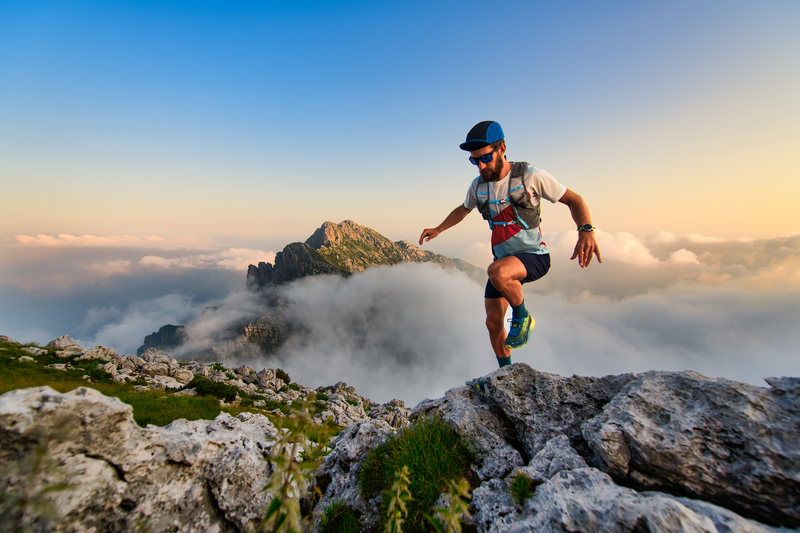
94% of researchers rate our articles as excellent or good
Learn more about the work of our research integrity team to safeguard the quality of each article we publish.
Find out more
SYSTEMATIC REVIEW article
Front. Psychiatry , 22 December 2022
Sec. Public Mental Health
Volume 13 - 2022 | https://doi.org/10.3389/fpsyt.2022.1058572
Background: The impact of screen time on mental health, including depression, has attracted increasing attention from not only children and adolescents but also the elderly. Thus, we conducted a meta-analysis of cohort studies to evaluate the association between screen time and depression risk.
Methods: The PubMed, Embase, Web of Science, and China National Knowledge Infrastructure databases were searched for cohort studies up to May 2022, and the reference lists of the included studies were also retrieved. A random-effect model was used to estimate the combined effect size. Heterogeneity was assessed with the I2 statistic. Potential publication bias was evaluated using a funnel plot and Begg’s and Egger’s tests.
Results: The final analysis included 18 cohort studies with a combined total of 241,398 participants. The pooled risk ratio (RR) was 1.10 (95% confidence interval: 1.05–1.14), with significant heterogeneity (I2 = 82.7%, P < 0.001). The results of subgroup analyses showed that the pooled RRs varied according to geographic locations, gender, age group, screen time in the control group, depression at the baseline, and whether the study was conducted during the COVID-19 pandemic. No obvious evidence of publication bias was found.
Conclusion: This study indicates that screen time is a predictor of depressive symptoms. The effects of screen time on depression risk may vary based on the participant’s age, gender, location, and screen time duration. The findings could have important implications for the prevention of depression.
Depression is a common and disabling psychiatric condition worldwide (1). According to estimates of the Global Burden of Diseases (GBD), injuries, risk factors study, depressive disorders accounted for 170.8 million cases in 1990 and 279.6 million cases in 2019, representing a 63.7% increase in prevalence (2). Depressive etiology is complex and results from interactions between biological vulnerabilities and environmental factors (3). According to the GBD (2), depression has been among the three top causes of non-fatal health losses for the past 30 years. Furthermore, research shows that depression is expected to be the leading global cause of disability-adjusted life year by 2030 (4). Depressive and anxiety disorders account for between one-quarter and one-third of all primary healthcare visits worldwide, and the World Health Organization (WHO) has estimated that neuropsychiatric disorders account for 1.2 million deaths annually, not including suicides (5). Moreover, social crises such as the coronavirus disease 2019 (COVID-19) pandemic can also lead to an epidemic of depression. WHO estimated that the cases of the major depressive disorder increased by 53.2 million (27.6%) globally because of the COVID-19 pandemic in 2020 (6). As one of the most widespread diseases affecting human physical and mental health, depression is receiving increasing attention.
With the popularization of modern information and communication technology, time spent using screens on devices, such as mobile phones, laptops, tablets, computers, and televisions, is becoming a core component of daily life (7), online studying, or work. People’s use of display screen equipment (DSE) during their free time has substantially increased in recent years (8, 9). With the increased screen time, both sedentary behavior and exposure to radiofrequency electromagnetic fields (RF-EMF) increase (10). Poitras et al. (11) and Carson et al. (12) indicated that screen time is associated with unfavorable health outcomes, and Canada, Australia, and USA have developed the “Sedentary Behavior Guidelines” to provide guidance and motivate people to reduce their screen time (13–15). Several studies have indicated that increased screen time duration could be associated with lagged development (16), psychosocial symptoms (10, 17), obesity (18, 19), sleep disorders (20, 21), and cardiovascular disease (22, 23). Thus, active or passive screen use has become a common issue among both adolescents and adults.
The relationship between screen time and depression remains controversial, and the biological mechanisms underlying this possible association are unclear. Some studies have reported an independent and interactive relationship between screen time and physical activity (21, 23–26). Tremblay et al. (27) suggested that, independent of physical activity levels, screen time-based sedentary behaviors were associated with an increased risk of various physiological and psychological problems. Furthermore, previous studies indicated that RF-EMF exposure may increase the risk of headaches, fatigue, sleep problems, chronic tinnitus, and depression (10, 28). Liu et al. (7) and Wang et al. (29) conducted meta-analyses in 2015 and 2019, respectively, to investigate the relationship between screen time-based sedentary behavior and depression. Although screen time is the time spent using devices with display screens (30), some studies have combined DSE use with other behaviors requiring low energy expenditure, such as sitting, driving, and reading, when defining the total duration of sedentary behavior, which should also be given attention. Considering the influence of RF-EMF on psychological problems, we summarized the available evidence and performed a meta-analysis of longitudinal studies to investigate the effects of screen time on depression risk. To achieve our goal, we applied the patients, intervention, comparison, and outcome (PICO) framework as follows: human being (P), time spent on screen-based equipment (I), no or little time spent on screen-based equipment (C), and increased depression prevalence (O).
This meta-analysis was conducted following the checklist of the Meta-analysis of Observational Studies in Epidemiology (MOOSE) guidelines (31) and the Preferred Reporting Items for Systematic Review and Meta-analysis (PRISMA) statement (Supplementary File 1) (32). We systematically searched the PubMed, Embase, Web of Science, and China National Knowledge Infrastructure (CNKI) databases from their inception to May 2022 for studies describing an association between screen time and depression risk regardless of language or publication status. We used the following keywords: “screen time,” “video game,” “computer use,” “watching television,” “television view,” “internet use,” “electronic game,” “smartphone use,” “tablets,” or “iPads” in combination with “depression” or “depressive symptom” as search terms. In addition, all listed references were reviewed.
Studies were included if they fulfilled the following criteria: (1) the exposure of interest was screen time, (2) the outcome of interest was depression, (3) the study design was longitudinal, and (4) provided risk estimates such as hazard ratios (HR), relative risks (RR), or odds ratios (OR) with corresponding 95% confidence intervals (CIs) or sufficient data to calculate them. Studies were excluded if they were as follows: (1) publications that were not full reports, (2) duplicate studies, (3) studies on screen time addiction, (4) studies with inadequate information to calculate risk estimates, or (5) studies reporting time spent on other behaviors as well, causing difficulty in separating only screen time. Two reviewers (QZ and DY) independently reviewed all identified studies by title and abstract or full text. Disagreements were resolved through consultation with a third reviewer (CW).
The following information was extracted for each included study: first author’s name, publication year, study source, country, follow-up years, participants’ age range or mean age at the baseline, participants’ gender, sample size, screen equipment, depression measurement, depression definition, depression at baseline, study period, source of participates, screen time measurement, screen time in exposure group, screen time in the control group, depression at the baseline or adjusted, adjusted covariates, and effect estimates with their corresponding 95% CIs. Data extraction was conducted independently by two authors (JZG and QZ). Interobserver agreement was assessed using Cohen’s kappa (κ), and any disagreements were resolved by discussion with a third author (ZL).
The methodological quality of the included studies was independently assessed by two reviewers (LL and GZ) using the Newcastle–Ottawa Scale (NOS) (33), which assesses the quality of cohort studies. The NOS includes eight items grouped into categories of selection, comparability, and outcome. Each study is assigned a score ranging between 0 and 9, and NOS scores over six indicate relatively high quality, five and six indicate medium quality, and less than five indicate low quality.
The RR was considered the common measure of the association between screen time and depression. The ORs were transformed into RRs using the formula where P0 indicates the incidence of the outcome of interest in the non-exposed group (34) and then synthesized with the RRs and the HRs into pooled RRs. The multivariable-adjusted RRs were preferentially pooled when such estimates were reported. If no adjusted analysis was available, the unadjusted estimate would be pooled. A fixed-effect model was applied when heterogeneity was not detected; otherwise, a random-effect model was used to summarize RRs for the association between screen time and depression. For further assessment of the association between screen time and depression risk, a subgroup analysis was conducted to explore sources of potential heterogeneity and examine the robustness of the primary results. This difference among subgroups was tested using meta-regression analysis (STATA “metareg” command). In sensitive analysis, we conducted a leave-one-out analysis to observe the magnitude of influence on the pooled RR of each study. Statistical heterogeneity among studies was evaluated using I2 statistics where values of 25, 50, and 75% represented the cutoff points for low, moderate, and high degrees of heterogeneity, respectively. Potential publication bias was evaluated using a funnel plot and Begg’s and Egger’s tests.
Figure 1 shows all steps and reasons for study exclusion. The process of screening studies on databases retrieved 7,329 studies in total, of which 3,558 were from the Web of Science, 2,102 were from PubMed, 894 were from Embase, and 775 were from the CNKI. After eliminating reviews and duplicate publications, 2,924 articles were excluded. After screening studies by titles and abstracts, 51 remained. At the full-text review stage, 28 articles were excluded for having a cross-sectional design. Of the remaining 23 longitudinal studies, five provide insufficient data for calculating risk estimates. Ultimately, 18 studies were included in the meta-analysis.
Table 1 and Supplementary File 2 show the characteristics of the 18 included studies (35–52). Overall, the studies included 241,398 individuals, with sample sizes ranging from 435 (51) to 100,517 (49). All included studies were observational studies. Half were conducted in Europe (35, 40, 44, 45, 47, 49–52), while five (38, 41, 42, 46, 48) and four (36, 37, 39, 43) were in Asia and North America, respectively. Two studies (43, 46) only included female participants, while four (36, 44, 45, 47) reported the results for male and female participants, respectively. Most of the included studies had been published since 2010, with only one study published in 2009 (41). The follow-up durations ranged from 2 (48, 52) to 144 months (51). Only one study (46) used a physician’s diagnosis or medication history as the assessment tool for depression, while the others used self-report questionnaires or scales. Eleven studies (35–38, 41, 44–46, 49–51) excluded baseline cases of depression, while seven (39, 40, 42, 43, 47, 48, 52) studies did not. In all included studies, screen time duration was based on self-report. Table 2 shows the results of the quality assessment. Based on NOS scores, 14 studies (35–39, 41–46, 49–51) were of moderate or high quality, and four (40, 47, 48, 52) were of weak quality.
Figure 2 shows the pooled results from the random-effect model. Among these studies, 12 reported a positive relationship between screen time and depression risk. The pooled RR was 1.10 (95% CI: 1.05–1.14). The results showed a positive association between screen time and depression risk with a high level of heterogeneity (I2 = 82.7%, P < 0.001).
Subgroup analyses were conducted by study location, age, gender, sample size, study quality, depression at baseline, depression definition, follow-up time, screen equipment, whether during the COVID-19 pandemic, screen time in the control group, and physical activity controlled for or absent in the models (Table 3). When stratified by gender, the results showed a positive association between screen time and depression in female participants (RR = 1.15, 95% CI: 1.01–1.31, I2 = 76.2%); however, this association was not statistically significant in male participants (RR = 1.12, 95% CI: 0.89–1.42, I2 = 77.4%). Screen time was significantly associated with an increased risk of depression in participants who watched television (RR = 1.13, 95% CI: 1.06–1.21, I2 = 65.0%) and used mobile phones (RR = 1.13, 95% CI: 1.01–1.25, I2 = 57.7%). A significant association between screen time and increased depression risk was observed among participants from Asia (RR = 1.24, 95% CI: 1.07–1.43, I2 = 21.7%). The pooled results of studies that excluded baseline depression in their analyses suggested a significantly positive association between screen time and depression risk (RR = 1.11, 95% CI: 1.06–1.16, I2 = 66.0%).
Sensitivity analysis was adopted to identify the potential sources of heterogeneity in the association between screen time and depression risk. This helped us to examine the influence of various exclusions on the combined RRs and test the stability of the quantitative synthesis results. The pooled RRs ranged from 1.09 (95% CI: 1.05–1.14) to 1.11 (95% CI: 1.06–1.16) when one study was omitted. The leave-one-out analysis indicated that none of the individual studies significantly influenced the overall results.
Visual inspection of the funnel plot did not reveal any significant asymmetry (Figure 3). Egger’s and Begg’s tests showed no obvious publication bias across studies (Egger’s test t = 1.10, P = 0.272; Begg’s test z = 1.08, P = 0.296).
We conducted a meta-analysis of 18 longitudinal studies to investigate the association between screen time and depression risk. The pooled results showed that screen time was associated with an increased risk of depression with an RR value of 1.10 (95% CI: 1.05–1.14; I2 = 82.7%).
All included studies were observational, with few conducting experimental validations, and the research value of observational studies will be affected if they are not rigorously designed. Experimental studies may provide an important proof of concept for intervention efficacy; however, they can sometimes be limited by factors, such as ethical or experimental conditions (53, 54). Although high heterogeneity was observed in the meta-analysis, subgroup and meta-regression analyses showed that study location, whether depression was excluded at baseline, screen time in the control group, and study quality could explain the potential heterogeneity. Heterogeneity moderately decreased when grouped by age and screen time category; thus, in addition to screen time duration, future studies should investigate the influence of screen time on different age groups and the effects of different screen mediums on mental health. Heterogeneity declined significantly as study quality improved. When studies that did not exclude depression at baseline were excluded, heterogeneity decreased to 54%. The original study’s design had a significant impact on the results, and future studies should consider study design rigor. In the subgroup analysis by screen equipment, watching television and using mobile phones increased the incidence of depression.
In the subgroup based on age, the association between screen time and depression was not statistically significant in the elders. Wang et al. (55) found that the elders who reported internet use had lower depression levels compared with those who did not. Another study on different types of internet use and depressive symptoms indicated that using the internet for social contact and entertainment decreased depression scores in the elderly, but when using the internet for learning, working, or commercial activity, the effect of relieving depressive symptoms disappeared (56). Elders spend more time online for recreational and leisure activities (56, 57). However, one study of internet use and mental health suggested that internet use affects mental health and increases the incidence of depressive symptoms in elders who may even experience feelings of technological panic (58). Future research should consider technological fear as an important influencing factor.
In the subgroup analysis by gender, screen time was significantly associated with an increased risk of depression in women; however, this association was not statistically significant in men. In general, screen time is considered a sedentary behavior, and this finding was consistent with previous meta-analyses on the association between sedentary behavior and mental health and screen time-based sedentary behavior on depression (29, 59). One explanation might be that women were more likely to be influenced by intimate social relationships that contribute to depression and thus would experience greater depressive symptoms than men. Kawachi et al. (60), Wirback et al. (61), and Altemus et al. (62) found that gender differences in support derived from social network participation may partly account for the higher prevalence of psychological distress among women compared with men, and smaller social networks, fewer close relationships, and lower perceived adequacy of social support have been linked to depressive symptoms (60–62). When screen time increases, women may have less time to communicate with others, which could result in developing fewer intimate relationships and smaller social networks, ultimately leading to mental disorders, such as depression.
Subgroup stratification according to whether a study was conducted during the COVID-19 pandemic showed that while screen time increased the risk of depression during the normal period, the opposite association was found during the COVID-19 pandemic (52). This may be because watching television and using mobile phones or computers could relieve an individual’s negative mood during lockdown conditions. Most of the subgroup effects were statistically significant. The population from Asia was at a higher risk than those from North America or Europe. An investigation of sitting time trends in 27 countries found that time spent on sedentary behaviors may not be increasing in the European region (8), and among these countries, the prevalence of depression has decreased steadily.
Electronic devices have become an integral part of many people’s lives, even among retired adults who do not need to work or study. In our study, we observed a significant effect of screen time on depression in youth (aged 0–20 years), although heterogeneity was high. Our findings support limiting screen time for adolescents. The results regarding the effect of screen time on depression in the elderly were not statistically significant, and our findings did not confirm if the relationship was positive or negative. Insufficient information in the original studies and the wide age range of participants made it difficult to perform a more detailed age group study. Future studies should focus on the effect of age and analyze the effect of screen time on depression risk separately for different age groups. Notably, the results stratified by subgroup, whether the study was conducted during the COVID-19 pandemic, suggested that screen time may alleviate depressive symptoms during lockdowns. Thus, the government should encourage people to use electronic devices to distract themselves and relieve their negative emotions during lockdown situations.
The underlying mechanisms of the relationship between screen time and depression risk remain unclear. With the increasing prevalence of depression, depression prevention has become a widespread public health concern. Therefore, identifying modifiable risk factors to aid in depression prevention is an important task. Several hypotheses may explain the impact of screen time on depression. First, increased screen time led to curtailed physical activity, which has been beneficial for reducing depression risk (63, 64). However, when stratified by physical activity regardless of if it was adjusted, the pooled results still indicated that screen time was a risk factor for depression. Tremblay et al. (27) suggested that independent of physical activity levels, screen time-based sedentary behaviors are associated with increased depression risk. As sedentary behavior and physical activity are both common human experiences, future studies are needed to explore the relationship between both behaviors and depression. Second, screen time, such as watching television and using mobile phones or computers, has been associated with sleep disorders, which could lead to sleep problems and increase the risk of depression (46, 65). Third, according to the causal model of social networks and social supports, social ties have protective effects on mental health and direct communication helps individuals build intimacy (60). Screen time can cause a reduction in social interactions and narrow social networks, and a lack of social networks may lead to social solitude and lower perceived adequacy of social support; thus, such changes could result in depressive symptoms. Fourth, RF-EMF may provide another explanation. With the rapid development and widespread use of electronic devices, health authorities have recognized the possible effects of long-term exposure to RF-EMF. Recent studies reported that RF-EMF may be linked to adverse health consequences, and several countries have made proposals to reduce the use of electronic devices. However, research findings on the relationship between EMFs and health problems have been inconsistent. Therefore, future studies should consider potential confounding or interactive effects, explore the underlying biological mechanism, and demonstrate an independent effect of screen time on depression risk.
This meta-analysis highlights the synthesized effects of screen time on depression risk. First, our research was based on cohort studies, which provided much stronger and more sufficient evidence. Second, it included a total of 237,146 participants from seven countries across Europe, Asia, and North America. The large sample size and wide range of locations significantly increased the statistical power and generalizability of the findings on the association between screen time and depression risk. Third, no obvious publication bias was detected in our study, which indicates that the combined results are reliable and convincing overall. Finally, our study excluded mixed sedentary behavior and screen time that could not be separated.
Some of this study’s potential limitations should also be discussed. First, recall bias and measurement errors are unavoidable when using self-report questionnaires to assess screen time. Electronic devices, such as mobile phones, computers, and smart televisions, can record time spent using the device in detail, thereby allowing for screen time to be measured objectively and conveniently. Researchers should obtain as much detailed information as possible and use objective measurements in future studies. Second, although included studies had adjusted for confounding factors, some of the included studies did not adjust for potential confounders, such as age, gender, and physical activity level, which might influence the association between screen time and depression risk. Future studies should pay more attention to these important covariates and measure and adjust for key variables. Third, the information in the included original studies was limited; therefore, we were unable to conduct dose-response analyses. Objective measurements of screen time are recommended, so we can easily collect more definite and detailed information about exposure to screen time and further analyze the dose-response effects.
This meta-analysis demonstrates that screen time is likely to increase the risk of depression. The high heterogeneity may be the result of a less rigorous original study design across the included studies. The effects of screen time on depression risk may vary widely, and the determinants or the benefits of screen time were shown to differ based on duration and individual characteristics (e.g., age, gender, and location). Among young and female populations, screen time was found to significantly increase depression risk. Compared with less screen time, screen time exceeding 1 h/day was associated with a higher risk of depression. Our findings support the recommendations to limit the prolonged use of electronic devices. Objective measures are recommended to use in future studies to explore complex relationships and specific time constraints of screen time. Considering the increasing prevalence of depression in modern society and the increasing number of people exposed to screens for long periods, our findings have important implications for depression prevention.
The raw data supporting the conclusions of this article will be made available by the authors, without undue reservation.
CW and LL conceived the study. GZ, HH, JZ, XK, SY, DY, and ZC were responsible for the collection and cleaning of the data and assisted in the writing of the manuscript. LL and QZ wrote the manuscript. LZ, YG, and ZL contributed to the review and revision of the study. CW was the guarantor of this work and, as such, had full access to all the data in the study and takes responsibility for the integrity of the data and the accuracy of the data analysis. All authors contributed to the article and approved the submitted version.
This work was supported by the Science and Technology Research Project of Education Department of Jiangxi Province (GJJ190814).
The authors declare that the research was conducted in the absence of any commercial or financial relationships that could be construed as a potential conflict of interest.
All claims expressed in this article are solely those of the authors and do not necessarily represent those of their affiliated organizations, or those of the publisher, the editors and the reviewers. Any product that may be evaluated in this article, or claim that may be made by its manufacturer, is not guaranteed or endorsed by the publisher.
The Supplementary Material for this article can be found online at: https://www.frontiersin.org/articles/10.3389/fpsyt.2022.1058572/full#supplementary-material
Supplementary File 1 | The PRISMA checklist.
Supplementary File 2 | Details of included articles.
1. Whiteford H, Degenhardt L, Rehm J, Baxter A, Ferrari A, Erskine H, et al. Global burden of disease attributable to mental and substance use disorders: findings from the Global burden of disease study 2010. Lancet. (2013) 382:1575–86. doi: 10.1016/S0140-6736(13)61611-6
2. Gbd 2019 Mental Disorders Collaborators. Global, regional, and national burden of 12 mental disorders in 204 countries and territories, 1990-2019: a systematic analysis for the Global burden of disease study 2019. Lancet Psychiatry. (2022) 9:137–50. doi: 10.1016/S2215-0366(21)00395-3
3. Izaki Y. Depression among adolescents: clinical features and interventions. J Med Invest. (2021) 68:22–8. doi: 10.2152/jmi.68.22
4. Lopez A, Mathers C. Measuring the global burden of disease and epidemiological transitions: 2002-2030. Ann Trop Med Parasitol. (2006) 100:481–99. doi: 10.1179/136485906X97417
5. Mathers C, Loncar D. Projections of global mortality and burden of disease from 2002 to 2030. PLoS Med. (2006) 3:e442. doi: 10.1371/journal.pmed.0030442
6. Covid-19 Mental Disorders Collaborators. Global prevalence and burden of depressive and anxiety disorders in 204 countries and territories in 2020 due to the COVID-19 pandemic. Lancet. (2021) 398:1700–12. doi: 10.1016/S0140-6736(21)02143-7
7. Liu M, Wu L, Yao S. Dose-response association of screen time-based sedentary behaviour in children and adolescents and depression: a meta-analysis of observational studies. Br J Sports Med. (2016) 50:1252–8. doi: 10.1136/bjsports-2015-095084
8. Milton K, Gale J, Stamatakis E, Bauman A. Trends in prolonged sitting time among European adults: 27 country analysis. Prev Med. (2015) 77:11–6. doi: 10.1016/j.ypmed.2015.04.016
9. Ten Broeke P, Olthof M, Beckers D, Hopkins N, Graves L, Carter S, et al. Temporal dynamics of sitting behavior at work. Proc Natl Acad Sci USA. (2020) 117:14883–9. doi: 10.1073/pnas.2001284117
10. Redmayne M, Smith E, Abramson M. The relationship between adolescents’ well-being and their wireless phone use: a cross-sectional study. Environ Health. (2013) 12:90. doi: 10.1186/1476-069X-12-90
11. Poitras V, Gray C, Janssen X, Aubert S, Carson V, Faulkner G, et al. Systematic review of the relationships between sedentary behaviour and health indicators in the early years (0-4 years). BMC Public Health. (2017) 17(Suppl. 5):868. doi: 10.1186/s12889-017-4849-8
12. Carson V, Hunter S, Kuzik N, Gray C, Poitras V, Chaput J, et al. Systematic review of sedentary behaviour and health indicators in school-aged children and youth: an update. Appl Physiol Nutr Metab. (2016) 41(6 Suppl. 3):S240–65. doi: 10.1139/apnm-2015-0630
13. Tremblay M, Carson V, Chaput J, Connor Gorber S, Dinh T, Duggan M, et al. Canadian 24-hour movement guidelines for children and youth: an integration of physical activity, sedentary behaviour, and sleep. Appl Physiol Nutr Metab. (2016) 41(6 Suppl. 3):S311–27. doi: 10.1139/apnm-2016-0151
14. Virgara R, Phillips A, Lewis L, Richardson M, Maher C. Physical activity and screen time in outside school hours care services across Australia: current versus best practice. BMC Public Health. (2022) 22:680. doi: 10.1186/s12889-022-13135-7
15. Hollman H, Updegraff J, Lipkus I, Rhodes R. Perceptions of physical activity and sedentary behaviour guidelines among end-users and stakeholders: a systematic review. Int J Behav Nutr Phys Act. (2022) 19:21. doi: 10.1186/s12966-022-01245-9
16. Yu Y, Hsieh T, Lin G, Lee S, Huang C, Chen K. High levels of screen time were associated with increased probabilities of lagged development in 3-year-old children. Acta Paediatr. (2022) 111:1736–42. doi: 10.1111/apa.16373
17. Niiranen J, Kiviruusu O, Vornanen R, Saarenpää-Heikkilä O, Paavonen E. High-dose electronic media use in five-year-olds and its association with their psychosocial symptoms: a cohort study. BMJ Open. (2021) 11:e040848. doi: 10.1136/bmjopen-2020-040848
18. Mitchell J, Rodriguez D, Schmitz K, Audrain-McGovern J. Greater screen time is associated with adolescent obesity: a longitudinal study of the BMI distribution from ages 14 to 18. Obesity (Silver Spring). (2013) 21:572–5. doi: 10.1002/oby.20157
19. Reyna-Vargas M, Parmar A, Lefebvre D, Azad M, Becker A, Turvey S, et al. Longitudinal associations between sleep habits, screen time and overweight, obesity in preschool children. Nat Sci Sleep. (2022) 14:1237–47. doi: 10.2147/NSS.S363211
20. Sakamoto N, Kabaya K, Nakayama M. Sleep problems, sleep duration, and use of digital devices among primary school students in Japan. BMC Public Health. (2022) 22:1006. doi: 10.1186/s12889-022-13389-1
21. Hale L, Guan S. Screen time and sleep among school-aged children and adolescents: a systematic literature review. Sleep Med Rev. (2015) 21:50–8. doi: 10.1016/j.smrv.2014.07.007
22. Ford E, Caspersen C. Sedentary behaviour and cardiovascular disease: a review of prospective studies. Int J Epidemiol. (2012) 41:1338–53. doi: 10.1093/ije/dys078
23. Jingjie W, Yang L, Jing Y, Ran L, Yiqing X, Zhou N. Sedentary time and its association with risk of cardiovascular diseases in adults: an updated systematic review and meta-analysis of observational studies. BMC Public Health. (2022) 22:286. doi: 10.1186/s12889-022-12728-6
24. Feng Q, Zhang Q, Du Y, Ye Y, He Q. Associations of physical activity, screen time with depression, anxiety and sleep quality among Chinese college freshmen. PLoS One. (2014) 9:e100914. doi: 10.1371/journal.pone.0100914
25. Li X, Buxton O, Lee S, Chang A, Berger L, Hale L. Sleep mediates the association between adolescent screen time and depressive symptoms. Sleep Med. (2019) 57:51–60. doi: 10.1016/j.sleep.2019.01.029
26. Liu M, Zhang J, Hu E, Yang H, Cheng C, Yao S. Combined patterns of physical activity and screen-related sedentary behavior among Chinese adolescents and their correlations with depression, anxiety and self-injurious behaviors. Psychol Res Behav Manag. (2019) 12:1041–50. doi: 10.2147/PRBM.S220075
27. Tremblay M, LeBlanc A, Kho M, Saunders T, Larouche R, Colley R, et al. Systematic review of sedentary behaviour and health indicators in school-aged children and youth. Int J Behav Nutr Phys Act. (2011) 8:98. doi: 10.1186/1479-5868-8-98
28. Belyaev I, Dean A, Eger H, Hubmann G, Jandrisovits R, Kern M, et al. EUROPAEM EMF Guideline 2016 for the prevention, diagnosis and treatment of EMF-related health problems and illnesses. Rev Environ Health. (2016) 31:363–97. doi: 10.1515/reveh-2016-0011
29. Wang X, Li Y, Fan H. The associations between screen time-based sedentary behavior and depression: a systematic review and meta-analysis. BMC Public Health. (2019) 19:1524. doi: 10.1186/s12889-019-7904-9
30. Diler F, Başkale H. The influence of sleep patterns and screen time on the sleep needs of infants and toddlers: a cross-sectional study. J Pediatr Nurs. (2022) 67:e201–7. doi: 10.1016/j.pedn.2022.07.014
31. Stroup D, Berlin J, Morton S, Olkin I, Williamson G, Rennie D, et al. Meta-analysis of observational studies in epidemiology: a proposal for reporting. meta-analysis of observational studies in epidemiology (MOOSE) group. JAMA. (2000) 283:2008–12. doi: 10.1001/jama.283.15.2008
32. Moher D, Liberati A, Tetzlaff J, Altman D, Prisma Group. Preferred reporting items for systematic reviews and meta-analyses: the PRISMA statement. PLoS Med. (2009) 6:e1000097. doi: 10.1371/journal.pmed.1000097
33. Wells G, Shea B, O’Connell D. The Newcastle-Ottawa Scale (NOS) for Assessing the Quality of Nonrandomised Studies in Meta-Analyses. Ottawa, ON: Ottawa Hospital Research Institute (2011).
34. Zhang J, Yu K. What’s the relative risk? A method of correcting the odds ratio in cohort studies of common outcomes. JAMA. (1998) 280:1690–1. doi: 10.1001/jama.280.19.1690
35. Sarris J, Thomson R, Hargraves F, Eaton M, de Manincor M, Veronese N, et al. Multiple lifestyle factors and depressed mood: a cross-sectional and longitudinal analysis of the UK Biobank (N = 84,860). BMC Med. (2020) 18:354. doi: 10.1186/s12916-020-01813-5
36. Liu W, Wu X, Huang K, Yan S, Ma L, Cao H, et al. Early childhood screen time as a predictor of emotional and behavioral problems in children at 4 years: a birth cohort study in China. Environ Health Prev Med. (2021) 26:3. doi: 10.1186/s12199-020-00926-w
37. Liu S, Wing Y, Hao Y, Li W, Zhang J, Zhang B. The associations of long-time mobile phone use with sleep disturbances and mental distress in technical college students: a prospective cohort study. Sleep. (2019) 42:zsy213. doi: 10.1093/sleep/zsy213
38. Sui X, Brown W, Lavie C, West D, Pate R, Payne J, et al. Associations between television watching and car riding behaviors and development of depressive symptoms: a prospective study. Mayo Clin Proc. (2015) 90:184–93. doi: 10.1016/j.mayocp.2014.12.006
39. Wu X, Tao S, Zhang S, Zhang Y, Chen K, Yang Y, et al. Impact of screen time on mental health problems progression in youth: a 1-year follow-up study. BMJ Open. (2016) 6:e011533. doi: 10.1136/bmjopen-2016-011533
40. Khouja J, Munafò M, Tilling K, Wiles N, Joinson C, Etchells P, et al. Is screen time associated with anxiety or depression in young people? Results from a UK birth cohort. BMC Public Health. (2019) 19:82. doi: 10.1186/s12889-018-6321-9
41. Primack B, Swanier B, Georgiopoulos A, Land S, Fine M. Association between media use in adolescence and depression in young adulthood: a longitudinal study. Arch Gen Psychiatry. (2009) 66:181–8. doi: 10.1001/archgenpsychiatry.2008.532
42. Zink J, Belcher B, Kechter A, Stone M, Leventhal A. Reciprocal associations between screen time and emotional disorder symptoms during adolescence. Prev Med Rep. (2019) 13:281–8. doi: 10.1016/j.pmedr.2019.01.014
43. Padmapriya N, Bernard J, Liang S, Loy S, Shen Z, Kwek K, et al. Association of physical activity and sedentary behavior with depression and anxiety symptoms during pregnancy in a multiethnic cohort of Asian women. Arch Womens Ment Health. (2016) 19:1119–28. doi: 10.1007/s00737-016-0664-y
44. Thomée S, Härenstam A, Hagberg M. Mobile phone use and stress, sleep disturbances, and symptoms of depression among young adults–a prospective cohort study. BMC Public Health. (2011) 11:66. doi: 10.1186/1471-2458-11-66
45. Thomée S, Härenstam A, Hagberg M. Computer use and stress, sleep disturbances, and symptoms of depression among young adults–a prospective cohort study. BMC Psychiatry. (2012) 12:176. doi: 10.1186/1471-244X-12-176
46. Lucas M, Mekary R, Pan A, Mirzaei F, O’Reilly E, Willett W, et al. Relation between clinical depression risk and physical activity and time spent watching television in older women: a 10-year prospective follow-up study. Am J Epidemiol. (2011) 174:1017–27. doi: 10.1093/aje/kwr218
47. Kandola A, Owen N, Dunstan D, Hallgren M. Prospective relationships of adolescents’ screen-based sedentary behaviour with depressive symptoms: the Millennium cohort study. Psychol Med. (2021) 19:1–9. doi: 10.1017/S0033291721000258
48. Meyer J, O’Connor J, McDowell C, Lansing J, Brower C, Herring M. High sitting time is a behavioral risk factor for blunted improvement in depression across 8 weeks of the COVID-19 pandemic in April-May 2020. Front Psychiatry. (2021) 12:741433. doi: 10.3389/fpsyt.2021.741433
49. Choi K, Stein M, Nishimi K, Ge T, Coleman J, Chen C, et al. An exposure-wide and mendelian randomization approach to identifying modifiable factors for the prevention of depression. Am J Psychiatry. (2020) 177:944–54. doi: 10.1176/appi.ajp.2020.19111158
50. Pimenta A, Mendonça R, Lahortiga-Ramos F, Fernandez-Lazaro C, Martínez-González M, Sánchez-Villegas A. Sedentary behaviors and risk of depression in the Seguimiento Universidad de Navarra cohort: the SUN Project. Cadernos de Saúde Pública [online]. (2022) 38:e00076621. doi: 10.1590/0102-311X00076621
51. Grøntved A, Singhammer J, Froberg K, Møller N, Pan A, Pfeiffer K, et al. A prospective study of screen time in adolescence and depression symptoms in young adulthood. Prev Med. (2015) 81:108–13. doi: 10.1016/j.ypmed.2015.08.009
52. Ayuso-Mateos J, Morillo D, Haro J, Olaya B, Lara E, Miret M. Changes in depression and suicidal ideation under severe lockdown restrictions during the first wave of the COVID-19 pandemic in Spain: a longitudinal study in the general population. Epidemiol Psychiatr Sci. (2021) 30:e49. doi: 10.1017/S2045796021000408
53. Liu Y, Liu X, Wang Y, Wang D, Ma P. [Interpretation of guidelines for controlling confounding factors and reporting results in causal inference studies]. Zhonghua Wei Zhong Bing Ji Jiu Yi Xue. (2021) 33:113–6. doi: 10.3760/cma.j.cn121430-20201127-00734
54. Sargeant J, Kelton D, O’Connor A. Study designs and systematic reviews of interventions: building evidence across study designs. Zoonoses Public Health. (2014) 61(Suppl. 1):10–7. doi: 10.1111/zph.12127
55. Wang Y, Zhang H, Feng T, Wang H. Does internet use affect levels of depression among older adults in China? A propensity score matching approach. BMC Public Health. (2019) 19:1474. doi: 10.1186/s12889-019-7832-8
56. Yang H, Zhang S, Zhang S, Xie L, Wu Y, Yao Y, et al. Internet use and depressive symptoms among older adults in China. Front Psychiatry. (2021) 12:739085. doi: 10.3389/fpsyt.2021.739085
57. Zhang H, Wang H, Yan H, Wang X. Impact of internet use on mental health among elderly individuals: a difference-in-differences study based on 2016-2018 CFPS data. Int J Environ Res Public Health. (2021) 19:101. doi: 10.3390/ijerph19010101
58. Xie L, Yang H, Lin X, Ti S, Wu Y, Zhang S, et al. Does the internet use improve the mental health of Chinese older adults? Front Public Health. (2021) 9:673368. doi: 10.3389/fpubh.2021.673368
59. Zhang J, Yang S, Wang L, Han L, Wu X. The influence of sedentary behaviour on mental health among children and adolescents: a systematic review and meta-analysis of longitudinal studies. J Affect Disord. (2022) 306:90–114. doi: 10.1016/j.jad.2022.03.018
60. Kawachi I, Berkman L. Social ties and mental health. J Urban Health. (2001) 78:458–67. doi: 10.1093/jurban/78.3.458
61. Barnett P, Gotlib I. Psychosocial functioning and depression: distinguishing among antecedents, concomitants, and consequences. Psychol Bull. (1988) 104:97–126. doi: 10.1037/0033-2909.104.1.97
62. Nolen-Hoeksema S. Sex differences in unipolar depression: evidence and theory. Psychol Bull. (1987) 101:259–82.
63. Rodriguez-Ayllon M, Cadenas-Sánchez C, Estévez-López F, Muñoz N, Mora-Gonzalez J, Migueles J, et al. Role of physical activity and sedentary behavior in the mental health of preschoolers, children and adolescents: a systematic review and meta-analysis. Sports Med. (2019) 49:1383–410. doi: 10.1007/s40279-019-01099-5
64. Biddle S, Asare M. Physical activity and mental health in children and adolescents: a review of reviews. Br J Sports Med. (2011) 45:886–95. doi: 10.1136/bjsports-2011-090185
Keywords: screen time, depression, meta-analysis, mental health, epidemiology
Citation: Li L, Zhang Q, Zhu L, Zeng G, Huang H, Zhuge J, Kuang X, Yang S, Yang D, Chen Z, Gan Y, Lu Z and Wu C (2022) Screen time and depression risk: A meta-analysis of cohort studies. Front. Psychiatry 13:1058572. doi: 10.3389/fpsyt.2022.1058572
Received: 30 September 2022; Accepted: 28 November 2022;
Published: 22 December 2022.
Edited by:
Liye Zou, Shenzhen University, ChinaReviewed by:
Sukrit Kanchanasurakit, University of Phayao, ThailandCopyright © 2022 Li, Zhang, Zhu, Zeng, Huang, Zhuge, Kuang, Yang, Yang, Chen, Gan, Lu and Wu. This is an open-access article distributed under the terms of the Creative Commons Attribution License (CC BY). The use, distribution or reproduction in other forums is permitted, provided the original author(s) and the copyright owner(s) are credited and that the original publication in this journal is cited, in accordance with accepted academic practice. No use, distribution or reproduction is permitted which does not comply with these terms.
*Correspondence: Chunmei Wu, ✉ d3VjaHVubWVpQGdtdS5lZHUuY24=
Disclaimer: All claims expressed in this article are solely those of the authors and do not necessarily represent those of their affiliated organizations, or those of the publisher, the editors and the reviewers. Any product that may be evaluated in this article or claim that may be made by its manufacturer is not guaranteed or endorsed by the publisher.
Research integrity at Frontiers
Learn more about the work of our research integrity team to safeguard the quality of each article we publish.