- 1Department of Psychology, University of Rochester, Rochester, NY, United States
- 2Department of Neuroscience, University of Rochester Medical Center, Rochester, NY, United States
Urban living is a growing worldwide phenomenon with more than two-thirds of people expected to live in cities by 2050. Although there are many benefits to living in an urban environment, urbanicity has also been associated with deleterious health outcomes, including increased risk for psychotic outcomes particularly when the urban exposure occurs in pre-adolescence. However, the mechanisms underlying this association is unclear. Here, we utilize one-year follow-up data from a large (N=7,979), nationwide study of pre-adolescence in the United States to clarify why urbanicity (i.e., census-tract population density) might impact psychotic-like experiences (PLE) by looking at the indirect effect of eight candidate urbanicity-related physical (e.g., pollution) and social (e.g., poverty) exposures. Consistent with other work, we found that of the evaluated exposures related to urbanicity, several were also related to increased number of PLE: PM2.5, proximity to roads, census-level homes at-risk for exposure to lead paint, census-level poverty, and census-level income-disparity. These same urban-related exposures were also related to the persistence of PLE after 1 year, but not new onset of PLE. Mediation analysis revealed that a substantial proportion the urbanicity-PLE association (number and persistence) could be explained by PM2.5 (23–44%), families in poverty (68–93%), and income disparity (67–80%). Together, these findings suggest that specific urban-related exposures contribute to the existence and maintenance, but not onset of PLE, which might help to explain why those in urban environments are disproportionately at-risk for psychosis and point toward areas for public health intervention.
Introduction
City living is an increasing societal phenomenon. The World Health Organization estimates that 68% of the world's population will reside in urban areas by the year 2050, compared to 55% of the population in 2018 (1). Those migrating to urban environments often do so to for better access to employment opportunities, education, health care, public utilities, among other benefits (2–4). While city living offers many social, economic, and health benefits, it also has significant drawbacks in these areas, making it critical to understand the potential net consequences of increasing global urbanization (3, 4). Of particular interest has been the impact of urbanicity on mental health. An already robust and growing body of retrospective and prospective research has shown that urban (as opposed to rural) birth, upbringing, or living increases risk for psychosis (5–12), and does so in a dose-response manner (13, 14). Meta-analytic estimates have shown that the risk for psychosis approximately doubles in urban environments (15–18), and population-based cohort studies have shown that urbanicity explains 30% or more of incident cases (8, 9), making the impact of urbanicity on psychosis risk as large as or larger than other known non-genetic environmental risk factors (19). Importantly, the association between urbanicity and psychosis remains after controlling for many potential confounding factors, including drug use and genetic risk, (16, 17, 20, 21), and also cannot be explained by selective migration (22). Other studies have demonstrated that changes in urbanicity prospectively predict changes in psychosis risk later in life (7, 14). Thus, together, the available data suggest that the association between urbanicity and psychosis could be causal in nature (17, 23), perhaps by way of an interaction between urbanicity and constitutional vulnerability factors (e.g., genetic vulnerability, birth complications) (17, 24).
Despite the clear etiological importance of the urban environment for psychosis, one of the most critical issues related to this association remains poorly understood: what is the mechanism through which urbanicity impacts risk? Urban environments involve a wide variety of physical (e.g., pollution) and social (e.g., poverty) exposures. It may be these exposures that explain, in part, why urban environments impact psychosis risk (20, 25, 26). To this point, a variety of urban-related physical exposures have been shown to increase risk for and exacerbate psychotic symptoms including air pollution (27–31), and xenobiotic heavy metal (i.e., lead) exposure (32–34). Similarly, many urban-related social exposures have been associated with psychosis risk and exacerbation, including socioeconomic disparities (35–37), exposure to violence and trauma (38, 39), and exposure to social exclusion, discrimination, and racism due to having an ethnic, migrant, sexual, or gender minority group membership (40–42).
When taken together, the possibility that the urban environment may impact psychosis risk indirectly through a variety of pathways seems strong. In line with this idea, some of the urban-related exposures have been shown to mediate the relation between urbanicity and psychosis risk in both child and adult samples. For example, on physical exposures, various air pollutants and level of traffic have been shown to mediate or attenuate the association between urbanicity and psychosis (43, 44). Similarly, for social exposures, such as low social cohesion and crime victimization, have been shown to moderate the relationship between childhood and adolescent psychotic symptoms and urbanicity (10, 45). In similar studies, the impact of racial/ethnic minority status (the hypothesized exposure being racism and discrimination) on increased psychosis risk remains even when controlling for the effect of urbanicity (13). Further, some of these findings have been shown to be specific to psychosis over other mental health outcomes (10, 45). Despite these findings, the literature on how urban exposures may mediate the urbanicity-psychosis risk association is limited. On this point, to our knowledge, there are no studies evaluating the indirect effect of both physical and social exposures in the same study.
Thus, the current study aims to further our understanding of the urbanicity-PLE association in pre-adolescence by evaluating whether urbanicity, defined by census-tract population density (people per km2), impacts PLE through a suite of candidate physical and social urban-related exposures in a large, nationally representative pre-adolescent sample. In doing so, we aimed to better understand the mechanisms through which urbanicity relates to PLE. Evaluating these issues in late childhood and early adolescence is critical given that this represents the developmental period where urbanicity seems to impact psychosis risk (7, 14), as well as when many of the urban-related exposures (e.g., lead) may be most potent in their impact [e.g., (27, 46)]. Further, urban living is associated with increased PLE in childhood and early adolescence (47) as well as the persistence of PLE in adulthood (48) making early life urbanicity an important marker of concurrent and prospective psychosis risk.
To address these aims, we utilized baseline and one-year follow-up data from the Adolescent Brain Cognitive Development (ABCD) study (49); abcdstudy.org; a longitudinal, nation-wide study on the development of nearly 12,000 children across the United States. This study follows children starting at ages nine and ten, and includes information on child psychotic-like experiences (50, 51) (PLE), as well as demographic, social, and geolocation data (52, 53). By using the ABCD dataset, we are able to explore the associations between urbanicity, candidate urban-related exposures, and later PLE. Further, in line with other work (10, 43), we are able to test the hypothesis that urban-related exposures mediate the urbanicity-PLE association. A recent study examined similar associations in the ABCD dataset, as well as the mediating role of brain volume using all baseline (i.e., cross-sectional) data (54). Importantly, here, our main goal was to investigate the mediating role of urban-related exposures (instead of brain volume), and to do so using one-year follow-up PLE data, which allowed us to look at longitudinal changes in PLE as a function of urbanicity and urban-related exposures. First, we evaluated whether urbanicity was associated with later PLE in pre-adolescence, as well as the specificity of this association by evaluating urbanicity's association with later externalizing and internalizing symptoms. Second, we evaluated whether urbanicity was associated with a broad range of candidate physical (i.e., air pollution, lead exposure), and social exposures (i.e., socioeconomic disadvantage) available in the ABCD dataset. Third, we evaluated whether those exposures related to urbanicity were also related to later PLE. Finally, we evaluated whether urbanicity was related to PLE by way of urban-exposures; that is, whether the physical and social urban exposures that were related to urbanicity and PLE, mediated the urbanicity-PLE association.
Methods
Participants
Data were obtained from participants in the Adolescent Brain Cognitive Development (ABCD) study; a longitudinal study following children beginning at ages nine- and ten-years from 21 research sites across the United States. The sociodemographic makeup of the sample closely matches that of the United States as a whole, with a slight oversampling of Black/African American and other race children that corresponds to a slight under-sampling of White and Hispanic children (49). We used baseline and one-year follow-up data from the ABCD Data Release 3.0, which was accessed through the National Institute of Health Data Archive (https://nda.nih.gov/abcd). Of the 11,878 participants who contributed baseline data, 11,235 participants (95%) contributed one-year follow-up data. We then removed 3,256 participants who were missing data on one or more of the baseline or one-year follow-up variables described below, leaving a final sample of 7,979 participants with complete datasets (Table 1). The final sample matched the excluded sample on all demographics except for race, where a greater proportion of data was missing for Black respondents and a lesser proportion of data was missing for White respondents (Table S1).
Measures
Urbanicity
Consistent with other work [e.g., (11)] urbanicity was defined as census-tract population density (persons per km2) at baseline. At baseline, respondents were able to identify up to six current residential addresses and the percentage of time they spent at each address. The ABCD dataset provides geolocation data for only current addresses and not previous addresses. For geolocation data to be appended to the ABCD dataset, respondents had to provide valid addresses. The ability to identify multiple residential addresses, allowed respondents to provide all the residences at which the pre-adolescent spent significant time. This takes into account pre-adolescents who, for example, have parents living separately, or who spend a significant time with a caregiver that is not their primary caregiver (e.g., grandparents, extended family, etc.). However, only one valid address was available for almost all (99%) included respondents, with the remaining offering two. Using the available valid addresses, a single score was created by weighting each residential address population density value by the percentage of time spent at that address. For respondents who accounted for more or less than 100% of their time across all usable addresses, a simple average was used.
Psychotic-Like Experiences and Psychopathology
PLE were assessed with the Prodromal Questionnaire-Brief Child Version [PQ-BC; (50)] completed at the 1-year follow-up. The PQ-BC is a 21-item self-report questionnaire modified and validated for children from the Prodromal Questionnaire-Brief (56) that assess a range of PLE such as unusual thought content, suspiciousness/persecutory ideas, grandiosity, and perceptual aberrations (50). Participants first indicated whether they had experienced the PLE. Those who indicated that they had experienced the PLE then indicated whether the PLE was bothersome. For each PLE that was identified as bothersome, participants rated how bothersome the experience was using a 1 to 5 visual response scale with higher scores denoting higher distress. Following other studies using the PQ-BC (50, 51, 57), total score and distress scores were used. The total score was calculated by summing the number of items endorsed (possible score: 0–21). The distress score was calculated by weighting the total score by the level of distress. A score of 0 indicates no PLE endorsed, 1 indicates one PLE endorsed with no distress, and scores of 2–6 indicate that a PLE was endorsed with some distress (possible score: 0-126). Prior work has demonstrated the PQ-BC to exhibit construct validity and adequate psychometric properties (50, 51). Because findings with the total and distress score were similar, and the total score more closely resembles the PLE score derived in other similar studies evaluating the impact of urbanicity on PLE through urban-related exposures (10, 43), we focused on the total score in the main text. Analyses with the distress score are provided in the supplement.
Following the analytical approach of others examining changes in PLE in the ABCD dataset (58), we created dichotomous outcome variables representing (a) the persistence of PLE from baseline to one-year follow-up and (b) the onset of new PLE at one-year follow-up. These variables were created by first evaluating the presence of any PLE at baseline (0 = no, 1 = yes) and follow-up (0 = no, 1 = yes). PLE persistence and onset were calculated such that persistence indicates the presence of at least one PLE at both baseline and follow-up (baseline = 1, follow-up = 1), and onset indicates the lack of any PLE at baseline and presence of at least one PLE at follow-up (baseline=0, follow-up=1). Analyses utilizing persistence or onset of PLE-associated distress are reported in the supplement.
To assess the specificity of the association between urbanicity and later PLE, we evaluated externalizing and internalizing symptoms using the respective broad band scores from the Child Behavior Checklist [CBCL; (59)]. The externalizing broad-band score indexes rule-breaking and aggressive behaviors, while the internalizing broad-band score indexes anxiety, depressed symptoms, and somatic complaints.
Candidate Mediators
Candidate mediators were selected from the baseline ABCD dataset if the variable (1) in theory related to city living, (2) was associated with increased risk for PLE or SSDs, and (3) in theory could explain the urbanicity-PLE association. Our selection of variables was such that the candidate mediators were physical (e.g., pollution) or social (e.g., violence) in nature, allowing us to test the impact of two classes of urban-associated exposures with potentially distinct mechanisms. We note that there were several other variables in the ABCD dataset that met these criteria but were not included either because other (non-composite) variables more directly indexed the exposure of interest (e.g., assessing families in poverty vs. area deprivation) or due to extremely low base rates (e.g., using marijuana sales vs. drug use). We identified 8 such candidate mediators (described below), with scores calculated using the same weighting procedure as for calculating urbanicity.
We included three measures of census-area air pollution: (1) annual average particulate matter with a diameter of 2.5 micrometers or less (PM2.5) levels for 2016 at 10 x 10 km2, (2) three-year ground-level average of nitrogen dioxide (NO2) at 10 x 10 km2, and (3) proximity to major roads, in meters. Both PM2.5 and NO2, have shown a relationship with higher incidence of psychotic disorders (27) and PLE (43), and with worsening psychotic symptoms (29, 30, 60–62). Similarly, proximity to major roads was used as a measure of traffic-related pollution, which is also associated with schizophrenia risk (44). We included one measure of census-area lead exposure: the estimated percentage of houses at-risk for lead exposure due to lead paint, which also has been linked to psychosis risk (32–34). Finally, using data from the US Federal Bureau of Investigation's Uniform Crime Reports, we included the number of arrests for marijuana sales in a census area as a proxy for use and exposure to marijuana, which also has been implicated as a component cause of psychosis (63–65).
We included two measures of census-area economic adversity: (1) the percentage of families below the poverty line (“families in poverty”), and (2) income disparity as defined by Singh (55) as the log of 100 x ratio of households with $50,000 in annual income). Both factors have been linked to the incidence and symptoms of psychosis (66–69). On social adversity, we included the total number of adult violent offenses in a participant's census-area as crime victimization has been shown to prospectively increase risk for PLE (10).
Data Analysis
Associations Between Urbanicity, Psychopathology, and Candidate Urban-Exposures
Planned analyses were preregistered on the Open Science Framework (https://osf.io/rdsuj). Data analysis was performed using R (70). Following the analysis strategy of Newbury et al. (10), we first evaluated the association between urbanicity and psychopathology 1 year after baseline. PLE outcomes included PLE total score, persistence of PLE (dichotomous), and onset of PLE. We also examined associations between urbanicity and externalizing and internalizing symptoms to test for specificity. Second, we examined the association between urbanicity and the 8 identified exposures that may account for the association between urbanicity and psychopathology. Third, we examined the association between psychopathology and those exposures that we found were related to urbanicity.
In order to account for the nested structure of the data whereby the participant was nested within family and site, we used linear mixed-effects models for continuous outcome variables and generalized mixed-effects models for dichotomous outcome variables using the lme4 package (71). These models included random intercepts for family and site. Results are reported as standardized beta coefficients for continuous outcomes and odds ratios for dichotomous outcomes along with 95% CIs, p values, and false-discovery rate (FDR) adjusted p values corrected for each group of tests. Associations are considered unexpected under the null hypothesis when FDR-adjusted p < 0.05. Analyses included age, sex, and familial risk for the relevant form of psychopathology outcome (i.e., psychosis, externalizing disorders, or internalizing disorders) as covariates.
A goal of this study was to maximize comparability with prior studies [e.g., (10, 43, 54)] thus, similar to those studies, we did not include race and ethnicity as covariates. Due to historical and current systematic racism in the United States, there is a disproportionate representation of racial and ethnic minorities in urban areas and disproportionate experiences with adverse urban-related exposures. This confounding would have made it difficult to tease apart the impact of race/ethnicity vs. the putative PLE risk factors investigated here. However, for those interested, sensitivity analyses where race/ethnicity are included as covariates in statistically significant mediation models are available in the supplement (Tables S4, S5).
Mediation Analyses
Lastly, of the exposures that were found to be associated with urbanicity and PLE, we evaluated whether they accounted for the urbanicity-PLE association; that is, whether these exposures mediated the relation between urbanicity and PLE. In order to simplify models and avoid non-convergence, we split the sample by family, creating one dataset (N = 6,775) which contained only one child from families in which more than one child from the family was represented. Because our hypotheses concerned level 1 (i.e., within-person) effects, and all variables were measured at level 1, we conducted a single-level mediation model using the lavaan package (72), and accounted for the clustering of data due to site by generating cluster-robust parameter estimates for continuous PLE outcomes. lavaan does not support cluster-robust parameter estimate analyses for categorical outcomes so the mediation models using persistence and onset as the outcomes did not take into account the nesting of data within site. We used a Monte Carlo test of mediation (73, 74) using the semTools package (75) by evaluating the 95% CI generated from 50,000 simulated values of the indirect effect. We similarly included age, sex, and familial risk for psychosis as covariates in these models. As mentioned, sensitivity analyses with race/ethnicity as covariates are available in the supplement (Tables S4, S5). As a measure of effect size, we provide the absolute proportion mediated (76), which denotes the proportion of the total effect that can be attributed to the indirect effect [i.e., a*b/(a*b+c); Figure 1].
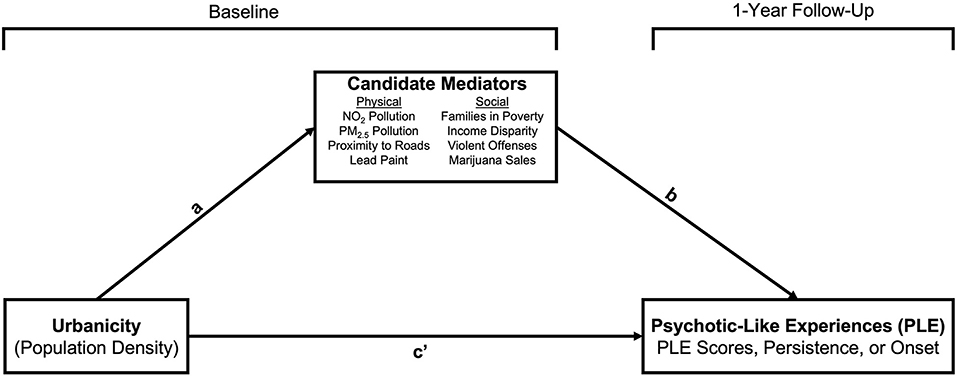
Figure 1. Mediation model. Age (in months) and sex-at-birth (M>F) were included as covariates on paths a and b.
Results
Urbanicity and Psychopathology
First, we evaluated whether similar to other reports, greater urbanicity was associated with greater later-PLE in a pre-adolescent sample. In line with this other work, greater urbanicity was associated with more PLE measured one-year later (Table 2). On the longitudinal nature of the association, we found that greater urbanicity was associated with PLE persistence, but not PLE onset. PLE distress variables showed the same pattern. Since PLE total onset was not associated with urbanicity, this variable was dropped from further analysis.
To evaluate the specificity of the association between urbanicity and PLE in pre-adolescence, we also tested the association between urbanicity and other forms of psychopathology, namely, externalizing and internalizing symptoms. Neither externalizing nor internalizing symptoms were associated with urbanicity (Table 2). These variables too were dropped from further analyses.
Urbanicity and Candidate Urban Exposures
Next, we evaluated which of the exposures selected were in fact concurrently related to urbanicity in our sample. All exposures were associated with urbanicity in the expected directions with the magnitude of association being largest with homes at risk for exposure to lead paint, families below the poverty line, and income disparity (Table 2).
PLE and Urban Exposures
Next, we evaluated the association between later PLE and all of the exposures that were linked to urbanicity. PLE total was associated with, in order of increasing magnitude, proximity to roads, homes at risk for exposure to lead paint, PM2.5, families in poverty, and income disparity, all in the expected direction (Table 3). While PLE total was also associated with NO2, this association did not survive FDR-correction, and as a result was not included in further analyses. The same pattern of findings was found for PLE total persistence.
The Impact of Urbanicity on PLE Through Urban-Related Exposures
Finally, we evaluated whether the association between urbanicity and later PLE could be explained by urban exposures; specifically, those exposures that were independently associated with both urbanicity and later PLE in our sample (specifically, PLE total score and PLE total persistence). The association between each PLE outcome and urbanicity were tested with the five previously identified urban-related exposures: PM2.5 pollution, proximity to roads, homes at-risk for lead paint, families in poverty, and income disparity. Monte Carlo mediation tests of the indirect effect demonstrated that the association between urbanicity and PLE total was mediated by PM2.5, accounting for 23.0% of the association, families in poverty, accounting for 67.7% of the association, and income disparity, accounting for 66.6% of the association (Table 4). Similarly, the association between urbanicity and PLE total persistence was mediated by PM2.5, accounting for 44.1% of the association, families in poverty, accounting for 93.0% of the association, and income disparity, accounting for 79.8% of the association. Neither proximity to roads nor homes at-risk for lead paint significantly mediated the association between PLE outcome and urbanicity. Sensitivity analyses in which race and ethnicity were included as covariates demonstrated that the magnitude of the mediations were generally reduced (Tables S4, S5). PM2.5 mediated both PLE total score and PLE total persistence. Income disparity only significantly mediated PLE total persistence. Families in poverty did not mediate either PLE total score or PLE total persistence. We note however that each racial and ethnic minority group was strongly associated with urbanicity and all three candidate mediators, suggesting that race and ethnicity variables acted as confounds.
Discussion
In this study, we aimed to better understand the nature of the association between urbanicity and PLE in a large, nation-wide pre-adolescent sample. Consistent with other work (18), we found that greater urbanicity was associated with greater number of PLE and associated distress measured one-year later. In contrast, we found no association between urbanicity and externalizing or internalizing symptoms, suggesting some specificity of the impact of urbanicity on mental health outcomes in pre-adolescence. Of those physical and social exposures we found to be related to urbanicity, only certain exposures—PM2.5, proximity to roads, houses at risk for lead paint, families in poverty, and income disparity—were related to the number of PLE assessed one-year later and persistence of PLE one year later. These variables were not related to the onset of PLE. These findings converge with other work showing PLE and SSD risk in children and adults is related to pollution (27, 28, 43), and living in countries or neighborhoods characterized by economic inequality and disadvantage (66–68). These findings also build upon prior cross-sectional analyses of urbanicity, urban-related exposures, and PLE in the ABCD dataset. Specifically, a prior study of baseline data revealed that a variety of environmental risk factors, including correlates of urbanicity, were associated with concurrent PLE, and that cortical volume partially mediated some of these relationships such as poverty, lead risk, and perception of neighborhood safety; as found in (54). Our study expands on that analysis by demonstrating that urbanicity has a significant longitudinal relationship with PLE.
Given the inter-relation between these variables, a key aim of this study was to evaluate the hypothesis that urbanicity is associated with PLE because of the impact of urban-related exposures. Partially consistent with this idea, we found that PM2.5, families in poverty, and income disparity mediated the association between urbanicity and number of PLE. Importantly, these variables explained a substantial proportion of the urbanicity-PLE association, ranging from 23.0% for PM2.5 to over 66% for families in poverty and income disparity. Further, these same exposures mediated the association between urbanicity and the one-year persistence of PLE, with exposures explaining an even greater proportion of the association, ranging from 44.1% for PM2.5 to over 79% for families in poverty and income disparity. In line with other work (10, 43), these findings suggest that it may not be urbanicity per se that impacts psychosis risk, but certain environmental attributes that covary with and perhaps result from urban environments.
Future work should investigate the processes through which pollution and economic inequality produces PLE, associated distress, and if the associations are causal in nature at all. Prior work has demonstrated that air pollution is associated with neuroinflammation, microglial activation, white matter deficits, and cognitive disturbances (77–80). These neurobiological changes are also associated with psychosis-risk states (81–83). On socio-economic disadvantage, poverty and inequality may promote unhealthy social comparisons related to social power and rank, which may promote views of oneself as a social subordinate or outsider; which are experiences connected to paranoia (84, 85). Socio-economic disadvantage may also erode social cohesion (i.e., interpersonal connectedness, shared values, mutual trust) and increase social disorganization (i.e., mobility, isolation, lack of social control, high crime); phenomena that also have been linked to increased PLE in childhood and adolescence (10, 45), and psychotic disorders in adulthood (35, 36, 66, 86, 87). Many of these experiences would fit within a social defeat model (42). Specifically, socio-economic disadvantage, and the consequences we speculate about above, promote the marginalization and subordination of disadvantaged individuals, leaving them socially isolated and more vulnerable to stress. Whatever the mechanisms, taken with other work, our findings suggest that urbanicity may set the physical and social context for experiences that are linked with increased PLE risk (26). It is notable and disconcerting that the association between these exposures and PLE are already present in late childhood.
The fact that the impact (presence of PLE and distress) of these exposures may occur as early as pre-adolescence highlights the importance of early prevention. Most psychosis-risk research has focused on older adolescents and young adults, which provides a short period for prevention given the modal age range of onset for psychotic spectrum disorders (88). Given that the association between well-established psychosis risk factors and PLE can be detected well before, it is important to consider the potential impact of intervening even earlier, and doing so by taking a public health-oriented approach that focuses on preventing the adverse exposures—like poverty and pollution—as opposed to the disorders themselves (89). Such interventions could include universal access to primary care, debt relief, or relocation to sustainable and green housing (90, 91). These actions could, in theory, reduce risk for psychosis and other health conditions impacted by the social and physical environment, as well as uplift underserved populations.
Several limitations are notable. First, we measured the association between urbanicity, urban-related exposures, and later PLE on a short timescale. It may be that some exposures require more time to impact PLE and mediate the urbanicity-PLE. Second, the short timescale may also explain the null results for PLE onset findings since few participants experienced an onset of PLE. It may be the case that some of these urban-related exposures are in fact related to PLE onset, but additional longitudinal data at a longer timescale would be needed to address this possibility. Third, many cases of childhood PLE do not persist (92). This leaves open the possibility that the urban exposures tested here may not predict PLE later in development. Fourth, it remains unclear the role these mediators may play in explaining the transition of PLE to psychotic illness vs. simply the persistence of PLE. Finally, we selected only a subset of all possible urban-related exposures available in the ABCD dataset that may explain the urbanicity-PLE association. Those tested here by no means provide an exhaustive account nor do they necessarily represent the most accurate way of assessing a given exposure (e.g., marijuana sales as a proxy for exposure to and use of marijuana). Similarly, some of the urban-related exposures are closely associated with other exposures not tested here that may also have an impact on PLE. For example, in addition to being a proxy for pollution, proximity to major roads may also be associated with noise pollution, which has been connected to schizophrenia in a work context (93, 94), cognitive impairment (95), and behavioral problems in children (96).
In summary, we find that many physical and social urban-related exposures are related to PLE numbers and distress measured one-year later. Further, a small number of these exposures—i.e., pollution, living in a high-poverty area, income disparity—explain, in part, the association between urbanicity and PLE in pre-adolescence, accounting for a substantial proportion of the total effect of urbanicity on PLE outcomes. Taken with other studies (10, 43), these findings help to explain why urbanicity has an impact on PLE, and intimate certain mechanisms that may be at work, which can explicitly be tested for in the future. The implications of such may be increasingly important as more of the global population moves into cities.
Data Availability Statement
Publicly available datasets were analyzed in this study. This data can be found here: https://dx.doi.org/10.15154/1519352; NIMH Data Archive (Adolescent Brain Cognitive Development Study).
Ethics Statement
The studies involving human participants were reviewed and approved by University of Rochester Research Subjects Review Board. Written informed consent to participate in this study was provided by the participants' legal guardian/next of kin.
Author Contributions
AS and DD-F contributed to all aspects of this work, with DD-F also providing supervision. All authors contributed to the article and approved the submitted version.
Funding
This work was supported indirectly by a grant from the National Institute of Mental Health (1L30MH117569-01 to DD-F).
Conflict of Interest
The authors declare that the research was conducted in the absence of any commercial or financial relationships that could be construed as a potential conflict of interest.
Publisher's Note
All claims expressed in this article are solely those of the authors and do not necessarily represent those of their affiliated organizations, or those of the publisher, the editors and the reviewers. Any product that may be evaluated in this article, or claim that may be made by its manufacturer, is not guaranteed or endorsed by the publisher.
Acknowledgments
Data used in the preparation of this article were obtained from the Adolescent Brain Cognitive DevelopmentSM (ABCD) Study (https://abcdstudy.org), held in the NIMH Data Archive (NDA). This is a multisite, longitudinal study designed to recruit more than 10,000 children age 9–10 and follow them over 10 years into early adulthood. A full list of supporters is available at: https://abcdstudy.org/federal-partners.html. A listing of participating sites and a complete listing of the study investigators can be found at: https://abcdstudy.org/consortium_members/. ABCD consortium investigators designed and implemented the study and/or provided data but did not necessarily participate in the analysis or writing of this report. This manuscript reflects the views of the authors and may not reflect the opinions or views of the NIH or ABCD consortium investigators. The ABCD data repository grows and changes over time. The ABCD data used in this report came from: https://dx.doi.org/10.15154/1519007. The NDA study for this project can be found at: https://dx.doi.org/10.15154/1519352. The authors would also like to thank Dr. Elizabeth D. Handley for advice on data analysis. The ABCD Study® is supported by the National Institutes of Health and additional federal partners under award numbers U01DA041048, U01DA050989, U01DA051016, U01DA041022, U01DA051018, U01DA051037, U01DA050987, U01DA041174, U01DA041106, U01DA041117, U01DA041028, U01DA041134, U01DA050988, U01DA051039, U01DA041156, U01DA041025, U01DA041120, U01DA051038, U01DA041148, U01DA041093, U01DA041089, U24DA041123, and U24DA041147.
Supplementary Material
The Supplementary Material for this article can be found online at: https://www.frontiersin.org/articles/10.3389/fpsyt.2022.831089/full#supplementary-material
References
1. United Nations Department of Economic and Social Affairs Population Division. World urbanization prospects: the 2018 revision. (2019).
2. Alarima CI. Factors influencing rural-urban migration of youths in Osun State, Nigeria. Agro-Sci. (2019) 17:34. doi: 10.4314/as.v17i3.6
4. Leon DA. Cities, urbanization and health. Int J Epidemiol. (2008) 37:4–8. doi: 10.1093/ije/dym271
5. Allardyce J, Boydell J, Van Os J, Morrison G, Castle D, Murray RM, et al. Comparison of the incidence of schizophrenia in rural Dumfries and Galloway and urban Camberwell. Br J Psychiat. (2001) 179:335–9. doi: 10.1192/bjp.179.4.335
6. Lewis G, David A, Andréassson S, Allebeck Schizophrenia P, city life. Lancet. (1992) 340:137–40. doi: 10.1016/0140-6736(92)93213-7
7. Marcelis M, Takei N, van Os Urbanization J, and risk for schizophrenia: does the effect operate before or around the time of illness onset? Psychol Med. (1999) 29:1197–203. doi: 10.1017/S0033291799008983
8. Marcelis M, Navarro-Mateu F, Murray R, Selten J-P, Van Os Urbanization J and and psychosis: a study of 1942–1978 birth cohorts in The Netherlands. Psychol Med. (1998) 28:871–9. doi: 10.1017/S0033291798006898
9. Mortensen PB, Pedersen CB, Westergaard T, Wohlfahrt J, Ewald H, Mors O, et al. Effects of family history and place and season of birth on the risk of schizophrenia. N Engl J Med. (1999) 340:603–8. doi: 10.1056/NEJM199902253400803
10. Newbury JB, Arseneault L, Caspi A, Moffitt TE, Odgers CL, Fisher HL. Why are children in urban neighborhoods at increased risk for psychotic symptoms? findings from a UK longitudinal cohort study. SCHBUL. (2016) 42:1372–83. doi: 10.1093/schbul/sbw052
11. Sundquist K, Frank G, Sundquist Urbanisation J. and incidence of psychosis and depression: Follow-up study of 4.4 million women and men in Sweden. Br J Psychiatry. (2004) 184:293–8. doi: 10.1192/bjp.184.4.293
12. van Os J, Hanssen M, Bijl RV, Vollebergh W. Prevalence of psychotic disorder and community level of psychotic symptoms: an urban-rural comparison. Arch Gen Psychiatry. (2001) 58:663. doi: 10.1001/archpsyc.58.7.663
13. Kirkbride JB, Fearon P, Morgan C, Dazzan P, Morgan K, Tarrant J, et al. Heterogeneity in incidence rates of schizophrenia and other psychotic syndromes: findings from the 3-center ÆSOP study. Arch Gen Psychiatry. (2006) 63:250. doi: 10.1001/archpsyc.63.3.250
14. Pedersen CB. Evidence of a dose-response relationship between urbanicity during upbringing and schizophrenia risk. Arch Gen Psychiatry. (2001) 58:1039–46. doi: 10.1001/archpsyc.58.11.1039
15. Castillejos MC, Martín-Pérez C, Moreno-Küstner B. A systematic review and meta-analysis of the incidence of psychotic disorders: the distribution of rates and the influence of gender, urbanicity, immigration and socio-economic level. Psychological Medicine. (2018) 1–15. doi: 10.1017/S0033291718000235
16. Kelly BD, O'Callaghan E, Waddington JL, Feeney L, Browne S, Scully PJ, et al. Schizophrenia and the city: A review of literature and prospective study of psychosis and urbanicity in Ireland. Schizophr Res. (2010) 116:75–89. doi: 10.1016/j.schres.2009.10.015
17. Krabbendam Schizophrenia L, urbanicity: a major environmental influence–conditional on genetic risk. Schizophr Bull. (2005) 31:795–9. doi: 10.1093/schbul/sbi060
18. Vassos E, Pedersen CB, Murray RM, Collier DA, Lewis CM. Meta-analysis of the association of urbanicity with schizophrenia. Schizophr Bull. (2012) 38:1118–23. doi: 10.1093/schbul/sbs096
19. Tandon R, Keshavan M, Nasrallah Schizophrenia H, “Just the Facts” What we know in 2008. 2 Epidemiology and etiology. Schizophr Res. (2008) 102:1–18. doi: 10.1016/j.schres.2008.01.022
20. Abrahamyan Empson L, Baumann PS, Söderström O, Codeluppi Z, Söderström D, Conus P. Urbanicity: the need for new avenues to explore the link between urban living and psychosis. Early Interv Psychiatry. (2019) 1–12. doi: 10.1111/eip.12861
21. March D, Hatch SL, Morgan C, Kirkbride JB, Bresnahan M, Fearon P, et al. Psychosis and Place. Epidemiol Rev. (2008) 30:84–100. doi: 10.1093/epirev/mxn006
22. Pedersen CB. Persons with schizophrenia migrate towards urban areas due to the development of their disorder or its prodromata. Schizophr Res. (2015) 168:204–8. doi: 10.1016/j.schres.2015.08.028
23. van Os J. Does the urban environment cause psychosis? Br J Psychiatry. (2004) 184:287–8. doi: 10.1192/bjp.184.4.287
24. van Os J, Kenis G, Rutten BPF. The environment and schizophrenia. Nature. (2010) 468:203–12. doi: 10.1038/nature09563
25. Brown AS. The environment and susceptibility to schizophrenia. Prog Neurobiol. (2011) 93:23–58. doi: 10.1016/j.pneurobio.2010.09.003
26. Krabbendam L, van Vugt M, Conus P, Söderström O, Abrahamyan Empson L, van Os J, et al. Understanding urbanicity: how interdisciplinary methods help to unravel the effects of the city on mental health. Psychol Med. (2020) 1–12. doi: 10.1017/S0033291720000355
27. Antonsen S, Mok PLH, Webb RT, Mortensen PB, McGrath JJ, Agerbo E, et al. Exposure to air pollution during childhood and risk of developing schizophrenia: A national cohort study. Lancet Planet Health. (2020) 4:e64–e73. doi: 10.1016/S2542-5196(20)30004-8
28. Gao Q, Xu Q, Guo X, Fan H, Zhu H. Particulate matter air pollution associated with hospital admissions for mental disorders: a time-series study in Beijing, China. Eur Psychiat. (2017) 44:68–75. doi: 10.1016/j.eurpsy.2017.02.492
29. Lary DJ, Lary T, Sattler B. Using machine learning to estimate global PM25 for environmental health studies environ?health?insights 9s1. EHIS. (2015) 15664. doi: 10.4137/EHI.S15664
30. Liang Z, Xu C, Cao Y, Kan H-D, Chen R-J, Yao C-Y, et al. The association between short-term ambient air pollution and daily outpatient visits for schizophrenia: a hospital-based study. Environ Pollut. (2019) 244:102–8. doi: 10.1016/j.envpol.2018.09.142
31. Tong L, Li K, Zhou Q. Season, sex and age as modifiers in the association of psychosis morbidity with air pollutants: a rising problem in a Chinese metropolis. Sci Total Environ. (2016) 541:928–33. doi: 10.1016/j.scitotenv.2015.09.066
32. Arinola G, Idonije B, Akinlade K, Ihenyen O. Essential trace metals and heavy metals in newly diagnosed schizophrenic patients and those on anti-psychotic medication. J Res Med Sci. (2010) 15:245−9.
33. Opler MGA, Buka SL, Groeger J, McKeague I, Wei C, Factor-Litvak P, et al. Prenatal exposure to lead, δ-aminolevulinic acid, and schizophrenia: further evidence. Environ Health Perspect. (2008) 116:1586–90. doi: 10.1289/ehp.10464
34. Opler MGA, Brown AS, Graziano J, Desai M, Zheng W, Schaefer C, et al. Prenatal lead exposure, delta-aminolevulinic acid, and schizophrenia. Environ Health Perspect. (2004) 112:548–52. doi: 10.1289/ehp.6777
35. Morgan C, Kirkbride J, Hutchinson G, Craig T, Morgan K, Dazzan P, et al. Cumulative social disadvantage, ethnicity and first-episode psychosis: a case-control study. Psychol Med. (2008) 38:1701. doi: 10.1017/S0033291708004534
36. Stilo SA, Gayer-Anderson C, Beards S, Hubbard K, Onyejiaka A, Keraite A, et al. Further evidence of a cumulative effect of social disadvantage on risk of psychosis. Psychol Med. (2017) 47:913–24. doi: 10.1017/S0033291716002993
37. Stilo SA, Di Forti M, Mondelli V, Falcone AM, Russo M, O'Connor J, et al. Social disadvantage: cause or consequence of impending psychosis? Schizophr Bull. (2013) 39:1288–95. doi: 10.1093/schbul/sbs112
38. Morgan C, Fisher H. Environment and schizophrenia: environmental factors in schizophrenia: childhood trauma–a critical review. Schizophr Bull. (2006) 33:3–10. doi: 10.1093/schbul/sbl053
39. Read J, Os J, Morrison AP, Ross CA. Childhood trauma, psychosis and schizophrenia: a literature review with theoretical and clinical implications. Acta Psychiatr Scand. (2005) 112:330–50. doi: 10.1111/j.1600-0447.2005.00634.x
40. Gevonden MJ, Selten JP, Myin-Germeys I, de Graaf R, ten Have M, van Dorsselaer S, et al. Sexual minority status and psychotic symptoms: findings from the Netherlands Mental Health Survey and Incidence Studies (NEMESIS). Psychol Med. (2014) 44, 421–433. doi: 10.1017/S0033291713000718
41. Kirkbride JB, Errazuriz A, Croudace TJ, Morgan C, Jackson D, Boydell J, et al. Incidence of Schizophrenia and Other Psychoses in England, 1950–2009: A Systematic Review and Meta-Analyses. PLoS ONE. (2012) 7:e31660. doi: 10.1371/journal.pone.0031660
42. Selten J-P, van der Ven E, Rutten BPF, Cantor-Graae E. The social defeat hypothesis of schizophrenia: an update. Schizophr Bull. (2013) 39:1180–6. doi: 10.1093/schbul/sbt134
43. Newbury JB, Arseneault L, Beevers S, Kitwiroon N, Roberts S, Pariante CM, et al. Association of air pollution exposure with psychotic experiences during adolescence. JAMA Psychiat. (2019) 76:614. doi: 10.1001/jamapsychiatry.2019.0056
44. Pedersen CB, Raaschou-Nielsen O, Hertel O, Mortensen PB. (2004). Air pollution from traffic and schizophrenia risk. Schizophrenia Res. 66:83–85. doi: 10.1016/S0920-9964(03)00062-8
45. Newbury J, Arseneault L, Caspi A, Moffitt TE, Odgers CL, Fisher HL. Cumulative effects of neighborhood social adversity and personal crime victimization on adolescent psychotic experiences. Schizophr Bull. (2018) 44:348–58. doi: 10.1093/schbul/sbx060
46. Croft J, Heron J, Teufel C, Cannon M, Wolke D, Thompson A, et al. Association of trauma type, age of exposure, and frequency in childhood and adolescence with psychotic experiences in early adulthood. JAMA Psychiat. (2019) 76:79. doi: 10.1001/jamapsychiatry.2018.3155
47. Polanczyk G, Moffitt TE, Arseneault L, Cannon M, Ambler A, Keefe RSE, et al. Etiological and clinical features of childhood psychotic symptoms: results from a birth cohort. Arch Gen Psychiatry. (2010) 67:328. doi: 10.1001/archgenpsychiatry.2010.14
48. Spauwen J, Krabbendam L, Lieb R, Wittchen H-U, Van Os J. Evidence that the outcome of developmental expression of psychosis is worse for adolescents growing up in an urban environment. Psychol Med. (2006) 36:407–15. doi: 10.1017/S0033291705006902
49. Garavan H, Bartsch H, Conway K, Decastro A, Goldstein RZ, Heeringa S, et al. Recruiting the ABCD sample: design considerations and procedures. Dev Cogn Neurosci. (2018) 32:16–22. doi: 10.1016/j.dcn.2018.04.004
50. Karcher NR, Barch DM, Avenevoli S, Savill M, Huber RS, Simon TJ, et al. Assessment of the prodromal questionnaire–brief child version for measurement of self-reported psychoticlike experiences in childhood. JAMA Psychiatry. (2018) 75:853. doi: 10.1001/jamapsychiatry.2018.1334
51. Karcher NR, Loewy RL, Savill M, Avenevoli S, Huber RS, Simon TJ, et al. Replication of Associations With Psychotic-Like Experiences in Middle Childhood From the Adolescent Brain Cognitive Development (ABCD) Study. Schizophrenia Bulletin Open. (2020) 1. doi: 10.1093/schizbullopen/sgaa009
52. Barch DM, Albaugh MD, Avenevoli S, Chang L, Clark DB, Glantz MD, et al. Demographic, physical and mental health assessments in the adolescent brain and cognitive development study: Rationale and description. Dev Cogn Neurosci. (2018) 32:55–66. doi: 10.1016/j.dcn.2017.10.010
53. Zucker RA, Gonzalez R, Feldstein Ewing SW, Paulus MP, Arroyo J, Fuligni A, et al. Assessment of culture and environment in the Adolescent Brain and Cognitive Development Study: Rationale, description of measures, early data. Dev Cogn Neurosci. (2018) 32:107–20. doi: 10.1016/j.dcn.2018.03.004
54. Karcher NR, Schiffman J, Barch DM. Environmental risk factors and psychotic-like experiences in children aged 9–10. J Am Acad Child Adolesc Psychiatry. (2021) 60:490–500. doi: 10.1016/j.jaac.2020.07.003
55. Singh GK. Area Deprivation and Widening Inequalities in US Mortality, 1969–1998. Am J Public Health. (2003) 93:1137–43. doi: 10.2105/AJPH.93.7.1137
56. Loewy RL, Pearson R, Vinogradov S, Bearden CE, Cannon TD. Psychosis risk screening with the prodromal questionnaire — brief version (PQ-B). Schizophr Res. (2011) 129:42–6. doi: 10.1016/j.schres.2011.03.029
57. Karcher NR, Niendam TA, Barch DM. Adverse childhood experiences and psychotic-like experiences are associated above and beyond shared correlates: Findings from the adolescent brain cognitive development study. Schizophrenia Res. (2020) doi: 10.1016/j.schres.2020.05.045
58. Reeve S, Bell V. Sleep disorders predict the one-year onset, persistence, but not remission of psychotic experiences in pre-adolescence: a longitudinal analysis of the ABCD cohort data (preprint). Psychiat Clini Psychol. (2021). doi: 10.1101/2021.01.15.21249864
59. Achenbach TM. Achenbach system of empirically based assessment (ASEBA): development, findings, theory, and applications. Burlington, Vt: University of Vermont, Research Center of Children, Youth & Families. (2009).
60. Duan J, Cheng Q, Luo X, Bai L, Zhang H, Wang S, et al. Is the serious ambient air pollution associated with increased admissions for schizophrenia? Sci Total Environ. (2018) 644:14–9. doi: 10.1016/j.scitotenv.2018.06.218
61. Eguchi R, Onozuka D, Ikeda K, Kuroda K, Ieiri I, Hagihara A. The relationship between fine particulate matter (PM2.5) and schizophrenia severity. Int Arch Occup Environ Health. (2018) 91:613–22. doi: 10.1007/s00420-018-1311-x
62. Qiu H, Zhu X, Wang L, Pan J, Pu X, Zeng X, et al. Attributable risk of hospital admissions for overall and specific mental disorders due to particulate matter pollution: A time-series study in Chengdu, China. Environ Research. (2019) 170:230–237. doi: 10.1016/j.envres.2018.12.019
63. Arseneault L, Cannon M, Witton J, Murray RM. Causal association between cannabis and psychosis: examination of the evidence. Br J Psychiatry. (2004) 184:110–7. doi: 10.1192/bjp.184.2.110
64. Gage SH, Hickman M, Zammit S. Association between cannabis and psychosis: epidemiologic evidence. Biol Psychiatry. (2016) 79:549–56. doi: 10.1016/j.biopsych.2015.08.001
65. Murray RM, Quigley H, Quattrone D, Englund A, Di Forti M. Traditional marijuana, high-potency cannabis and synthetic cannabinoids: increasing risk for psychosis. World Psychiatry. (2016) 15:195–204. doi: 10.1002/wps.20341
66. Burns JK, Tomita A, Kapadia AS. Income inequality and schizophrenia: Increased schizophrenia incidence in countries with high levels of income inequality. Int J Soc Psychiatry. (2014) 60:185–96. doi: 10.1177/0020764013481426
67. Johnson SL, Wibbels E, Wilkinson R. Economic inequality is related to cross-national prevalence of psychotic symptoms. Soc Psychiatry Psychiatr Epidemiol. (2015) 50:1799–807. doi: 10.1007/s00127-015-1112-4
68. Kirkbride JB, Jones PB, Ullrich S, Coid JW. Social deprivation, inequality, and the neighborhood-level incidence of psychotic syndromes in East London. Schizophr Bull. (2014) 40:169–80. doi: 10.1093/schbul/sbs151
69. Longden E, Sampson M, Read J. Childhood adversity and psychosis: generalised or specific effects? Epidemiol Psychiatr Sci. (2016) 25:349–59. doi: 10.1017/S204579601500044X
70. R Core Team (2018). R: A language and environment for statistical computing. Vienna, Austria: R Foundation for Statistical Computing.
71. Bates D, Mächler M, Bolker B, Walker S. Fitting Linear Mixed-Effects Models Using lme4. J Stat Softw. (2015) 67. doi: 10.18637/jss.v067.i01
72. Rosseel Y. lavaan: an R package for structural equation modeling. J Stat Softw. (2012) 48:1–36. doi: 10.18637/jss.v048.i02
73. MacKinnon DP, Lockwood CM, Williams J. Confidence limits for the indirect effect: distribution of the product and resampling methods. Multivariate Behav Res. (2004) 39:99–128. doi: 10.1207/s15327906mbr3901_4
74. Preacher KJ, Selig JP. Advantages of Monte Carlo confidence intervals for indirect effects. Commun Methods Meas. (2012) 6:77–98. doi: 10.1080/19312458.2012.679848
75. Jorgensen TD, Pornprasertmanit S, Schoemann AM, Rosseel Y. semTools: Useful tools for structural equation modeling. R package version 0.5-1. (2018). Retrieved from: https://CRAN.R-project.org/package=semTools
76. Alwin DF, Hauser RM. The decomposition of effects in path analysis. Am Sociol Rev. (1975) 40:37. doi: 10.2307/2094445
77. Attademo L, Bernardini F, Garinella R, Compton MT. Environmental pollution and risk of psychotic disorders: A review of the science to date. Schizophr Res. (2017) 181:55–9. doi: 10.1016/j.schres.2016.10.003
78. Block ML, Calderón-Garcidueñas L. Air pollution: mechanisms of neuroinflammation and CNS disease. Trends Neurosci. (2009) 32:506–16. doi: 10.1016/j.tins.2009.05.009
79. Calderón-Garcidueñas L, Solt AC, Henríquez-Roldán C, Torres-Jardón R, Nuse B, Herritt L, et al. Long-term air pollution exposure is associated with neuroinflammation, an altered innate immune response, disruption of the blood-brain barrier, ultrafine particulate deposition, and accumulation of amyloid β-42 and α-synuclein in children and young adults. Toxicol Pathol. (2008) 36:289–310. doi: 10.1177/0192623307313011
80. Genc S, Zadeoglulari Z, Fuss SH, Genc K. The adverse effects of air pollution on the nervous system. Journal of Toxicology. (2012) 2012:1–23. doi: 10.1155/2012/782462
81. Bora E, Murray RM. Meta-analysis of cognitive deficits in ultra-high risk to psychosis and first-episode psychosis: do the cognitive deficits progress over, or after, the onset of psychosis? Schizophr Bull. (2014) 40:744–55. doi: 10.1093/schbul/sbt085
82. Cannon TD, Chung Y, He G, Sun D, Jacobson A, van Erp TGM, et al. Progressive reduction in cortical thickness as psychosis develops: a multisite longitudinal neuroimaging study of youth at elevated clinical risk. Biol Psychiatry. (2015) 77:147–57. doi: 10.1016/j.biopsych.2014.05.023
83. Karlsgodt KH, Niendam TA, Bearden CE, Cannon TD. White matter integrity and prediction of social and role functioning in subjects at ultra-high risk for psychosis. Biol Psychiatry. (2009) 66:562–9. doi: 10.1016/j.biopsych.2009.03.013
84. Freeman D, Evans N, Lister R, Antley A, Dunn G, Slater M. Height, social comparison, and paranoia: An immersive virtual reality experimental study. Psychiatry Res. (2014) 218:348–52. doi: 10.1016/j.psychres.2013.12.014
85. Freeman D, Garety PA, Bebbington PE, Smith B, Rollinson R, Fowler D, et al. Psychological investigation of the structure of paranoia in a non-clinical population. Br J Psychiatry. (2005) 186:427–35. doi: 10.1192/bjp.186.5.427
86. Allardyce J, Gilmour H, Atkinson J, Rapson T, Bishop J, McCreadie RG. Social fragmentation, deprivation and urbanicity: relation to first-admission rates for psychoses. Br J Psychiatry. (2005) 187:401–6. doi: 10.1192/bjp.187.5.401
87. Veling W, Susser E, Selten J-P, Hoek HW. Social disorganization of neighborhoods and incidence of psychotic disorders: a 7-year first-contact incidence study. Psychol Med. (2015) 45:1789–98. doi: 10.1017/S0033291714002682
88. Schimmelmann BG, Walger P, Schultze-Lutter F. The significance of at-risk symptoms for psychosis in children and adolescents. Can J Psychiatry. (2013) 58:32–40. doi: 10.1177/070674371305800107
89. Kirkbride JB, Jones PB. The prevention of schizophrenia–what can we learn from eco-epidemiology? Schizophr Bull. (2011) 37:262–71. doi: 10.1093/schbul/sbq120
90. Anglin DM, Galea S, Bachman P. Going Upstream to Advance Psychosis Prevention and Improve Public Health. JAMA Psychiatry. (2020) 77:665. doi: 10.1001/jamapsychiatry.2020.0142
91. Wahlbeck K, Cresswell-Smith J, Haaramo P, Parkkonen J. Interventions to mitigate the effects of poverty and inequality on mental health. Soc Psychiatry Psychiatr Epidemiol. (2017) 52:505–14. doi: 10.1007/s00127-017-1370-4
92. Healy C, Brannigan R, Dooley N, Coughlan H, Clarke M, Kelleher I, et al. Childhood and adolescent psychotic experiences and risk of mental disorder: a systematic review and meta-analysis. Psychol Med. (2019) 49:1589–99. doi: 10.1017/S0033291719000485
93. Muntaner C, Tien AY, Eaton WW, Garrison R. Occupational characteristics and the occurrence of psychotic disorders. Soc Psychiatry Psychiatr Epidemiol. (1991) 26:273–80. doi: 10.1007/BF00789219
94. Link BG, Dohrenwend BP, Skodol AE. Socio-economic status and schizophrenia: Noisome occupational characteristics as a risk factor. Am Sociol Rev. (1986) 51:242–58. doi: 10.2307/2095519
95. Thompson R, Smith RB, Bou Karim Y, Shen C, Drummond K, Teng C, et al. Noise pollution and human cognition: An updated systematic review and meta-analysis of recent evidence. Environ Int. (2022) 158:106905. doi: 10.1016/j.envint.2021.106905
Keywords: psychotic-like experiences, psychosis, urbanicity, poverty, deprivation, pollution, pre-adolescence
Citation: Saxena A and Dodell-Feder D (2022) Explaining the Association Between Urbanicity and Psychotic-Like Experiences in Pre-Adolescence: The Indirect Effect of Urban Exposures. Front. Psychiatry 13:831089. doi: 10.3389/fpsyt.2022.831089
Received: 07 December 2021; Accepted: 27 January 2022;
Published: 11 March 2022.
Edited by:
Apolline Saucy, Instituto Salud Global Barcelona (ISGlobal), SpainReviewed by:
Gerard Hutchinson, University of the West Indies, Trinidad and TobagoYi Nam Suen, The University of Hong Kong, Hong Kong SAR, China
Copyright © 2022 Saxena and Dodell-Feder. This is an open-access article distributed under the terms of the Creative Commons Attribution License (CC BY). The use, distribution or reproduction in other forums is permitted, provided the original author(s) and the copyright owner(s) are credited and that the original publication in this journal is cited, in accordance with accepted academic practice. No use, distribution or reproduction is permitted which does not comply with these terms.
*Correspondence: Abhishek Saxena, YWJoaXNoZWsuc2F4ZW5hQHJvY2hlc3Rlci5lZHU=