FH. The influence of early nutrition on brain growth and neurodevelopment in extremely preterm babies: a narrative review. Nutrients. (2019) 11:2029. doi: 10.3390/nu11092029
27. Almaas AN, Tamnes CK, Nakstad B, Henriksen C, Walhovd KB, Fjell AM, et al. Long-Chain Polyunsaturated fatty acids and cognition in VLBW infants at 8 years: an RCT. Pediatrics. (2015) 135:972–80. doi: 10.1542/peds.2014-4094
28. Almaas AN, Tamnes CK, Nakstad B, Henriksen C, Grydeland H, Walhovd KB, et al. Diffusion tensor imaging and behavior in premature infants at 8 years of age, a randomized controlled trial with long-chain polyunsaturated fatty acids. Early Hum Dev. (2016) 95:41–6. doi: 10.1016/j.earlhumdev.2016.01.021
29. Strømmen K, Blakstad EW, Moltu SJ, Almaas AN, Westerberg AC, Amlien IK, et al. Enhanced nutrient supply to very low birth weight infants is associated with improved white matter maturation and head growth. Neonatology. (2015) 107:68–75. doi: 10.1159/000368181
30. Tam EWY, Chau V, Barkovich AJ, Ferriero DM, Miller SP, Rogers EE, et al. Early postnatal docosahexaenoic acid levels and improved preterm brain development. Pediatr Res. (2016) 79:723–30. doi: 10.1038/pr.2016.11
31. Beauport L, Schneider J, Faouzi M, Hagmann P, Hüppi PS, Tolsa JF, et al. Impact of early nutritional intake on preterm brain: a magnetic resonance imaging study. J Pediatr. (2017) 181:29–36.e1. doi: 10.1016/j.jpeds.2016.09.073
32. Schneider J, Fumeaux CJF, Duerden EG, Guo T, Foong J, Graz MB, et al. Nutrient intake in the first two weeks of life and brain growth in preterm neonates. Pediatrics. (2018) 141:e20172169. doi: 10.1542/peds.2017-2169
33. Ding W, Zhao L, Sheng N, Ma J, Zhang Y. Impact of neonatal intensive care unit oral feeding on neuropsychomotor outcomes at 9 months of corrected age in Chinese low-birthweight preterm infants: a retrospective study. J Clin Nurs. (2019) 28:420–9. doi: 10.1111/jocn.14537
34. Gidrewicz DA, Fenton TR. A systematic review and meta-analysis of the nutrient content of preterm and term breast milk. BMC Pediatr. (2014) 14:216. doi: 10.1186/1471-2431-14-216
35. Harding JE, Cormack BE, Alexander T, Alsweiler JM, Bloomfield FH. Advances in nutrition of the newborn infant. Lancet. (2017) 389:1660–8. doi: 10.1016/S0140-6736(17)30552-4
36. Barker M, Dombrowski SU, Colbourn T, Fall CHD, Kriznik NM, Lawrence WT, et al. Intervention strategies to improve nutrition and health behaviours before conception. Lancet. (2018) 391:1853–64. doi: 10.1016/S0140-6736(18)30313-1
37. Nguyen PH, Gonzalez-Casanova I, Young MF, Truong TV, Hoang H, Nguyen H, et al. Preconception micronutrient supplementation with iron and folic acid compared with folic acid alone affects linear growth and fine motor development at 2 years of age: a randomized controlled trial in Vietnam. J Nutr. (2017) 147:1593–601. doi: 10.3945/jn.117.250597
38. Di Liegro I. Thyroid hormones and the central nervous system of mammals (Review). Mol Med Rep. (2008) 1:279–95. doi: 10.3892/mmr.1.3.279
39. Robinson SM, Crozier SR, Miles EA, Gale CR, Calder PC, Cooper C, et al. Preconception maternal iodine status is positively associated with IQ but not with measures of executive function in childhood. J Nutr. (2018) 148:959–66. doi: 10.1093/jn/nxy054
40. Roige-Castellvi J, Murphy M, Fernandez-Ballart J, Canals J. Moderately elevated preconception fasting plasma total homocysteine is a risk factor for psychological problems in childhood. Public Health Nutr. (2019) 22:1615–23. doi: 10.1017/S1368980018003610
41. Morse NL. Benefits of docosahexaenoic acid, folic acid, vitamin D and iodine on foetal and infant brain development and function following maternal supplementation during pregnancy and lactation. Nutrients. (2012) 4:799–840. doi: 10.3390/nu4070799
42. McNulty H, Rollins M, Cassidy T, Caffrey A, Marshall B, Dornan J, et al. Effect of continued folic acid supplementation beyond the first trimester of pregnancy on cognitive performance in the child: a follow-up study from a randomized controlled trial (FASSTT Offspring Trial). BMC Med. (2019) 17:196. doi: 10.1186/s12916-019-1432-4
43. Ars CL, Nijs IM, Marroun HE, Muetzel R, Schmidt M, Steenweg-De Graaff J, et al. Prenatal folate, homocysteine and Vitamin B12 levels and child brain volumes, cognitive development and psychological functioning: the generation R study. Br J Nutr. (2019) 122:S1–9. doi: 10.1017/S0007114515002081
44. Wiens D, Desoto MC. Is high folic acid intake a risk factor for autism?—a review. Brain Sci. (2017) 7:149. doi: 10.3390/brainsci7110149
45. Jaime-Perez JC, Herrera-Garza JL, Gomez-Almaguer D. Sub-optimal fetal iron acquisition under a maternal environment. Arch Med Res. (2005) 36:598–602. doi: 10.1016/j.arcmed.2005.03.023
46. Georgieff MK, Wewerka SW, Nelson CA, DeRegnier RA. Iron status at 9 months of infants with low iron stores at birth. J Pediatr. (2002) 141:405–9. doi: 10.1067/mpd.2002.127090
47. Tamura T, Goldenberg RL, Hou J, Johnston KE, Cliver SP, Ramey SL, et al. Cord serum ferritin concentrations and mental and psychomotor development of children at five years of age. J Pediatr. (2002) 140:165–70. doi: 10.1067/mpd.2002.120688
48. Algarín C, Nelson CA, Peirano P, Westerlund A, Reyes S, Lozoff B. Iron-deficiency anemia in infancy and poorer cognitive inhibitory control at age 10 years. Dev Med Child Neurol. (2013) 55:453–8. doi: 10.1111/dmcn.12118
49. Corapci F, Calatroni A, Kaciroti N, Jimenez E, Lozoff B. Longitudinal evaluation of externalizing and internalizing behavior problems following iron deficiency in infancy. J Pediatr Psychol. (2010) 35:296–305. doi: 10.1093/jpepsy/jsp065
50. Lukowski AF, Koss M, Burden MJ, Jonides J, Nelson CA, Kaciroti N, et al. Iron deficiency in infancy and neurocognitive functioning at 19 years: evidence of long-term deficits in executive function and recognition memory. Nutr Neurosci. (2010) 13:54–70. doi: 10.1179/147683010X12611460763689
51. Puig-Domingo M, Vila L. The Implications of Iodine and its Supplementation During Pregnancy in Fetal Brain Development. Curr Clin Pharmacol. (2013) 8:97–109. doi: 10.2174/1574884711308020002
52. Markhus MW, Dahl L, Moe V, Abel MH, Brantsæter AL, Øyen J, et al. Maternal iodine status is associated with offspring language skills in infancy and toddlerhood. Nutrients. (2018) 10:1270. doi: 10.3390/nu10091270
53. Georgieff MK, Brunette KE, Tran PV. Early life nutrition and neural plasticity. Dev Psychopathol. (2015) 27:411–23. doi: 10.1017/S0954579415000061
54. Gogia S, Sachdev HS. Zinc supplementation for mental and motor development in children. Cochrane Database Syst Rev. (2012) 12:CD007991. doi: 10.1002/14651858.cd007991.pub2
55. Korsmo HW, Jiang X, Caudill MA. Choline: exploring the growing science on its benefits for moms and babies. Nutrients. (2019) 11:1823. doi: 10.3390/nu11081823
56. Catena A, Angela Muñoz-Machicao J, Torres-Espínola FJ, Martínez-Zaldívar C, Diaz-Piedra C, Gil A, et al. Folate and long-chain polyunsaturated fatty acid supplementation during pregnancy has long-term effects on the attention system of 8.5-y-old offspring: a randomized controlled trial. Am J Clin Nutr. (2016) 103:115–27. doi: 10.3945/ajcn.115.109108
57. Zimmerman MS, Smith AGC, Sable CA, Echko MM, Wilner LB, Olsen HE, et al. Global, regional, and national burden of congenital heart disease, 1990–2017: a systematic analysis for the Global Burden of Disease Study 2017. Lancet Child Adolesc Health. (2020) 4:185–200. doi: 10.1016/S2352-4642(19)30402-X
58. Velasco I, Carreira M, Santiago P, Muela JA, García-Fuentes E, Sánchez-Muñoz B, et al. Effect of iodine prophylaxis during pregnancy on neurocognitive development of children during the first two years of life. J Clin Endocrinol Metab. (2009) 94:3234–41. doi: 10.1210/jc.2008-2652
59. Derbyshire E, Obeid R. Choline, neurological development and brain function: a systematic review focusing on the first 1000 days. Nutrients. (2020) 12:1731. doi: 10.3390/nu12061731
60. Meldrum SJ, D’Vaz N, Simmer K, Dunstan JA, Hird K, Prescott SL. Effects of high-dose fish oil supplementation during early infancy on neurodevelopment and language: a randomised controlled trial. Br J Nutr. (2012) 108:1443–54. doi: 10.1017/S0007114511006878
61. Morton SU, Vyas R, Gagoski B, Vu C, Litt J, Larsen RJ, et al. Maternal Dietary Intake of Omega-3 Fatty Acids Correlates Positively with Regional Brain Volumes in 1-Month-Old Term Infants. Cereb Cortex. (2020) 30:2057–69. doi: 10.1093/cercor/bhz222
62. Belfort MB, Woodward LJ, Cherkerzian S, Pepin H, Ellard D, Steele T, et al. Targeting human milk fortification to improve very preterm infant growth and brain development: study protocol for Nourish, a single-center randomized, controlled clinical trial. BMC Pediatr. (2021) 21:167. doi: 10.1186/s12887-021-02635-x
63. Isaacs EB, Fischl BR, Quinn BT, Chong WK, Gadian DG, Lucas A. Impact of breast milk on intelligence quotient, brain size, and white matter development. Pediatr. Res. (2010) 67:357–62. doi: 10.1203/PDR.0b013e3181d026da
64. Kafouri S, Kramer M, Leonard G, Perron M, Pike B, Richer L, et al. Breastfeeding and brain structure in adolescence. Int J Epidemiol. (2013) 42:150–9. doi: 10.1093/ije/dys172
65. Luby JL, Belden AC, Whalen D, Harms MP, Barch DM. Breastfeeding and childhood IQ: the mediating role of gray matter volume. J Am Acad Child Adolesc Psychiatry. (2016) 55:367–75. doi: 10.1016/j.jaac.2016.02.009
66. Ou X, Andres A, Cleves MA, Pivik RT, Snow JH, Ding Z, et al. Sex-specific association between infant diet and white matter integrity in 8-y-old children. Pediatr Res. (2014) 76:535–43. doi: 10.1038/pr.2014.129
67. Ou X, Andres A, Pivik RT, Cleves MA, Snow JH, Ding Z, et al. Voxel-based morphometry and fMRI revealed differences in brain gray matter in breastfed and milk formula-fed children. Am J Neuroradiol. (2016) 37:713–9. doi: 10.3174/ajnr.A4593
68. Deoni SCL, Rutt BK, Arun T, Pierpaoli C, Jones DK. Gleaning multicomponent T1 and T2 information from steady-state imaging data. Magn Reson Med. (2008) 60:1372–87. doi: 10.1002/mrm.21704
69. Deoni SCL, Dean DC, Piryatinsky I, O’Muircheartaigh J, Waskiewicz N, Lehman K, et al. Breastfeeding and early white matter development: a cross-sectional study. Neuroimage. (2013) 82:77–86. doi: 10.1016/j.neuroimage.2013.05.090
70. Hallowell SG, Spatz DL. The relationship of brain development and breastfeeding in the late-preterm infant. J Pediatr Nurs. (2012) 27:154–62. doi: 10.1016/j.pedn.2010.12.018
71. Knudsen EI. Sensitive periods in the development of the brain and behavior. J Cogn Neurosci. (2004) 16:1412–25. doi: 10.1162/0898929042304796
72. Chaudry A, Wimer C. Poverty is not just an indicator: the relationship between income Poverty, and Child Well-Being. Acad Pediatr. (2016) 16:S23–9. doi: 10.1016/j.acap.2015.12.010
73. Braun K, Champagne FA. Paternal influences on offspring development: behavioural and epigenetic pathways. J Neuroendocrinol. (2014) 26:697–706. doi: 10.1111/jne.12174
74. Walker SP, Wachs TD, Grantham-Mcgregor S, Black MM, Nelson CA, Huffman SL, et al. Inequality in early childhood: risk and protective factors for early child development. Lancet. (2011) 378:1325–38. doi: 10.1016/S0140-6736(11)60555-2
75. Buss C, Entringer S, Moog NK, Toepfer P, Fair DA, Simhan HN, et al. Intergenerational transmission of maternal childhood maltreatment exposure: implications for fetal brain development. J Am Acad Child Adolesc Psychiatry. (2017) 56:373–82. doi: 10.1016/j.jaac.2017.03.001
76. Moog NK, Entringer S, Rasmussen JM, Styner M, Gilmore JH, Kathmann N, et al. Intergenerational Effect of Maternal Exposure to Childhood Maltreatment on Newborn Brain Anatomy. Biol Psychiatry. (2018) 83:120–7. doi: 10.1016/j.biopsych.2017.07.009
77. Provençal N, Binder EB. The effects of early life stress on the epigenome: from the womb to adulthood and even before. Exp Neurol. (2015) 268:10–20. doi: 10.1016/j.expneurol.2014.09.001
78. Braun JM. Early-life exposure to EDCs: role in childhood obesity and neurodevelopment. Nat Rev Endocrinol. (2017) 13:161–73. doi: 10.1038/nrendo.2016.186
79. Garner AS, Shonkoff JP, Siegel BS, Dobbins MI, Earls MF, Garner AS, et al. Early Childhood Adversity, Toxic Stress, and the Role of the Pediatrician: translating Developmental Science Into Lifelong Health. Pediatrics. (2012) 129:e224–31. doi: 10.1542/peds.2011-2662
80. Blair C, Raver CC. Poverty, stress, and brain development: new directions for prevention and intervention. Acad Pediatr. (2016) 16:S30–6. doi: 10.1016/j.acap.2016.01.010
81. Berens AE, Jensen SKG, Nelson CA. Biological embedding of childhood adversity: from physiological mechanisms to clinical implications. BMC Med. (2017) 15:135. doi: 10.1186/s12916-017-0895-4
82. Dzwilewski KLC, Schantz SL. Prenatal chemical exposures and child language development. J Commun Disord. (2015) 57:41–65. doi: 10.1016/j.jcomdis.2015.07.002
83. Borre YE, O’Keeffe GW, Clarke G, Stanton C, Dinan TG, Cryan JF. Microbiota and neurodevelopmental windows: implications for brain disorders. Trends Mol Med. (2014) 20:509–18. doi: 10.1016/j.molmed.2014.05.002
84. Krebs NF, Lozoff B, Georgieff MK. Neurodevelopment: the impact of nutrition and inflammation during infancy in low-resource settings. Pediatrics. (2017) 139:S50–8. doi: 10.1542/peds.2016-2828G
85. Nemeth E, Ganz T. Anemia of inflammation. Hematol Oncol Clin North Am. (2014) 28:671–81. doi: 10.1016/j.hoc.2014.04.005
86. Chaparro CM, Suchdev PS. Anemia epidemiology, pathophysiology, and etiology in low- and middle-income countries. Ann N Y Acad Sci. (2019) 1450:15–31. doi: 10.1111/nyas.14092
87. Miranda A, Sousa N. Maternal hormonal milieu influence on fetal brain development. Brain Behav. (2018) 8:e00920. doi: 10.1002/brb3.920
88. Haddow JE, Palomaki GE, Allan WC, Williams JR, Knight GJ, Gagnon J, et al. Maternal Thyroid Deficiency during Pregnancy and Subsequent Neuropsychological Development of the Child. N Engl J Med. (1999) 341:549–55. doi: 10.1056/nejm199908193410801
89. Devarshi PP, Grant RW, Ikonte CJ, Mitmesser SH. Maternal omega-3 nutrition, placental transfer and fetal brain development in gestational diabetes and preeclampsia. Nutrients. (2019) 11:1107. doi: 10.3390/nu11051107
90. Homberg JR, Kyzar EJ, Scattoni ML, Norton WH, Pittman J, Gaikwad S, et al. Genetic and environmental modulation of neurodevelopmental disorders: translational insights from labs to beds. Brain Res Bull. (2016) 125:79–91. doi: 10.1016/j.brainresbull.2016.04.015
91. Torres-Espínola FJ, Altmäe S, Segura MT, Jerez A, Anjos T, Chisaguano M, et al. Maternal PPARG Pro12Ala polymorphism is associated with infant’s neurodevelopmental outcomes at 18months of age. Early Hum Dev. (2015) 91:457–62. doi: 10.1016/j.earlhumdev.2015.05.001
92. Ng S, Lin CC, Hwang YH, Hsieh WS, Liao HF, Chen PC. Mercury, APOE, and children’s neurodevelopment. Neurotoxicology. (2013) 37:85–92. doi: 10.1016/j.neuro.2013.03.012
93. Wahlberg K, Love TM, Pineda D, Engström K, Watson GE, Thurston SW, et al. Maternal polymorphisms in glutathione-related genes are associated with maternal mercury concentrations and early child neurodevelopment in a population with a fi sh-rich diet. Environ Int. (2018) 115:142–9. doi: 10.1016/j.envint.2018.03.015
94. Lee M, Ha M, Hong YC, Park H, Kim Y, Kim EJ, et al. Exposure to prenatal secondhand smoke and early neurodevelopment: mothers and children’s environmental health (MOCEH) study. Environ Health. (2019) 18:22. doi: 10.1186/s12940-019-0463-9
95. Innis SM. Impact of maternal diet on human milk composition and neurological development of infants. Am J Clin Nutr. (2014) 99:734S–41S. doi: 10.3945/ajcn.113.072595
96. McLeod ER, Campbell KJ, Hesketh KD. Nutrition knowledge: a mediator between socioeconomic position and diet quality in Australian first-time mothers. J Am Diet Assoc. (2011) 111:696–704. doi: 10.1016/j.jada.2011.02.011
97. Maddock J, Wulaningsih W, Fernandez JC, Ploubidis GB, Goodman A, Bell J, et al. Associations between body size, nutrition and socioeconomic position in early life and the epigenome: a systematic review. PLoS One. (2018) 13:e0201672. doi: 10.1371/journal.pone.0201672
98. Collins FS, Varmus H. A New Initiative on Precision Medicine. N Engl J Med. (2015) 372:793–5. doi: 10.1056/nejmp1500523
99. National Institutes of Health [NIH]. 2020-2030 Strategic Plan for NIH Nutrition Research | NIDDK. (2020). Available online at: https://www.niddk.nih.gov/about-niddk/strategic-plans-reports/strategic-plan-nih-nutrition-research (Accessed December 2, 2020).
100. Sizonenko SV, Babiloni C, De Bruin EA, Isaacs EB, Jönsson LS, Kennedy DO, et al. Brain imaging and human nutrition: which measures to use in intervention studies? Br J Nutr. (2013) 110:S1–30. doi: 10.1017/S0007114513001384
101. Allison DB, Bassaganya-Riera J, Burlingame B, Brown AW, le Coutre J, Dickson SL, et al. Goals in Nutrition Science 2015–2020. Front Nutr. (2015) 2:26. doi: 10.3389/fnut.2015.00026
102. Moore K, Hughes CF, Ward M, Hoey L, McNulty H. Diet, nutrition and the ageing brain: current evidence and new directions. Proc Nutr Soc. (2018) 77:152–63. doi: 10.1017/S0029665117004177
103. Tanaka K, Kon N, Ohkawa N, Yoshikawa N, Shimizu T. Does breastfeeding in the neonatal period influence the cognitive function of very-low-birth-weight infants at 5 years of age? Brain Dev. (2009) 31:288–93. doi: 10.1016/j.braindev.2008.05.011
104. Meldrum S, Simmer K. Docosahexaenoic acid and neurodevelopmental outcomes of term infants. Ann Nutr Metab. (2016) 69:23–8. doi: 10.1159/000448271
105. Ramakrishnan U, Gonzalez-Casanova I, Schnaas L, DiGirolamo A, Quezada AD, Pallo BC, et al. Prenatal supplementation with DHA improves attention at 5 y of age: a randomized controlled trial. Am J Clin Nutr. (2016) 104:1075–82. doi: 10.3945/ajcn.114.101071
106. Agostoni C, Nobile M, Ciappolino V, Delvecchio G, Tesei A, Turolo S, et al. The role of omega-3 fatty acids in developmental psychopathology: a systematic review on early psychosis, autism, and ADHD. Int J Mol Sci. (2017) 18:2608. doi: 10.3390/ijms18122608
107. Devlin A, Chau C, Dyer R, Matheson J, McCarthy D, Yurko-Mauro K, et al. Developmental outcomes at 24 months of age in toddlers supplemented with arachidonic acid and docosahexaenoic acid: results of a double blind randomized controlled trial. Nutrients. (2017) 9:975. doi: 10.3390/nu9090975
108. Ottolini KM, Andescavage N, Keller S, Limperopoulos C. Nutrition and the developing brain: the road to optimizing early neurodevelopment: a systematic review. Pediatr Res. (2020) 87:194–201. doi: 10.1038/s41390-019-0508-3
109. Coviello C, Keunen K, Kersbergen KJ, Groenendaal F, Leemans A, Peels B, et al. Effects of early nutrition and growth on brain volumes, white matter microstructure, and neurodevelopmental outcome in preterm newborns. Pediatr Res. (2018) 83:102–10. doi: 10.1038/pr.2017.227
110. Croll PH, Voortman T, Ikram MA, Franco OH, Schoufour JD, Bos D, et al. Better diet quality relates to larger brain tissue volumes. Neurology. (2018) 90:e2155–65. doi: 10.1212/WNL.0000000000005691
111. Power VA, Spittle AJ, Lee KJ, Anderson PJ, Thompson DK, Doyle LW, et al. Nutrition, Growth, Brain Volume, and Neurodevelopment in Very Preterm Children. J Pediatr. (2019) 215:50–5.e3. doi: 10.1016/j.jpeds.2019.08.031
112. van Beek PE, Claessens NHP, Makropoulos A, Groenendaal F, de Vries LS, Counsell SJ, et al. Increase in Brain Volumes after Implementation of a Nutrition Regimen in Infants Born Extremely Preterm. J Pediatr. (2020) 223:57–63.e5. doi: 10.1016/j.jpeds.2020.04.063
113. Bernardoni F, King JA, Geisler D, Birkenstock J, Tam FI, Weidner K, et al. Nutritional status affects cortical folding: lessons learned from anorexia nervosa. Biol Psychiatry. (2018) 84:692–701. doi: 10.1016/j.biopsych.2018.05.008
114. Cascino G, Canna A, Monteleone AM, Russo AG, Prinster A, Aiello M, et al. Cortical thickness, local gyrification index and fractal dimensionality in people with acute and recovered Anorexia Nervosa and in people with Bulimia Nervosa. Psychiatry Res Neuroimaging. (2020) 299:111069. doi: 10.1016/j.pscychresns.2020.111069
115. Schwarz C, Horn N, Benson G, Wrachtrup Calzado I, Wurdack K, Pechlaner R, et al. Spermidine intake is associated with cortical thickness and hippocampal volume in older adults. Neuroimage. (2020) 221:117132. doi: 10.1016/j.neuroimage.2020.117132
116. Ghassaban K, Liu S, Jiang C, Haacke EM. Quantifying iron content in magnetic resonance imaging. Neuroimage. (2019) 187:77–92. doi: 10.1016/j.neuroimage.2018.04.047
117. Lehéricy S, Roze E, Goizet C, Mochel F. MRI of neurodegeneration with brain iron accumulation. Curr Opin Neurol. (2020) 33:462–73. doi: 10.1097/WCO.0000000000000844
118. Shahmaei V, Faeghi F, Mohammdbeigi A, Hashemi H, Ashrafi F. Evaluation of iron deposition in brain basal ganglia of patients with Parkinson’s disease using quantitative susceptibility mapping. Eur J Radiol Open. (2019) 6:169–74. doi: 10.1016/j.ejro.2019.04.005
119. Ravanfar P, Loi SM, Syeda WT, Van Rheenen TE, Bush AI, Desmond P, et al. Systematic review: quantitative susceptibility mapping (QSM) of brain iron profile in neurodegenerative diseases. Front Neurosci. (2021) 15:618435. doi: 10.3389/fnins.2021.618435
120. Blesa M, Sullivan G, Anblagan D, Telford EJ, Quigley AJ, Sparrow SA, et al. Early breast milk exposure modifies brain connectivity in preterm infants. Neuroimage. (2019) 184:431–9. doi: 10.1016/j.neuroimage.2018.09.045
121. Inoue T, Kozawa E, Ishikawa M, Okada H. Application of magnetic resonance imaging in the evaluation of nutritional status: a literature review with focus on dialysis patients. Nutrients. (2021) 13:2037. doi: 10.3390/nu13062037
122. Akitsuki Y, Nakawaga S, Sugiura M, Kawashima R. Nutritional quality of breakfast affects cognitive function: an fMRI study. Neurosci Med. (2011) 02:192–7. doi: 10.4236/nm.2011.23026
123. Fulford J, Varley-Campbell JL, Williams CA. The effect of breakfast versus no breakfast on brain activity in adolescents when performing cognitive tasks, as assessed by fMRI. Nutr Neurosci. (2016) 19:110–5. doi: 10.1179/1476830515Y.0000000011
124. Coquery N, Meurice P, Janvier R, Bobillier E, Quellec S, Fu M, et al. fMRI-based brain responses to quinine and sucrose gustatory stimulation for nutrition research in the minipig model: a proof-of-concept study. Front Behav Neurosci. (2018) 12:151. doi: 10.3389/fnbeh.2018.00151
125. Zwilling CE, Talukdar T, Zamroziewicz MK, Barbey AK. Nutrient biomarker patterns, cognitive function, and fMRI measures of network efficiency in the aging brain. Neuroimage. (2019) 188:239–51. doi: 10.1016/j.neuroimage.2018.12.007
126. Yazigi Solis M, De Salles Painelli V, Artioli GG, Roschel H, Otaduy MC, Gualano B. Brain creatine depletion in vegetarians? A cross-sectional 1H-magnetic resonance spectroscopy (1H-MRS) study. Br J Nutr. (2014) 111:1272–4. doi: 10.1017/S0007114513003802
127. Liu N, Yu P. Recent research and progress in food, feed and nutrition with advanced synchrotron-based SR-IMS and DRIFT molecular spectroscopy. Crit Rev Food Sci Nutr. (2016) 56:910–8. doi: 10.1080/10408398.2012.733895
128. Scavuzzo CJ, Moulton CJ, Larsen RJ. The use of magnetic resonance spectroscopy for assessing the effect of diet on cognition. Nutr Neurosci. (2018) 21:1–15. doi: 10.1080/1028415X.2016.1218191
129. Larsen RJ, Gagoski B, Morton SU, Ou Y, Vyas R, Litt J, et al. Quantification of magnetic resonance spectroscopy data using a combined reference: application in typically developing infants. NMR Biomed. (2021) 34:e4520. doi: 10.1002/nbm.4520
130. Galler JR, Bringas-Vega ML, Tang Q, Rabinowitz AG, Musa KI, Chai WJ, et al. Neurodevelopmental effects of childhood malnutrition: a neuroimaging perspective. Neuroimage. (2021) 231:117828. doi: 10.1016/j.neuroimage.2021.117828
131. Bartel PR, Griesel RD, Freiman I, Rosen EU, Geefhuysen J. Long-term effects of kwashiorkor on the electroencephalogram. Am J Clin Nutr. (1979) 32:753–7. doi: 10.1093/ajcn/32.4.753
132. Rajanna B, Mascarenhas C, Desiraju T. Deviations in brain development due to caloric undernutrition and scope of their prevention by rehabilitation: alterations in the power spectra of the EEG of areas of the neocortex and limbic system. Dev Brain Res. (1987) 37:97–113. doi: 10.1016/0165-3806(87)90232-X
133. Taboada-Crispi A, Bringas-Vega ML, Bosch-Bayard J, Galán-García L, Bryce C, Rabinowitz AG, et al. Quantitative EEG tomography of early childhood malnutrition. Front Neurosci. (2018) 12:595. doi: 10.3389/fnins.2018.00595
134. Bringas Vega ML, Guo Y, Tang Q, Razzaq FA, Calzada Reyes A, Ren P, et al. An age-adjusted EEG source classifier accurately detects school-aged barbadian children that had protein energy malnutrition in the first year of life. Front Neurosci. (2019) 13:1222. doi: 10.3389/fnins.2019.01222
135. Pivik RT, Andres A, Tennal KB, Gu Y, Downs H, Bellando BJ, et al. Resting gamma power during the postnatal critical period for GABAergic system development is modulated by infant diet and sex. Int J Psychophysiol. (2019) 135:73–94. doi: 10.1016/j.ijpsycho.2018.11.004
136. Jing H, Gilchrist JM, Badger TM, Pivik RT. A longitudinal study of differences in electroencephalographic activity among breastfed, milk formula-fed, and soy formula-fed infants during the first year of life. Early Hum Dev. (2010) 86:119–25. doi: 10.1016/j.earlhumdev.2010.02.001
137. Beatty S, Nolan J, Kavanagh H, O’Donovan O. Macular pigment optical density and its relationship with serum and dietary levels of lutein and zeaxanthin. Arch Biochem Biophys. (2004) 430:70–6. doi: 10.1016/j.abb.2004.03.015
138. Favé G, Beckmann ME, Draper JH, Mathers JC. Measurement of dietary exposure: a challenging problem which may be overcome thanks to metabolomics? Genes Nutr. (2009) 4:135–41. doi: 10.1007/s12263-009-0120-y
139. Belfort MB, Rifas-Shiman SL, Sullivan T, Collins CT, McPhee AJ, Ryan P, et al. Infant growth before and after term: effects on neurodevelopment in preterm infants. Pediatrics. (2011) 128:e899–906. doi: 10.1542/peds.2011-0282
140. Sammallahti S, Pyhälä R, Lahti M, Lahti J, Pesonen AK, Heinonen K, et al. Infant growth after preterm birth and neurocognitive abilities in young adulthood. J Pediatr. (2014) 165:1109–15.e3. doi: 10.1016/j.jpeds.2014.08.028
141. Neubauer V, Fuchs T, Griesmaier E, Kager K, Pupp-Peglow U, Kiechl-Kohlendorfer U. Poor postdischarge head growth is related to a 10% lower intelligence quotient in very preterm infants at the chronological age of five years. Acta Paediatr Int J Paediatr. (2016) 105:501–7. doi: 10.1111/apa.13336
142. O’Gorman A, Brennan L. The role of metabolomics in determination of new dietary biomarkers. Proc Nutr Soc. (2017) 76:295–302. doi: 10.1017/S0029665116002974
143. Rudolph MC, Young BE, Lemas DJ, Palmer CE, Hernandez TL, Barbour LA, et al. Early infant adipose deposition is positively associated with the n-6 to n-3 fatty acid ratio in human milk independent of maternal BMI. Int J Obes. (2017) 41:510–7. doi: 10.1038/ijo.2016.211
144. Gridneva Z, Rea A, Hepworth AR, Ward LC, Lai CT, Hartmann PE, et al. Relationships between breastfeeding patterns and maternal and infant body composition over the first 12 months of lactation. Nutrients. (2018) 10:45. doi: 10.3390/nu10010045
145. Al-Hassan A, Vyas R, Zhang Y, Sisitsky M, Gagoski B, Litt JS, et al. Assessment of maternal macular pigment optical density (MPOD) as a potential marker for dietary carotenoid intake during lactation in humans. Nutrients. (2022) 14:182. doi: 10.3390/nu14010182
146. Bell KA, Matthews LG, Cherkerzian S, Palmer C, Drouin K, Pepin HL, et al. Associations of growth and body composition with brain size in preterm infants. J Pediatr. (2019) 214:20–6.e2. doi: 10.1016/j.jpeds.2019.06.062
147. Andrews ET, Beattie RM, Johnson MJ. Measuring body composition in the preterm infant: evidence base and practicalities. Clin Nutr. (2019) 38:2521–30. doi: 10.1016/j.clnu.2018.12.033
148. Pfister KM, Zhang L, Miller NC, Ingolfsland EC, Demerath EW, Ramel SE. Early body composition changes are associated with neurodevelopmental and metabolic outcomes at 4 years of age in very preterm infants. Pediatr Res. (2018) 84:713–8. doi: 10.1038/s41390-018-0158-x
149. Hammond BR, Stephen Miller L, Bello MO, Lindbergh CA, Mewborn C, Renzi-Hammond LM. Effects of lutein/zeaxanthin supplementation on the cognitive function of community dwelling older adults: a randomized, double-masked, placebo-controlled trial. Front Aging Neurosci. (2017) 9:254. doi: 10.3389/fnagi.2017.00254
150. Renzi-Hammond LM, Bovier ER, Fletcher LM, Miller LS, Mewborn CM, Lindbergh CA, et al. Effects of a lutein and zeaxanthin intervention on cognitive function: a randomized, double-masked, placebo-controlled trial of younger healthy adults. Nutrients. (2017) 9:1246. doi: 10.3390/nu9111246
151. Sasano H, Obana A, Sharifzadeh M, Bernstein PS, Okazaki S, Gohto Y, et al. Optical detection of macular pigment formation in premature infants. Transl Vis Sci Technol. (2018) 7:3. doi: 10.1167/tvst.7.4.3
152. Henriksen BS, Chan G, Hoffman RO, Sharifzadeh M, Ermakov IV, Gellermann W, et al. Interrelationships between maternal carotenoid status and newborn infant macular pigment optical density and carotenoid status. Investig Ophthalmol Vis Sci. (2013) 54:5568–78. doi: 10.1167/iovs.13-12331
153. Sherry CL, Oliver JS, Renzi LM, Marriage BJ. Lutein supplementation increases breast milk and plasma lutein concentrations in lactating women and infant plasma concentrations but does not affect other carotenoids. J Nutr. (2014) 144:1256–63. doi: 10.3945/jn.114.192914
154. Liu Z, Meyers KJ, Johnson EJ, Snodderly M, Tinker L, Wallace R, et al. Exposure to lutein in infancy via breast milk and later life macular pigment optical density. Investig Ophthalmol Vis Sci. (2015) 56:192.
155. Barnett SM, Khan NA, Walk AM, Raine LB, Moulton C, Cohen NJ, et al. Macular pigment optical density is positively associated with academic performance among preadolescent children. Nutr Neurosci. (2018) 21:632–40. doi: 10.1080/1028415X.2017.1329976
156. Block G, Hartman AM, Dresser CM, Carroll MD, Gannon J, Gardner L. A data-based approach to diet questionnaire design and testing. Am J Epidemiol. (1986) 124:453–69. doi: 10.1093/oxfordjournals.aje.a114416
157. Willett WC, Reynolds RD, Cottrell-Hoehner S, Sampson L, Browne ML. Validation of a semi-quantitative food frequency questionnaire: comparison with a 1-year diet record. J Am Diet Assoc. (1987) 87:43–7.
158. Cade J, Thompson R, Burley V, Warm D. Development, validation and utilisation of food-frequency questionnaires – a review. Public Health Nutr. (2002) 5:567–87. doi: 10.1079/phn2001318
159. Mulligan AA, Luben RN, Bhaniani A, Parry-Smith DJ, O’Connor L, Khawaja AP, et al. A new tool for converting food frequency questionnaire data into nutrient and food group values: FETA research methods and availability. BMJ Open. (2014) 4:e004503. doi: 10.1136/bmjopen-2013-004503
160. Coathup V, Wheeler S, Smith L. A method comparison of a food frequency questionnaire to measure folate, choline, betaine, Vitamin C and carotenoids with 24-h dietary recalls in women of reproductive age. Eur J Clin Nutr. (2016) 70:346–51. doi: 10.1038/ejcn.2015.159
161. Archer E, Marlow ML, Lavie CJ. Controversy and debate: memory-Based Methods Paper 1: the fatal flaws of food frequency questionnaires and other memory-based dietary assessment methods. J Clin Epidemiol. (2018) 104:113–24. doi: 10.1016/j.jclinepi.2018.08.003
162. Mun JG, Legette LL, Ikonte CJ, Mitmesser SH. Choline and DHA in maternal and infant nutrition: synergistic implications in brain and eye health. Nutrients. (2019) 11:1125. doi: 10.3390/nu11051125
163. Saraya AK, Choudhry VP, Ghai OP. Interrelationships of vitamin B 12, folic acid, and iron in anemia of infancy and childhood: effect of vitamin B 12 and iron therapy on folate metabolism. Am J Clin Nutr. (1973) 26:640–6. doi: 10.1093/ajcn/26.6.640
164. Bastian M, Heymann S, Jacomy M. Gephi?: an open source software for exploring and manipulating networks visualization and exploration of large graphs. Proc Int AAAI Conf Web Soc Media. (2009) 3:361–2.
165. Mohn ES, Kuchan MJ, Erdman JW, Neuringer M, Matthan NR, Oliver Chen CY, et al. The subcellular distribution of alpha-tocopherol in the adult primate brain and its relationship with membrane arachidonic acid and its oxidation products. Antioxidants. (2017) 6:97. doi: 10.3390/antiox6040097
166. Mohn ES, Erdman JW, Kuchan MJ, Neuringer M, Johnson EJ. Lutein accumulates in subcellular membranes of brain regions in adult rhesus macaques: relationship to DHA oxidation products. PLoS One. (2017) 12:e0186767. doi: 10.1371/journal.pone.0186767
167. Gómez-Pinilla F. Brain foods: the effects of nutrients on brain function. Nat Rev Neurosci. (2008) 9:568–78. doi: 10.1038/nrn2421
168. Rodgers GP, Collins FS. Precision nutrition-the answer to “what to eat to stay healthy.”. JAMA. (2020) 324:735–6. doi: 10.1001/jama.2020.13601
169. Ferguson LR, De Caterina R, Görman U, Allayee H, Kohlmeier M, Prasad C, et al. Guide and position of the International society of nutrigenetics/Nutrigenomics on personalised nutrition: part 1 - fields of precision nutrition. J Nutrigenet Nutrigenomics. (2016) 9:12–27. doi: 10.1159/000445350
170. de Toro-Martín J, Arsenault BJ, Després JP, Vohl MC. Precision nutrition: a review of personalized nutritional approaches for the prevention and management of metabolic syndrome. Nutrients. (2017) 9:913. doi: 10.3390/nu9080913
171. Wang DD, Hu FB. Precision nutrition for prevention and management of type 2 diabetes. Lancet Diabetes Endocrinol. (2018) 6:416–26. doi: 10.1016/S2213-8587(18)30037-8
172. Larsen PS, Kamper-Jørgensen M, Adamson A, Barros H, Bonde JP, Brescianini S, et al. Pregnancy and birth cohort resources in Europe: a large opportunity for aetiological child health research. Paediatr Perinat Epidemiol. (2013) 27:393–414. doi: 10.1111/ppe.12060
173. Pandolfini C, Ricci C, Siziba LP, Huhn S, Genuneit J, Bonati M. Intrauterine exposures and maternal health status during pregnancy in relation to later child health: a review of pregnancy cohort studies in Europe. Int J Environ Res Public Health. (2021) 18:7702. doi: 10.3390/ijerph18147702
174. Poldrack RA, Gorgolewski KJ. Making big data open: data sharing in neuroimaging. Nat Neurosci. (2014) 17:1510–7. doi: 10.1038/nn.3818
175. Feng X, Lipton ZC, Yang J, Small SA, Provenzano FA. Estimating brain age based on a uniform healthy population with deep learning and structural magnetic resonance imaging. Neurobiol Aging. (2020) 91:15–25. doi: 10.1016/j.neurobiolaging.2020.02.009
176. Pomponio R, Erus G, Habes M, Doshi J, Srinivasan D, Mamourian E, et al. Harmonization of large MRI datasets for the analysis of brain imaging patterns throughout the lifespan. Neuroimage (2020) 208:116450. doi: 10.1016/j.neuroimage.2019.116450
177. Volkow ND, Gordon JA, Freund MP. The Healthy Brain and Child Development Study - Shedding Light on Opioid Exposure, COVID-19, and Health Disparities. JAMA Psychiatry (2021) 78:471–2. doi: 10.1001/jamapsychiatry.2020.3803
178. Oishi K, Mori S, Donohue PK, Ernst T, Anderson L, Buchthal S, et al. Multi-contrast human neonatal brain atlas: application to normal neonate development analysis. Neuroimage. (2011) 56:8–20. doi: 10.1016/j.neuroimage.2011.01.051
179. Shi F, Yap PT, Wu G, Jia H, Gilmore JH, Lin W, et al. Infant brain atlases from neonates to 1- and 2-year-olds. PLoS One. (2011) 6:e18746. doi: 10.1371/journal.pone.0018746
180. Ou Y, Zöllei L, Retzepi K, Castro V, Bates SV, Pieper S, et al. Using clinically acquired MRI to construct age-specific ADC atlases: quantifying spatiotemporal ADC changes from birth to 6-year old. Hum Brain Mapp. (2017) 38:3052–68. doi: 10.1002/hbm.23573
181. Sotardi S, Gollub RL, Bates SV, Weiss R, Murphy SN, Grant PE, et al. Voxelwise and Regional Brain Apparent Diffusion Coefficient Changes on MRI from Birth to 6 Years of Age. Radiology. (2021) 298:415–24. doi: 10.1148/RADIOL.2020202279
182. Gholipour A, Rollins CK, Velasco-Annis C, Ouaalam A, Akhondi-Asl A, Afacan O, et al. A normative spatiotemporal MRI atlas of the fetal brain for automatic segmentation and analysis of early brain growth. Sci Rep. (2017) 7:476. doi: 10.1038/s41598-017-00525-w
183. Gilmore JH, Knickmeyer RC, Gao W. Imaging structural and functional brain development in early childhood. Nat Rev Neurosci. (2018) 19:123–37. doi: 10.1038/nrn.2018.1
184. Rollins CK, Ortinau CM, Stopp C, Friedman KG, Tworetzky W, Gagoski B, et al. Regional brain growth trajectories in fetuses with congenital heart disease. Ann Neurol. (2021) 89:143–57. doi: 10.1002/ana.25940
185. Oishi K, Chang L, Huang H. Baby brain atlases. Neuroimage. (2019) 185:865–80. doi: 10.1016/j.neuroimage.2018.04.003
186. Cole JH, Franke K. Predicting Age Using Neuroimaging: innovative Brain Ageing Biomarkers. Trends Neurosci. (2017) 40:681–90. doi: 10.1016/j.tins.2017.10.001
187. He S, Pereira D, David Perez J, Gollub RL, Murphy SN, Prabhu S, et al. Multi-channel attention-fusion neural network for brain age estimation: accuracy, generality, and interpretation with 16,705 healthy MRIs across lifespan. Med Image Anal. (2021) 72:102091. doi: 10.1016/j.media.2021.102091
188. He S, Grant PE, Ou Y. Global-Local Transformer for Brain Age Estimation. IEEE Trans Med Imaging. (2022) 41:213–24. doi: 10.1109/TMI.2021.3108910
189. National Institutes of Health [NIH]. NIH Nutrition Research Task Force | NIDDK. (2020). Available online at: https://www.niddk.nih.gov/about-niddk/advisory-coordinating-committees/nih-nutrition-research-task-force (Accessed December 2, 2020).
190. National Institutes of Health [NIH]. NIH releases strategic plan to accelerate nutrition research over next 10 years | National Institutes of Health (NIH). (2020). Available online at: https://www.nih.gov/news-events/news-releases/nih-releases-strategic-plan-accelerate-nutrition-research-over-next-10-years (Accessed December 2, 2020).
191. Bahnfleth C, Canfield R, Nevins J, Caudill M, Strupp B. Prenatal choline supplementation improves child color-location memory task performance at 7 Y of age (FS05-01-19). Curr Dev Nutr. (2019) 3:nzz052.FS05-01-19. doi: 10.1093/CDN/NZZ052.FS05-01-19
192. Caudill MA, Strupp BJ, Muscalu L, Nevins JEH, Canfield RL. Maternal choline supplementation during the third trimester of pregnancy improves infant information processing speed: a randomized, double-blind, controlled feeding study. FASEB J. (2018) 32:2172–80. doi: 10.1096/FJ.201700692RR
193. Ross RG, Hunter SK, Hoffman MC, McCarthy L, Chambers BM, Law AJ, et al. Perinatal phosphatidylcholine supplementation and early childhood behavior problems: evidence for CHRNA7 moderation. Am J Psychiatry. (2016) 173:509–16. doi: 10.1176/APPI.AJP.2015.15091188
194. Helland IB, Smith L, Saarem K, Saugstad OD, Drevon CA. Maternal supplementation with very-long-chain n-3 fatty acids during pregnancy and lactation augments children’s IQ at 4 years of age. Pediatrics. (2003) 111:e39–44. doi: 10.1542/peds.111.1.e39
195. Braarud HC, Markhus MW, Skotheim S, Stormark KM, Frøyland L, Graff IE, et al. Maternal DHA status during pregnancy has a positive impact on infant problem solving: a Norwegian prospective observation study. Nutrients. (2018) 10:529. doi: 10.3390/NU10050529
196. Yan J, Zhu Y, Cao LJ, Liu YY, Zheng YZ, Li W, et al. Effects of maternal folic acid supplementation during pregnancy on infant neurodevelopment at 1 month of age: a birth cohort study in China. Eur J Nutr. (2020) 59:1345–56. doi: 10.1007/s00394-019-01986-7
197. Shah-Kulkarni S, Ha M, Kim BM, Kim E, Hong YC, Park H, et al. Neurodevelopment in early childhood affected by prenatal lead exposure and iron intake. Medicine. (2016) 95:e2508. doi: 10.1097/MD.0000000000002508
198. Berglund SK, Torres-Espínola FJ, García-Valdés L, Segura MT, Martínez-Zaldívar C, Padilla C, et al. The impacts of maternal iron deficiency and being overweight during pregnancy on neurodevelopment of the offspring. Br J Nutr. (2017) 118:533–40. doi: 10.1017/S0007114517002410
199. Jones KM, Black MM, Mejia RM, Ramirez-Zea M, Zuleta C, Allen LH. Cognitive function, motor skills, and behavior of Guatemalan infants with highly prevalent deficient and marginal plasma vitamin B-12 concentrations. FASEB J. (2006) 20:A1048–1048. doi: 10.1096/FASEBJ.20.5.A1048-B
200. Keskin M. Hematological findings associated with neurodevelopmental delay in infants with vitamin B12 deficiency. Acta Neurol Belg. (2020) 120:921–6. doi: 10.1007/s13760-020-01388-1
201. Morales E, Guxens M, Llop S, Rodríguez-Bernal CL, Tardón A, Riaño I, et al. Circulating 25-hydroxyvitamin D3 in pregnancy and infant neuropsychological development. Pediatrics. (2012) 130:e913–20. doi: 10.1542/peds.2011-3289
202. Hanieh S, Ha TT, Simpson JA, Thuy TT, Khuong NC, Thoang DD, et al. Maternal vitamin D status and infant outcomes in rural Vietnam: a prospective cohort study. PLoS One. (2014) 9:e99005. doi: 10.1371/JOURNAL.PONE.0099005
203. Tylavsky FA, Kocak M, Murphy LE, Graff JC, Palmer FB, Völgyi E, et al. Gestational vitamin 25(OH)D status as a risk factor for receptive language development: a 24-month, longitudinal, observational study. Nutrients. (2015) 7:9918–30. doi: 10.3390/nu7125499
204. Colombo J, Zavaleta N, Kannass KN, Lazarte F, Albornoz C, Kapa LL, et al. Zinc supplementation sustained normative neurodevelopment in a randomized, controlled trial of Peruvian infants aged 6-18 months. J Nutr. (2014) 144:1298–305. doi: 10.3945/JN.113.189365
205. Mathur NB, Agarwal DK. Zinc supplementation in preterm neonates and neurological development, a randomized controlled trial. Indian Pediatr. (2015) 52:951–5. doi: 10.1007/S13312-015-0751-6
206. Boeke CE, Gillman MW, Hughes MD, Rifas-Shiman SL, Villamor E, Oken E. Choline intake during pregnancy and child cognition at age 7 years. Am J Epidemiol. (2013) 177:1338–47. doi: 10.1093/AJE/KWS395
207. Catena A, Angela Muñoz-Machicao J, Torres-Espínola FJ, Martínez-Zaldívar C, Diaz-Piedra C, Gil A, et al. Folate and long-chain polyunsaturated fatty acid supplementation during pregnancy has long-term effects on the attention system of 8.5-y-old offspring: a randomized controlled trial. Am J Clin Nutr. (2016) 103:115–27. doi: 10.3945/ajcn.115.109108
208. Cheatham CL, Sheppard KW. Synergistic effects of human milk nutrients in the support of infant recognition memory: an observational study. Nutrients. (2015a) 7:9079–95. doi: 10.3390/nu7115452
209. D’souza N, Behere RV, Patni B, Deshpande M, Bhat D, Bhalerao A, et al. Pre-conceptional maternal vitamin B12 supplementation improves offspring neurodevelopment at 2 years of age: PRIYA trial. Front. Pediatr. (2021) 9:755977. doi: 10.3389/FPED.2021.755977
210. Deoni S, Dean D, Joelson S, O’Regan J, Schneider N. Early nutrition influences developmental myelination and cognition in infants and young children. Neuroimage. (2018) 178:649–59. doi: 10.1016/J.NEUROIMAGE.2017.12.056
211. Mahmassani HA, Switkowski KM, Scott TM, Johnson EJ, Rifas-Shiman SL, Oken E, et al. Maternal intake of lutein and zeaxanthin during pregnancy is positively associated with offspring verbal intelligence and behavior regulation in mid-childhood in the project viva cohort. J Nutr. (2021) 151:615–27. doi: 10.1093/jn/nxaa348
212. Ramakrishnan U, Gonzalez-Casanova I, Schnaas L, DiGirolamo A, Quezada AD, Pallo BC, et al. Prenatal supplementation with DHA improves attention at 5 y of age: a randomized controlled trial. Am J Clin Nutr. (2016) 104:1075–82. doi: 10.3945/ajcn.114.101071
213. Robinson SM, Crozier SR, Miles EA, Gale CR, Calder PC, Cooper C, et al. Preconception maternal iodine status is positively associated with IQ but not with measures of executive function in childhood. J Nutr. (2018) 148:959–66. doi: 10.1093/jn/nxy054
214. Veena SR, Krishnaveni GV, Srinivasan K, Wills AK, Muthayya S, Kurpad AV, et al. Higher maternal plasma folate but not vitamin B-12 concentrations during pregnancy are associated with better cognitive function scores in 9- to 10- year-old children in South India. J Nutr. (2010) 140:1014–22. doi: 10.3945/JN.109.118075
Keywords: brain development, data science, magnetic resonance image, neonate, nutrition
Citation: Morton SU, Leyshon BJ, Tamilia E, Vyas R, Sisitsky M, Ladha I, Lasekan JB, Kuchan MJ, Grant PE and Ou Y (2022) A Role for Data Science in Precision Nutrition and Early Brain Development. Front. Psychiatry 13:892259. doi: 10.3389/fpsyt.2022.892259
Received: 08 March 2022; Accepted: 17 May 2022;
Published: 23 June 2022.
Edited by:
Dorothea L. Floris, University of Zurich, SwitzerlandReviewed by:
Bryan Kolb, University of Lethbridge, CanadaKiyotaka Nemoto, University of Tsukuba, Japan
Copyright © 2022 Morton, Leyshon, Tamilia, Vyas, Sisitsky, Ladha, Lasekan, Kuchan, Grant and Ou. This is an open-access article distributed under the terms of the Creative Commons Attribution License (CC BY). The use, distribution or reproduction in other forums is permitted, provided the original author(s) and the copyright owner(s) are credited and that the original publication in this journal is cited, in accordance with accepted academic practice. No use, distribution or reproduction is permitted which does not comply with these terms.
*Correspondence: Sarah U. Morton, sarah.morton@childrens.harvard.edu; Yangming Ou, yangming.ou@childrens.harvard.edu