- 1Chinese Open Science Network, Xiamen, China
- 2Department of Strategy and Entrepreneurship, College of Business, Oregon State University, Corvallis, OR, United States
- 3Department of Speech-Language-Hearing Sciences, Texas Tech University Health Sciences Center, Lubbock, TX, United States
- 4UniSA Business, University of South Australia, Adelaide, SA, Australia
- 5Department of Innovation, Entrepreneurship and Strategy, School of Economic and Management, Tsinghua University, Beijing, China
- 6Department of Sociology and Interuniversity Center for Social Science Theory and Methodology (ICS), University of Groningen, Groningen, Netherlands
- 7Department of Psychology and Behavioral Sciences, Zhejiang University, Hangzhou, China
- 8Department of Clinical, Neuro- and Developmental Psychology, Vrije Universiteit Amsterdam, Amsterdam, Netherlands
- 9Department of Social, Health and Organizational Psychology, Utrecht University, Utrecht, Netherlands
- 10Entrepreneurship, Innovation and Family Enterprise Discipline, Adelaide Business School, University of Adelaide, Adelaide, SA, Australia
Objective: This paper used meta-regression to analyze the heterogenous factors contributing to the prevalence rate of mental health symptoms of the general and frontline healthcare workers (HCWs) in China under the COVID-19 crisis.
Method: We systematically searched PubMed, Embase, Web of Science, and Medrxiv and pooled data using random-effects meta-analyses to estimate the prevalence rates, and ran meta-regression to tease out the key sources of the heterogeneity.
Results: The meta-regression results uncovered several predictors of the heterogeneity in prevalence rates among published studies, including severity (e.g., above severe vs. above moderate, p < 0.01; above moderate vs. above mild, p < 0.01), type of mental symptoms (PTSD vs. anxiety, p = 0.04), population (frontline vs. general HCWs, p < 0.01), sampling location (Wuhan vs. Non-Wuhan, p = 0.04), and study quality (p = 0.04).
Conclusion: The meta-regression findings provide evidence on the factors contributing to the prevalence rate of mental health symptoms of the general and frontline healthcare workers (HCWs) to guide future research and evidence-based medicine in several specific directions.
Systematic Review Registration: https://www.crd.york.ac.uk/prospero/display_record.php?RecordID=220592, identifier: CRD42020220592.
Introduction
Since the first publicly known cases in Wuhan, China, on November 17, 2019, the COVID-19 (coronavirus disease 2019) crisis has become one of the worst epidemics in human record (1). The sudden outburst of this highly infectious disease and the containment measures such as quarantine and social distancing have posed immense pressure on the work and life of the healthcare workers (HCWs) (2–4). During the COVID-19 pandemic, HCWs have to face increased workload and extended working hours, shortage of medical resources, risk of nosocomial infection, stigmatization and other related problems (5–7). These work-related issues may induce the emotional distress of HCWs to cause mental health symptoms such as anxiety, depression, burnout, or sleep issues (8). Frontline HCWs are in a unique position to suffer mentally in particular. They have to deal with the ethical dilemma of resources allocation and the work pressure of hospice care (5, 9, 10) while being exposed to a high risk of infection in COVID-infected wards. The infection or death of any family member or colleague could act as additional stressors resulting mental health problems (11, 12).
Several early (rapid) meta-analysis papers have appeared but they pooled HCW of different countries all together. However, such practices inadvertently contribute to the differences in their prevalence rates, given the large heterogeneity in terms of not only the COVID cases and deaths but also the containment strategies and hospital capacities and readiness to handle COVID-19 cases across countries (13, 14). To rule out such heterogeneity at the same time, we conducted meta-regression analysis by focusing on a single country, China, which has had a sufficient number of empirical studies to analyze several factors at the same time to better understand the heterogenous factors contributing to the prevalence rate of mental health symptoms of the general and frontline healthcare workers (HCWs) (15, 16). Such evidence on the heterogenous factors contributing to the prevalence rate of mental health symptoms provide directions to better guide this important and proliferating stream of research.
Methods
This meta-regression analysis with a systematic review and meta-analysis conducted in accordance with the Preferred Reporting Items for Systematic Reviews and Meta-Analyses (PRISMA) statement 2019 and registered in the International Prospective Register of Systematic Reviews (PROSPERO: CRD42020220592).
Data Sources and Search Strategy
We conducted a comprehensive literature search in the databases of PubMed, Embase, and Web of Science. Our search query, shown in Supplementary Table S1, was entered with Boolean operators to search the titles, abstracts, keywords, and subject headings (for example, Mesh terms) in each database. To account for preprints, we searched medRxiv (medrxiv.org). We started our search on November 10, 2020, and finalized it on November 16, 2020, in order to cover the first year when HCWs faced a crisis of the COVID-19, as after 1 year the number of COVID-19 cases dropped significantly in China to no longer pose a crisis situation for HCWs (17). Figure 1 details the flow chart of our search process.
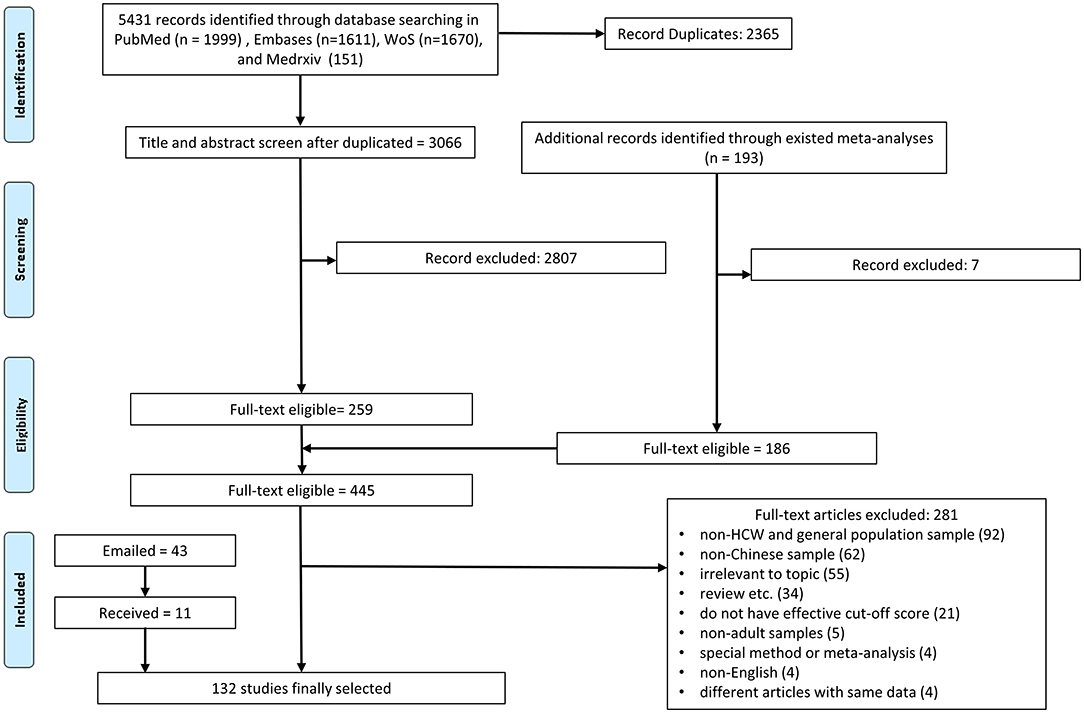
Figure 1. The PRISMA (Preferred Reporting Items for Systematic Reviews and Meta-Analysis) flow diagram.
Selection Criteria
The studies are included in our meta-analysis based on the following criteria:
a. Context: COVID-19 crisis in China.
b. Population: frontline HCWs, general HCWs, and general adult population (for comparison).
c. Outcome: at least one mental symptom outcomes, e.g., anxiety, depression, distress, insomnia, and PTSD.
d. Instrument: validated scales with cutoff points for the mental health outcomes.
e. Language: English.
According, we excluded studies that meet the following criteria:
a. Population: children, adolescents, or specific niche adult populations such as COVID-19 patients, inpatients, or other patients, adults under quarantine, pregnant/postpartum women.
b. Methodological approaches: Non-primary studies such as reviews or meta-analyses, qualitative or case studies without a validated instrument, interventional studies, interviews, or news reports.
c. Measurements: Non-validated mental health instruments (i.e., self-made questionnaire) or instruments without a validated cutoff score to calculate a prevalence rate (i.e., STAI, SCL-90 for anxiety and depression).
We contacted the authors of papers that missed some critical information if the articles:
a. Contain primary data on mental health of relevant population using established instruments under COVID-19 period but do not report the prevalence rates. For example, a study may report the mean and SD of our outcomes but not their prevalence rates.
b. Surveyed a sample that mixed our targeted population and other populations, such as children, in a manner such that we could not extract the prevalence rate(s) for our targeted population. We included the studies that authors provided prevalence rate for our targeted population only and excluded the studies with mixed populations.
c. Miss some critical information, such as the data collection time or location.
d. Are unclear on critical information. For example, some articles are unclear whether they used the cutoff for above mild or above moderate symptoms to calculate the overall prevalence rates of mental health symptoms. When a cutoff point is reported for an overall prevalence, above mild or above moderate was assigned based on the typical cutoff point of that instrument.
Selection Process and Data Extraction
The articles that passed the inclusion criteria were exported into an EndNote library where we identified duplications and then imported to Rayyan for screening. Two researchers (L.T. and Y.Y.) independently screened the articles based on their titles and abstracts. If both coders excluded an article independently, it was excluded.
Six researchers (X.C, M.Z., R.C., Z.D., R.D., B.C.) were paired to assess the eligibility of each paper based on reading its full text and extracting the relevant data into a coding book based on a coding protocol. The coding book records information such as the authors and year of the paper, title, publication status, sample locations, date of data collection, sample size, response rate, population, age (mean, SD, min and max), gender proportion, instruments, cutoff scores used, the prevalence/mean/SD of the mental health outcome, and other notes or comments. Pairs of researchers first double-coded and crosschecked each paper independently. The remaining discrepancies after the crosscheck were discussed between the pair of coders. In cases where a pair of coders continued to disagree, a lead coder (X.C.) checked the paper independently and discussed it with the two original coders to determine its coding. The lead coder also integrated and reviewed all the coding information. Particularly, the lead coder checked the mental outcomes, instruments, outcome levels, and cutoff scores reported given the multitude of reporting practices in individual papers. We were able to identify papers that used unusual cutoff scores later for sensitivity analysis.
Assessment of Bias Risk
Following other meta-analyses (18, 19), we used the Mixed Methods Appraisal Tool (MMAT) (20), including seven questions to conduct the quality assessment of the studies. Pairs of coders independently evaluated the risk of bias and quality of the studies and rated them based on the MMAT. Most discrepancies were resolved through a discussion between the pair of researchers, and any disagreement after discussions was resolved by a lead researcher. Papers were classed into high (6–7) or medium quality (lower than 6).
Statistical Analysis
To analyze the data in a consistent manner, we ensure the independence of mental health symptoms and samples. For instance, for studies that examine a mental health outcome with more than one instrument, we report the results based on the most popular instrument. If a study reported several prevalence rates by several cutoffs, we use one of them, in the following order of preference: above severe, above moderate, and above mild. Thus, only one prevalence rate for a mental health outcome in a sample is entered to ensure the samples remain independent.
The overall prevalence and 95% confidence intervals of psychological outcomes were pooled using Stata 16.1. Similar to prior studies on the prevalence of mental symptoms, the random-effects model was used to extract the pooled estimates (21). We reported the heterogeneity by the I2 statistic, which measures the percentage of variance resulting from true differences in the effect sizes rather than the sampling error (22). We performed subgroup analyses by the key potential sources of heterogeneity of outcomes (five types of mental health symptoms), severity of outcome (above mild/above moderate/above severe), three major population groups (frontline HCWs, general HCWs, and general population for comparison), and instrument type for each outcome. Furthermore, given the high degree of heterogeneity of the true differences in the effect sizes, we ran a meta-regression to regress the prevalence upon not only these three category variables (outcome, severity, and population) but also female proportion, data collection time, data collection location (Wuhan vs. Non-Wuhan), sample size, and study quality. We included data collection time to examine whether the mental symptoms change over time dynamically. While the COVID-19 crisis continues to evolve, there is a lack of dynamic analysis on the mental symptoms of any population over time. Sensitivity analysis was conducted, and Funnel plots were used to assess publication bias. Significance level was set as two-sided and p < 0.05.
Results
Study Screening
Our systematic search (Figure 1) across all the databases yielded 5,431 potentially relevant papers, out of which 2,365 were duplications and removed. Of the remaining 3,066 papers, we screened their titles and abstracts in the first stage and the full text of the 445 articles in the second stage. We also emailed the authors of 43 articles that missed critical information and were able to get the information to include 11 additional studies. Altogether, the process generated 132 articles.
Study Characteristics
The 132 papers included contains 171 samples (Supplementary Table S2) with a total of 645,805 individual participants. Table 1 summarizes their key characteristics. Among the 171 independent samples, about a quarter of them studied frontline HCWs and general HCWs (27.5 and 26.2%, respectively), and almost half studied the general population (43.3%) as a comparison. More than one-third of samples covered anxiety and depression. Another one-third investigated other mental symptoms including insomnia, PTSD, and distress, (15.9, 9.3, and 3.0%, respectively). Respectively, 20.7, 41.6, and 32.0% of samples reported prevalence rates at the mild above, moderate above, and severe above level by the severity of the symptoms.
Almost all the studies, 126 out of 131, employed cross-sectional surveys; specifically, 9 (6.1%) conducted the survey in January 2020, 90 (65.9%) in February, 23 (17.4%) in March, and 14 (10.6%) in April or later. Almost one-quarter of them (20.5%) contained a sample targeting populations in Wuhan. Most studies were published in journals, and 10 (7.6%) studies remained as preprints. The assessment based on the Mixed Methods Appraisal Tool (MMAT) indicated 100 (77.3%) studies were of good quality (score no <6 out of 7) and 31 studies were of medium quality (score <6 but >4). The median number of individuals per sample was 742 (range: 30–123,768) with a median female proportion of 69% (range: 12–100%) and a median response rate of 85% (range: 14–100%).
The 131 papers employed a wide arrange of instruments to assess mental health (Supplementary Table S3). GAD (61.8%) and SAS (23.6%) are the first and second most popular measures for anxiety, and PHQ (65.0%) and SDS (14.5%) for depression; distress is measured the most by K6 (50.0%); insomnia is measured by ISI (66.0%) and PSQI (26.4%); and PTSD by IES-R (41.9%), PCL-C (25.8%), and PCL-5 (25.8%). Please see the details in Supplementary Table S3.
Pooled Prevalence Rates of Mental Health Symptoms
The prevalence rates of the 171 samples were pooled by the subgroups one at a time (Table 2). First, the overall prevalence rates of mental health symptoms that surpassed the cutoff values of mild, moderate, and severe were 30, 15, and 2%, respectively. The overall prevalence of mental health symptom frontline HCWs and general HCWs are 16 and 13%, respectively, and in comparison, the prevalence in the general population is 13%. The overall prevalence of anxiety, depression, distress, insomnia, and PTSD are 11, 14, 15, 17, and 21%. Figure 2 graphically depicts such findings of the pooled analysis by subgroups using forest plots.
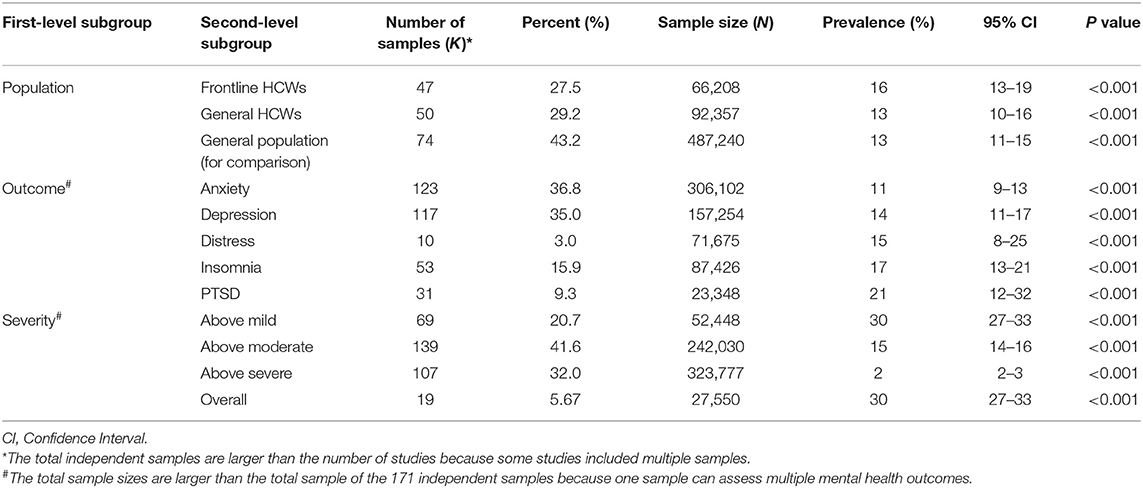
Table 2. The pooled prevalence rates of mental health symptoms by subgroups of population, outcome, and severity.
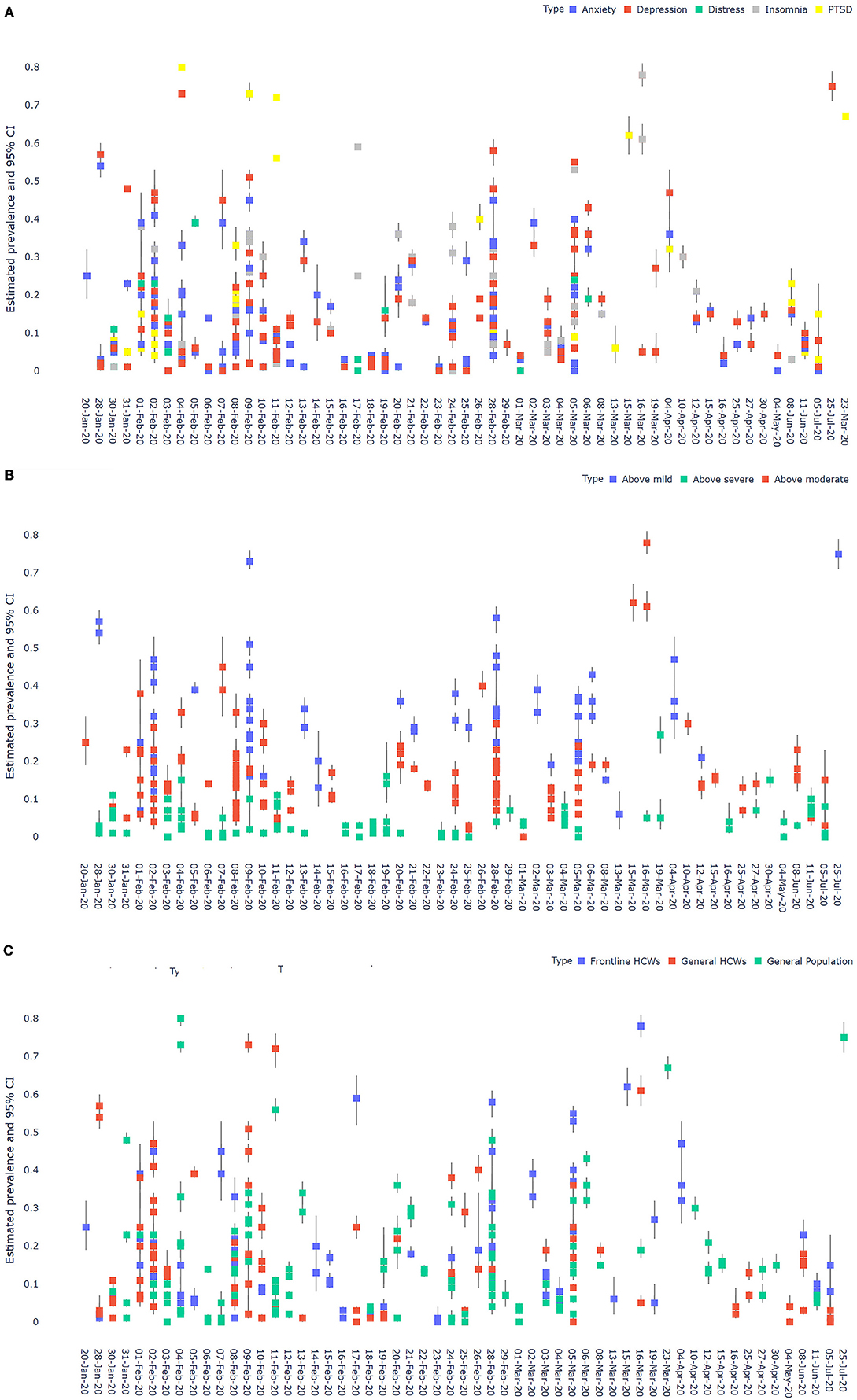
Figure 2. (A) A forest plot of the pooled prevalence by outcomes. (B) A forest plot of the pooled prevalence by outcome levels. (C) A forest plot of the pooled prevalence by population.
Meta-Regression on the Prevalence of Mental Health Symptoms
As pooled sub-group analysis takes account of only one factor at a time, to better explain the heterogeneity of the prevalence of mental health symptoms, Table 3 reports the results of a meta-regression analysis that takes account of several factors at the same time. The meta-analytical model explained over 40% of the variance of mental health symptoms among these studies (R-squared = 56.8%, tau2 = 0.09).
The prevalence of severe mental health symptoms is significantly lower than that of moderate mental illness (p < 0.001), which is in turn significantly lower than those of mild mental illness (p < 0.001). The prevalence of mental health symptoms of frontline HCWs is significantly higher than that of general HCWs (p = 0.005). General HCWs and the general population do not differ in their mental health prevalence rates. The prevalence rates of PTSD (p = 0.039) is significantly higher than that of anxiety. Interestingly, the prevalence of mental health symptoms of participants in Wuhan, the epicenter of the COVID-19 crisis in China, was significantly lower than that in Non-Wuhan samples (p = 0.038). The prevalence rates of mental health symptoms were higher in studies of papers with a higher quality rating (p = 0.036). The female proportion (p = 0.233), date of data collection (p = 0.392), sample size of studies (p = 0.124), or publication status (p = 0.265) did not predict the prevalence rates significantly.
The meta-analytical regression results enable the prediction of prevalence rates while taking account of the influence of multiple factors and hence offer a superior model over the earlier pooled analyses. In other words, the meta-regression model considers multiple predictors of mental health symptoms in a single model at the same time instead of the approach of considering one predictor at a time by pooled prevalence, the typical method to estimate the prevalence of mental health symptom in prior meta-analytical papers in COVID-19 literature.
Hence, based on the results of the meta-regression, we report the predicted prevalence rates of varying severity levels of the different mental health symptoms of frontline HCWs, general HCWs, and the general population. Table 4 show the predicted prevalence rates of mental health symptoms by populations, outcomes, and severity by the meta-analytical regression model. The prevalence rates vary greatly by the mental health outcomes and severity. The prevalence rates are lower when using a higher level of severity, which drives the heterogeneity of prevalence rate to a large degree. Among the different types of mental health outcomes, distress seems to be the most prevalent among all three populations.
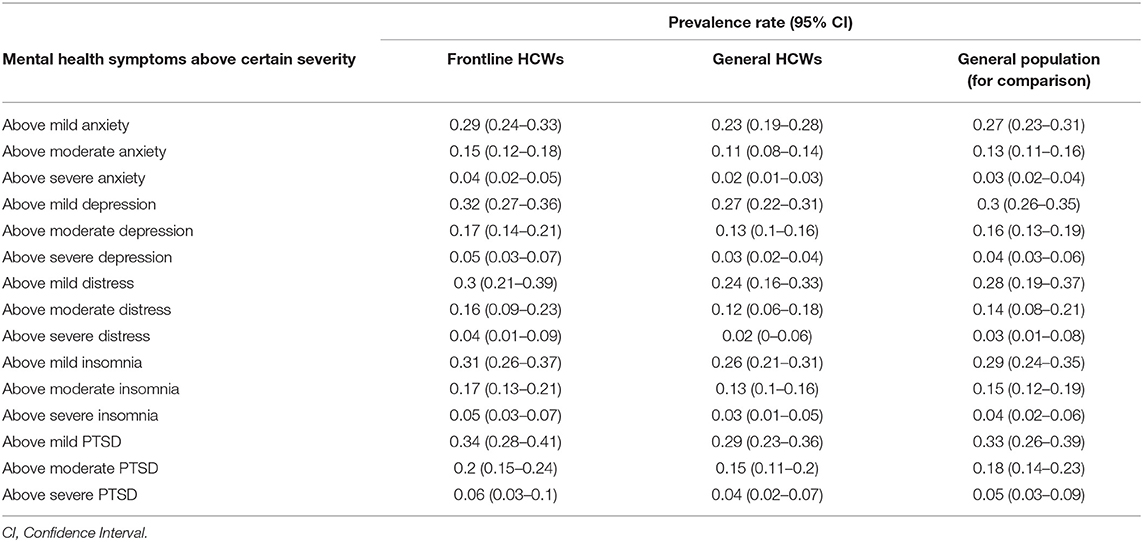
Table 4. The predicted prevalence rates of mental health symptoms by populations, outcomes, and severity by the meta-analytical regression model.
Sensitivity Analysis
Our meta-analytical regression model was able to take account of the impact of several factors, such as publication status (insignificant), sample size (insignificant), and article quality score (significant). Furthermore, we conducted our analysis with the exclusion of each study one-by-one from the meta-analytic model and found it did not significantly alter the findings. The visual inspection of the sensitivity plot however revealed that there is significant asymmetry. Figure 3 reports the DOI plot in combination with the Luis-Kanamori (LFK) index, which has higher sensitivity and power than a funnel plot (23, 24). An LFK index scores of ±1, between ±1 and ±2, or ±2 indicating “no asymmetry”, “minor asymmetry”, and “major asymmetry”, respectively, and hence the LFK index of 3.7 represents major asymmetry. Therefore, the presence of publication bias is likely.
Discussion
Our meta-regression analysis from a systematic review comprises 171 independent samples with 645,805 participants from 132 studies, at least an order of magnitude larger than the prior meta-analyses that included 7–50 studies with 2,123–62,382 participants (15, 16, 25–27). Moreover, prior meta-analyses examined the prevalence rates of mental health symptoms based on one level of the severity of symptoms (i.e., above mild), and we included articles that reported the prevalence at varying levels of severity of symptoms. Our meta-regression results based on multiple factors are consistent yet fine-tune the previous results, a comparison reveals that our pooled prevalence rates largely fall between the findings of previous meta-analyses, suggesting. For example, our findings show similar prevalence of depression (32 vs. 32%) but a higher prevalence of anxiety (29 vs. 24%) for frontline HCWs reported by Bareeqa et al. (15). Similarly, our findings show similar prevalence of anxiety for the general HCWs (23 vs. 23%), but a higher prevalence of depression (27 vs. 23%) in Pappa et al. (16). The two differences between our prevalence rates and the prior reports are statistically significant given the large sample size involved, and hence we significantly update the cumulative evidence on mental health prevalence rates in COVID-19.
Meta-Regression Findings
We were able to conduct meta-regression to account for the influence of multiple predictors at the same time to enable better prediction on the prevalence of each mental health symptom thanks to the large number of samples in China over a year of the COVID-19 crisis. The meta-regression evidence shows that several predictors are significantly associated with prevalence rates of mental symptoms during COVID-19, including the population, the severity and type of mental symptoms, sampling location, and study quality.
Frontline HCWs suffered more than general HCWs and the general population did across all five types of mental symptoms. It is also worth noting the general HCWs did not significantly differ from general populations across any mental symptoms. Such a result implies that whether a HCW is frontline could be a major factor in shaping her/his mental health, because of the risk of more direct exposure to the COVID crisis situation. In other words, the fact that general HCWs work in the medical field alone may not trigger much mental health symptoms than the general population has. Hence, our evidence suggests that policymakers need to prioritize frontline HCWs in particular in this ongoing pandemic. We call upon healthcare organizations to test specific psychological support intervention programs as well as mental health prevention plans to help HCWs (28).
The severity of mental symptoms, which has been unaccounted for in prior meta-analyses, was found to contribute greatly to the heterogeneity of prevalence rates, hence individual mental health papers need to pay special attention to the severity with clarity. Otherwise, researchers and practitioners might mix the severity of severe, moderate, and mild mental illness. Since prior meta-analyses largely examined the prevalence rates of mild mental health symptoms, yet psychiatrists care not only the mild symptoms, and the significant differences revealed by this study call for more meta-regression analyses on varying levels of severity to provide evidence for practitioners relevant to their concerns.
Among the five mental health symptoms examined, PTSD had the highest prevalence rates in both general and frontline HCWs. Our findings suggest that practitioners need to be aware and pay more attention to PTSD under the COVID-19 pandemic. Moreover, given that more than three-quarters of existing empirical studies focused on anxiety and depression, we call out for future research to focus on mental PTSD.
Past mental health research has reported inconsistent results on the relationship between individuals' mental symptoms and their locations. Some studies reported that mental symptoms increase along with the distance to the epicenter in the COVID-19 pandemic, known as “typhoon eye effect” (29–31). However, other findings have demonstrated an opposite effect, where mental symptoms decrease as the distance to the epicenter increases, known as the “ripple effect” (32, 33). Our accumulative evidence shows that people in the epicenter of China in Wuhan suffered less mental symptoms than those outside of Wuhan, lending support to the typhoon eye effect. This finding suggests future research to differentiate, report, and possibly model sampling locations based on the epicenter of a pandemic to enable better geographical identification of mental symptoms (34–36).
Our findings that the samples in papers with higher quality tend to find higher prevalent rates of mental symptoms suggest study quality may matter. Particularly, future meta-analysis may pay attention to the representativeness of sampling, the response rate, etc., to better account for the heterogeneity in the pooled prevalence rates.
As the COVID-19 epidemic evolves, we expected the mental symptoms may change over time. However, the evidence of meta-regression using time as a predictor failed to reveal significant effect, and a potential reason might be the development of COVID-19 in various parts of China happened at varying paces, and more refined studies are needed to uncover the change of prevalence rates effect over time across COVID waves (37).
Study Limitations and Future Research
This research has a few limitations. First, the validity of our findings rests upon the quality and reporting of the original studies. While we paid extra attention to the severity, the cutoff points, and the ways in which individual articles used this information, the multitude of varying practices contributes to additional noise and variance in the analysis. Second, since we included studies in English, which may result in some biases. Third, 97.9% of the primary studies included were cross-sectional surveys, and we call for more cohort studies to examine the effect of time. Fourth, we examine the major adult population of interest, and future research could examine other populations that could be vulnerable, such as hospitality workers, professional athletes, and managers (38–41). As research on COVID pandemic continues to develop, future research may also explore other factors, such as age, health conditions, COVID testing availability, and conspiracy belief in COVID (42–44).
Finally, we only focus on studies that collected data in one country (China) to reduce the heterogeneity of different situations across countries, and we call for future meta-analyses in other countries or regions where data are sufficient -see meta-analyses on several regions including Africa, Eastern Europe, Latin America, South Asia, Southeast Asia, and Spain (45–50).
Conclusion
This meta-regression analysis takes account of several heterogeneities to analyze the evidence on the prevalence rates of mental health symptoms of healthcare workers under the COVID-19 crisis to provide a foundation of the past research and to guide future effort. Our findings suggest further research and practices on mental health symptoms need to better specify and account for the heterogeneous factors identified as such heterogeneity contribute to significant differences of the prevalence of mental health symptoms reported.
Author Contributions
XC: investigation, data curation, visualization, writing—original draft, writing—review and editing, and project administration. JC: conceptualization, methodology, validation, formal analysis, investigation, resources, data curation, visualization, writing—original draft, writing—review and editing, and supervision. JL: investigation, writing—review and editing, and resources. MZ, RD, ZD, YY, and LT: investigation (Data). RZ, WC, and PL: investigation. SZ: conceptualization, methodology, validation, formal analysis, investigation, data curation, writing—original draft, writing—review and editing, and supervision. XC, JC, and SZ: co-lead this project. All authors were involved in approving the manuscript. All authors contributed to the article and approved the submitted version.
Funding
This work was supported by the National Natural Science Foundation of China (71772103).
Conflict of Interest
The authors declare that the research was conducted in the absence of any commercial or financial relationships that could be construed as a potential conflict of interest.
Publisher's Note
All claims expressed in this article are solely those of the authors and do not necessarily represent those of their affiliated organizations, or those of the publisher, the editors and the reviewers. Any product that may be evaluated in this article, or claim that may be made by its manufacturer, is not guaranteed or endorsed by the publisher.
Acknowledgments
We thank Richard Z. Chen, Bryan Z. Chen, Haixing Zheng, Shaokun Xu, Fei Liang, and Zhehong Xu for their help.
Supplementary Material
The Supplementary Material for this article can be found online at: https://www.frontiersin.org/articles/10.3389/fpsyt.2022.833865/full#supplementary-material
References
1. WHO. World Health Organization Coronavirus Disease (Covid-19) Dashboard. (2020). Available online at: https://covid19.who.int/ (accessed January 1, 2021).
2. Zhang SX, Wang Y, Rauch A, Wei F. Unprecedented disruption of lives and work: health, distress and life satisfaction of working adults in China one month into the Covid-19 outbreak. Psychiatry Res. (2020) 288:112958. doi: 10.1016/j.psychres.2020.112958
3. Douglas M, Katikireddi SV, Taulbut M, McKee M, McCartney G. Mitigating the wider health effects of Covid-19 pandemic response. BMJ. (2020) 369. doi: 10.1136/bmj.m1557
4. Braquehais MD, Vargas-Cáceres S, Gómez-Durán E, Nieva G, Valero S, Casas M, et al. The impact of the Covid-19 pandemic on the mental health of healthcare professionals. QJM Int J Med. (2020) 113:613–7. doi: 10.1093/qjmed/hcaa207
5. Taylor S, Landry CA, Rachor GS, Paluszek MM, Asmundson GJ. Fear and avoidance of healthcare workers: an important, under-recognized form of stigmatization during the Covid-19 pandemic. J Anxiety Disord. (2020) 75:102289. doi: 10.1016/j.janxdis.2020.102289
6. Lai J, Ma S, Wang Y, Cai Z, Hu J, Wei N, et al. Factors associated with mental health outcomes among health care workers exposed to coronavirus disease 2019. JAMA Netw Open. (2020) 3:e203976-e. doi: 10.1001/jamanetworkopen.2020.3976
7. Chen Q, Liang M, Li Y, Guo J, Fei D, Wang L, et al. Mental health care for medical staff in China during the Covid-19 outbreak. Lancet Psychiatry. (2020) 7:e15–6. doi: 10.1016/S2215-0366(20)30078-X
8. Chirico F, Ferrari G, Nucera G, Szarpak L, Crescenzo P, Ilesanmi O. Prevalence of anxiety, depression, burnout syndrome, and mental health disorders among healthcare workers during the Covid-19 pandemic: a rapid umbrella review of systematic reviews. J Health Soc Sci. (2021) 6:209–20. doi: 10.19204/2021/prvl7
9. Menon V, Padhy SK. Ethical dilemmas faced by health care workers during Covid-19 pandemic: issues, implications and suggestions. Asian J Psychiatry. (2020) 51:102116. doi: 10.1016/j.ajp.2020.102116
10. Greenberg N, Docherty M, Gnanapragasam S, Wessely S. Managing mental health challenges faced by healthcare workers during Covid-19 pandemic. BMJ. (2020) 368:m1211. doi: 10.1136/bmj.m1211
11. Greenberg N. Mental health of health-care workers in the Covid-19 era. Nat Rev Nephrol. (2020) 16:425–6. doi: 10.1038/s41581-020-0314-5
12. Muller RAE, Stensland RSØ, van de Velde RSJPr. The mental health impact of the Covid-19 pandemic on healthcare workers, and interventions to help them: a rapid systematic review. Psychiatry Res. (2020) 113441. doi: 10.1016/j.psychres.2020.113441
13. Jahanshahi AA, Dinani MM, Madavani AN, Li J, Zhang SX. The distress of iranian adults during the Covid-19 pandemic - more distressed than the Chinese and with different predictors. Brain Behav Immun. (2020) 87:124–5. Epub 2020/05/04. doi: 10.1016/j.bbi.2020.04.081
14. Zhuo K, Gao C, Wang X, Zhang C, Wang Z. Stress and sleep: a survey based on wearable sleep trackers among medical and nursing staff in Wuhan during the Covid-19 pandemic. Gen Psychiatry. (2020) 33:e100260. doi: 10.1136/gpsych-2020-100260
15. Bareeqa SB, Ahmed SI, Samar SS, Yasin W, Zehra S, Monese GM, et al. Prevalence of depression, anxiety and stress in China during Covid-19 pandemic: a systematic review with meta-analysis. Int J Psychiatry Med. (2020) 56:210–27. doi: 10.1177/0091217420978005
16. Pappa S, Ntella V, Giannakas T, Giannakoulis VG, Papoutsi E, Katsaounou P. Prevalence of depression, anxiety, and insomnia among healthcare workers during the Covid-19 pandemic: a systematic review and meta-analysis. Brain Behav Immun. (2020) 88:901–7. doi: 10.1016/j.bbi.2020.05.026
17. Burki T. China's successful control of Covid-19. Lancet Infect Dis. (2020) 20:1240–1. doi: 10.1016/S1473-3099(20)30800-8
18. de Pablo GS, Serrano JV, Catalan A, Arango C, Moreno C, Ferre F, et al. Impact of coronavirus syndromes on physical and mental health of health care workers: systematic review and meta-analysis. J Affect Disord. (2020) 275:48–57. doi: 10.1016/j.jad.2020.06.022
19. Usher K, Jackson D, Durkin J, Gyamfi N, Bhullar N. Pandemic-related behaviours and psychological outcomes; a rapid literature review to explain Covid-19 behaviours. Int J Ment Health Nurs. (2020) 29:1018–34. doi: 10.1111/inm.12790
20. Hong QN, Pluye P, Fàbregues S, Bartlett G, Boardman F, Cargo M, et al. Mixed Methods Appraisal Tool (Mmat), Version 2018. Registration of Copyright (# 1148552), Canadian Intellectual Property Office, Industry Canada. Canadian Intellectual Property Office (2018).
21. DerSimonian R, Laird N. Meta-analysis in clinical trials. Control Clin Trials. (1986) 7:177–88. doi: 10.1016/0197-2456(86)90046-2
22. Higgins JP, Thomas J, Chandler J, Cumpston M, Li T, Page MJ, et al. Cochrane Handbook for Systematic Reviews of Interventions. John Wiley & Sons (2019).
23. Mboua PC, Keubo FRN, Fouaka SGN. Anxiety and depression associated with the management of Covid-19 among healthcare personnel in Cameroon. L'Evolution Psychiatrique. (2020) 86:131–9. doi: 10.1016/j.evopsy.2020.11.002
24. Teshome A, Glagn M, Shegaze M, Tekabe B, Getie A, Assefa G, et al. Generalized anxiety disorder and its associated factors among health care workers fighting Covid-19 in Southern Ethiopia. Psychol Res Behav Manage. (2020) 13:907. doi: 10.2147/PRBM.S282822
25. Ren X, Huang W, Pan H, Huang T, Wang X, Ma Y. Mental health during the Covid-19 outbreak in China: a meta-analysis. Psychiatr Q. (2020) 91:1033–45. doi: 10.1007/s11126-020-09796-5
26. Salari N, Khazaie H, Hosseinian-Far A, Ghasemi H, Mohammadi M, Shohaimi S, et al. The prevalence of sleep disturbances among physicians and nurses facing the Covid-19 patients: a systematic review and meta-analysis. Global Health. (2020) 16:1–14. doi: 10.1186/s12992-020-00620-0
27. Krishnamoorthy Y, Nagarajan R, Saya GK, Menon V. Prevalence of psychological morbidities among general population, healthcare workers and Covid-19 patients amidst the Covid-19 pandemic: a systematic review and meta-analysis. Psychiatry Res. (2020) 293:113382. doi: 10.1016/j.psychres.2020.113382
28. Chirico F, Ferrari G. Role of the workplace in implementing mental health interventions for high-risk groups among the working age population after the Covid-19 pandemic. J Health Soc Sci. (2021) 6:145–50. doi: 10.19204/2021/rlft1
29. Tang PM, Zhang SX, Li CH, Wei F. Geographical identification of the vulnerable groups during Covid-19 crisis: the typhoon eye effect and its boundary conditions. medRxiv []. (2020). doi: 10.1101/2020.04.28.20083667
30. Zhang L, Ma M, Li D, Xin Z. The psychological typhoon eye effect during the Covid-19 outbreak in China: the role of coping efficacy and perceived threat. Global Health. (2020) 16:1–10. doi: 10.1186/s12992-020-00626-8
31. Zhang SX, Wang Y, Jahanshahi AA, Li J, Schmitt VGH. Early evidence and predictors of mental distress of adults one month in the Covid-19 epidemic in Brazil. J Psychosom Res. (2021) 142:110366. doi: 10.1016/j.jpsychores.2021.110366
32. Zhang Y, Cao X, Wang P, Wang G, Lei G, Shou Z, et al. Emotional “Inflection Point” in public health emergencies with the 2019 new Coronavirus pneumonia (Ncp) in China. J Affect Disord. (2020) 276:797–803. doi: 10.1016/j.jad.2020.07.097
33. Huang L, Lei W, Xu F, Liu H, Yu L. Emotional responses and coping strategies in nurses and nursing students during Covid-19 outbreak: a comparative study. PLoS One. (2020) 15:e0237303. Epub 2020/08/09. doi: 10.1371/journal.pone.0237303
34. Zhang SX, Huang H, Wei F. Geographical distance to the epicenter of Covid-19 predicts the burnout of the working population: ripple effect or typhoon eye effect? Psychiatry Res. (2020) 288:112998. doi: 10.1016/j.psychres.2020.112998
35. Zhang SX, Wang Y, Jahanshahi AA, Schmitt VGH. First study on mental distress in Brazil during the Covid-19 crisis. medRxiv. (2020). doi: 10.1101/2020.04.18.20070896
36. Yáñez JA, Jahanshahi AA, Alvarez-Risco A, Li J, Zhang SX. Anxiety, distress, and turnover intention of healthcare workers in Peru by their distance to the epicenter during the Covid-19 crisis. Am J Trop Med Hyg. (2020) 103:1614–20. doi: 10.4269/ajtmh.20-0800
37. Zhang SX, Marioli FA, Gao R, Wang S. A second wave? What do people mean by Covid waves?–a working definition of epidemic waves. Risk Manage Healthc Policy. (2021) 14:3775. doi: 10.2147/RMHP.S326051
38. Sun S, Zhang SX, Jahanshahi AA, Jahanshahi M. Drilling under the Covid-19 pandemic: a diary study of professional football players' mental health and workout performance. Stress Health. (2021) 1–16. doi: 10.1002/smi.3059
39. Li L, Zhang SX, Graf-Vlachy L. Predicting managers' mental health across countries using country-level Covid-19 statistics. medRxiv. (2021). doi: 10.1101/2021.07.18.21260567
40. Graf-Vlachy L, Sun S, Zhang SX. Predictors of managers' mental health during the Covid-19 pandemic. Eur J Psychotraumatol. (2020) 11:1834195. doi: 10.1080/20008198.2020.1834195
41. Yan J, Kim S, Zhang SX, Foo M-D, Alvarez-Risco A, Del-Aguila-Arcentales S, et al. Hospitality workers' Covid-19 risk perception and depression: a contingent model based on transactional theory of stress model. Int J Hospital Manage. (2021) 95:102935. doi: 10.1016/j.ijhm.2021.102935
42. Chen J, Zhang SX, Wang Y, Afshar Jahanshahi A, Mokhtari Dinani M, Nazarian Madavani A, et al. The relationship between age and mental health among adults in Iran during the Covid-19 pandemic. Int J Mental Health Addict. (2021). doi: 10.1007/s11469-021-00571-6
43. Chen X, Zhang SX, Jahanshahi AA, Alvarez-Risco A, Dai H, Li J, et al. Belief in conspiracy theory about Covid-19 predicts mental health and well-being: a study of healthcare staff in Ecuador. MedRxiv. (2020). doi: 10.1101/2020.05.26.20113258
44. Dai H, Zhang SX, Looi KH, Su R, Li JJIjoer, health p. Perception of health conditions and test availability as predictors of adults' mental health during the Covid-19 pandemic: a survey study of adults in Malaysia. Int J Environ Res Public Health. (2020) 17:5498. doi: 10.3390/ijerph17155498
45. Zhang SX, Batra K, Liu T, Dong RK, Xu W, Yin A, et al. Meta-analytical evidence on mental disorder symptoms during the Covid-19 pandemic in Latin America. SSRN. (2021). doi: 10.2139/ssrn.3858820
46. Chen RZ, Zhang SX, Xu W, Yin A, Dong RK, Chen BZ, et al. A systematic review and meta-analysis on mental illness symptoms in Spain in the Covid-19 crisis. medRxiv. (2021). doi: 10.1101/2021.04.11.21255274
47. Zhang SX, Chen J. Scientific evidence on mental health in key regions under the Covid-19 pandemic–meta-analytical evidence from Africa, Asia, China, Eastern Europe, Latin America, South Asia, Southeast Asia, and Spain. Eur J Psychotraumatol. (2021) 12:2001192. doi: 10.1080/20008198.2021.2001192
48. Chen J, Farah N, Dong RK, Chen RZ, Xu W, Yin J, et al. Mental health during the Covid-19 crisis in Africa: a systematic review and meta-analysis. Int J Environ Res Public Health. (2021) 18:10604. doi: 10.3390/ijerph182010604
49. Pappa S, Chen J, Barnett J, Chang A, Dong RK, Xu W, et al. A systematic review and meta-analysis of the mental health symptoms during the Covid-19 pandemic in Southeast Asia. Psychiatry Clin Neurosci. (2021). doi: 10.1111/pcn.13306
Keywords: meta-regression, systematic review, meta-analysis, COVID-19, mental health, healthcare workers, frontline healthcare workers
Citation: Chen X, Chen J, Zhang M, Dong RK, Li J, Dong Z, Ye Y, Tong L, Zhao R, Cao W, Li P and Zhang SX (2022) Meta-Regression on the Heterogenous Factors Contributing to the Prevalence of Mental Health Symptoms During the COVID-19 Crisis Among Healthcare Workers. Front. Psychiatry 13:833865. doi: 10.3389/fpsyt.2022.833865
Received: 12 December 2021; Accepted: 21 February 2022;
Published: 18 March 2022.
Edited by:
Ashwani Kumar Mishra, All India Institute of Medical Sciences, IndiaReviewed by:
Francesco Chirico, Catholic University of the Sacred Heart, ItalyChandra Bhushan Tripathi, University of Delhi, India
Copyright © 2022 Chen, Chen, Zhang, Dong, Li, Dong, Ye, Tong, Zhao, Cao, Li and Zhang. This is an open-access article distributed under the terms of the Creative Commons Attribution License (CC BY). The use, distribution or reproduction in other forums is permitted, provided the original author(s) and the copyright owner(s) are credited and that the original publication in this journal is cited, in accordance with accepted academic practice. No use, distribution or reproduction is permitted which does not comply with these terms.
*Correspondence: Stephen X. Zhang, stephen.x.zhang@gmail.com