- 1Department of Forensic Medicine, School of Medicine & Forensics, Xi’an Jiaotong University, Xi’an, China
- 2Department of Health Science, Chang’an Drug Rehabilitation Center, Xi’an, China
- 3Department of Ultrasound, The Second Affiliated Hospital, Xi’an Jiaotong University, Xi’an, China
- 4Department of Epidemiology and Biostatistics, School of Public Health, Xi’an Jiaotong University, Xi’an, China
- 5Department of Psychiatry, Xi’an Mental Health Center, Xi’an, China
Objectives: Methamphetamine (METH) is a central nervous psychostimulant and one of the most frequently used illicit drugs. Numerous genetic loci that influence complex traits, including alcohol abuse, have been discovered; however, genetic analyses for METH dependence remain limited. An increased histone deacetylase 3 (HDAC3) expression has been detected in Fos-positive neurons in the dorsomedial striatum following withdrawal after METH self-administration. Herein, we aimed to systematically investigate the contribution of HDAC3 to the vulnerability to METH dependence in a Han Chinese population.
Methods: In total, we recruited 1,221 patients with METH dependence and 2,328 age- and gender-matched controls. For genotyping, we selected 14 single nucleotide polymorphisms (SNPs) located within ± 3 kb regions of HDAC3. The associations between genotyped genetic polymorphisms and the vulnerability to METH dependence were examined by single marker- and haplotype-based methods using PLINK. The effects of expression quantitative trait loci (eQTLs) on targeted gene expressions were investigated using the Genotype-Tissue Expression (GTEx) database.
Results: The SNP rs14251 was identified as a significant association signal (χ2 = 9.84, P = 0.0017). An increased risk of METH dependence was associated with the A allele (minor allele) of rs14251 [odds ratio (95% CI) = 1.25 (1.09–1.43)]. The results of in silico analyses suggested that SNP rs14251 could be a potential eQTL signal for FCHSD1, PCDHGB6, and RELL2, but not for HDAC3, in various human tissues.
Conclusion: We demonstrated that genetic polymorphism rs14251 located at 5q31.3 was significantly associated with the vulnerability to METH dependence in Han Chinese population.
Introduction
Methamphetamine (METH), a well-known powerful central nervous psychostimulant, is currently the most commonly used illicit drug in China (1). METH can cause damage to multiple organs, such as the heart, gut, and brain (2). Meanwhile, individuals with a history of chronic METH use are likely to develop METH-related psychosis, including auditory hallucinations and paranoid thinking (3). METH dependence is a huge financial burden on people with addiction and their families. In addition, it can provoke a series of violent events, leading to several social problems. Although the precise mechanism underlying METH dependence remains unknown, METH-induced changes in gene expression were shown to be closely related to severe dysregulation of normal neurophysiological brain activity. More recently, genome-wide association studies have been extensively employed to detect correlations between genetic variants and complex diseases, such as schizophrenia, coronary heart disease, and height in samples from various populations (4, 5). Numerous loci that influence complex traits, such as alcohol and other substance abuse, have been identified, facilitating our understanding of underlying molecular mechanisms (6–8). However, data on METH dependence remains scarce despite multiple reports of candidate genes (9).
Epigenetic alterations can lead to persistent structural chromatin adaptations, indicating that epigenetic modifications may play a critical role in the METH-induced gene expression changes (10, 11). Furthermore, abnormal microRNA expression is known to mediate METH dependence (12). Histone deacetylases (HDACs) are proteins involved in histone acetylation and can be classified into four classes according to the homologous similarity of their sequences. Class I, including HDAC1, HDAC2, HDAC3, and HDAC8, is considered critical for transcriptional repression and epigenetic modulation (13). As HDAC3 is highly expressed in the adult brain, its effects on transcriptional regulation related to learning and memory have attracted considerable attention (14). In the METH-induced conditioned place preference (CPP) model, an increased histone 3 acetylation was identified in the limbic forebrain of mice and found in specific gene-promoter regions related to synaptic plasticity, such as Nrxn, Gria1, Grin2a, and Grin2b, thus indicating its essential role in METH dependence (15). An increased expression of HDAC3 mRNA has been noted in Fos-positive neurons in the dorsomedial striatum after withdrawal following METH self-administration (16). Studies have shown that sodium butyrate, a non-selective inhibitor of class I/II HDACs, can help overcome a previously established CPP and suppress the reinstatement of METH-induced CPP (17). These findings suggest that HDAC3 may be a key molecule for regulating METH-associated gene expression and clarifying the underlying biological mechanism. However, the relationship between the HDAC3 gene and METH dependence remains elusive. In this study, we aimed to systematically explore the risk susceptibility of HDAC3 to METH dependence among Han Chinese population.
Materials and Methods
Study Subjects
Herein, we enrolled 1,221 patients with METH dependence and 2,328 age-matched healthy controls from the Chang’an Drug Rehabilitation Center of Xi’an City and the Second Affiliated Hospital of Xi’an Jiaotong University, respectively. All participants were genetically unrelated individuals of Han Chinese origin (at least three generations were of Han descent and had no history of migration). The inclusion criteria for the METH dependence group were as follows: (1) 11 criteria for substance use disorders (Supplementary Table 1) according to Diagnostic and Statistical Manual of Mental Disorders-Fifth Edition (DSM-V); (2) METH use >2 days per week for >1 year; (3) no use disorders (DSM-V criteria) considering other addictive substances, including alcohol and marijuana. Participants with tumors, neurodegenerative disorders, and other severe organic disorders were excluded from the study. In addition, participants were excluded if they met the criteria for past or current manic episodes, schizophrenia, schizoaffective disorder, or other psychotic disorders based on the DSM-V. Peripheral blood samples were collected from participants and preserved for further genotyping experiments. Demographic characteristics of all participants were collected using questionnaires and are presented in Table 1. All participants provided written informed consent. The study procedures were approved by the Medical Ethics Committee of Xi’an Jiaotong University Health Science Center and performed in accordance with the ethical guidelines of the Declaration of Helsinki (version 2013).
Candidate Single Nucleotide Polymorphisms Selection and Genotyping
Single nucleotide polymorphisms with a minor allele frequency (MAF) >0.05 in mixed population data and located within ± 3 kb regions of HDAC3 were selected. This strategy formed a set of 22 SNPs. We excluded 5 SNPs that were non-polymorphic in the 1000 Genomes database for the Han Chinese population. In addition, we excluded 3 indels; accordingly, 14 candidate SNPs were selected for further genotyping experiments (Supplementary Table 2). Peripheral blood was drawn from each participant, and genomic DNA was extracted using a commercial DNA kit according to the manufacturer’s protocol (Axygen Scientific Inc., Union City, CA, United States). SNP genotyping experiments were conducted using the Sequenom MassARRAY platform. The raw data were processed, and the genotypic data were released using the Typer Analyzer. Technicians involved in experimental processes were blinded to case or control labels. A small portion of study samples (5%) were randomly selected for replication experiments to assess the accuracy of SNP genotyping.
Statistical Analyses
To estimate the statistical power of the study, a power analysis was implemented using the Genetic Association Study (GAS) Power Calculator.1 The power analysis results are summarized in Supplementary Figure 1. The power analysis results indicated that the sample size level was sufficient to detect a SNP with moderate effect. The Hardy-Weinberg equilibrium (HWE) tests were performed for genotyping quality control based on obtained data for control individuals. The associations between genotyped genetic polymorphisms and vulnerability to METH dependence were examined using single marker- and haplotype-based methods. Both allelic and genotypic distributions of genetic polymorphisms in participants with METH dependence and healthy controls were determined using PLINK (18) for single marker-based association analyses. Linkage disequilibrium (LD) patterns of the 14 candidate genetic polymorphisms were defined based on a standard algorithm (19). Visualization of the LD structure was achieved using the Haploview version 4.2 (20). The statistical significance of the association analyses was examined using χ2 tests. Multiple testing was performed using the Bonferroni corrections. The P-value threshold was set as 0.05 divided by the number of independent tests.
Bioinformatics Analyses
We examined the effects of expression quantitative trait loci (eQTLs) on targeted gene expressions using the Genotype-Tissue Expression (GTEx) database (21), which integrates genetic polymorphism information and gene expression data from various types of human tissues to depict the patterns of human genome eQTLs. Sorting Intolerant From Tolerant (SIFT) (22) and Polymorphism Phenotyping v2 (Polyphen-2) (23) were utilized to explore the potential functional consequences of non-synonymous DNA variants. Both tools are designed to predict the impact of amino acid changes on the structure and function of the protein. The protein-protein interaction (PPI) networks of HDAC3 were explored using the Search Tool for the Retrieval of Interacting Genes/Proteins (STRING) version 11.5 database (24). STRING is a publicly available database of PPIs stemming from computational prediction or knowledge obtained from functional studies.
Results
Demographic and Characteristic Features of Participants
A total of 1,221 patients with METH dependence and 2,328 controls were enrolled. No significant differences were observed in terms of age and gender between patients and controls, as both variables were matched at enrollment (Table 1). We noted significant differences in employment and marital status between the patients with METH dependence and controls. In addition, certain socioeconomic status (SES) variables were distinctly distributed among the two groups.
Association Between Genetic Polymorphisms of HDAC3 and Methamphetamine Dependence
All 14 SNPs showed HWE in the control group, and the results are presented in Supplementary Table 2. The SNP rs14251 was identified as a significant signal in the association analyses (Table 2), and its P-value was significant in allelic analyses following Bonferroni correction (χ2 = 9.84, P = 0.0017, Supplementary Table 3). Nominal significance was observed at the genotype level of this SNP (χ2 = 10.37, P = 0.0056). An increased risk of METH dependence was associated with the A allele (minor allele) of rs14251. The odds of exhibiting a copy of A allele vs. C allele was 25% higher in patients with METH dependence than in controls [odds ratio (OR): 1.25; 95% CI: 1.09–1.43]. Three LD blocks were constructed and visualized, as shown in Figure 1. The haplotype-based analyses revealed significant differences in haplotypic frequencies between patients and controls in the rs56221992-rs11741808 LD block (Table 3). Notably, a significant difference was observed between the two groups for the TA haplotype (χ2 = 47.89, P = 4.50 × 10–11).
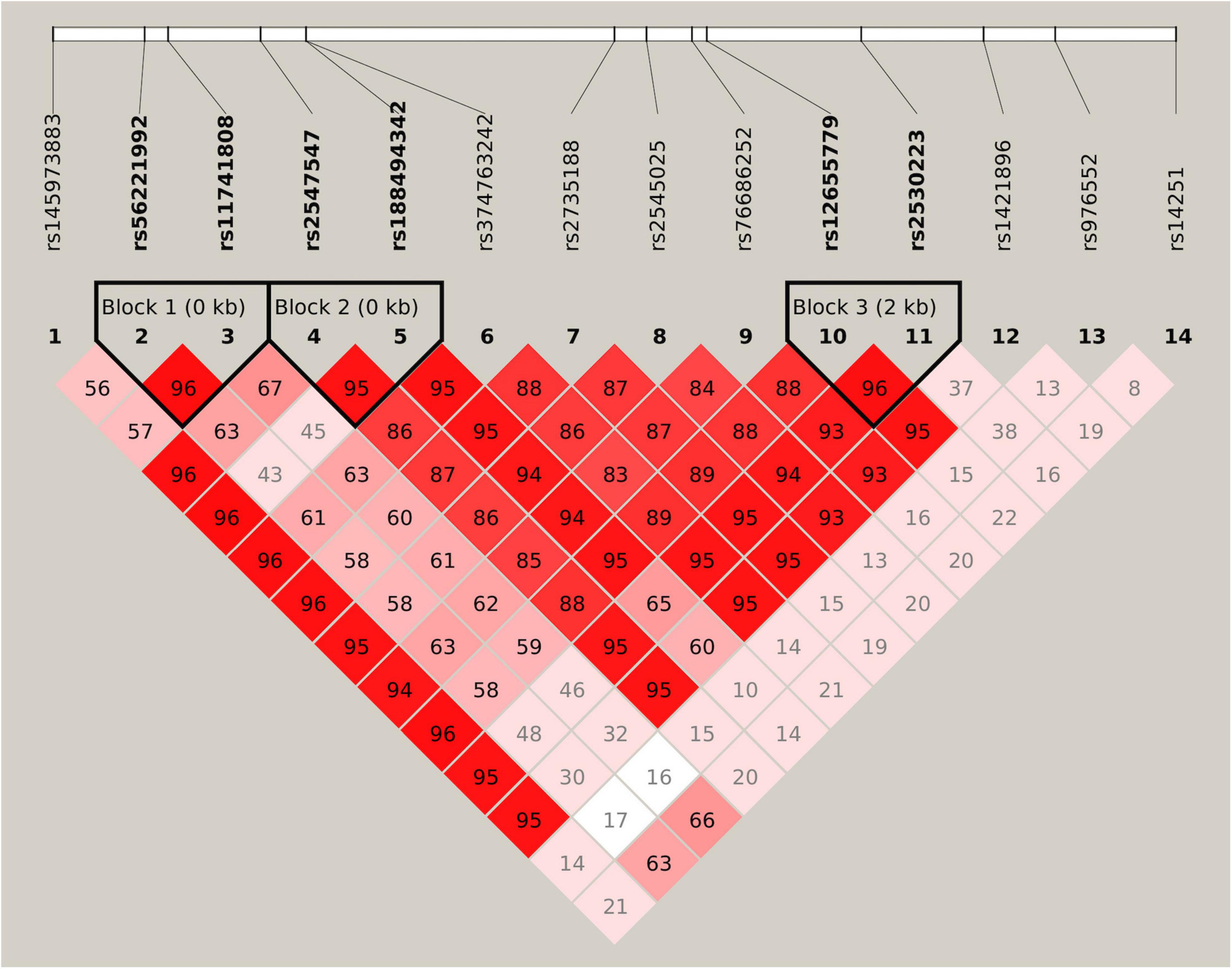
Figure 1. Linkage disequilibrium (LD) structure of 14 selected single nucleotide polymorphisms (SNPs). Values of D’ are indicated in each cell. The LD blocks are shown in thick-lined boxes, and SNPs of the haplotype blocks are highlighted in bold font.
Functional Consequences of Single Nucleotide Polymorphism rs14251
The data acquired from the GTEx database suggested that rs14251 is not involved in the expression level of HDAC3 in any human tissue (Supplementary Table 4), including 13 brain tissues. Significant eQTL effects of this SNP were observed for other genes physically located around HDAC3 (Table 4). These genes included FCHSD1, PCDHGB6, and RELL2. It should be noted that SNP rs14251 exerted varying effects on the expression levels of these genes. The A allele of SNP rs14251 increased the expression of FCHSD1 and decreased the expression of both PCDHGB6 and RELL2. Given that SNP rs14251 is located in the exonic region of RELL2 and is a non-synonymous change, its functional consequence on this gene was predicted using SIFT and PolyPhen-2. SIFT indicated this SNP as “tolerated” and PolyPhen-2 as “benign,” respectively. The PPI network of HDAC3 is depicted in Supplementary Figure 2.
Discussion
A variety of neurotransmitter and receptor system-related genes, including BDNF, DRD2, and GABRB2, were found to contain genetic variants that contribute to the vulnerability of METH use disorder (9). Although multiple lines of evidence have associated epigenetic alterations with METH dependence (25, 26), no relevant population-based studies focusing on epigenetic-related genes have been conducted. To the best of our knowledge, this study is the first to link DNA variants of HDAC3 and vulnerability to METH dependence in a population-based study. Recently, Rudzinskas et al. have indicated that METH alters HDAC and DNA methyltransferase (DNMT) activity in the posterior dorsal medial amygdala of rats (26); therefore, our findings align with these results. Genetic markers of specific genes may modify the effects of METH on human epigenetic patterns.
Significant associations between haplotypes and vulnerability to METH dependence have been previously reported. Both SNPs rs56221992 and rs11741808 showed moderate levels of LD with rs14251 (r2 = 0.3 for rs56221992 and r2 = 0.3 for rs11741808, respectively). Although we cannot exclude the possibility that this haplotypic association signal occurred by chance or originated from other underlying independent association signals, it could probably arise from SNP rs14251.
Single nucleotide polymorphism rs14251 is located in the 3′-untranslated region of HDAC3. It is also located in the exonic region of RELL2 as a non-synonymous change. This double identity increases the complexity of predicting its functional consequences. Both SIFT and PolyPhen-2 predicted that this SNP exerts mild functional consequences on the protein structure of RELL2. Nevertheless, our in silico analyses revealed that SNP rs14251 could affect the expression levels of RELL2, FCHSD1, and PCDHGB6 (but not that of HDAC3) in various kinds of human tissues. A recent study has linked HDAC3 expression to schizophrenia (27). However, neither RELL2 nor FCHSD1 appears to be associated with psychiatric or brain-related traits. RELL2 reportedly encodes receptors expressed in lymphoid tissues such as 2 protein, which participates in the positive regulation of the p38MAPK cascade and is speculated to be a human tumor necrosis factor (28). FCHSD1 encodes F-BAR protein and double SH3 domains protein 1. A recent study by Kawasaki et al. has indicated that the loss of FCHSD1 could ameliorate chronic obstructive pulmonary disease (29). In addition, the third gene, PCDHGB6, encodes a calcium-dependent cell-adhesion protein, potentially related to the activity of specific neuronal connections in the human brain (30). However, this study results are insufficient to functionally map SNP rs14251 to any of the four genes. Therefore, it might be more appropriate to map this SNP to the 5q31.3 genomic region instead of a specific gene.
Although eQTL data in the GTEx database indicated that SNP rs14251 could be mapped to the three surrounding genes, the results of in silico analyses should be cautiously interpreted. First, SNP rs14251 is related to gene expressions in various kinds of human tissues; however, 13 types of brain tissues (e.g., cortex, cerebellar, and others) are not listed. In other words, METH-dependence targeted tissues are not included. In addition, the METH addiction status for patients whose data were obtained from the GTEx database remains largely unknown. A gene expression pattern has specific spatial and temporal features, and it may considerably differ between patients and healthy participants. Accordingly, animal-based functional studies remain crucial to properly map SNP rs14251 to its functionally relevant gene and unravel potential consequences.
The PPI network constructed in this study indicates that HDAC3 interacts with several other genes for implementing its biochemical and biological functions. Among the genes included in this network, PPARG is of particular interest. PPARG is known to encode peroxisome proliferator-activated receptor (PPAR) gamma, which is a member of the PPARs, a subfamily of nuclear receptors (31). A recent genome-association study has linked PPARG genetic polymorphisms to human cognitive measurements (32). As METH-use disorders are widely associated with cognitive functions, this study highlights the complexity of pathogenic mechanisms underlying METH dependence.
In addition, the limitations of this study need to be addressed. Although we have selected SNPs located at the gene region of HDAC3 with MAF >0.05, the genetic information coverage may be insufficient. Recent studies on several psychiatric disorders, including bipolar disorder and nicotine dependence, have indicated that genetic variants with low frequency might substantially contribute to the susceptibility of relevant disorders (33, 34). In the future, low-frequency and rare DNA variants should be explored to exhaustively examine the genetic contribution of HDAC3 to the vulnerability to METH dependence. Replication studies are also needed to rule out the “winner’s curse.” In addition, the controls recruited in this study might not be comparable with METH-dependent individuals. To examine the vulnerability to METH dependence, ideal controls would be those individuals who use METH but are not addicted; this, in turn, might affect the association measures.
Conclusion
In summary, we demonstrated that genetic polymorphism rs14251 located at 5q31.3 was significantly associated with the vulnerability to METH dependence in a Han Chinese population.
Data Availability Statement
According to national legislation/guidelines, specifically the Administrative Regulations of the People’s Republic of China on Human Genetic Resources (http://www.gov.cn/zhengce/content/2019-06/10/content_5398829.htm, http://english.www.gov.cn/policies/latest_releases/2019/06/10/content_281476708945462. htm), no additional raw data is available at this time. Data of this project can be accessed after an approval application to the China National Genbank (CNGB, https://db.cngb.org/cnsa/). Please refer to https://db.cngb.org/, or email: Q05HQmRiQGNuZ2Iub3Jn for detailed application guidance. The accession code CNP0002872 should be included in the application.
Ethics Statement
The studies involving human participants were reviewed and approved by the Medical Ethics Committee of Xi’an Jiaotong University Health Science Center. The patients/participants provided their written informed consent to participate in this study.
Author Contributions
FG, TZ, and HLin conceived and designed the study. JX performed the computational analyses and a candidate SNP selection and wrote the first draft of the manuscript. YM and XW performed the statistical analyses of genotype and haplotype data. CW, ML, WH, and HLiu conducted the study subjects’ phenotypic screening. HLiu, HWe, WZ, HWa, and LZ contributed to the collections and preparations of control subjects’ DNA samples. All authors revised the current manuscript.
Funding
This study was supported by the National Natural Science Foundation of China (82171873 and 31900407) and Xi’an Innovation Capability of Strong Foundation Program-Medical Research Project (21YXYJ0079). The funding body did not participate in the design, conduct, or writing of the study.
Conflict of Interest
The authors declare that the research was conducted in the absence of any commercial or financial relationships that could be construed as a potential conflict of interest.
Publisher’s Note
All claims expressed in this article are solely those of the authors and do not necessarily represent those of their affiliated organizations, or those of the publisher, the editors and the reviewers. Any product that may be evaluated in this article, or claim that may be made by its manufacturer, is not guaranteed or endorsed by the publisher.
Acknowledgments
We are grateful to all the patients and their families and the general practitioners who referred patients to our service and collaborated with the study.
Supplementary Material
The Supplementary Material for this article can be found online at: https://www.frontiersin.org/articles/10.3389/fpsyt.2022.870322/full#supplementary-material
Footnotes
References
1. Liu Y, Hao B, Shi Y, Xue L, Wang X, Chen Y, et al. Violent offences of methamphetamine users and dilemmas of forensic psychiatric assessment. Forensic Sci Res. (2017) 2:11–7. doi: 10.1080/20961790.2017.1287155
2. Prakash MD, Tangalakis K, Antonipillai J, Stojanovska L, Nurgali K, Apostolopoulos V. Methamphetamine: effects on the brain, gut and immune system. Pharmacol Res. (2017) 120:60–7. doi: 10.1016/j.phrs.2017.03.009
3. Glasner-Edwards S, Mooney LJ. Methamphetamine psychosis: epidemiology and management. CNS Drugs. (2014) 28:1115–26. doi: 10.1007/s40263-014-0209-8
4. Visscher PM, Wray NR, Zhang Q, Sklar P, McCarthy MI, Brown MA, et al. 10 years of gwas discovery: biology, function, and translation. Am J Hum Genet. (2017) 101:5–22. doi: 10.1016/j.ajhg.2017.06.005
5. Tam V, Patel N, Turcotte M, Bosse Y, Pare G, Meyre D. Benefits and limitations of genome-wide association studies. Nat Rev Genet. (2019) 20:467–84. doi: 10.1038/s41576-019-0127-1
6. Buchwald J, Chenoweth MJ, Palviainen T, Zhu G, Benner C, Gordon S, et al. Genome-wide association meta-analysis of nicotine metabolism and cigarette consumption measures in smokers of European descent. Mol Psychiatry. (2021) 26:2212–23. doi: 10.1038/s41380-020-0702-z
7. Kinreich S, Meyers JL, Maron-Katz A, Kamarajan C, Pandey AK, Chorlian DB, et al. Predicting risk for alcohol use disorder using longitudinal data with multimodal biomarkers and family history: a machine learning study. Mol Psychiatry. (2021) 26:1133–41. doi: 10.1038/s41380-019-0534-x
8. Passchier RV, Stein DJ, Uhlmann A, van der Merwe C, Dalvie S. Schizophrenia polygenic risk and brain structural changes in methamphetamine-associated psychosis in a South African population. Front Genet. (2020) 11:1018. doi: 10.3389/fgene.2020.01018
9. Guerin AA, Nestler EJ, Berk M, Lawrence AJ, Rossell SL, Kim JH. Genetics of methamphetamine use disorder: a systematic review and meta-analyses of gene association studies. Neurosci Biobehav Rev. (2021) 120:48–74. doi: 10.1016/j.neubiorev.2020.11.001
11. Rogge GA, Wood MA. The role of histone acetylation in cocaine-induced neural plasticity and behavior. Neuropsychopharmacology. (2013) 38:94–110. doi: 10.1038/npp.2012.154
12. Liu D, Zhu L, Ni T, Guan FL, Chen YJ, Ma DL, et al. Ago2 and dicer1 are involved in METH-induced locomotor sensitization in mice via biogenesis of MiRNA. Addict Biol. (2019) 24:498–508. doi: 10.1111/adb.12616
13. Witt O, Deubzer HE, Milde T, Oehme I. HDAC family: what are the cancer relevant targets? Cancer Lett. (2009) 277:8–21. doi: 10.1016/j.canlet.2008.08.016
14. Emmett MJ, Lazar MA. Integrative regulation of physiology by histone deacetylase 3. Nat Rev Mol Cell Biol. (2019) 20:102–15. doi: 10.1038/s41580-018-0076-0
15. Shibasaki M, Mizuno K, Kurokawa K, Ohkuma S. L-type voltage-dependent calcium channels facilitate acetylation of histone H3 through Pkcγ phosphorylation in mice with methamphetamine-induced place preference. J Neurochem. (2011) 118:1056–66. doi: 10.1111/j.1471-4159.2011.07387.x
16. Li X, Davis IR, Lofaro OM, Zhang JJ, Cimbro R, Rubio FJ. Distinct gene alterations between fos-expressing striatal and thalamic neurons after withdrawal from methamphetamine self-administration. Brain Behav. (2019) 9:e01378. doi: 10.1002/brb3.1378
17. Zhu J, Zhao N, Chen Y, Zhu L, Zhong Q, Liu J, et al. Sodium butyrate modulates a methamphetamine-induced conditioned place preference. J Neurosci Res. (2017) 95:1044–52. doi: 10.1002/jnr.23835
18. Purcell S, Neale B, Todd-Brown K, Thomas L, Ferreira MA, Bender D, et al. PLINK: a tool set for whole-genome association and population-based linkage analyses. Am J Hum Genet. (2007) 81:559–75. doi: 10.1086/519795
19. Gabriel SB, Schaffner SF, Nguyen H, Moore JM, Roy J, Blumenstiel B, et al. The structure of haplotype blocks in the human genome. Science. (2002) 296:2225–9. doi: 10.1126/science.1069424
20. Barrett JC, Fry B, Maller J, Daly MJ. Haploview: analysis and visualization of LD and haplotype maps. Bioinformatics. (2005) 21:263–5. doi: 10.1093/bioinformatics/bth457
22. Vaser R, Adusumalli S, Leng SN, Sikic M, Ng PC. SIFT missense predictions for genomes. Nat Protoc. (2016) 11:1–9. doi: 10.1038/nprot.2015.123
23. Adzhubei IA, Schmidt S, Peshkin L, Ramensky VE, Gerasimova A, Bork P, et al. A method and server for predicting damaging missense mutations. Nat Methods. (2010) 7:248–9. doi: 10.1038/nmeth0410-248
24. Szklarczyk D, Gable AL, Lyon D, Junge A, Wyder S, Huerta-Cepas J, et al. STRING V11: protein-protein association networks with increased coverage, supporting functional discovery in genome-wide experimental datasets. Nucleic Acids Res. (2019) 47:D607–13. doi: 10.1093/nar/gky1131
25. Godino A, Jayanthi S, Cadet JL. Epigenetic landscape of amphetamine and methamphetamine addiction in rodents. Epigenetics. (2015) 10:574–80. doi: 10.1080/15592294.2015.1055441
26. Rudzinskas SA, Mong JA. Methamphetamine alters DNMT and HDAC Activity in the posterior dorsal medial amygdala in an ovarian steroid-dependent manner. Neurosci Lett. (2018) 683:125–30. doi: 10.1016/j.neulet.2018.06.042
27. Jia X, Zhang T, Li L, Fu D, Lin H, Chen G, et al. Two-stage additional evidence support association of common variants in the HDAC3 with the increasing risk of schizophrenia susceptibility. Am J Med Genet B Neuropsychiatr Genet. (2016) 171:1105–11. doi: 10.1002/ajmg.b.32491
28. Moua P, Checketts M, Xu LG, Shu HB, Reyland ME, Cusick JK. RELT family members activate p38 and induce apoptosis by a mechanism distinct from TNFR1. Biochem Biophys Res Commun. (2017) 491:25–32. doi: 10.1016/j.bbrc.2017.07.022
29. Kawasaki T, Sugihara F, Fukushima K, Matsuki T, Nabeshima H, Machida T, et al. Loss of FCHSD1 leads to amelioration of chronic obstructive pulmonary disease. Proc Natl Acad Sci USA. (2021) 118:e2019167118. doi: 10.1073/pnas.2019167118
30. Yagi T, Takeichi M. Cadherin superfamily genes: functions, genomic organization, and neurologic diversity. Genes Dev. (2000) 14:1169–80. doi: 10.1101/gad.14.10.1169
31. Liu C, Tate T, Batourina E, Truschel ST, Potter S, Adam M, et al. Pparg promotes differentiation and regulates mitochondrial gene expression in bladder epithelial cells. Nat Commun. (2019) 10:4589. doi: 10.1038/s41467-019-12332-0
32. Demange PA, Malanchini M, Mallard TT, Biroli P, Cox SR, Grotzinger AD, et al. Investigating the genetic architecture of noncognitive skills using GWAS-by-subtraction. Nat Genet. (2021) 53:35–44. doi: 10.1038/s41588-020-00754-2
33. Zhang TX, Saccone NL, Bierut LJ, Rice JP. Targeted sequencing identifies genetic polymorphisms of flavin-containing monooxygenase genes contributing to susceptibility of nicotine dependence in European American and African American. Brain Behav. (2017) 7:e00651. doi: 10.1002/brb3.651
Keywords: methamphetamine dependence, genetic polymorphisms, case-control study, HDAC3, Han Chinese
Citation: Xiao J, Ma Y, Wang X, Wang C, Li M, Liu H, Han W, Wang H, Zhang W, Wei H, Zhao L, Zhang T, Lin H and Guan F (2022) The Vulnerability to Methamphetamine Dependence and Genetics: A Case-Control Study Focusing on Genetic Polymorphisms at Chromosomal Region 5q31.3. Front. Psychiatry 13:870322. doi: 10.3389/fpsyt.2022.870322
Received: 06 February 2022; Accepted: 20 April 2022;
Published: 20 May 2022.
Edited by:
Tianhua Niu, Tulane University, United StatesReviewed by:
Feng Zhu, Xi’an Jiaotong University, ChinaThomas Nicholas Ferraro, Cooper Medical School of Rowan University, United States
Copyright © 2022 Xiao, Ma, Wang, Wang, Li, Liu, Han, Wang, Zhang, Wei, Zhao, Zhang, Lin and Guan. This is an open-access article distributed under the terms of the Creative Commons Attribution License (CC BY). The use, distribution or reproduction in other forums is permitted, provided the original author(s) and the copyright owner(s) are credited and that the original publication in this journal is cited, in accordance with accepted academic practice. No use, distribution or reproduction is permitted which does not comply with these terms.
*Correspondence: Tianxiao Zhang, am9zaHVhekB4anR1LmVkdS5jbg==; Huali Lin, bGluaHVhbGkxOTcyQDEyNi5jb20=; Fanglin Guan, ZmFuZ2xpbmdndWFuQDE2My5jb20=
†These authors have contributed equally to this work