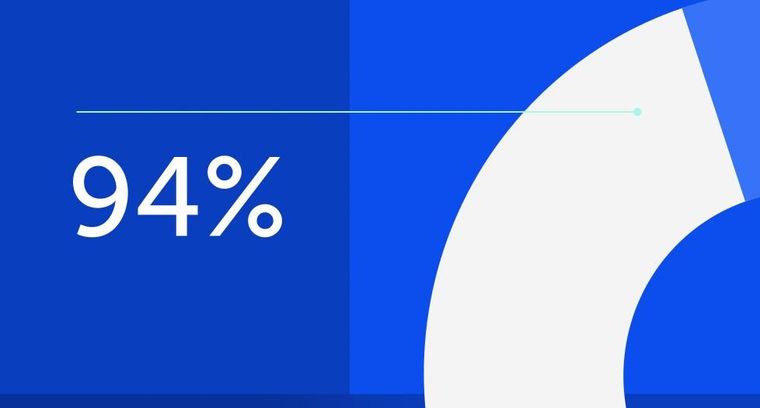
94% of researchers rate our articles as excellent or good
Learn more about the work of our research integrity team to safeguard the quality of each article we publish.
Find out more
SYSTEMATIC REVIEW article
Front. Psychiatry, 06 April 2022
Sec. Addictive Disorders
Volume 13 - 2022 | https://doi.org/10.3389/fpsyt.2022.779239
This article is part of the Research TopicA Changing Epidemic and the Rise of Opioid and Stimulant Co-UseView all 13 articles
Abuses of most illegal drugs, including methamphetamine, marijuana, cocaine, heroin, and polydrug, are usually in conjunction with alcohol and tobacco. There are similarities and associations between the behavior, gene, and neurophysiology of such abusers, but the neural overlaps of their cue-reactivity and the correlation of neural overlap with drug craving still needs to be further explored. In this study, an Activation Likelihood Estimation (ALE) was performed on brain activation under legal (tobacco, alcohol) and illegal drug cues, for identifying the similarities in brain functions between different craving states. A Comprehensive meta-analysis (CMA) on the correlation coefficient between brain activation and craving scores in the selected literatures with subjective craving reports explained the degree of the craving via brain imaging results. In ALE, co-activation areas of the three cue-reactivity (posterior cingulate, caudate, and thalamus) suggest that the three cue-reactivity may all arouse drug-use identity which is a predictor of relapse and generation of conditioned reflexes under reward memory, thus leading to illegal drug relapses. In CMA, the brain activation was significantly correlated with subjective craving, with a correlation coefficient of 0.222. The neural overlap of tobacco, alcohol and most of the prevalent illegal drug cues not only further helps us understand the neural mechanism of substance co-abuse and relapse, but also provides implications to detoxification. Furthermore, the correlation between brain activation and craving is low, suggesting the accuracy of craving-based quantitative evaluation by neuroimaging remains unclear.
Substance abuse is a major culprit damaging human physical and mental health and can even lead to death. Tobacco, alcohol, and illegal drug abuse are particularly serious. Alcohol and tobacco use cause the loss of more than 250 million disability-adjusted life years to humans, and illegal drugs cost tens of millions (1). Alcohol and tobacco are the most commonly abused legal drugs, but the legalization of common drugs of abuse is arbitrary and there is a lack of scientific and systematic criteria for classifying drugs of abuse (2). This may lead to misconceptions about the harm of each drug, and people may simply assume that the abuse of legal drugs is less important than the abuse of illegal drugs, which may not be the case. Nutt et al. (3) developed a nine-category matrix of harm to classify drugs based on physiological impairment, drug dependence, and social impact, and found that tobacco and alcohol were more harmful than some Class A drugs (the most harmful class according to the UK Misuse of Drugs Act) and that their co-abuse with illegal drugs exacerbated the damage.
Tobacco and alcohol abuse can cause damage to the human body in multiple ways. Alcohol abuse causes impairment in executive function, memory, emotional function, and is also a major risk factor for traumatic brain injury (4). Nicotine abuse is strongly associated with the occurrence of sleep disorders, depression, schizophrenia, and anxiety disorders (5). The abuse of illegal drugs has even more serious consequences, as it can lead to acute or subacute leukoencephalopathy, as well as vascular complications, including vasoconstriction, vasculitis, and hypertension (6); it can also severely impair prospective memory—the higher the frequency of cocaine use, the stronger the degree of memory deficit (7).
Illegal drug abuse is often accompanied by tobacco and alcohol abuse (8). Research has found evidence of co-abuse of alcohol, tobacco and illegal drugs. Smoking rates among methamphetamine abusers typically exceed 80% (9). 86.4% of cocaine abusers reported co-abusing tobacco, 99.4% co-abusing alcohol and 95.1% co-abusing cannabis. In a dire co-substance abuse situation, it cannot be ignored that both tobacco and alcohol abuse have significant effects on illegal drug abuse, and alcohol abuse serves as a mediating factor between tobacco and illegal drug use (10). Some studies have found that simultaneous abuse of alcohol and psychostimulants can lead to neurophysiological dysfunctions, such as decreased antioxidant enzymes in the brain, disruption of learning and memory processes, inadequate brain perfusion, and neurotransmitter depletion; as well as increased heart rate, blood pressure, myocardial oxygen consumption, cellular stress, and increased risk of different types of cancer (11). Joint abuse of cocaine and nicotine enhances co-induced locomotor activity, as well as the induction and expression of locomotor sensitization, making each other mutually reinforcing abuse (12). Thus, the concurrence of tobacco, alcohol and illegal drug abuse is highly harmful.
Since abusers' cravings for tobacco and alcohol increase their cravings for drugs, it is proposed that smoking and drinking cessation should be performed simultaneously with detoxification (13–18). But institutional issues and individual health care providers often skip providing concomitant treatment to tobacco and alcohol abusers. Despite evidence that co-abuse of alcohol and cocaine produces unique neuroadaptations, their concomitant treatment needs are far from being met (19). Among alcohol abusers, methamphetamine is the most commonly co-abused illegal drug, but there is no effective treatment for this methamphetamine addiction comorbidity (20). Exploring the exact relationship between tobacco and alcohol abuse and illegal drug abuse can shed light on this dilemma.
Strong genetic and neurophysiological correlations among tobacco abuse, alcohol abuse and drug abuse have been identified. Research on the genetics of co-drug abusers could help develop more effective treatment programs (21–23). By measuring genetic variation, people initially found a certain genetic correlation between nicotine and marijuana (24). Drug abuse can lead to drug addiction. The widespread changes in hippocampal gene expression in both cocaine dependents and alcohol dependents may reflect neuronal adaptation common to both addictions (25). In terms of neurophysiological changes, when both illegal and legal drugs are abused, their interactive effects on neurophysiological mechanisms exacerbate the damage. After co-abuse of tobacco, alcohol and illegal drugs, the brain and biological mechanisms of abusers will have abnormal changes. It has been found that alcohol will increase the concentration of different psychostimulants and their active metabolites in the blood (26). When alcohol is used in conjunction with these drugs, the pharmacokinetics of methamphetamine, cocaine, and nicotine may change (11). Drinking alcohol alone did not affect the levels of dopamine and serotonin in the striatum and prefrontal cortex, but injecting methamphetamine after previously consuming alcohol somehow enhances methamphetamine-induced dopamine and serotonin (27). It can be seen that the abuse of tobacco and alcohol will aggravate the neurophysiological damage of illegal drugs. In addition, the three drug abuses have a common neurophysiological mechanism, such as the reward circuit of abnormal dopamine release (28). Are there overlaps between different cue-induced craving state?
Exploring the neurophysiological mechanism of craving can not only provide theoretical guidance for the “regression model of craving,” but also provide enlightenment for considering whether the craving for one drug triggers the intake of another addictive substance while solving concomitant drug use. In previous studies, methods of “induction under cues” or “physical withdrawal” are generally used to induce subjects' craving for psychoactive substances (29, 30). The measurement of brain changes under cue induction in neuroimaging only proves that the neurophysiological mechanisms caused by the two inducing conditions are different but cannot prove the exactly differences in craving. Therefore, the accuracy of neuroimaging to assess drug craving is often illustrated by the correlation coefficient between its results and subjective self-evaluated craving scores (31). However, the degree of correlation between drug craving scores and activated brain regions was different in different studies. Therefore, in this meta-analysis, we need to clarify the degree of correlation between cue induction and craving.
In a word, Tobacco, alcohol, and drugs are often abused jointly. They have a certain mutual predictive relationship and a common biological mechanism (32). Since craving is a major cause of relapse, research on the impact of tobacco and alcohol craving on drug relapse is critical. Presently, the similarities between the brain mechanisms of legal drug (tobacco and alcohol) cravings and illegal drug cravings are unclear. This study employed activation likelihood estimation meta-analysis (ALE meta-analysis) to conclude similarities in activated brain areas in drug-dependent patients under induction by legal drug (tobacco, alcohol) and illegal drug cues. We hypothesize that these three cues induce some co-activated brain regions. In addition, a Comprehensive Meta-Analysis (CMA) was performed for the correlation coefficients between the brain activation levels and self-reported scores of the cravings. The level of activation of co-activated brain regions may to some extent represent the degree of craving. The results of this study are expected to provide enlightenment for the treatment sequence of tobacco, alcohol, and drugs and the effectiveness of neuroimaging measurements of drug cravings.
After determining the issue for investigation, three sets of search keywords were determined (each set separated by “or”): (1) related words for craving induction by cues—craving/cue; (2) words related to drug addiction—addiction/drug use/drug abuse/drug dependence/substance use/substance abuse/substance dependence/alcohol/ heroin/cocaine/opiate/cannabis/marijuana/nicotine/smoke/tobacco/MDMA/polydrug; and (3) words related to brain/imaging—fMRI/functional Magnetic Resonance Imaging/BOLD/blood oxygen level dependent/neuroimaging/PET/Positron Emission Computed Tomography/fNIRS/ functional near-infrared spectroscopy. Data bases including Web of Science, PubMed, PsycINFO, CNKI, and others were searched. The publication time was set from January 1975 to March 2021, and the search contents were three sets of search terms connected by “AND.” Supplemental screening was conducted for the included literature.
The downloaded literature was screened according to the inclusion criteria: (1) the coordinates of the enhancement point of the drug cue-neutral cue were reported; (2) it uses the statistics contrasts(drug cue > Neutral cue); (2) it was a whole brain study, not a specific brain area study; (3) the drug craving was induced by the cue; (4) it adopted an in-group design—the brain activation areas of drug-dependent patients under drug and neutral cues were compared; (5) research subjects were substance abusers; (6) fMRI, PET, or fNIRS was used; (7) literature review and meta-analysis were excluded; and (8) subject had no mental illness.
The final coordinates were organized into text, and GingerALE 2.3.6 was used to convert the coordinates based on Talarich template to the coordinates based on Montreal Neurological Institute (MNI) template; to be conservative, according to the recommendations of the ALE instruction manual, the threshold of the diagram of activation likelihood estimation was set to p < 0.001 and corrected by the method of Uncorrected P (33). The minimum cluster size was 250 mm3 (34), and the default preferences were set. The following meta-analysis was performed: (1) meta-analyses were performed for legal drug-related (tobacco-related and alcohol-related) and illegal drug-related literature separately; (2) a conjunction meta-analysis was performed between legal drug-related (tobacco-related and alcohol-related) literature and illegal drug-related literature, separately (see Figure 1). Each meta-analysis produced their respective activation area pictures and cluster files. Mango4.1 (http://rii.uthscsa.edu/mango/) was used to cover the activation area on the MNI standard brain (http://www.brainmap.org/ale/) (35).
Of the 49 included articles, two papers reported the correlation coefficient between an activated brain area (drug cue > neutral cue) and craving score; seven papers reported the correlation coefficients between several activated brain areas (drug cue > neutral cue) and craving score. Ultimately, we obtained a total of 26 correlation coefficients as effect sizes.
Current meta-analyses mainly use fixed-effect models or random-effect models. The fixed-effects model assumes that there is only one true effect size behind all studies in the meta-analysis, and that the difference in effect size for each study is due to sampling error. The random effects model assumes that the true effect size is different for each study and that the difference in effect size for each study is due to a combination of the difference in true effect size and sampling error (36). If the total effect sizes from the meta-analysis are not only for the included studies but need to be extended to other groups, we should use a random-effect model (36). Since the age, gender, occupation, etc. of the subjects in the meta-analysis varied, the effect sizes obtained from our meta-analysis could not be limited to just one, so we chose a random-effect model.
Publication bias means that the published research literature does not systematically and comprehensively represent the total body of research that has been done in the field (37). The most effective way to remove publication bias is to increase the sample size (including published and unpublished studies), as a lack of representative sample, particularly of dissertations with insignificant or unpublished findings, may affect the reliability of the meta-analysis results. To address this issue, firstly, we obtained as many unpublished papers as possible during the literature search stage; secondly, in the specific meta-analysis process, we used three methods including funnel plot, Rosenthal's Classic Fail-safe N-test, Egger's test to further evaluate publication bias.
CMA (comprehensive meta-analysis) is a commercial software package dedicated to meta-analysis (www.meta-analysis.com), developed by Borenstein et al. (36). It was released in 2007 with Version 2.0 and above, and is now available in Version 3.0. In our experiments, we used CMA version 2.2. The software has a user-friendly interface, is easy to operate, can import more than 100 kinds of data structures, and can implement advanced statistical analysis functions such as subgroup analysis, meta regression and cumulative meta-analysis.
Using correlation coefficient as the effect size, random effects models were used and CMA 2.2 was adopted for meta-analysis. Methods such as funnel plot, Begg's test, Egger's tests and the Trim and Fill method were used to evaluate the publication bias of this meta-analysis.
Of the 49 articles that met the inclusion criteria, one article contained two addiction groups with different lengths of detoxification, one article included two addiction groups with different drug cues and one contained three addiction groups with different addictive substances. There were altogether 53 sub-studies from the above mentioned articles included in this study, and they could be classified by addictive substance, 14 articles explored heroin; 8, alcohol; 13, tobacco; 9, cocaine; 6, marijuana; 2, methamphetamine; and 1, polydrug addiction. With consideration of cue exposure, treatment status of the participants, abstinence of the samples included, and diagnosis modulating the brain reactions to drug cues (38), we collated relevant information from the included literature (See Table 1 in the additional file).
There were 32 experiments, 687 subjects, 18 activity enhancement points, and 13 activation clusters with enhanced activity for drug data. The brain regions of drug-dependent patients with enhanced activity after induction by cues were concentrated in the amygdala, hippocampus, middle occipital gyrus, middle temporal gyrus, fusiform gyrus, cingulate gyrus, anterior central gyrus, caudate, middle frontal gyrus, thalamus, and inferior frontal gyrus.
The ALE meta-analysis on alcohol and tobacco included 21 experiments, 687 subjects, 14 activity enhancement points, and 10 activation clusters with enhanced activity. The brain regions of alcohol-dependent patients and tobacco-dependent patients with enhanced activity induced by cues were gathered in the caudate, posterior cingulate gyrus, anterior cingulate gyrus, middle frontal gyrus, thalamus, insula, superior temporal gyrus, and precuneus (see Table 2 in the additional file).
Regarding the comparative ALE meta-analysis of nicotine and drugs, five activity enhancement points and three activation clusters with enhanced activity were generated. The brain areas co-activated by the two were the posterior cingulate and caudate (see Table 3, Figure 2).
Figure 2. Co-activated clusters about alcohol, nicotine, and illegal drugs. Slices taken at X = 0; Y = −18.
First, the Heterogeneity test was performed. The Q-test result was significant (P < 0.001), indicating that the effect sizes of the original research were not similar.
Second, the publication bias of this meta-analysis was checked by a funnel plot (see Figure 3).
Figure 3. Funnel plot. The vertical axis is the log standard error of the effect size, the horizontal axis is the effect size, the inside of the funnel is the confidence interval, and the central axis is the combined effect size.
Regarding the funnel plot, the point on the left is farther from the axis of symmetry than the point on the right. This distribution characteristic indicates the possible occurrence of publication bias. Because the funnel plot is a preliminary check from a subjective point of view, we further performed Rosenthal's Failsafe N and Egger's tests to more accurately test the possibility of publication bias (see Table 4).
According to the Egger's test, the results suggest that there is no publication bias. From Rosenthal's N-value, it is necessary to include 238 (<2,200) articles to neutralize the two total effect sizes, indicating the presence of publication bias in this study.
Of the three publication bias tests described above, two results (funnel plot and Rosenthal's N) indicated the presence of publication bias and one result (Egger's test) indicated the absence of publication bias, and no results were obtained for all three tests. Therefore, further analysis is still required and the Trim and Fill method needs to be employed to examine the effect of publication bias on the results of the meta-analysis.
The Trim and Fill method proposed by Duval and Tweedie was further used to test the influence of publication bias on the results of meta-analysis (87). It was found that after trimming and filling the research literature, the overall effects obtained by using the random effects model were still significant. In addition, our unpublished literature represents 14.3%, which is already a significant proportion. Taken together, these results suggest that although there may be a slight publication bias in this study, the main findings of the meta-analysis are valid. Thus, although there may be publication bias in the two meta-analyses in this study, the main conclusion drawn from the comprehensive meta-analysis is valid.
The relationship between brain imaging data and craving scores was tested from an overall perspective. The results show that there are a total of 26 independent effect sizes, with the total subjects number of 6,663, and the overall correlation coefficient of 0.222 (see Table 5).
Findings indicate that the main co-activated brain area of tobacco-, alcohol-, and drug-related data is the posterior cingulate cortex (PCC); its voxel is far more than other co-activated brain areas. The PCC's most common identifier in the addiction field is as the self-function center of the default mode network (DMN), which is mainly responsible for the processing of “self” information such as autobiographical recall, self-evaluation, and reflection of one's own emotional state (88). In general, PCC guides attention to the internal (89), transmitting internal information for further evaluation via the ventromedial prefrontal lobe (mPFC) (90). Previous studies have found that changes in the PCC gyrus of different drug-dependent patients in craving states are often closely related to the DMN (91). In heroin-dependent patients, the PCC → mPFC pathway is activated in the process of reducing the significance of drug-related cues (92). After 24 h abstinence in alcohol-dependent patients, PCC has high synchronicity with other parts of the DMN (93). PCC damage can even lead to the disappear of drug cravings and its damage causes tobacco-dependent patients to lose interest in smoking tobacco (94). Regarding concomitant substance use, attention should be paid to cultivating patients' positive self-concept to enhance withdrawal motivation and mitigate relapses. Simultaneously, attention should be paid to the self-identity of successful abstainers to allow them to fully integrate into social groups and resume normal work and life.
The caudate is the second co-activation area. Habit formation is a cause of substance addiction and, here, the caudate produces neuronal responses (95). Using reward methods for individuals form conditioned reflexes is an effective way to form habits and the caudate and related cortical-striatal loop brain regions are crucial parts of the addiction reward loop. This suggests that the caudate may promote the formation of drug-taking habits through the activation of reward loops. Additionally, the caudate participates in the cognitive process of inhibiting control (96, 97). The dual disorders of cognitive control and craving processing can cause addiction. The activation of the caudate in drug craving is beneficial for inhibiting relapse behavior; however, it cannot effectively inhibit the spontaneous activities of DMN in heroin-dependent patients, thus it cannot perform cognitive control on some target-directed activities (e.g., seeking drugs, drug use) (98). Therefore, the caudate, a part crucial to the brain's learning and memory, accelerates the addiction process. Its control function allows it to inhibit individual relapse to a certain extent in the craving state, but abnormal changes in the caudate may explain why patients cannot control relapses or take other drugs to relieve their cravings. Treatment providers should pay more attention to cognitive control training for people who use substances concomitantly, such as high-intensity interval training, mindfulness training, and cognitive behavioral therapy.
The thalamus is the third co-activation area. As a sensory center, thalamus abnormality can cause patients to disassociate themselves from reality (99). After ketamine enters the human body, it inhibits the thalamus-neocortical system, selectively blocks pain, and activates the limbic system leading to excitement; the combination of alcohol with GABAA receptors in the thalamus makes people unresponsive as they temporarily detach from painful realities (100, 101). Here, the thalamus is also an important part of the memory system and addiction memory often causes relapse (102). The thalamus downstream loop is closely related to addiction-related memory: the PVT → CeA loop is the key neural pathway for the formation of drug addiction memory and is responsible for connecting rewards produced by opioids with the environment; the PVT → NAc → LH loop is important for maintaining addiction-related memory. Through optogenetic and other technical means, the PVT → NAc or NAc → LH pathway can be manipulated in the memory extraction stage to eliminate addiction-related memory, for preventing relapse (103). It can be seen that the thalamus is like an eraser that erases the memory of addiction. The two subregions of the thalamus are also involved in cognitive control and craving, revealing the implications of the thalamic subnucleus in the pathology of acute abstinent heroin users (104). Thus, the thalamus has become a new focus for solving drug addiction. Regarding concomitant substance abuse, the “eraser” is a new development proposed for wiping addiction-related memory from patients during detoxification.
Tobacco, alcohol, and drug-dependent patients will process self-information in a craving state. Relevant studies have shown that self-concept is related to drug craving (105). Drug users adopt negative coping mechanisms when facing social pressure or pressure caused by drug withdrawal because of their low self-concept (106). Additionally, self-concept is positively correlated with the motivation of drug withdrawal (107, 108), which is an important factor in the treatment of craving (109). Notably, the self-concept of drug use involves a drug-use identity the degree to which drug use behavior is included in the self-concept by the drug-dependent patients. The higher the level of inclusion, the higher the identity of drug use. Drug-use identity can significantly predict drug craving, as confirmed in alcohol, tobacco, and drug use (110–114). Furthermore, substance users' drug craving has a cross-cue response mode when they try to withdraw from one addictive substance, and continuous exposure to another drug may induce craving for both substances, thus increasing the possibility of treatment failure (115). Therefore, drug-dependent patients may also experience drug cravings under tobacco and alcohol cues, arousing drug-use identity and resulting in a low sense of self-identity and loss of determination to abstain from drug-use.
That said, addiction-related memory (a pathological memory formed by repeatedly associating the pleasure of drugs with the drug-use environment) is activated by patients' craving state. Like other long-term memories, addiction-related memory contains both narrative scenarios and emotional memories such as reward memories, habitual actions, and drug-use techniques that are formed during long-term drug use and belong to procedural memory (116). Therefore, tobacco and alcohol-dependent patients may activate the reward circuit in the craving state, producing conditioned reflexes and abnormal reward circuits that may cause drug abstainers to relapse (117).
Thus, both self-information processing and the arousal of addiction-related memories can trigger relapses. However, in the current social status of addiction treatment, many people mistakenly think that focusing on drug rehabilitation and ignoring tobacco and alcohol withdrawal or using them to replace drugs are effective treatments. In fact, such treatments may cause drug-dependent patients with tobacco and alcohol addiction to give up on themselves because their identity of drug use is induced by craving for tobacco and alcohol after successful drug withdrawal, and they may regard themselves as patients in their mind. At the same time, the reward memory in the addiction-related memory will induce conditioned reflexes and activate the action of drug use. Therefore, drug-dependent patients can start to abstain from tobacco and alcohol in the early stage of detoxification, so as to avoid the tragedy of “penny wise and pound foolish” at a later stage.
We found that only nine of the 51 studies reported a correlation between craving scores and activated brain regions. Therefore, this result (r = 0.222) does not fully indicate that ALE meta-analysis results can be represented by craving but it suggests to some extent that the accuracy of neuroimaging indirect measurement of craving needs to be improved. Neuroimaging provides a quantitative measurement for the evaluation of drug craving. However, these results can only show that neurophysiological changes are related to craving, and they cannot prove that there is a causal relationship between these factors. Sayette et al. (118) proposes that craving and hunger are both subjective experiences of the desire to ingest a substance, they are not necessarily related to physiological signals, and neither is necessarily related to physiological indicators that express biological needs. However, (for example, the blood sugar level in the circulation when hunger does not necessarily decrease), but both can be stimulated by environmental stimuli (such as stimulated by signals that indicate availability). A study also shows that craving and relapsing do not depend on direct physiological drug effects (119). Furthermore, the ecological validity of the cue-induced paradigm is poor, as the subject may be affected by response tendency and social expectations, which may influence the correlation between brain activation and craving scores.
There are few published studies on cravings for new drugs, and the proportion of new drugs explored in this study is low, thus further work is needed to improve the representativeness of the current status of drug dependence. Conditions that induce craving are mostly shown in pictures, so the retrieved literature is not enough to conduct a comparative meta-analysis of brain activation induced by different cues.
Future research can examine related unpublished research on new drugs, emerging conditions for induction, and different imaging conditions to supplement the literature and correct the unpublished deviations of meta-analysis. Concerning craving in drug addiction, researchers should consider current social situations and increase research efforts on new drug addiction in future studies. Additionally, scholars should actively explore experimental conditions that can better induce real psychological craving, such as the use of multi-sensory stimulation, and specific conditions for induction should be formulated based on different regions and drugs.
The co-activation areas of tobacco, alcohol, and drug-dependent patients induced by cues are mainly the PCC, followed by the caudate and thalamus. The PCC is closely related to the DMN and is the main component of the DMN self-function center; the caudate and thalamus are both related to addiction-related memory. This indicates that the three drug cravings all involve the processing of self-information and the initiation of addiction-related memories.
Because these cravings induce the processing of self-information, including self-concept, drug-dependent patients will stimulate their drug-use identity. As these drug abstainers may induce drug cravings under tobacco and alcohol cues, they may also arouse drug-use identity under these cues, thereby increasing the rate of relapse. Moreover, addiction-related memories evoked under tobacco and alcohol cues include reward memories, which can activate drug abstainers' reward circuits, produce a conditioned reflex, and cause relapse. Therefore, professionals should pay attention to tobacco and alcohol withdrawal in the early stage of drug rehabilitation.
This study found that neuroimaging only mildly represents subjective craving. Thus, researchers should not use neuroimaging results exclusively to represent subjective craving. Furthermore, the ecological validity of the environment for cue-induced craving should be increased in the laboratory to improve the present research.
HL: study concept and design. YL, JX, YQ, and QZ: literature acquisition. HH: data analysis and interpretation. DZ: manuscript preparation and editing. YL: guidance of methodology and language revision. YJ: data curation and editing of writing. All authors contributed substantially and according to Frontiers in Psychiatry guidelines to be recognized as authors. All authors have read and approved the final version of the manuscript.
This study was supported by the National Social Science Foundation of China (Grant No. 20BSH047).
The authors declare that the research was conducted in the absence of any commercial or financial relationships that could be construed as a potential conflict of interest.
All claims expressed in this article are solely those of the authors and do not necessarily represent those of their affiliated organizations, or those of the publisher, the editors and the reviewers. Any product that may be evaluated in this article, or claim that may be made by its manufacturer, is not guaranteed or endorsed by the publisher.
1. Peacock A, Leung J, Larney S, Colledge S, Hickman M, Rehm J, et al. Global statistics on alcohol, tobacco and illicit drug use: 2017 status report. Addiction. (2018) 113:1905–26. doi: 10.1111/add.14234
2. Nutt D. Equasy — an overlooked addiction with implications for the current debate on drug harms. J Psychopharmacol. (2009) 23:3–5. doi: 10.1177/0269881108099672
3. Nutt D, King LA, Saulsbury W, Blakemore C. Development of a rational scale to assess the harm of drugs of potential misuse. Lancet. (2007) 369:1047–53. doi: 10.1016/S0140-6736(07)60464-4
4. Unsworth DJ, Mathias JL. Traumatic brain injury and alcohol/substance abuse: a Bayesian meta-analysis comparing the outcomes of people with and without a history of abuse. J Clin Exp Neuropsychol. (2017) 39:547–62. doi: 10.1080/13803395.2016.1248812
5. Jia B, Lin H, Xu Y, Wang J, Ni F, Zhang S, et al. Review on the association of nicotine abuse and mental disease-related sleep disorders. Open J Nat Sci. (2015) 3:172. doi: 10.12677/OJNS.2015.34021
6. Geibprasert S, Gallucci M, Krings T. Addictive illegal drugs: structural neuroimaging. AJNR Am J Neuroradiol. (2010) 31:803–8. doi: 10.3174/ajnr.A1811
7. Fisk JE, Gallagher DT, Hadjiefthyvoulou F, Montgomery C. Temporal and visual source memory deficits among ecstasy/polydrug users. Hum Psychopharmacol. (2014) 29:172–82. doi: 10.1002/hup.2385
8. Yurtseven A, Turan C, Yuncu Z, Annette Akgur S, Saz EU. Substance use frequency and related characteristics among adolescents presenting to an emergency department in Turkey. J Ethn Subst Abuse. (2021) 20:614–24. doi: 10.1080/15332640.2019.1685048
9. Weinberger AH, Sofuoglu M. The impact of cigarette smoking on stimulant addiction. Am J Drug Alcohol Abuse. (2009) 35:12–7. doi: 10.1080/00952990802326280
10. Delgado-Lobete L, Montes-Montes R, Vila-Paz A, Cruz-Valiño JM, Gándara-Gafo B, Talavera-Valverde MÁ, et al. Individual and environmental factors associated with tobacco smoking, alcohol abuse and illegal drug consumption in university students: a mediating analysis. Int J Environ Res Public Health. (2020) 17:3019. doi: 10.3390/ijerph17093019
11. Althobaiti YS, Sari Y. Alcohol interactions with psychostimulants: an overview of animal and human studies. J Addict Res Ther. (2016) 7:281. doi: 10.4172/2155-6105.1000281
12. Barbosa-Méndez S, Salazar-Juárez A. Cocaine+ nicotine mixture enhances induction and expression of behavioral sensitization in rats. J Psychiatric Res. (2018) 100:88–98. doi: 10.1016/j.jpsychires.2018.02.024
13. Davis AK, Walton MA, Bohnert KM, Bourque C, Ilgen MA. Factors associated with alcohol consumption among medical cannabis patients with chronic pain. Addict Behav. (2018) 77:166–71. doi: 10.1016/j.addbeh.2017.10.007
14. Epstein DH, Marrone GF, Heishman SJ, Schmittner J, Preston KL. Tobacco, cocaine, and heroin: craving and use during daily life. Addict Behav. (2010) 35:318–24. doi: 10.1016/j.addbeh.2009.11.003
15. Morgan N, Daniels W, Subramaney U. An inverse relationship between alcohol and heroin use in heroin users post detoxification. Subst Abuse Rehabil. (2020) 11:1–8. doi: 10.2147/SAR.S228224
16. Thrul J, Gubner NR, Tice CL, Lisha NE, Ling PM. Young adults report increased pleasure from using e-cigarettes and smoking tobacco cigarettes when drinking alcohol. Addict Behav. (2019) 93:135–40. doi: 10.1016/j.addbeh.2019.01.011
17. Veliz PT, McCabe SE, Evans-Polce RJ, Boyd CJ. Assessing how the history of e-cigarette and cigarette use are associated with the developmental course of marijuana use in a sample of United States adolescents. Drug Alcohol Depend. (2020) 216:108308. doi: 10.1016/j.drugalcdep.2020.108308
18. Weinberger AH, Gbedemah M, Wall MM, Hasin DS, Zvolensky MJ, Goodwin RD. Cigarette use is increasing among people with illicit substance use disorders in the United States, 2002-14: emerging disparities in vulnerable populations. Addiction. (2018) 113:719–28. doi: 10.1111/add.14082
19. Kong Q, Li Y, Yue J, Wu X, Xu M. Reducing alcohol and/or cocaine-induced reward and toxicity via an epidermal stem cell-based gene delivery platform. Mol Psychiatry. (2021) 26:5266–76. doi: 10.1038/s41380-021-01043-y
20. Fultz EK, Martin DL, Hudson CN, Kippin TE, Szumlinski KK. Methamphetamine-alcohol interactions in murine models of sequential and simultaneous oral drug-taking. Drug Alcohol Depend. (2017) 177:178–86. doi: 10.1016/j.drugalcdep.2017.03.026
21. Anderson EM, Sun H, Guzman D, Taniguchi M, Cowan CW, Maze I, et al. Knockdown of the histone di-methyltransferase G9a in nucleus accumbens shell decreases cocaine self-administration, stress-induced reinstatement, and anxiety. Neuropsychopharmacology. (2019) 44:1370–6. doi: 10.1038/s41386-018-0305-4
22. Su H, Zhu L, Li J, Wang R, Liu D, Han W, et al. Regulation of microRNA-29c in the nucleus accumbens modulates methamphetamine -induced locomotor sensitization in mice. Neuropharmacology. (2019) 148:160–8. doi: 10.1016/j.neuropharm.2019.01.007
23. Zhang YX, Akumuo RC, España RA, Yan CX, Gao WJ, Li YC. The histone demethylase KDM6B in the medial prefrontal cortex epigenetically regulates cocaine reward memory. Neuropharmacology. (2018) 141:113–25. doi: 10.1016/j.neuropharm.2018.08.030
24. Tielbeek JJ, Vink JM, Polderman T, Popma A, Posthuma D, Verweij K. Genetic correlation of antisocial behaviour with alcohol, nicotine, cannabis use. Drug Alcohol Depend. (2018) 187:296–9. doi: 10.1016/j.drugalcdep.2018.03.020
25. Zhou Z, Yuan Q, Mash DC, Goldman D. Substance-specific and shared transcription and epigenetic changes in the human hippocampus chronically exposed to cocaine and alcohol. Proc Natl Acad Sci USA. (2011) 108:6626–31. doi: 10.1073/pnas.1018514108
26. Deehan GA Jr, Hauser SR, Wilden JA, Truitt WA, Rodd ZA. Elucidating the biological basis for the reinforcing actions of alcohol in the mesolimbic dopamine system: the role of active metabolites of alcohol. Front Behav Neurosci. (2013) 7:104. doi: 10.3389/fnbeh.2013.00104
27. Blaker AL, Yamamoto BK. Methamphetamine-Induced brain injury and alcohol drinking. J Neuroimmune Pharmacol. (2018) 13:53–63. doi: 10.1007/s11481-017-9764-3
28. Nimitvilai S, Arora DS, You C, McElvain M, Brodie MS. Phorbol ester reduces ethanol excitation of dopaminergic neurons of the ventral tegmental area: involvement of protein kinase C theta. Front Integr Neurosci. (2013) 7:96. doi: 10.3389/fnint.2013.00096
29. Luijten M, Veltman DJ, van den Brink W, Hester R, Field M, Smits M, et al. Neurobiological substrate of smoking-related attentional bias. Neuroimage. (2011) 54:2374–81. doi: 10.1016/j.neuroimage.2010.09.064
30. Thompson-Lake DG, Cooper KN, Mahoney JJ III, Bordnick PS, Salas R, Kosten TR, et al. Withdrawal symptoms and nicotine dependence severity predict virtual reality craving in cigarette-deprived smokers. Nicotine Tobacco Res. (2015) 17:796–802. doi: 10.1093/ntr/ntu245
31. Tanabe J, Regner M, Sakai J, Martinez D, Gowin J. Neuroimaging reward, craving, learning, and cognitive control in substance use disorders: review and implications for treatment. Br J Radiol. (2019) 92:20180942. doi: 10.1259/bjr.20180942
32. Cross SJ, Lotfipour S, Leslie FM. Mechanisms and genetic factors underlying co-use of nicotine and alcohol or other drugs of abuse. Am J Drug Alcohol Abuse. (2017) 43:171–185. doi: 10.1080/00952990.2016.1209512
33. Fox PT, Laird AR, Eickhoff SB, Lancaster JL, Fox M, Uecker AM, et al. User Manual for GingerALE 2.3. San Antonio, TX: Research Imaging Institute, UT Health Science Center San Antonio (2013).
34. Eickhoff SB, Laird AR, Grefkes C, Wang LE, Zilles K, Fox PT. Coordinate-based activation likelihood estimation meta-analysis of neuroimaging data: a random-effects approach based on empirical estimates of spatial uncertainty. Hum Brain Mapp. (2009) 30:2907–26. doi: 10.1002/hbm.20718
35. Lancaster JL, Cykowski MD, McKay DR, Kochunov PV, Fox PT, Rogers W, et al. Anatomical global spatial normalization. Neuroinformatics. (2010) 8:171–82. doi: 10.1007/s12021-010-9074-x
36. Borenstein M, Hedges L, Higgins J, Rothstein H. Introduction to Meta-Analysis. West Sussex: Wiley & Sons (2009). doi: 10.1002/9780470743386
37. Rothstein HR, Sutton AJ, Borenstein M editors. Publication Bias in Meta-Analysis: Prevention, Assessment and Adjustments. Hoboken, NJ: Wiley (2006). doi: 10.1002/0470870168
38. Jasinska AJ, Stein EA, Kaiser J, Naumer MJ, Yalachkov Y. Factors modulating neural reactivity to drug cues in addiction: a survey of human neuroimaging studies. Neurosci Biobehav Rev. (2014) 38:1–16. doi: 10.1016/j.neubiorev.2013.10.013
39. *Wang AL, Elman I, Lowen SB, Blady SJ, Lynch KG, Hyatt JM, et al. Neural correlates of adherence to extended-release naltrexone pharmacotherapy in heroin dependence. Transl Psychiatry. (2015) 5:e531. doi: 10.1038/tp.2015.20
40. *Tabatabaei-Jafari H, Ekhtiari H, Ganjgahi H, Hassani-Abharian P, Oghabian MA, Moradi A, et al. Patterns of brain activation during craving in heroin dependents successfully treated by methadone maintenance and abstinence-based treatments. J Addict Med. (2014) 8:123–9. doi: 10.1097/ADM.0000000000000022
41. *Li Q, Wang Y, Li W, Yang W, Zhu J, Zheng Y, et al. FMRI study of drug cues in heroin abusers with long-term forced withdrawal. J Clin Radiol. (2013) 32:313–6.
42. *Chang H. fMRI Study on Heroin-Related Cues Induced Craving in Heroin Addicts Long After Nucleus Accumbens Ablation. Air Force Medical University (2012). Available online at: https://kns.cnki.net/KCMS/detail/detail.aspx?dbname=CMFD201301&filename=1012039156.nh
43. *Qiang L, Wang Y, Wei L. fMRI study on craving and brain response to heroin related clues in heroin d. J Pract Radiol. (2012) 28:1–5. doi: 10.3969/j.issn.1002-1671.2012.01.001
44. *Lou M, Wang E, Shen Y, Wang J. Cue-elicited craving in heroin addicts at different abstinent time: an fMRI pilot study. Subst Use Misuse. (2012) 47:631–9. doi: 10.3109/10826084.2011.646381
45. *Wang Y, Yang L, Li Q, Yang W, Du P, Wang W. Heroin cue-induced craving and its relationship with nucleus accumbens activation: a functional MRI study. Chin J Radiol. (2010) 2:137–41.
46. *Song XG, Li CF, Hu L, Ma N, Wang J, Li N, et al. Effect of acupuncture on heroin cue-induced functional magnetic resonance images in heroin-addicted human subjects. Acupunct Res. (2011) 36:121–7.
47. *Yang L. Changes of Brain 1H-MRS Clues-Induced Craving fMRI in Heroin Addicts. Air Force Medical University (2009). Available online at: https://kns.cnki.net/KCMS/detail/detail.aspx?dbname=CMFD2009&filename=2009145655.nh
48. *Zijlstra F, Veltman DJ, Booij J, van den Brink W, Franken IH. Neurobiological substrates of cue-elicited craving and anhedonia in recently abstinent opioid-dependent males. Drug Alcohol Depend. (2009) 99:183–92. doi: 10.1016/j.drugalcdep.2008.07.012
49. *Shao C, Jiang K, Li Y, Zhao M, Sun H, Xu Y, et al. A functional magnetic resonance imaging study of cue-elicited heroin craving. Chin J Psychiatry. (2005) 2:65–8. Available online at: https://kns.cnki.net/kcms/detail/detail.aspx?FileName=ZHMA200502000&DbName=CJFQ2005
50. *Xiao Z, Lee T, Zhang JX, Wu Q, Wu R, Weng X, et al. Thirsty heroin addicts show different fMRI activations when exposed to water-related and drug-related cues. Drug Alcohol Depend. (2006) 83:157–62. doi: 10.1016/j.drugalcdep.2005.11.012
51. *Sun H, Feng X, He H, Liu H, Yao Z, Tang W, et al. Functional magnetic resonance study on cue induced craving in heroin addicts during withdrawal. Chin J Med Comp Imaging. (2004) 6:366–70.
52. *Zhang S, Zhornitsky S, Angarita GA, Li CR. Hypothalamic response to cocaine cues and cocaine addiction severity. Addict Biol. (2020) 25:e12682. doi: 10.1111/adb.12682
53. *Ma L, Steinberg JL, Cunningham KA, Bjork JM, Lane SD, Schmitz JM, et al. Altered anterior cingulate cortex to hippocampus effective connectivity in response to drug cues in men with cocaine use disorder. Psychiatry Res Neuroimaging. (2018) 271:59–66. doi: 10.1016/j.pscychresns.2017.10.012
54. *Prisciandaro JJ, Myrick H, Henderson S, McRae-Clark AL, Santa Ana EJ, Saladin ME, et al. Impact of DCS-facilitated cue exposure therapy on brain activation to cocaine cues in cocaine dependence. Drug Alcohol Depend. (2013) 132:195–201. doi: 10.1016/j.drugalcdep.2013.02.009
55. Volkow ND, Tomasi D, Wang GJ, Fowler JS, Telang F, Goldstein RZ, et al. Reduced metabolism in brain “control networks” following cocaine-cues exposure in female cocaine abusers. PLoS ONE. (2011) 6:e16573. doi: 10.1371/journal.pone.0016573
56. Kilts CD, Gross RE, Ely TD, Drexler KP. The neural correlates of cue-induced craving in cocaine-dependent women. Am J Psychiatry. (2004) 161:233–41. doi: 10.1176/appi.ajp.161.2.233
57. *Bonson KR, Grant SJ, Contoreggi CS, Links JM, Metcalfe J, Weyl HL, et al. Neural systems and cue-induced cocaine craving. Neuropsychopharmacology. (2002) 26:376–86. doi: 10.1016/S0893-133X(01)00371-2
58. *Kilts CD, Schweitzer JB, Quinn CK, Gross RE, Faber TL, Muhammad F, et al. Neural activity related to drug craving in cocaine addiction. Arch Gen Psychiatry. (2001) 58:334–41. doi: 10.1001/archpsyc.58.4.334
59. *Sell LA, Morris JS, Bearn J, Frackowiak RS, Friston KJ, Dolan RJ. Neural responses associated with cue evoked emotional states and heroin in opiate addicts. Drug Alcohol Depend. (2000) 60:207–16. doi: 10.1016/S0376-8716(99)00158-1
60. *Garavan H, Pankiewicz J, Bloom A, Cho JK, Sperry L, Ross TJ, et al. Cue-induced cocaine craving: neuroanatomical specificity for drug users and drug stimuli. Am J Psychiatry. (2000) 157:1789–98. doi: 10.1176/appi.ajp.157.11.1789
61. *Zhou X, Zimmermann K, Xin F, Zhao W, Derckx RT, Sassmannshausen A, et al. Cue reactivity in the ventral striatum characterizes heavy cannabis use, whereas reactivity in the dorsal striatum mediates dependent use. Biol Psychiatry Cogn Neurosci Neuroimaging. (2019) 4:751–62. doi: 10.1016/j.bpsc.2019.04.006
62. *Karoly HC, Schacht JP, Meredith LR, Jacobus J, Tapert SF, Gray KM, et al. Investigating a novel fMRI cannabis cue reactivity task in youth. Addict Behav. (2019) 89:20–8. doi: 10.1016/j.addbeh.2018.09.015
63. *Charboneau EJ, Dietrich MS, Park S, Cao A, Watkins TJ, Blackford JU, et al. Cannabis cue-induced brain activation correlates with drug craving in limbic and visual salience regions: preliminary results. Psychiatry Res. (2013) 214:122–31. doi: 10.1016/j.pscychresns.2013.06.005
64. *Cousijn J, Goudriaan AE, Ridderinkhof KR, van den Brink W, Veltman DJ, Wiers RW. Neural responses associated with cue-reactivity in frequent cannabis users. Addict Biol. (2013) 18:570–80. doi: 10.1111/j.1369-1600.2011.00417.x
65. *Ray S, Hanson C, Hanson SJ, Bates ME. fMRI BOLD response in high-risk college students (Part 1): during exposure to alcohol, marijuana, polydrug and emotional picture cues. Alcohol Alcohol. (2010) 45:437–43. doi: 10.1093/alcalc/agq042
66. *Filbey FM, Schacht JP, Myers US, Chavez RS, Hutchison KE. Marijuana craving in the brain. Proc Natl Acad Sci USA. (2009) 106:13016–21. doi: 10.1073/pnas.0903863106
67. Guterstam J, Jayaram-Lindström N, Berrebi J, Petrovic P, Ingvar M, Fransson P, et al. Cue reactivity and opioid blockade in amphetamine dependence: a randomized, controlled fMRI study. Drug Alcohol Depend. (2018) 191:91–7. doi: 10.1016/j.drugalcdep.2018.06.023
68. Grodin EN, Courtney KE, Ray LA. Drug-induced craving for methamphetamine is associated with neural methamphetamine Cue reactivity. J Stud Alcohol Drugs. (2019) 80:245–51. doi: 10.15288/jsad.2019.80.245
69. *Bi Y. Effects of Smoking Internet Addiction on Structural Functional Connectivity of Brain. Xidian University (2018). Available online at: https://kns.cnki.net/KCMS/detail/detail.aspx?dbname=CDFDLAST2018&filename=1018046126.nh
70. *Zhao H. The Relationship Between Craving Response Inhibition in Smokers—a Functional MRI Study. Southwest University (2017). Available online at: https://kns.cnki.net/kcms/detail/detail.aspx?FileName=1017845775.nh&DbName=CMFD2018
71. Yang L, Wang S, Su B, Li S, Cao H. The influencing mechanism of reward on executive function in heroin addict. Prog Psychol Sci. (2017) 25:1888–97. doi: 10.3724/SP.J.1042.2017.01888
72. Do KT, Galván A. Neural sensitivity to smoking stimuli is associated with cigarette craving in adolescent smokers. J Adolesc Health. (2016) 58:186–94. doi: 10.1016/j.jadohealth.2015.10.004
73. Ko CH, Liu GC, Yen JY, Yen CF, Chen CS, Lin WC. The brain activations for both cue-induced gaming urge and smoking craving among subjects comorbid with Internet gaming addiction and nicotine dependence. J Psychiatric Res. (2013) 47:486–93. doi: 10.1016/j.jpsychires.2012.11.008
74. *Wilson SJ, Sayette MA, Fiez JA. Neural correlates of self-focused and other-focused strategies for coping with cigarette cue exposure. Psychol Addict Behav. (2013) 27:466–76. doi: 10.1037/a0027055
75. *Hartwell KJ, Johnson KA, Li X, Myrick H, LeMatty T, George MS, et al. Neural correlates of craving and resisting craving for tobacco in nicotine dependent smokers. Addict Biol. (2011) 16:654–66. doi: 10.1111/j.1369-1600.2011.00340.x
76. *Goudriaan AE, Veltman DJ, van den Brink W, Dom G, Schmaal L. Neurophysiological effects of modafinil on cue-exposure in cocaine dependence: a randomized placebo-controlled cross-over study using pharmacological fMRI. Addict Behav. (2013) 38:1509–17. doi: 10.1016/j.addbeh.2012.04.006
77. *Weinstein A, Greif J, Yemini Z, Lerman H, Weizman A, Even-Sapir E. Attenuation of cue-induced smoking urges and brain reward activity in smokers treated successfully with bupropion. J Psychopharmacol. (2010) 24:829–38. doi: 10.1177/0269881109105456
78. *McClernon FJ, Hiott FB, Liu J, Salley AN, Behm FM, Rose JE. Selectively reduced responses to smoking cues in amygdala following extinction-based smoking cessation: results of a preliminary functional magnetic resonance imaging study. Addict Biol. (2007) 12:503–12. doi: 10.1111/j.1369-1600.2007.00075.x
79. *McBride D, Barrett SP, Kelly JT, Aw A, Dagher A. Effects of expectancy and abstinence on the neural response to smoking cues in cigarette smokers: an fMRI study. Neuropsychopharmacology. (2006) 31:2728–38. doi: 10.1038/sj.npp.1301075
80. *Bach P, Schuster R, Koopmann A, Vollstaedt-Klein S, Spanagel R, Kiefer F. Plasma calcium concentration during detoxification predicts neural cue-reactivity and craving during early abstinence in alcohol-dependent patients. Euro Arch Psychiatry Clin Neurosci. (2021) 272:341–8. doi: 10.1007/s00406-021-01240-4
81. *Ray LA, Courtney KE, Hutchison KE, MacKillop J, Galvan A, Ghahremani DG. Initial evidence that OPRM1 genotype moderates ventral and dorsal striatum functional connectivity during alcohol cues. Alcohol Clin Exp Res. (2014) 38:78–89. doi: 10.1111/acer.12136
82. Kreusch F, Goffaux V, Siep N, Houben K, Quertemont E, Wiers RW. Brain activation associated with automatic processing of alcohol-related cues in young heavy drinkers and its modulation by alcohol administration. Alcohol Clin Exp Res. (2015) 39:1957–66. doi: 10.1111/acer.12835
83. *Vollstädt-Klein S, Hermann D, Rabinstein J, Wichert S, Klein O, Ende G, et al. Increased activation of the ACC during a spatial working memory task in alcohol-dependence versus heavy social drinking. Alcohol Clin Exp Res. (2010) 34:771–6. doi: 10.1111/j.1530-0277.2010.01149.x
84. *Vollstädt-Klein S, Loeber S, Richter A, Kirsch M, Bach P, von der Goltz C, et al. Validating incentive salience with functional magnetic resonance imaging: association between mesolimbic cue reactivity and attentional bias in alcohol-dependent patients. Addict Biol. (2012) 17:807–16. doi: 10.1111/j.1369-1600.2011.00352.x
85. *Park MS, Sohn JH, Suk JA, Kim SH, Sohn S, Sparacio R. Brain substrates of craving to alcohol cues in subjects with alcohol use disorder. Alcohol Alcohol. (2007) 42:417–22. doi: 10.1093/alcalc/agl117
86. *Myrick H, Anton RF, Li X, Henderson S, Drobes D, Voronin K, et al. Differential brain activity in alcoholics and social drinkers to alcohol cues: relationship to craving. Neuropsychopharmacology. (2004) 29:393–402. doi: 10.1038/sj.npp.1300295
87. Duval S, Tweedie R. Trim and fill: a simple funnel-plot-based method of testing and adjusting for publication bias in meta-analysis. Biometrics. (2000) 56:455–63. doi: 10.1111/j.0006-341X.2000.00455.x
88. Craik FIM, Moroz TM, Moscovitch M, Stuss DT, Winocur G, Tulving E, et al. In search of the self: a positron emission tomography study. Psychol Sci. (1999) 10:26–34. doi: 10.1111/1467-9280.00102
89. Leach R, Sharp DJ. The role of the posterior cingulate cortex in cognition and disease. Brain. (2014) 137 (Pt. 1):12–32. doi: 10.1093/brain/awt162
90. Small DM, Gitelman DR, Gregory MD, Nobre AC, Parrish TB, Mesulam MM. The posterior cingulate and medial prefrontal cortex mediate the anticipatory allocation of spatial attention. Neuroimage. (2003) 18:633–41. doi: 10.1016/S1053-8119(02)00012-5
91. Zhang R, Volkow ND. Brain default-mode network dysfunction in addiction. Neuroimage. (2019) 200:313–31. doi: 10.1016/j.neuroimage.2019.06.036
92. Wei X, Chen J, Zhu J, Li Q, Li W, Wang W. Functional connectivity of posterior cingulate gyrus in heroin dependents treated by methadone maintenance and protracted abstinence measures: an event-related fMRI study. Brain Imaging Behav. (2021) 15:2472–0. doi: 10.1007/s11682-020-00447-8
93. Zhu X, Cortes CR, Mathur K, Tomasi D, Momenan R. Model-free functional connectivity and impulsivity correlates of alcohol dependence: a resting-state study. Addict Biol. (2017) 22:206–17. doi: 10.1111/adb.12272
94. Jarraya B, Brugières P, Tani N, Hodel J, Grandjacques B, Fénelon G, et al. Disruption of cigarette smoking addiction after posterior cingulate damage. J Neurosurg. (2010) 113:1219–21. doi: 10.3171/2010.6.JNS10346
95. Sjoerds Z, de Wit S, van den Brink W, Robbins TW, Beekman AT, Penninx BW, et al. Behavioral and neuroimaging evidence for overreliance on habit learning in alcohol-dependent patients. Transl Psychiatry. (2013) 3:e337. doi: 10.1038/tp.2013.107
96. Diamond A. Executive functions. Annu Rev Psychol. (2013) 64:135–68. doi: 10.1146/annurev-psych-113011-143750
97. Malenka RC, Nestler EJ, Hyman SE. Chapter 13: higher cognitive function and behavioral control. In: Sydor A, Brown RY. Molecular Neuropharmacology: A Foundation for Clinical Neuroscience. 2nd edn. New York, NY: McGraw-Hill Medical (2009). p. 313–21.
98. Kelly C, de Zubicaray G, Di Martino A, Copland DA, Reiss PT, Klein DF, et al. L-dopa modulates functional connectivity in striatal cognitive and motor networks: a double-blind placebo-controlled study. J Neurosci. (2009) 29:7364–78. doi: 10.1523/JNEUROSCI.0810-09.2009
99. McCormick DA, Bal T. Sensory gating mechanisms of the thalamus. Curr Opin Neurobiol. (1994) 4:550–6. doi: 10.1016/0959-4388(94)90056-6
100. Sprenger T, Valet M, Woltmann R, Zimmer C, Freynhagen R, Kochs EF, et al. (2006). Imaging pain modulation by subanesthetic S-(+)-ketamine. Anesth. Analg. (2006) 103:729–37. doi: 10.1213/01.ane.0000231635.14872.40
101. Rogers R, Wise RG, Painter DJ, Longe SE, Tracey I. An investigation to dissociate the analgesic and anesthetic properties of ketamine using functional magnetic resonance imaging. Anesthesiology. (2004) 100:292–301. doi: 10.1097/00000542-200402000-00018
102. Van der Werf YD, Scheltens P, Lindeboom J, Witter MP, Uylings HB, Jolles J. Deficits of memory, executive functioning and attention following infarction in the thalamus; a study of 22 cases with localised lesions. Neuropsychologia. (2003) 41:1330–44. doi: 10.1016/S0028-3932(03)00059-9
103. Keyes PC, Adams EL, Chen Z, Bi L, Nachtrab G, Wang VJ, et al. Orchestrating opiate-associated memories in thalamic circuits. Neuron. (2020) 107:1113–23.e4. doi: 10.1016/j.neuron.2020.06.028
104. Zhang M, Liu S, Wang S, Xu Y, Chen L, Shao Z, et al. Reduced thalamic resting-state functional connectivity and impaired cognition in acute abstinent heroin users. Hum Brain Mapp. (2021) 42:2077–88. doi: 10.1002/hbm.25346
105. Barta WD, Kurth ME, Stein MD, Tennen H, Kiene SM. Craving and self-efficacy in the first five weeks of methadone maintenance therapy: a daily process study. J Stud Alcohol Drugs. (2009) 70:735–40. doi: 10.15288/jsad.2009.70.735
106. Wong DFK, Zhuang XY, Ng TK. Effectiveness of a culturally-attuned integrated cognitive behavioural therapy for Chinese young people with drug abuse in Hong Kong. Children Youth Services Rev. (2020) 113:104970. doi: 10.1016/j.childyouth.2020.104970
107. Downey L, Rosengren DB, Donovan DM. To thine own self be true: self-concept and motivation for abstinence among substance abusers. Addict Behav. (2000) 25:743–57. doi: 10.1016/S0306-4603(00)00091-5
108. Lu FY, Wen S, Deng G, Tang YL. Self-concept mediate the relationship between childhood maltreatment and abstinence motivation as well as self-efficacy among drug addicts. Addict Behav. (2017) 68:52–8. doi: 10.1016/j.addbeh.2017.01.017
109. Gregoire TK, Burke AC. The relationship of legal coercion to readiness to change among adults with alcohol and other drug problems. J Subst Abuse Treat. (2004) 26:337–43. doi: 10.1016/S0740-5472(03)00155-7
110. Brener L, Von Hippel W, Von Hippel C. Exploring the relationship between implicit self-representation and drug use. Addict Res Theory. (2012) 20:133–7. doi: 10.3109/16066359.2011.580067
111. Foster DW. Drinking identity as a mediator of the relationship between drinking motives and weekly alcohol consumption among heavy drinking undergraduate students. Addict Behav. (2014) 39:1811–5. doi: 10.1016/j.addbeh.2014.07.013
112. Hertel AW, Mermelstein RJ. Smoker identity development among adolescents who smoke. Psychol Addict Behav. (2016) 30:475–83. doi: 10.1037/adb0000171
113. Lindgren KP, Neighbors C, Teachman BA, Gasser ML, Kaysen D, Norris J, et al. Habit doesn't make the predictions stronger: implicit alcohol associations and habitualness predict drinking uniquely. Addict Behav. (2015) 45:139–45. doi: 10.1016/j.addbeh.2015.01.003
114. Vangeli E, West R. Transition towards a 'non-smoker' identity following smoking cessation: an interpretative phenomenological analysis. Br J Health Psychol. (2012) 17:171–84. doi: 10.1111/j.2044-8287.2011.02031.x
115. Drobes DJ. Cue reactivity in alcohol and tobacco dependence. Alcohol Clin Exp Res. (2002) 26:1928–9. doi: 10.1111/j.1530-0277.2002.tb02506.x
116. Zhu L, Lu C, Wang S, Zeng H. Addictive memory fading based on the association of “related cue-automated medication behavior. Adv Psychol Sci. (2017) 7:1139–44. doi: 10.3724/SP.J.1042.2017.01139
117. *Yang LZ, Shi B, Li H, Zhang W, Liu Y, Wang H, et al. Electrical stimulation reduces smokers' craving by modulating the coupling between dorsal lateral prefrontal cortex and parahippocampal gyrus. Soc Cogn Affect Neurosci. (2017) 12:1296–302. doi: 10.1093/scan/nsx055
118. Sayette MA, Shiffman S, Tiffany ST, Niaura RS, Martin CS, Shadel WG. The measurement of drug craving. Addiction. (2000) 95 (Suppl. 2):S189–210. doi: 10.1080/09652140050111762
119. Thompson BM, Bevins RA, Murray JE. Interoceptive stimulus effects of drugs of abuse. In: Neural Mechanisms of Addiction. Academic Press (2019). p. 89–101. doi: 10.1016/B978-0-12-812202-0.00007-5
*^represents included literature.
Keywords: neuroimaging, cue-reactivity, tobacco, alcohol, drug
Citation: Li H, Zhao D, Liu Y, Xv J, Huang H, Jin Y, Lu Y, Qi Y and Zhou Q (2022) Are There Neural Overlaps of Reactivity to Illegal Drugs, Tobacco, and Alcohol Cues? With Evidence From ALE and CMA. Front. Psychiatry 13:779239. doi: 10.3389/fpsyt.2022.779239
Received: 18 September 2021; Accepted: 07 March 2022;
Published: 06 April 2022.
Edited by:
Saeed Ahmed, Rutland Regional Medical Center, United StatesReviewed by:
Meenal Pathak, Penn State Milton S. Hershey Medical Center, United StatesCopyright © 2022 Li, Zhao, Liu, Xv, Huang, Jin, Lu, Qi and Zhou. This is an open-access article distributed under the terms of the Creative Commons Attribution License (CC BY). The use, distribution or reproduction in other forums is permitted, provided the original author(s) and the copyright owner(s) are credited and that the original publication in this journal is cited, in accordance with accepted academic practice. No use, distribution or reproduction is permitted which does not comply with these terms.
*Correspondence: Yiying Lu, bHV5eUBsaW5nbmFuLmVkdS5jbg==; YuanYuan Qi, eXVhbnl1YW5fcWltZ3NAMTYzLmNvbQ==; Qiang Zhou, enFAd211LmVkdS5jbg==; orcid.org/0000-0002-3045-0198
Disclaimer: All claims expressed in this article are solely those of the authors and do not necessarily represent those of their affiliated organizations, or those of the publisher, the editors and the reviewers. Any product that may be evaluated in this article or claim that may be made by its manufacturer is not guaranteed or endorsed by the publisher.
Research integrity at Frontiers
Learn more about the work of our research integrity team to safeguard the quality of each article we publish.