- 1Department of Psychology, School of Public Health, Southern Medical University, Guangzhou, China
- 2Department of Neurosurgery, Huashan Hospital, Fudan University, Shanghai, China
- 3Department of Rehabilitation Medicine, School of Rehabilitation Medicine, Southern Medical University, Guangzhou, China
- 4Department of Psychiatry, Zhujiang Hospital, Southern Medical University, Guangzhou, China
Background: With the development of quantitative electroencephalography (QEEG), an increasing number of studies have been published on the clinical use of QEEG in the past two decades, particularly in the diagnosis, treatment, and prognosis of neuropsychiatric disorders. However, to date, the current status and developing trends of this research field have not been systematically analyzed from a macroscopic perspective. The present study aimed to identify the hot spots, knowledge base, and frontiers of QEEG research in neuropsychiatric disorders from 2000 to 2021 through bibliometric analysis.
Methods: QEEG-related publications in the neuropsychiatric field from 2000 to 2021 were retrieved from the Web of Science Core Collection (WOSCC). CiteSpace and VOSviewer software programs, and the online literature analysis platform (bibliometric.com) were employed to perform bibliographic and visualized analysis.
Results: A total of 1,904 publications between 2000 and 2021 were retrieved. The number of QEEG-related publications in neuropsychiatric disorders increased steadily from 2000 to 2021, and research in psychiatric disorders requires more attention in comparison to research in neurological disorders. During the last two decades, QEEG has been mainly applied in neurodegenerative diseases, cerebrovascular diseases, and mental disorders to reveal the pathological mechanisms, assist clinical diagnosis, and promote the selection of effective treatments. The recent hot topics focused on QEEG utilization in neurodegenerative disorders like Alzheimer's and Parkinson's disease, traumatic brain injury and related cerebrovascular diseases, epilepsy and seizure, attention-deficit hyperactivity disorder, and other mental disorders like major depressive disorder and schizophrenia. In addition, studies to cross-validate QEEG biomarkers, develop new biomarkers (e.g., functional connectivity and complexity), and extract compound biomarkers by machine learning were the emerging trends.
Conclusion: The present study integrated bibliometric information on the current status, the knowledge base, and future directions of QEEG studies in neuropsychiatric disorders from a macroscopic perspective. It may provide valuable insights for researchers focusing on the utilization of QEEG in this field.
Introduction
Electroencephalography (EEG) is a tool for recording spontaneous electrical activity generated in the cerebral cortex using multiple electrodes placed on the scalp (1), providing real-time assessment of cerebral physiological functions (2). Since the German psychiatrist Hans Berger first tried to record human cerebral electrical activity from the scalp in 1928 (3), EEG technology has continued to develop, which is currently one of the most influential noninvasive tools available to clinicians for evaluating a patient's neurophysiological functions (4).
Quantitative EEG (QEEG) techniques separate complex EEG signals into components such as amplitude, frequency, and compress time, permitting the display of several hours of data on one image (5). QEEG empowers a neurologist or a psychiatrist's unprecedented ability to look at summarized EEG information, which was not previously possible with a visual examination of EEG traces. More importantly, QEEG can provide an objective, replicable measure of brain functions that is less dependent on subjective or behavioral reports that may vary across settings and informants (6). Therefore, QEEG can be used in more productive ways than non-QEEG to identify and categorize neuropsychiatric diseases, as well as to predict the outcome of therapeutic intervention (7).
To date, several studies have summarized the development of QEEG methodology (8–10) or systematically reviewed the research papers on the possible use of QEEG as a biomarker in adult or child psychiatric disorders (11, 12) and in Alzheimer's disease (13). However, to our knowledge, there is no existing study to analyze the developing status of the QEEG research field from a macroscopic perspective.
Bibliometrics, an important branch of intelligence science (14), uses the literature system and bibliometric characteristics as the research object and conducts quantitative and qualitative analyses of the literature (15). In recent years, bibliometric analysis has been applied to visualize the knowledge status, features, evolution, and emerging trends in various research fields (16). It can help scholars extract quantitative information on distribution by country/region, institution, author, journal, research hot spots, and frontiers in a particular field in a short time, providing in-depth reviews and insights about the research field (17). Therefore, the present study used bibliometric tools to analyze the QEEG studies in neuropsychiatric disorders from 2000 to 2021 to provide a comprehensive overview.
The specific research questions in the present study for QEEG research in neuropsychiatric disorders were as follows: 1) What are the overall publication trends, the geographic distributions, the most important journals, and who are the potential collaborators? 2) What is the knowledge framework in this field in terms of research hot spots and knowledge base? 3) What are the future directions of this field?
Method and Data Source
Data Collection
Data for the present study were retrieved from the Web of Science Core Collection (WOSCC) database in January 2022. We used TS = [(quantitative electroencephalography) OR (QEEG) OR (quantitative EEG)] AND WC = [(neurology) OR (psychiatry) OR (neuropsychiatry)] as the search terms, where “TS” represents term subject and “WC” represents “Web of Science categories.” The time limitation was between 1 January 2000 and 31 December 2021. Only literature published in English was included, and duplicated articles were deleted. To avoid bias due to daily database updates, we performed the literature retrieval from WOSCC on a single day, that is, 27 January 2022. A total of 1,904 publications were included and consisted of original articles and reviews. The search strategy is depicted in Figure 1.
Analysis Tools
All the collected data were converted into TXT format and exported for further visual analysis by bibliometric software, including CiteSpaceV (5.8.R3) and VOSviewer (1.6.16), and the online literature analysis platform (https://bibliometric.com/). Visualized information, such as yearly output, subject categories of WOS, and impact factor (IF), was analyzed based on the function of literature analysis on WOSCC. The number of countries, institutions, and international collaborations were analyzed by bibliometric.com. CiteSpaceV was used to perform collaboration network analysis (including authors, institutions, journals, co-cited journals, co-cited authors, and co-cited references) and related centrality. The specific parameters used in CiteSpaceV were set as follows. For the selection of time slices, a slice of 1 year was used for determining the connection strength, and cosine was used for this purpose. For the threshold, we selected the top 50 nodes in each time slice. Moreover, the pruning used a pathfinder and merged network. VOSviewer was used to analyze the keywords. The keyword co-occurrence map in VOSviewer only includes terms that appear at least 15 times under the binary count. The purpose of the algorithm is to ensure that the terms that occur more frequently have larger bubble images and the terms with high similarity are close to each other with a similar color. Finally, the keyword overlay map was used based on the occurrence of keywords to visualize the emerging topics from 2000 to 2021.
Results
Annual Publications
A total of 1904 publications that met the retrieval criteria were included in further analysis. The total number of annual publications is shown in Figure 2A, which depicts an increasing trend from 2000 to 2021. As shown in Figure 2B, the trend of the annual publications in the neurology field showed steady growth. However, the yearly output of articles in the psychiatry area increased relatively slowly, indicating that the application of QEEG in psychiatric disorders requires more attention.
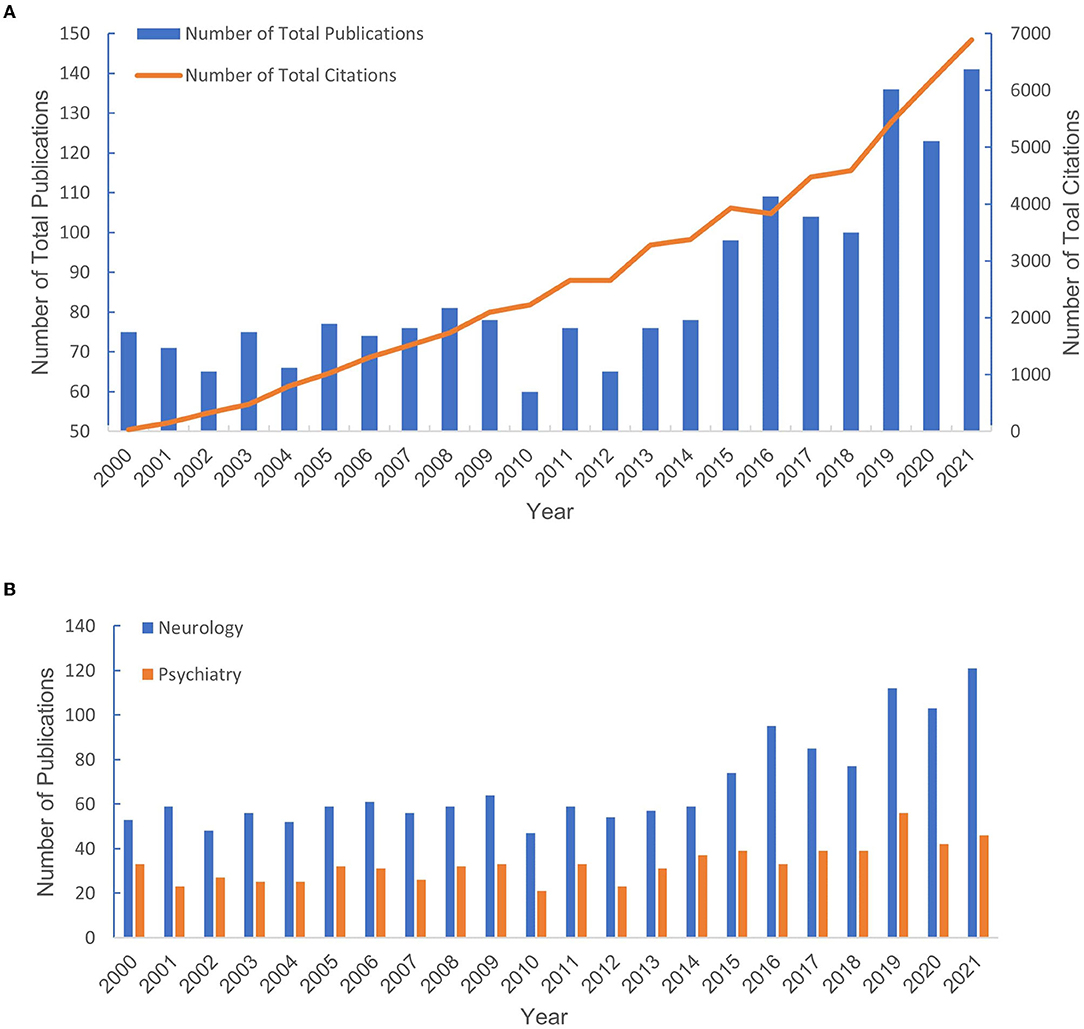
Figure 2. The number of publications from 2000 to 2021. (A) The number of publications in neurology and psychiatry. (B) The total number of publications and the percentage of total publications.
Analysis of Countries and Institutions
Publications of QEEG research were obtained from 71 countries. About 89.92% of the publications were from the top 10 countries (Table 1). The trends of annual output from the top 10 countries are shown in Figure 3A. Most publications were from the United States (n = 657, 34.5%). The centrality analysis in CiteSpaceV represented the influence of a node, and a node was of great significance when the centrality value is greater than 0.1. Countries with a centrality value greater than 0.1 were the United States (n = 0.95) and Germany (n = 0.14), suggesting that publications from these two countries had a greater influence on QEEG research. The international cooperation between countries is shown in Figure 3C. The most frequent collaboration was observed between the United States and Canada, followed by Australia.
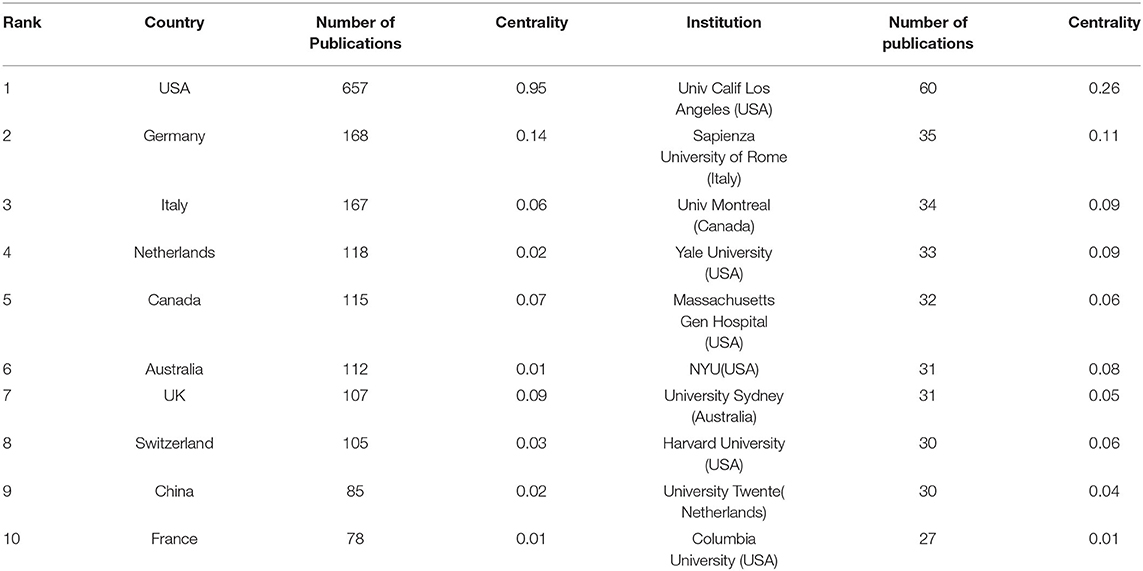
Table 1. Top 10 countries and institutions with the highest number of publications from 2000 to 2021.
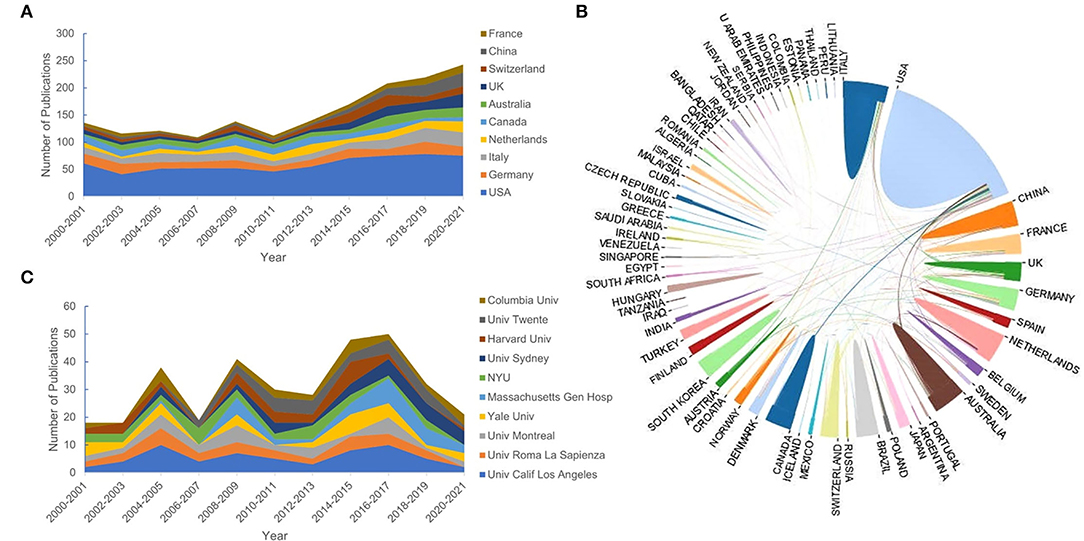
Figure 3. The top 10 countries and institutions. (A) The number of publications of the top 10 countries. (B) The cooperative relationship between countries. (C) The number of publications of the top 10 institutions.
A total of 2,433 institutions published QEEG-related articles. The trends of annual output from the top 10 institutions are shown in Figure 3B. The distribution of institutions is scattered. Table 1 lists the top 10 productive institutions, and 343 papers have been published by these institutions, accounting for 18.01% of the total publications. About 60% of the top 10 institutions are from the United States, including the University of California, Los Angeles, Yale University, Massachusetts General Hospital, New York University, Harvard University, and Columbia University.
Analysis of Journals and Co-cited Journals
A total of 245 journals were involved, and the top 10 most active QEEG-related journals published 47.53% of the total publications (n = 905; Figure 4A). Among the top 10 journals in QEEG research, Clinical Neurophysiology (n = 281) was the most active journal, followed by Clinical EEG and Neuroscience (n = 144) and Journal of Clinical Neurophysiology (n = 84).
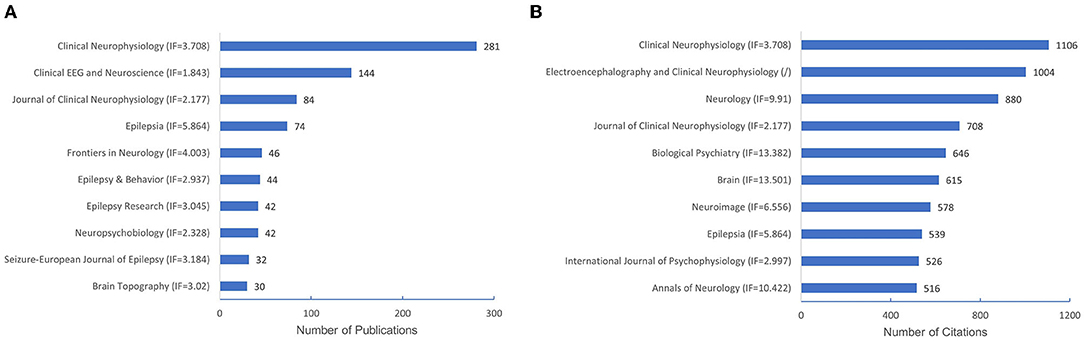
Figure 4. The number of publications of the top 10 journals and co-cited journals. (A) The number of publications of the top 10 journals. (B) The number of citations of the top 10 co-cited journals.
Co-cited journals were journals cited together by researchers, which usually reflected the foundation of a research field and were one of the most important indicators in bibliometric analysis. Half of the top 10 co-cited journals in QEEG research in neuropsychiatric disorders were published in Q1 according to JCR. Biological Psychiatry showed the highest impact factor (IF = 13.382). Clinical Neurophysiology (n = 1,106) ranked first in co-cited journals, followed by Electroencephalography and Clinical Neurophysiology (n = 1,004) and Neurology (n = 880), suggesting these journals were well recognized in QEEG research in the neuropsychiatric field (Figure 4B).
Analysis of Authors
A total of 8,003 authors contributed to QEEG research in neuropsychiatric disorders. As presented in Table 2, six authors published over 25 articles. The most productive authors were Cook IA and Leuchter AF who published 36 articles in this field. Their research direction is mainly focused on the abnormality of QEEG in major depression and its rehabilitation by pharmacological treatments.
Research Hot Topics Based on Keyword Analysis
To reveal the hot topics in the research field, we used VOSviewer to produce a keyword co-occurrence map (see Figure 6). The keyword co-occurrence map retrieved five major keyword clusters of QEEG research in neuropsychiatric disorders. As shown in Figure 5, each keyword cluster is depicted in a distinct color. That is, the blue cluster represents research in attention-deficit hyperactivity disorder (ADHD), the yellow cluster represents research in neurodegenerative disorders, such as Alzheimer's disease, the green cluster represents research in epilepsy, the purple cluster represents research in traumatic brain injury and related cerebrovascular diseases, and the red cluster represents research in psychiatric disorders, such as major depressive disorder and schizophrenia. These five research areas are currently hot topics in the research field.
Knowledge Base by Cluster Analysis of Co-cited References
Co-cited references are co-cited articles in the reference lists of other articles. The co-citation network by CiteSpaceV revealed 1,067 nodes, 3,989 co-citation links, and 22 clusters. These clusters represented the knowledge base and networks of QEEG studies in neuropsychiatric disorders. Figure 6 shows all automatically extracted clusters. Each cluster is depicted with a unique color. The nodes in each cluster represent the co-cited documents, and the lines between the nodes represent the co-cited relationship. The labels of the clusters were extracted from the keywords of the citing publications, based on the latent semantic indexing (LSI) method. The general information about the co-citation clusters is summarized in Table 3, including the number of cited references, the average publication year of the cited references, and the silhouette value of each cluster. The silhouette value of a cluster ranges from 0 to 1, and a larger value indicated greater discrimination from other clusters (18). In addition, Table 4 presented the research disease categories and domains retrieved from the co-cited reference clusters. Supplementary Table S1 summarized highly co-cited references and the most relevant citing articles in each co-citation cluster.
Research Emerging Trends Based on Keyword Analysis
We also used VOSviewer to produce the overlay map to show the latest emerging topics (Figure 7). In VOSviewer, we set the threshold of occurrence frequency to 15, and 253 of the total 7,458 keywords met the criteria. Among these keywords, “biomarker,” “connectivity,” and “machine learning” have emerged since 2018 and represented the future directions in QEEG studies in neuropsychiatric disorders. These emerging topics are depicted in yellow color in Figure 7.
Discussion
General Information
The current study applied a visualized bibliometric method to analyze the research hot spots, the knowledge base, and the emerging topics of the publications about QEEG in the neuropsychiatric research field. A total of 1,904 papers were collected based on 2000–2021 data from WoSCC. All papers were published by 2,433 institutions from 71 countries in 245 peer-reviewed journals with 57,237 co-cited references. The annual publication output and annual citation number revealed a steady growth in the research field. About 34.5% of the total publications were from the United States. Close cooperation between the United States, Canada, and Australia was found, suggesting their significant contribution to QEEG research in neuropsychiatric disorders. Among the 10 top institutions, 60% were from the United States, such as the University of California, Los Angeles, Yale University, Massachusetts General Hospital, New York University, Harvard University, and Columbia University. Among the top 10 authors, Cook IA and Leuchter AF published more studies and received higher co-citations, suggesting that their teams could be potential collaborators for researchers. Additionally, we found journals with high impact factors in our top 10 co-cited journals, such as Biological Psychiatry (IF = 13.382), Brain (IF = 13.501), and Annual of Neurology (IF = 10.422), which could be important sources of references.
Hot Topics of QEEG Research in the Neuropsychiatric Disorders
According to the keyword co-occurrence map by VOSviewer, five clusters were retrieved and represented five major categories of neuropsychiatric diseases in QEEG research. The blue cluster represented the psychiatric disorder “ADHD.” ADHD is characterized by excessive restlessness and an extremely poor concentration span, resulting in impulsive and disruptive behavior. Bresnahan and Barry (19) suggested that QEEG might be used to differentiate ADHD adults from normal adults and adults who display the symptoms of ADHD without meeting the diagnostic criteria of ADHD. Elevated resting theta power and reduced alpha and beta power, together with elevated theta/alpha and theta/beta ratios, were found to be most reliably associated with ADHD (20, 21). Recently, novel measurements have emerged. For example, gamma power abnormalities might provide an opportunity to investigate the neurobiological mechanisms that underlie the clinical symptoms of ADHD (22–24).
The yellow cluster represented the neurodegeneration disorders, particularly Alzheimer's disease (AD) and Parkinson's disease (PD). Excessive slow wave activity has been shown in dementia of the Alzheimer's type that increases with disease progression (25). Compared to AD, the inter-hemispheric coherence values for the delta and theta bands in the fronto-temporo-central regions were higher in dementia with Lewy bodies (DLB). For patients with AD, the beta band was lower than DLB in almost all temporo-centro-parieto-occipital regions (26). Additionally, in patients with PD, abnormalities in QEEG, such as an increase in posterior theta power, were found with the occurrence of mild cognitive impairment or dementia (27). QEEG could also provide reliable biomarkers for objective monitoring of disease severity and progression in PD, as well as for promoting early diagnosis of nonmotor symptoms (28). For example, decreased dominant frequency and increased theta power, which reflect EEG slowing, were biomarkers of cognitive deterioration.
The green cluster represented “epilepsy” and “seizure,” which included the temporal lobe epilepsy, electroconvulsive-induced epilepsy, and so on. Larsson and colleagues showed that peak alpha frequency (PAF) variability was compromised in patients with epilepsy (29). Park et al. suggested that the automatic quantitative ictal high-gamma oscillation analysis may be effective in delineating the epileptogenic zone (30). QEEG background activity may also provide useful information on seizure duration. A higher theta power ratio in the temporal region contralateral to the epileptic focus may suggest a longer epilepsy duration (31).
The purple cluster represented traumatic brain injury (TBI) and related cerebrovascular diseases, such as stroke and subarachnoid hemorrhage (SAH). QEEG might predict the prognosis after TBI. In particular, measures of alpha power and variability were indicative of relatively better functional outcomes within the first year after TBI. This was hypothesized to reflect intact thalamo-cortical loops and thus the potential for recovery of consciousness even in the apparent absence of current consciousness (32). QEEG can also be used to identify patients at risk of cerebral infarction. In patients with SAH, there was a moderate correlation between transcranial Doppler/color-coded duplex sonography (TCD/TCCS) frequencies and QEEG alpha power reduction, but only QEEG could differentiate patients with and without cerebral infarction (33). Moreover, worsening alpha/delta ratio (ADR) on QEEG was a reliable predictor of delayed cerebral ischemia (DCI) in patients with aneurysmal SAH. Further studies are still needed to confirm the role of QEEG in the prediction of DCI (34).
The red cluster consisted of QEEG studies in several common psychiatric disorders, including major depressive disorder (MDD), anxiety, and schizophrenia (35, 36). Delta power values could be potentially used in the differential diagnosis between schizophrenia and depression. In patients with MDD, delta power over Fp1, Fp2, F4, and F8 regions was lower in comparison to schizophrenia patients (37). Impaired development of a resting-state brain network in adolescents with MDD may represent an intermediate phenotype that can be assessed with QEEG. Youth with MDD showed decreased resting connectivity in the alpha and theta frequency bands, particularly in the frontal cortex (38). In addition, Moon et. al found increased overall absolute delta power and relative gamma power as potential markers that could differentiate post-traumatic stress disorder (PTSD) from anxiety disorders (39).
Knowledge Base of QEEG Research in the Neuropsychiatric Field
To better clarify the knowledge base of QEEG research in the neuropsychiatric field, we analyzed the cited literature and the citing literature in each co-citation cluster (Figure 6 and Table 3). A total of 22 clusters were extracted by CiteSpace through co-cited references analysis. These co-citation reference clusters could be classified into three disease categories: neurodegenerative diseases, cerebrovascular diseases, and mental disorders (Table 4). In these three disease categories, QEEG has been applied to investigate the pathological mechanisms, assist clinical diagnosis, and promote the selection of appropriate treatments. The most relevant co-cited articles and citing articles are listed in Supplementary Table S1.
In chronic neurodegenerative diseases, the majority of QEEG research is about Alzheimer's disease (AD) and Parkinson's disease (PD). In AD research, QEEG was mainly used to explore the pathology and diagnosis of AD. In the study of pathological mechanisms underlying AD, cluster 8 “schizophrenia” showed that there were similar QEEG characteristics between AD and schizophrenia (40–42) and that schizophrenia was associated with an elevated risk of developing AD (43), which suggested that the pathological mechanisms of these two diseases may be related. The co-cited references of cluster 10 “human immunodeficiency virus (HIV)” indicated that cortical source mapping by low-resolution brain electromagnetic source tomography (LORETA) of resting state EEG rhythms could characterize neurodegenerative disorders-induced cognitive impairment, such as Parkinson's disease related dementia (PDD) and Alzheimer's disease (AD) (44, 45), while the citing documents in this cluster showed that HIV research also applied LORETA in evaluating the cognitive functions in patients with HIV (46, 47). In the study of AD diagnosis, cluster 9 “Alzheimer's disease” indicated that QEEG can accurately differentiate the stage of AD (48–51). Cluster 0 “mild cognitive impairment (MCI)” suggested that QEEG was a valuable tool for the early diagnosis of AD (52–56). For PD patients (co-cited references of cluster 4 “machine learning” indicated that QEEG could provide reliable biomarkers for nonmotor symptom severity and progression (28, 57). Besides, the citing articles in this cluster pointed out that preoperative QEEG biomarkers could predict cognitive deterioration of PD after subthalamic deep brain stimulation with high accuracy by using a machine learning pipeline (58, 59).
In the study of acute neurodegenerative diseases, QEEG was also an important method to study epilepsy pathology, epilepsy prediction, epilepsy detection, and epilepsy treatment. In the research of pathological mechanisms of epilepsy, co-cited references of cluster 17 “stereo eeg” demonstrated that QEEG could be used to explore the desynchronization and synchronous discharge of neurons in different stages of epilepsy (60, 61). The citing literature of this cluster showed that quantitative stereo EEG could be used to analyze the inhibitory and promoting factors of seizures in inter-ictal period (62, 63). Based on the hypersynchronization hypothesis of epileptic seizures, cluster 13 “seizure anticipation” found that the trend of abnormal synchronization of neurons can be detected by QEEG nonlinear analysis to predict epileptic seizures (64–66). In terms of epilepsy detection, the co-cited literature of cluster 5 “nonconvulsive status epilepticus” showed that the use of QEEG could accurately diagnose epilepsy (67–69), and the citing literature of this cluster showed that QEEG could also be used to monitor nonconvulsive status epilepticus (70). In addition, cluster 15 “seizure detection” showed that QEEG combined with quantitative electromyography (EMG) can identify the characteristics of different epileptic subtypes (71, 72). In terms of epilepsy treatment, citing articles of cluster 16 “dysplasia focal cortical” indicated that the QEEG index can provide a reliable basis for determining epileptic focus before the surgical treatment of focal epilepsy (73), and the co-cited documents in this cluster proved that QEEG index could accurately predict the surgical prognosis of epilepsy (74, 75). Cluster 15 also showed that QEEG could help to predict and prevent sudden unexpected death in epilepsy (SUDEP) (76, 77).
In cerebrovascular diseases, according to the co-cited literature of cluster 2 “cardiac arrest” (CA) and cluster 7 “migraine,” EEG signals mainly come from the activities of pyramidal cells in the cerebral cortex, which are vulnerable to cerebral ischemia (78), so QEEG is suitable for detecting abnormal neural activities of ischemic stroke (IS) and evaluating the IS prognosis (79–81). The study of EEG characteristics of cerebral ischemia is also helpful to investigate other related diseases. Citing documents of cluster 2 suggested that cardiac arrest would cause secondary ischemic stroke. Therefore, even if cardiopulmonary resuscitation is successfully accepted, patients may have neurological sequelae. QEEG index can reflect brain activity in real time and assist doctors to judge the prognosis of patients with CA and take corresponding treatment in time (82, 83). The citing literature of cluster 7 showed that because migraine and ischemic stroke have similar EEG characteristics, it was speculated that the change in cerebral blood activity may be one of the manifestations of migraine (84, 85). In addition, according to cluster 29 “peri-/intraventricular hemorrhage (PIVH),” QEEG has also been used in the early diagnosis of intracerebral hemorrhage in premature infants in recent years (86–89). In brief, the knowledge base of QEEG in cerebrovascular diseases is mainly about monitoring and treating the abnormal brain functions related to cerebrovascular disease by using QEEG.
In the studies related to mental disorders, attention-deficit hyperactivity disorder (ADHD) and depression are two major application fields of QEEG. The cited and citing literature in cluster 1 “attention-deficit hyperactivity disorder” included various types of QEEG studies on ADHD, including the use of QEEG to study the etiology, diagnostic biomarkers, prognostic biomarkers, and add-on treatment of ADHD (20, 52, 86–93). First, the literature of cluster 1 indicated that QEEG could verify different etiological hypotheses of ADHD (90). Second, cluster 1 also denoted that QEEG could accurately judge the abnormal brain activities associated with ADHD (20, 93), and cluster 3 “theta/beta ratio” indicated that theta/beta ratio might be used as an index to identify ADHD subtypes (21, 94, 95). Third, the literature in cluster 20 “methylphenidate” mainly used the QEEG index to evaluate the efficacy of different drugs in the treatment of ADHD (96–98). Among them, methylphenidate has been proven to be a drug that can effectively alleviate the symptoms of ADHD (97, 98). Finally, it is particularly noteworthy that ADHD is the major application field of neurofeedback therapy. Cluster 11 “neurofeedback” showed that many studies have proved that neurofeedback therapy can effectively treat ADHD (54, 99–102), particularly when targeted, personalized neurofeedback treatment was applied (102). Moreover, the co-cited references of cluster 20 also showed the long-term efficacy of neurofeedback in the treatment of ADHD (103, 104). Therefore, the application of QEEG in the field of ADHD has a relatively good research foundation.
For the knowledge base of QEEG studies in other mental disorders, most studies on depression focused on evaluating the efficacy of antidepressants with QEEG indicators. The citing literature of cluster 6 “depression” (105, 106) and the co-cited literature in cluster 14 “biomarker” (107, 108) suggested that there may be methodological differences among studies and a lack of replications in this research area, so there is still no widely recognized QEEG index that can accurately predict the efficacy of antidepressants. Particularly, on the one hand, the co-cited literature in cluster 12 “cordance” suggested that cordance, a QEEG index that can comprehensively analyze relative EEG power and absolute EEG power and highlight the brain pathological activities (109), has not been able to predict the efficacy of antidepressants (110–112). On the other hand, the co-cited literature in cluster 6 (113–115) and the citing literature from cluster 12 (80, 116) showed that prefrontal theta cordance has the value of predicting the response of antidepressants, which indicated that the cordance index still has the potential for further research. Moreover, citing articles of cluster 14 argued that researchers can try using machine learning to explore QEEG biomarkers for evaluating the efficacy of antidepressants (117, 118).
Caffeine withdrawal response and sleep disorder are the remaining two clusters identified by CiteSpace, suggesting wide applications of QEEG in the neuropsychiatric field. The literature in cluster 21 “caffeine” showed that QEEG can be used to study the neural mechanism underlying the withdrawal response to drugs, such as caffeine and cocaine (119, 120). Cluster 24 “obstructive sleep apnea (OSA)” suggested that QEEG during sleep could help to reveal the pathological mechanism of OSA, while awake QEEG could evaluate the impact of OSA on cognitive functions (118, 121, 122).
Emerging Trends and Future Direction of QEEG Research in Neuropsychiatric Disorders
Overlay visualization presented the time of emergence of the keywords and reflected the latest and emerging research topics. From the overlay map shown in Figure 7, we can see that the recently searched keywords are shown by yellow nodes. The emerging keywords were “biomarker,” “connectivity,” and “machine learning.”
As for QEEG biomarker research, recent studies started to cross-validate the prognostic value of previously suggested EEG biomarkers in larger independent datasets, since an increasing number of QEEG biomarkers in neuropsychiatric disorders were revealed in prior studies. For example, Ip and colleagues showed that alpha asymmetry seems to be the most promising EEG biomarker for the prediction of treatment response in women with MDD in comparison to alpha power, delta and theta activity at the anterior cingulate cortex (ACC) (123). Moreover, new QEEG biomarkers have also been investigated. Interictal high-frequency oscillation and modulation index have been found to improve the prediction accuracy of post-operative seizure outcomes (124).
QEEG-based functional connectivity has also been investigated in recent years as a diagnostic tool to predict the symptom severity of neuropsychiatric disorders. EEG functional connectivity has shown promising results as a diagnostic tool for AD. Similarly, in Down syndrome (DS) with Alzheimer's dementia, decreased alpha and increased delta coherence and weighted phase lag index were observed when compared to DS (125). EEG functional connectivity and complexity were used to predict depression severity among depressive patients. A significant negative relationship was found between graph metrics (i.e., degree and clustering coefficient) and depression severity in the alpha band, while the EEG complexity measures in alpha and delta bands by the nonlinear analysis were positively associated with symptom severity (126).
Another breakthrough is QEEG-based machine learning studies. Through machine learning, a compound of automatically extracted EEG biomarkers differentiated good vs. poor cognitive function of PD patients with higher accuracy than a single spectral EEG feature (58). More QEEG biomarkers (e.g., coherence, spectral, and event-related potentials) should be investigated and combined with machine learning or deep learning methods to predict the occurrence, severity, and treatment response for neuropsychiatric disorders.
Strengths and Limitations
Our bibliometric study has several strengths. First of all, it is the first study to use the scientometric method to summarize the research history and development trends of QEEG studies in the neuropsychiatric field. It included the most comprehensive analysis, covering nearly all aspects of previous publications, and provided valuable information to QEEG researchers and helped them gain a better insight into the evolving research foci and trends. However, our study was also subjective to several limitations. First, the data come merely from WoSCC, and other databases, such as Embase or PubMed, were not searched, and hence this study may not completely represent all QEEG data. But notably, WoSCC is the most frequently used database for scientometric research. Second, the retrieved articles were restricted to those published in English, resulting in some linguistic bias.
Conclusion
The present study performed a bibliometric analysis of the overall scientific output of QEEG research in the neuropsychiatric field from 2000 to 2021. During the last two decades, QEEG has been applied to reveal the pathological mechanisms, assist clinical diagnosis, and promote the selection of effective treatments for a variety of neuropsychiatric diseases, including neurodegenerative diseases, cerebrovascular diseases, and mental diseases. Studies in these disease categories and domains added to the knowledge base of this research field. The hot topics of research included five major neuropsychiatric disorders, including ADHD, neurodegenerative disorders like Alzheimer's and Parkinson's disease, traumatic brain injury and related cerebrovascular diseases, epilepsy and seizure, and other psychiatric diseases, such as MDD and schizophrenia. Besides, future studies should focus on cross-validating promising QEEG biomarkers, developing new biomarkers (e.g, functional connectivity and complexity), and extracting biomarkers by machine learning.
Author Contributions
SY and JZ contributed equally to the analysis of the data and wrote the manuscript. SL collected the bibliometric data and prepared the figures. RZ interpreted the data and revised the manuscript. JZ revised the manuscript. XY and YW designed the study, interpreted the data, and revised the manuscript. All authors contributed to the article and approved the submitted version.
Funding
This study was funded by the National Natural Science Foundation of China (grant nos: 31800928 and 72174082), Guangdong Provincial Philosophy and Social Sciences 13th Five-Year Plan Co-construction Project (grant no: GD18XXL04), and Guangzhou Philosophy and Social Sciences Development 13th Five-Year Plan Young Scholar Project of City of Rams (grant no: 2020GZQN42). Innovation and Entrepreneurship Training Program for College Students (project no: S202012121176).
Conflict of Interest
The authors declare that the research was conducted in the absence of any commercial or financial relationships that could be construed as a potential conflict of interest.
Publisher's Note
All claims expressed in this article are solely those of the authors and do not necessarily represent those of their affiliated organizations, or those of the publisher, the editors and the reviewers. Any product that may be evaluated in this article, or claim that may be made by its manufacturer, is not guaranteed or endorsed by the publisher.
Acknowledgments
We would like to thank Yabin Tang from Southern Medical University for grammar revision.
Supplementary Material
The Supplementary Material for this article can be found online at: https://www.frontiersin.org/articles/10.3389/fpsyt.2022.830819/full#supplementary-material
References
1. Paul K, Dittrichova J. The process of falling asleep in infancy [proceedings]. Act Nerv Super (Praha). (1977) 19:272–3.
2. Huang X, Fan X, Ying J, Chen S. Emerging trends and research foci in gastrointestinal microbiome. J Transl Med. (2019) 17:67. doi: 10.1186/s12967-019-1810-x
3. Berger H. Über das Elektrenkephalog“.ramm des Menschen. Archiv für Psychiatrie und Nervenkrankheiten. (1929) 87:527–70. doi: 10.1007/BF01797193
4. Mari-Acevedo J, Yelvington K, Tatum WO. Chapter 9 - Normal EEG variants. In: Levin KH, Chauvel P, editors. Handb Clin Neurol. Vol 160. Amsterdam: Elsevier (2019). p. 143–60. doi: 10.1016/B978-0-444-64032-1.00009-6
5. Okumura A. Chapter 8-electroencephalography in children with acute encephalitis/encephalopathy. In: Yamanouchi H, Moshé SL, Okumura A, editors. Acute Encephalopathy and Encephalitis in Infancy and Its Related Disorders. Missouri: Elsevier (2018). p. 63–70. doi: 10.1016/B978-0-323-53088-0.00008-7
6. Bailey T. Chapter thirteen-diagnosing and treating developmental disorders with qEEG and neurotherapy. In: Cantor DS, Evans JR, editors. Clinical Neurotherapy. Boston, MA: Academic Press (2014). p. 321–55.
7. Johnstone J, Lunt J. Chapter 1-use of quantitative EEG to Predict therapeutic outcome in neuropsychiatric disorders. In: Coben R, Evans JR, editors. Neurofeedback and Neuromodulation Techniques and Applications. San Diego, CA: Academic Press (2011). p. 3–23.
8. Nuwer M. Assessment of digital EEG, quantitative EEG, and EEG brain mapping: report of the American Academy of Neurology and the American Clinical Neurophysiology Society. Neurology. (1997) 49:277–92. doi: 10.1212/WNL.49.1.277
9. Nuwer MR. Quantitative EEG: I. Techniques and problems of frequency analysis and topographic mapping. J Clin Neurophysiol. (1988) 5:1–43. doi: 10.1097/00004691-198801000-00001
10. Nuwer MR. Quantitative EEG: II. Frequency analysis and topographic mapping in clinical settings. J Clin Neurophysiol. (1988) 5:45–85. doi: 10.1097/00004691-198801000-00002
11. Livint Popa L, Dragos H, Pantelemon C, Verisezan Rosu O, Strilciuc S. The role of quantitative EEG in the diagnosis of neuropsychiatric disorders. J Med Life. (2020) 13:8–15. doi: 10.25122/jml-2019-0085
12. McVoy M, Lytle S, Fulchiero E, Aebi ME, Adeleye O, Sajatovic M. A systematic review of quantitative EEG as a possible biomarker in child psychiatric disorders. Psychiatry Res. (2019) 279:331–44. doi: 10.1016/j.psychres.2019.07.004
13. Smailovic U, Jelic V. Neurophysiological markers of Alzheimer's disease: quantitative EEG approach. Neurol Ther. (2019) 8(Suppl. 2):37–55. doi: 10.1007/s40120-019-00169-0
14. Wang X, Xu Z, Su S-F, Zhou W. A comprehensive bibliometric analysis of uncertain group decision making from 1980 to 2019. Inf Sci. (2021) 547:328–53. doi: 10.1016/j.ins.2020.08.036
15. Chen C, Lou Y, Li X-Y, Lv Z-T, Zhang L-Q, Mao W. Mapping current research and identifying hotspots on mesenchymal stem cells in cardiovascular disease. Stem Cell Res Ther. (2020) 11:498. doi: 10.1186/s13287-020-02009-7
16. Guo Y-M, Huang Z-L, Guo J, Guo X-R, Li H, Liu M-Y, et al. A bibliometric analysis and visualization of blockchain. Future Generat Comput Syst. (2021) 116:316–32. doi: 10.1016/j.future.2020.10.023
17. Bertoglio R, Corbo C, Renga F, Matteucci M. The digital agricultural revolution: a bibliometric analysis literature review. IEEE Access. (2021) 9:134762–82. doi: 10.1109/ACCESS.2021.3115258
18. Rousseeuw PJ. Silhouettes: a graphical aid to the interpretation and validation of cluster analysis. J Comput Appl Math. (1987) 20:53–65. doi: 10.1016/0377-0427(87)90125-7
19. Bresnahan SM, Barry RJ. Specificity of quantitative EEG analysis in adults with attention deficit hyperactivity disorder. Psychiatry Res. (2002) 112:133–44. doi: 10.1016/S0165-1781(02)00190-7
20. Barry RJ, Clarke AR, Johnstone SJ. A review of electrophysiology in attention-deficit/hyperactivity disorder: I. Qualitative and quantitative electroencephalography. Clin Neurophysiol. (2003) 114:171–83. doi: 10.1016/S1388-2457(02)00362-0
21. Ogrim G, Kropotov J, Hestad K. The quantitative EEG theta/beta ratio in attention deficit/hyperactivity disorder and normal controls: sensitivity, specificity, and behavioral correlates. Psychiatry Res. (2012) 198:482–8. doi: 10.1016/j.psychres.2011.12.041
22. Tombor L, Kakuszi B, Papp S, Réthelyi J, Bitter I, Czobor P. Decreased resting gamma activity in adult attention deficit/hyperactivity disorder. World J Biol Psychiatry. (2019) 20:691–702. doi: 10.1080/15622975.2018.1441547
23. Liao YC, Guo NW, Su BY, Chen SJ, Tsai HF, Lee KY. Frontal beta activity in the meta-intention of children with attention deficit hyperactivity disorder. Clin EEG Neurosci. (2021) 52:136–43. doi: 10.1177/1550059420933142
24. Müller A, Vetsch S, Pershin I, Candrian G, Baschera GM, Kropotov JD, et al. EEG/ERP-based biomarker/neuroalgorithms in adults with ADHD: development, reliability, and application in clinical practice. World J Biol Psychiatry. (2020) 21:172–82. doi: 10.1080/15622975.2019.1605198
25. Knott V, Mohr E, Mahoney C, Ilivitsky V. Quantitative electroencephalography in Alzheimer's disease: comparison with a control group, population norms and mental status. J Psychiatry Neurosci. (2001) 26:106–16.
26. Kai T, Asai Y, Sakuma K, Koeda T, Nakashima K. Quantitative electroencephalogram analysis in dementia with Lewy bodies and Alzheimer's disease. J Neurol Sci. (2005) 237:89–95. doi: 10.1016/j.jns.2005.05.017
27. Fonseca LC, Tedrus GM, Letro GH, Bossoni AS. Dementia, mild cognitive impairment and quantitative EEG in patients with Parkinson's disease. Clin EEG Neurosci. (2009) 40:168–72. doi: 10.1177/155005940904000309
28. Geraedts VJ, Boon LI, Marinus J, Gouw AA, van Hilten JJ, Stam CJ, et al. Clinical correlates of quantitative EEG in Parkinson disease: a systematic review. Neurology. (2018) 91:871–83. doi: 10.1212/WNL.0000000000006473
29. Larsson PG, Kostov H. Lower frequency variability in the alpha activity in EEG among patients with epilepsy. Clin Neurophysiol. (2005) 116:2701–6. doi: 10.1016/j.clinph.2005.07.019
30. Park SC, Lee SK, Che H, Chung CK. Ictal high-gamma oscillation (60-99 Hz) in intracranial electroencephalography and postoperative seizure outcome in neocortical epilepsy. Clin Neurophysiol. (2012) 123:1100–10. doi: 10.1016/j.clinph.2012.01.008
31. Fonseca E, Quintana M, Seijo-Raposo I, Ortiz de Zárate Z, Abraira L, Santamarina E, et al. Interictal brain activity changes in temporal lobe epilepsy: a quantitative electroencephalogram analysis. Acta Neurol Scand. (2022) 145:239–48. doi: 10.1111/ane.13543
32. Pauli R, O'Donnell A, Cruse D. Resting-State electroencephalography for prognosis in disorders of consciousness follocluster traumatic brain injury. Front Neurol. (2020) 11:586945. doi: 10.3389/fneur.2020.586945
33. Mueller TM, Gollwitzer S, Hopfengärtner R, Rampp S, Lang JD, Stritzelberger J, et al. Alpha power decrease in quantitative EEG detects development of cerebral infarction after subarachnoid hemorrhage early. Clin Neurophysiol. (2021) 132:1283–9. doi: 10.1016/j.clinph.2021.03.005
34. Yu Z, Wen D, Zheng J, Guo R, Li H, You C, et al. Predictive accuracy of alpha-delta ratio on quantitative electroencephalography for delayed cerebral ischemia in patients with aneurysmal subarachnoid hemorrhage: meta-analysis. World Neurosurg. (2019) 126:e510–6. doi: 10.1016/j.wneu.2019.02.082
35. Grin-Yatsenko VA, Ponomarev VA, Pronina MV, Poliakov YI, Plotnikova IV, Kropotov JD. Local and widely distributed EEG activity in schizophrenia with prevalence of negative symptoms. Clin EEG Neurosci. (2017) 48:307–15. doi: 10.1177/1550059416683283
36. Grin-Yatsenko VA, Baas I, Ponomarev VA, Kropotov JD. EEG power spectra at early stages of depressive disorders. J Clin Neurophysiol. (2009) 26:401–6. doi: 10.1097/WNP.0b013e3181c298fe
37. Begić D, Popović-Knapić V, Grubišin J, Kosanović-Rajačić B, Filipčić I, Telarović I, et al. Quantitative electroencephalography in schizophrenia and depression. Psychiatr Danub. (2011) 23:355–62.
38. McVoy M, Aebi ME, Loparo K, Lytle S, Morris A, Woods N, et al. Resting-state quantitative electroencephalography demonstrates differential connectivity in adolescents with major depressive disorder. J Child Adolesc Psychopharmacol. (2019) 29:370–7. doi: 10.1089/cap.2018.0166
39. Moon SY, Choi YB, Jung HK, Lee YI, Choi SH. Increased frontal gamma and posterior delta powers as potential neurophysiological correlates differentiating posttraumatic stress disorder from anxiety disorders. Psychiatry Investig. (2018) 15:1087–93. doi: 10.30773/pi.2018.09.30
40. Chiaramonti R, Muscas GC, Paganini M, Müller TJ, Fallgatter AJ, Versari A, et al. Correlations of topographical EEG features with clinical severity in mild and moderate dementia of Alzheimer type. Neuropsychobiology. (1997) 36:153–8. doi: 10.1159/000119375
41. Pascual-Marqui RD, Lehmann D, Koenig T, Kochi K, Merlo MC, Hell D, et al. Low resolution brain electromagnetic tomography (LORETA) functional imaging in acute, neuroleptic-naive, first-episode, productive schizophrenia. Psychiatry Res. (1999) 90:169–79. doi: 10.1016/S0925-4927(99)00013-X
42. Wienbruch C, Moratti S, Elbert T, Vogel U, Fehr T, Kissler J, et al. Source distribution of neuromagnetic slow wave activity in schizophrenic and depressive patients. Clin Neurophysiol. (2003) 114:2052–60. doi: 10.1016/S1388-2457(03)00210-4
43. Kochunov P, Zavaliangos-Petropulu A, Jahanshad N, Thompson PM, Ryan MC, Chiappelli J, et al. A white matter connection of schizophrenia and Alzheimer's disease. Schizophr Bull. (2021) 47:197–206. doi: 10.1093/schbul/sbaa078
44. Caviness JN, Hentz JG, Belden CM, Shill HA, Driver-Dunckley ED, Sabbagh MN, et al. Longitudinal EEG changes correlate with cognitive measure deterioration in Parkinson's disease. J Parkinsons Dis. (2015) 5:117–24. doi: 10.3233/JPD-140480
45. Babiloni C, De Pandis MF, Vecchio F, Buffo P, Sorpresi F, Frisoni GB, et al. Cortical sources of resting state electroencephalographic rhythms in Parkinson's disease related dementia and Alzheimer's disease. Clin Neurophysiol. (2011) 122:2355–64. doi: 10.1016/j.clinph.2011.03.029
46. Babiloni C, Pennica A, Del Percio C, Noce G, Cordone S, Muratori C, et al. Abnormal cortical sources of resting state electroencephalographic rhythms in single treatment-naïve HIV individuals: a statistical z-score index. Clin Neurophysiol. (2016) 127:1803–12. doi: 10.1016/j.clinph.2015.12.007
47. Babiloni C, Buffo P, Vecchio F, Onorati P, Muratori C, Ferracuti S, et al. Cortical sources of resting-state EEG rhythms in ”experienced“ HIV subjects under antiretroviral therapy. Clin Neurophysiol. (2014) 125:1792–802. doi: 10.1016/j.clinph.2014.01.024
48. Wolf H, Jelic V, Gertz HJ, Nordberg A, Julin P, Wahlund LO. A critical discussion of the role of neuroimaging in mild cognitive impairment. Acta Neurol Scand Suppl. (2003) 179:52–76. doi: 10.1034/j.1600-0404.107.s179.10.x
49. Huang C, Wahlund L, Dierks T, Julin P, Winblad B, Jelic V. Discrimination of Alzheimer's disease and mild cognitive impairment by equivalent EEG sources: a cross-sectional and longitudinal study. Clin Neurophysiol. (2000) 111:1961–7. doi: 10.1016/S1388-2457(00)00454-5
50. Rodriguez G, Copello F, Vitali P, Perego G, Nobili F. EEG spectral profile to stage Alzheimer's disease. Clin Neurophysiol. (1999) 110:1831–7. doi: 10.1016/S1388-2457(99)00123-6
51. Jelic V, Johansson SE, Almkvist O, Shigeta M, Julin P, Nordberg A, et al. Quantitative electroencephalography in mild cognitive impairment: longitudinal changes and possible prediction of Alzheimer's disease. Neurobiol Aging. (2000) 21:533–40. doi: 10.1016/S0197-4580(00)00153-6
52. Jeong J. EEG dynamics in patients with Alzheimer's disease. Clin Neurophysiol. (2004) 115:1490–505. doi: 10.1016/j.clinph.2004.01.001
53. Jackson CE, Snyder PJ. Electroencephalography and event-related potentials as biomarkers of mild cognitive impairment and mild Alzheimer's disease. Alzheimers Dement. (2008) 4(1 Suppl. 1):S137–43. doi: 10.1016/j.jalz.2007.10.008
54. Jelic V, Kowalski J. Evidence-based evaluation of diagnostic accuracy of resting EEG in dementia and mild cognitive impairment. Clin EEG Neurosci. (2009) 40:129–42. doi: 10.1177/155005940904000211
55. Babiloni C, Binetti G, Cassetta E, Cerboneschi D, Dal Forno G, Del Percio C, et al. Mapping distributed sources of cortical rhythms in mild Alzheimer's disease. A multicentric EEG study. Neuroimage. (2004) 22:57–67. doi: 10.1016/j.neuroimage.2003.09.028
56. Babiloni C, Binetti G, Cassetta E, Dal Forno G, Del Percio C, Ferreri F, et al. Sources of cortical rhythms change as a function of cognitive impairment in pathological aging: a multicenter study. Clin Neurophysiol. (2006) 117:252–68. doi: 10.1016/j.clinph.2005.09.019
57. Engedal K, Snaedal J, Hoegh P, Jelic V, Bo Andersen B, Naik M, et al. Quantitative EEG applying the statistical recognition pattern method: a useful tool in dementia diagnostic workup. Dement Geriatr Cogn Disord. (2015) 40:1–12. doi: 10.1159/000381016
58. Geraedts VJ, Koch M, Contarino MF, Middelkoop HAM, Wang H, van Hilten JJ, et al. Machine learning for automated EEG-based biomarkers of cognitive impairment during deep brain stimulation screening in patients with Parkinson's disease. Clin Neurophysiol. (2021) 132:1041–48. doi: 10.1016/j.clinph.2021.01.021
59. Geraedts VJ, Koch M, Kuiper R, Kefalas M, Bäck THW, van Hilten JJ, et al. Preoperative electroencephalography-based machine learning predicts cognitive deterioration after subthalamic deep brain stimulation. Mov Disord. (2021) 36:2324–34. doi: 10.1002/mds.28661
60. Frauscher B, von Ellenrieder N, Ferrari-Marinho T, Avoli M, Dubeau F, Gotman J. Facilitation of epileptic activity during sleep is mediated by high amplitude slow waves. Brain. (2015) 138(Pt 6):1629–41. doi: 10.1093/brain/awv073
61. Jiruska P, de Curtis M, Jefferys JG, Schevon CA, Schiff SJ, Schindler K. Synchronization and desynchronization in epilepsy: controversies and hypotheses. J Physiol. (2013) 591:787–97. doi: 10.1113/jphysiol.2012.239590
62. Zubler F, Rubino A, Lo Russo G, Schindler K, Nobili L. Correlating interictal spikes with sigma and delta dynamics during non-rapid-eye-movement-sleep. Front Neurol. (2017) 8:288. doi: 10.3389/fneur.2017.00288
63. Campana C, Zubler F, Gibbs S, de Carli F, Proserpio P, Rubino A, et al. Suppression of interictal spikes during phasic rapid eye movement sleep: a quantitative stereo-electroencephalography study. J Sleep Res. (2017) 26:606–613. doi: 10.1111/jsr.12533
64. Martinerie J, Adam C, Le Van Quyen M, Baulac M, Clemenceau S, Renault B, et al. Epileptic seizures can be anticipated by non-linear analysis. Nat Med. (1998) 4:1173–6. doi: 10.1038/2667
65. Le Van Quyen M, Adam C, Martinerie J, Baulac M, Clémenceau S, Varela F. Spatio-temporal characterizations of non-linear changes in intracranial activities prior to human temporal lobe seizures. Eur J Neurosci. (2000) 12:2124–34. doi: 10.1046/j.1460-9568.2000.00088.x
66. van Drongelen W, Nayak S, Frim DM, Kohrman MH, Towle VL, Lee HC, et al. Seizure anticipation in pediatric epilepsy: use of Kolmogorov entropy. Pediatr Neurol. (2003) 29:207–13. doi: 10.1016/S0887-8994(03)00145-0
67. Swisher CB, White CR, Mace BE, Dombrowski KE, Husain AM, Kolls BJ, et al. Diagnostic accuracy of electrographic seizure detection by neurophysiologists and non-neurophysiologists in the adult ICU using a panel of quantitative EEG trends. J Clin Neurophysiol. (2015) 32:324–30. doi: 10.1097/WNP.0000000000000144
68. Finnigan S, Wong A, Read S. Defining abnormal slow EEG activity in acute ischaemic stroke: delta/alpha ratio as an optimal QEEG index. Clin Neurophysiol. (2016) 127:1452–59. doi: 10.1016/j.clinph.2015.07.014
69. Haider HA, Esteller R, Hahn CD, Westover MB, Halford JJ, Lee JW, et al. Sensitivity of quantitative EEG for seizure identification in the intensive care unit. Neurology. (2016) 87:935–44. doi: 10.1212/WNL.0000000000003034
70. Hernández-Hernández MA, Fernández-Torre JL. Color density spectral array of bilateral bispectral index system: electroencephalographic correlate in comatose patients with nonconvulsive status epilepticus. Seizure. (2016) 34:18–25. doi: 10.1016/j.seizure.2015.11.001
71. Beniczky S, Conradsen I, Pressler R, Wolf P. Quantitative analysis of surface electromyography: biomarkers for convulsive seizures. Clin Neurophysiol. (2016) 127:2900–7. doi: 10.1016/j.clinph.2016.04.017
72. Beniczky S, Conradsen I, Henning O, Fabricius M, Wolf P. Automated real-time detection of tonic-clonic seizures using a wearable EMG device. Neurology. (2018) 90:e428–34. doi: 10.1212/WNL.0000000000004893
73. Lemesle M, Berriolo-Riedinger A, Touzery C, Guy F, Toubeau M, Crevenat E, et al. Correlation between inter-ictal regional cerebral blood flow and sphenoidal electrodes–recorded inter-ictal spikes in mesial temporal lobe epilepsy. Neurol Res. (2000) 22:674–8. doi: 10.1080/01616412.2000.11740738
74. Cascino GD, Trenerry MR, So EL, Sharbrough FW, Shin C, Lagerlund TD, et al. Routine EEG and temporal lobe epilepsy: relation to long-term EEG monitoring, quantitative MRI, and operative outcome. Epilepsia. (1996) 37:651–6. doi: 10.1111/j.1528-1157.1996.tb00629.x
75. Lee BI, Lee JD, Kim JY, Ryu YH, Kim WJ, Lee JH, et al. Single photon emission computed tomography-EEG relations in temporal lobe epilepsy. Neurology. (1997) 49:981–91. doi: 10.1212/WNL.49.4.981
76. Alexandre V, Mercedes B, Valton L, Maillard L, Bartolomei F, Szurhaj W, et al. Risk factors of postictal generalized EEG suppression in generalized convulsive seizures. Neurology. (2015) 85:1598–603. doi: 10.1212/WNL.0000000000001949
77. Arbune AA, Conradsen I, Cardenas DP, Whitmire LE, Voyles SR, Wolf P, et al. Ictal quantitative surface electromyography correlates with postictal EEG suppression. Neurology. (2020) 94:e2567–76. doi: 10.1212/WNL.0000000000009492
78. Finnigan S, van Putten MJ. EEG in ischaemic stroke: quantitative EEG can uniquely inform (sub-)acute prognoses and clinical management. Clin Neurophysiol. (2013) 124:10–9. doi: 10.1016/j.clinph.2012.07.003
79. Finnigan SP, Walsh M, Rose SE, Chalk JB. Quantitative EEG indices of sub-acute ischaemic stroke correlate with clinical outcomes. Clin Neurophysiol. (2007) 118:2525–32. doi: 10.1016/j.clinph.2007.07.021
80. Hunter AM, Cook IA, Leuchter AF. The promise of the quantitative electroencephalogram as a predictor of antidepressant treatment outcomes in major depressive disorder. Psychiatr Clin North Am. (2007) 30:105–24. doi: 10.1016/j.psc.2006.12.002
81. Claassen J, Taccone FS, Horn P, Holtkamp M, Stocchetti N, Oddo M. Recommendations on the use of EEG monitoring in critically ill patients: consensus statement from the neurointensive care section of the ESICM. Intensive Care Med. (2013) 39:1337–51. doi: 10.1007/s00134-013-2938-4
82. Herman ST, Abend NS, Bleck TP, Chapman KE, Drislane FW, Emerson RG, et al. Consensus statement on continuous EEG in critically ill adults and children, part I: indications. J Clin Neurophysiol. (2015) 32:87–95. doi: 10.1097/WNP.0000000000000166
83. Feng G, Jiang G, Li Z, Wang X. Prognostic value of electroencephalography (EEG) for brain injury after cardiopulmonary resuscitation. Neurol Sci. (2016) 37:843–9. doi: 10.1007/s10072-016-2475-3
84. Bjørk MH, Stovner LJ, Engstrøm M, Stjern M, Hagen K, Sand T. Interictal quantitative EEG in migraine: a blinded controlled study. J Headache Pain. (2009) 10:331–9. doi: 10.1007/s10194-009-0140-4
85. Bjørk MH, Stovner LJ, Nilsen BM, Stjern M, Hagen K, Sand T. The occipital alpha rhythm related to the ”migraine cycle" and headache burden: a blinded, controlled longitudinal study. Clin Neurophysiol. (2009) 120:464–71. doi: 10.1016/j.clinph.2008.11.018
86. Vecchierini MF, André M, d'Allest AM. Normal EEG of premature infants born between 24 and 30 weeks gestational age: terminology, definitions and maturation aspects. Neurophysiol Clin. (2007) 37:311–23. doi: 10.1016/j.neucli.2007.10.008
87. André M, Lamblin MD, d'Allest AM, Curzi-Dascalova L, Moussalli-Salefranque F, T SNT, et al. Electroencephalography in premature and full-term infants. Developmental features and glossary. Neurophysiol Clin. (2010) 40:59–124. doi: 10.1016/j.neucli.2010.02.002
88. Bowen JR, Paradisis M, Shah D. Decreased aEEG continuity and baseline variability in the first 48 hours of life associated with poor short-term outcome in neonates born before 29 weeks gestation. Pediatr Res. (2010) 67:538–44. doi: 10.1203/PDR.0b013e3181d4ecda
89. Soubasi V, Mitsakis K, Sarafidis K, Griva M, Nakas CT, Drossou V. Early abnormal amplitude-integrated electroencephalography (aEEG) is associated with adverse short-term outcome in premature infants. Eur J Paediatr Neurol. (2012) 16:625–30. doi: 10.1016/j.ejpn.2012.02.008
90. Clarke AR, Barry RJ, McCarthy R, Selikowitz M. Electroencephalogram differences in two subtypes of attention-deficit/hyperactivity disorder. Psychophysiology. (2001) 38:212–21. doi: 10.1111/1469-8986.3820212
91. Barry RJ, Clarke AR, McCarthy R, Selikowitz M. EEG coherence in attention-deficit/hyperactivity disorder: a comparative study of two DSM-IV types. Clin Neurophysiol. (2002) 113:579–85. doi: 10.1016/S1388-2457(02)00036-6
92. Hermens DF, Soei EX, Clarke SD, Kohn MR, Gordon E, Williams LM. Resting EEG theta activity predicts cognitive performance in attention-deficit hyperactivity disorder. Pediatr Neurol. (2005) 32:248–56. doi: 10.1016/j.pediatrneurol.2004.11.009
93. Monastra VJ, Lubar JF, Linden M, VanDeusen P, Green G, cluster W, et al. Assessing attention deficit hyperactivity disorder via quantitative electroencephalography: an initial validation study. Neuropsychology. (1999) 13:424–33. doi: 10.1037/0894-4105.13.3.424
94. Arns M, Conners CK, Kraemer HC. A decade of EEG theta/beta ratio research in ADHD: a meta-analysis. J Atten Disord. (2013) 17:374–83. doi: 10.1177/1087054712460087
95. Loo SK, Cho A, Hale TS, McGough J, McCracken J, Smalley SL. Characterization of the theta to beta ratio in ADHD: identifying potential sources of heterogeneity. J Atten Disord. (2013) 17:384–92. doi: 10.1177/1087054712468050
96. Ogrim G, Kropotov J, Brunner JF, Candrian G, Sandvik L, Hestad KA. Predicting the clinical outcome of stimulant medication in pediatric attention-deficit/hyperactivity disorder: data from quantitative electroencephalography, event-related potentials, and a go/no-go test. Neuropsychiatr Dis Treat. (2014) 10:231–42. doi: 10.2147/NDT.S56600
97. Janssen TW, Bink M, Geladé K, van Mourik R, Maras A, Oosterlaan J. A randomized controlled trial into the effects of neurofeedback, methylphenidate, and physical activity on EEG power spectra in children with ADHD. J Child Psychol Psychiatry. (2016) 57:633–44. doi: 10.1111/jcpp.12517
98. Bioulac S, Purper-Ouakil D, Ros T, Blasco-Fontecilla H, Prats M, Mayaud L, et al. Personalized at-home neurofeedback compared with long-acting methylphenidate in an european non-inferiority randomized trial in children with ADHD. BMC Psychiatry. (2019) 19:237. doi: 10.1186/s12888-019-2218-0
99. Gevensleben H, Holl B, Albrecht B, Vogel C, Schlamp D, Kratz O, et al. Is neurofeedback an efficacious treatment for ADHD? A randomised controlled clinical trial. J Child Psychol Psychiatry. (2009) 50:780–9. doi: 10.1111/j.1469-7610.2008.02033.x
100. Larsen S, Sherlin L. Neurofeedback: an emerging technology for treating central nervous system dysregulation. Psychiatr Clin North Am. (2013) 36:163–8. doi: 10.1016/j.psc.2013.01.005
101. Hurt E, Arnold LE, Lofthouse N. Quantitative EEG neurofeedback for the treatment of pediatric attention-deficit/hyperactivity disorder, autism spectrum disorders, learning disorders, and epilepsy. Child Adolesc Psychiatr Clin N Am. (2014) 23:465–86. doi: 10.1016/j.chc.2014.02.001
102. Arns M, Drinkenburg W, Leon Kenemans J. The effects of QEEG-informed neurofeedback in ADHD: an open-label pilot study. Appl Psychophysiol Biofeedback. (2012) 37:171–80. doi: 10.1007/s10484-012-9191-4
103. Arns M, Heinrich H, Strehl U. Evaluation of neurofeedback in ADHD: the long and winding road. Biol Psychol. (2014) 95:108–15. doi: 10.1016/j.biopsycho.2013.11.013
104. Van Doren J, Arns M, Heinrich H, Vollebregt MA, Strehl U, S KL. Sustained effects of neurofeedback in ADHD: a systematic review and meta-analysis. Eur Child Adolesc Psychiatry. (2019) 28:293–305. doi: 10.1007/s00787-018-1121-4
105. Leuchter AF, Cook IA, Hunter A, Korb A. Use of clinical neurophysiology for the selection of medication in the treatment of major depressive disorder: the state of the evidence. Clin EEG Neurosci. (2009) 40:78–83. doi: 10.1177/155005940904000207
106. Leuchter AF, Cook IA, Hamilton SP, Narr KL, Toga A, Hunter AM, et al. Biomarkers to predict antidepressant response. Curr Psychiatry Rep. (2010) 12:553–62. doi: 10.1007/s11920-010-0160-4
107. Arns M, Bruder G, Hegerl U, Spooner C, Palmer DM, Etkin A, et al. EEG alpha asymmetry as a gender-specific predictor of outcome to acute treatment with different antidepressant medications in the randomized iSPOT-D study. Clin Neurophysiol. (2016) 127:509–19. doi: 10.1016/j.clinph.2015.05.032
108. Widge AS, Bilge MT, Montana R, Chang W, Rodriguez CI, Deckersbach T, et al. Electroencephalographic biomarkers for treatment response prediction in major depressive illness: a meta-analysis. Am J Psychiatry. (2019) 176:44–56. doi: 10.1176/appi.ajp.2018.17121358
109. Leuchter AF, Cook IA, Lufkin RB, Dunkin J, Newton TF, Cummings JL, et al. Cordance: a new method for assessment of cerebral perfusion and metabolism using quantitative electroencephalography. Neuroimage. (1994) 1:208–19. doi: 10.1006/nimg.1994.1006
110. Cook IA, Leuchter AF, Witte E, Abrams M, Uijtdehaage SH, Stubbeman W, et al. Neurophysiologic predictors of treatment response to fluoxetine in major depression. Psychiatry Res. (1999) 85:263–73. doi: 10.1016/S0165-1781(99)00010-4
111. Cook IA, Leuchter AF, Morgan M, Witte E, Stubbeman WF, Abrams M, et al. Early changes in prefrontal activity characterize clinical responders to antidepressants. Neuropsychopharmacology. (2002) 27:120–31. doi: 10.1016/S0893-133X(02)00294-4
112. Leuchter AF, Cook IA, Witte EA, Morgan M, Abrams M. Changes in brain function of depressed subjects during treatment with placebo. Am J Psychiatry. (2002) 159:122–9. doi: 10.1176/appi.ajp.159.1.122
113. Cook IA, Leuchter AF, Morgan ML, Stubbeman W, Siegman B, Abrams M. Changes in prefrontal activity characterize clinical response in SSRI nonresponders: a pilot study. J Psychiatr Res. (2005) 39:461–6. doi: 10.1016/j.jpsychires.2004.12.002
114. Bares M, Brunovsky M, Kopecek M, Stopkova P, Novak T, Kozeny J, et al. Changes in QEEG prefrontal cordance as a predictor of response to antidepressants in patients with treatment resistant depressive disorder: a pilot study. J Psychiatr Res. (2007) 41:319–25. doi: 10.1016/j.jpsychires.2006.06.005
115. Bares M, Brunovsky M, Kopecek M, Novak T, Stopkova P, Kozeny J, et al. Early reduction in prefrontal theta QEEG cordance value predicts response to venlafaxine treatment in patients with resistant depressive disorder. Eur Psychiatry. (2008) 23:350–5. doi: 10.1016/j.eurpsy.2008.03.001
116. Leuchter AF, Morgan M, Cook IA, Dunkin J, Abrams M, Witte E. Pretreatment neurophysiological and clinical characteristics of placebo responders in treatment trials for major depression. Psychopharmacology (Berl). (2004) 177:15–22. doi: 10.1007/s00213-004-1919-2
117. Jaworska N, de la Salle S, Ibrahim MH, Blier P, Knott V. Leveraging machine learning approaches for predicting antidepressant treatment response using electroencephalography (EEG) and clinical data. Front Psychiatry. (2018) 9:768. doi: 10.3389/fpsyt.2018.00768
118. Appleton SL, Vakulin A, D'Rozario A, Vincent AD, Teare A, Martin SA, et al. Quantitative electroencephalography measures in rapid eye movement and nonrapid eye movement sleep are associated with apnea-hypopnea index and nocturnal hypoxemia in men. Sleep. (2019) 42:zsz092 doi: 10.1093/sleep/zsz092
119. Roemer RA, Cornwell A, Dewart D, Jackson P, Ercegovac DV. Quantitative electroencephalographic analyses in cocaine-preferring polysubstance abusers during abstinence. Psychiatry Res. (1995) 58:247–57. doi: 10.1016/0165-1781(95)02474-B
120. Jones HE, Herning RI, Cadet JL, Griffiths RR. Caffeine withdrawal increases cerebral blood flow velocity and alters quantitative electroencephalography (EEG) activity. Psychopharmacology (Berl). (2000) 147:371–7. doi: 10.1007/s002130050005
121. D'Rozario AL, Cross NE, Vakulin A, Bartlett DJ, Wong KKH, Wang D, et al. Quantitative electroencephalogram measures in adult obstructive sleep apnea-Potential biomarkers of neurobehavioural functioning. Sleep Med Rev. (2017) 36:29–42. doi: 10.1016/j.smrv.2016.10.003
122. Stevens D, Leong CWY, Cheung H, Arciuli J, Vakulin A, Kim JW, et al. Sleep spindle activity correlates with implicit statistical learning consolidation in untreated obstructive sleep apnea patients. Sleep Med. (2021) 86:126–34. doi: 10.1016/j.sleep.2021.01.035
123. Ip CT, Olbrich S, Ganz M, Ozenne B, Köhler-Forsberg K, Dam VH, et al. Pretreatment qEEG biomarkers for predicting pharmacological treatment outcome in major depressive disorder: independent validation from the NeuroPharm study. Eur Neuropsychopharmacol. (2021) 49:101–12. doi: 10.1016/j.euroneuro.2021.03.024
124. Kuroda N, Sonoda M, Miyakoshi M, Nariai H, Jeong JW, Motoi H, et al. Objective interictal electrophysiology biomarkers optimize prediction of epilepsy surgery outcome. Brain Commun. (2021) 3:fcab042. doi: 10.1093/braincomms/fcab042
125. Musaeus CS, Salem LC, Kjaer TW, Waldemar G. Electroencephalographic functional connectivity is altered in persons with Down syndrome and Alzheimer's disease. J Intell Disability Res. (2021) 65:236–45. doi: 10.1111/jir.12803
Keywords: bibliometrics, quantitative electroencephalogram, neuropsychiatric disorders, CiteSpace, VOSviewer
Citation: Yao S, Zhu J, Li S, Zhang R, Zhao J, Yang X and Wang Y (2022) Bibliometric Analysis of Quantitative Electroencephalogram Research in Neuropsychiatric Disorders From 2000 to 2021. Front. Psychiatry 13:830819. doi: 10.3389/fpsyt.2022.830819
Received: 07 December 2021; Accepted: 05 April 2022;
Published: 23 May 2022.
Edited by:
Jing Xiang, Cincinnati Children's Hospital Medical Center, United StatesReviewed by:
Yury (Juri) Kropotov, N. P. Bechtereva Institute of the Human Brain (RAS), RussiaDeniz Doruk, Mayo Clinic, United States
Copyright © 2022 Yao, Zhu, Li, Zhang, Zhao, Yang and Wang. This is an open-access article distributed under the terms of the Creative Commons Attribution License (CC BY). The use, distribution or reproduction in other forums is permitted, provided the original author(s) and the copyright owner(s) are credited and that the original publication in this journal is cited, in accordance with accepted academic practice. No use, distribution or reproduction is permitted which does not comply with these terms.
*Correspondence: Xueling Yang, eWh0eXhsMjAwNiYjeDAwMDQwOzEyNi5jb20=; You Wang, d2FuZ3lvdXBzeSYjeDAwMDQwO2ZveG1haWwuY29t
†These authors have contributed equally to this work and share first authorship