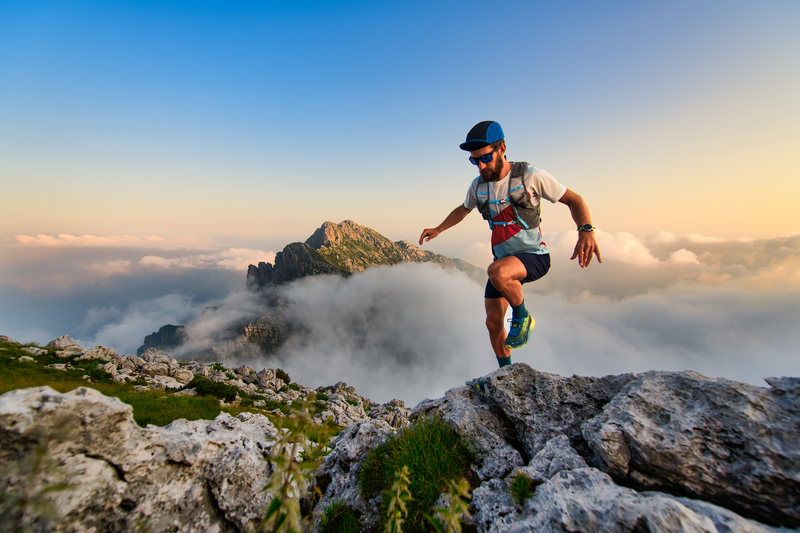
94% of researchers rate our articles as excellent or good
Learn more about the work of our research integrity team to safeguard the quality of each article we publish.
Find out more
MINI REVIEW article
Front. Psychiatry , 18 October 2022
Sec. Sleep Disorders
Volume 13 - 2022 | https://doi.org/10.3389/fpsyt.2022.1037927
This article is part of the Research Topic Sleep Problems: Diagnosis, Biomarkers, Interventions, and Treatments View all 22 articles
Sleep disorders are prevalent nowadays, leading to anxiety, depression, high blood pressure, and other health problems. Due to the proliferation of mobile devices and the development of communication technologies, mobile apps have become a popular way to deliver sleep disorder therapy or manage sleep. This scoping review aims to conduct a systematic investigation of mobile apps and technologies supporting sleep, including the essential functions of sleep apps, how they are used to improve sleep and the facilitators of and barriers to using apps among patients and other stakeholders. We searched articles (2010 to 2022) from Scopus, Web of Science, Science Direct, PubMed, and IEEE Xplore using the keyword sleep apps. In total, 1,650 peer-reviewed articles were screened, and 51 were selected for inclusion. The most frequently provided functions by the apps are sleep monitoring, measuring sleep, providing alarms, and recording sleep using a sleep diary. Several wearable devices have been used with mobile apps to record sleep duration and sleep problems. Facilitators and barriers to using apps were identified, along with the evidence-based design guidelines. Existing studies have proved the initial validation and efficiency of delivering sleep treatment by mobile apps; however, more research is needed to improve the performance of sleep apps and devise a way to utilize them as a therapy tool.
Sleep is an essential biological need for human beings that will support us in getting resting, healing, and being ready for the next day. It is widely accepted that disturbed sleep is an influential factor leading to many mental health disorders. According to the Sleep Health Survey of Australian Adults (1), inadequate sleep has affected 33–45% of adults in Australia. Sleep disorders include short sleep duration, insomnia, snoring, sleep apnea, parasomnias, and restless leg syndrome. The treatment of sleep disorders varies, and some can be delivered online, such as Cognitive Behavioral Therapy for insomnia. With the development of technology, there are fewer barriers to accessing mobile phones, and we can establish mobile apps with many useful functions. Thus, mobile apps have become a popular tool for delivering sleep treatments. For example, with the microphone and sound sensor of the mobile phone, we can monitor people's breath while sleeping. It is crucial to analyse the utilization of mobile apps to support sleep and further improve the quality of daily life.
Previous studies have already analyzed the validation and efficiency of sleep apps. For example, Baron et al. (2) conducted a scoping review of the use of consumer-targeted wearable and mobile technology. They found that most of the articles they reviewed focused on validation of sleep application, and there was a gap in interventions in more target populations such as patient populations (2). Shin et al. (3) stated that mobile phone interventions could attenuate sleep disorders and improve sleep quality. With a three-piece test set up by Stippig et al. (4), the result shows that most apps cannot distinguish and record snoring noises from various disturbing noises in real-life situations. Cajita et al. (5) also stated in their scoping review that the utility of wearable activity monitors in improving sleep needs more evidence to support it. An app review completed by Choi et al. (6) found that most sleep apps in the market cannot meet the quality, content, and functionality requirements to manage sleep by users. Therefore, guidelines are needed to improve the performance of sleep apps, and this paper aims to review the current apps to understand the research gaps and provide guidelines to design better sleep apps. We have conducted a scoping review of the articles published in 2010–2022 to understand the features of the sleep apps, user requirements and the design guidelines to improve those apps.
We applied the scoping review methodology proposed by Arksey and O'Malley (7), which had been further improved by the methodology developed by the Joanna Briggs Institute (8).
In this step, we reviewed previous research works and determined the gaps. The research questions are as follows:
1. What are the key functions of the current sleep apps?
2. What are the limitations of sleep apps?
3. What are the user requirements and design guidelines of sleep apps?
We used keywords such as sleep apps and sleep monitoring. To find relevant articles, we searched Scopus, Web of Science, Science Direct, PubMed, and IEEE Xplore. Only peer-reviewed articles written in English and published from 2010 to 2022 were considered.
After exporting research results into Endnote and deleting the duplication, we screened the titles and abstracts of all these articles and removed those unrelated to our topic. The following inclusion criteria were followed: (1) Does the article involve a sleep app? (2) Does the article report the testing of a sleep app? and (3) Is the article written in English?
A full-text review was carried out for these references, and those only 51 articles were selected to extract data for analysis. More detailed information can be found in Figure 1.
All included studies were reviewed and charted using a data extraction sheet. The details about the articles such as the country of publication, research method, year of publication, target group, target sleep issue, and the information about the functions provided by the sleep apps, the limitation and outstanding of sleep apps, the user requirement of the app and the design guidelines were extracted for analysis.
The filtered studies were analyzed, and the details were presented using tables and graphs.
In total, 51 articles were included in the final review. Thirty-three percentage (17/51) of these publications come from the United States, 20% (10/51) from the UK, 12% (6/51) from China, 10% (5/51) from Canada, 8% (4/51) from Korea, 6% (3/51) from Ireland and 6% (3/51) from Austria. Thirty-one percentage (16/51) of the articles did not focus on one particular issue of sleep disorders or changing sleep habits. Only 20% (10/51) of them are related to apnoea issues, 12% (6/51) of them aimed to help people to develop healthy sleep habits, 22% (11/51) tried to treat insomnia and 12% (6/51) focused on recording sleep and wake detection. Two articles focused on sleep onset and utilized external stimuli to help people fall asleep faster.
Sixty-six percentage (34/51) articles have mentioned the monitoring functions of mobile sleep applications, indicating that monitoring sleep is the most common function among the existing applications (9–11). Rönkkö (12) has connected mobile apps with an activity tracker; thus, the data collected by the external tracker can be transferred to the mobile app. The mobile app has several functions such as monitoring, reminding, alarm and goal setting. Validated smartphone apps can help detect sleep times and related sleep issues to support clinical treatment. Such a treatment can be provided by a smartphone app called UP! that is capable of accurately measuring sleep durations for individuals with bipolar disorder (13). Non-invasive sleep monitoring using Ballistocardiography (BCG) can help people wake up smoothly compared to Polysomnography (PSG) (14). In addition, a smartphone meditation app can help the prehypertensive population to measure sleep quality (15).
According to the data extracted from these 51 articles, an alarm is the second most frequent function. The concept of alarm in different apps can sometimes have different meanings. Firstly, it is the regular clock that users need to set a time in the app in advance, and then the app will start an alarm at that time. In this case, users determine the time when to awake. Some apps monitor users' sleep and measure light and deep sleep so that the app will awaken users in light sleep (16). In this case, the app will determine the time to awake users.
CBT-I Coach is a mobile application that delivers cognitive behavioral therapy for insomnia (17–19). These apps offer sleep diaries, education, alarm and relaxation exercise functions. The app enables insomnia patients to update their sleep prescription from CBT-I providers to the app, and then the app will help manage and remind users of their own needs, such as recommended bedtime and wake time. Users can also export their sleep diary for further usages, such as translation, to health professionals for treatment.
Several other apps have been developed to support people with insomnia. For instance, researchers developed a mobile app to deliver Sleep restriction therapy (SRT) for people with insomnia (20). Another mobile app called “MIND MORE” can help with the self-management of insomnia (21). Similar to “MIND MORE,” “Insomnia Coach” app helps people to self-manage insomnia (22). KANOPEE app provides behavioral intervention for individuals with insomnia symptoms through an interaction with a virtual agent (23). This app provides more benefit than an electronic sleep diary to support people with insomnia.
Sleep quality and quantity are two factors for measuring sleep. Most mobile apps are implemented by consumer sleep tracking devices to collect and utilize data to measure users' sleep quality and quantity (24). The measurement accuracy of these tracking devices and mobile apps is always low compared to traditional polysomnography, the gold standard for sleep assessment. The current technology cannot support mobile apps as accurate as polysomnography; however, it can be a suitable replacement because of its high cost and low convenience (25). Researchers have developed an EarlySense contact-free sensor and smartphone app to collect vital signs and analyse sleep patterns (26). Validation of the EarlySense sensor showed accuracy in detecting sleep and wake states relative to the gold standard polysomnography.
Sleep disorder detection is one crucial function provided by mobile apps (27). Disorders in the early stage are much easier to be treated than later. Tseng et al. (28) and Behar et al. (29) developed an intelligent mobile app to screen users and detect obstructive sleep apnoea patients. The SleepAp (29) uses signal processing and machine learning algorithm to screen for obstructive sleep apnoea at a negligible cost. Researchers also developed a smartphone app called “Firefly” to measure obstructive sleep apnoea (30). The app is reliable and accurate in detecting obstructive sleep apnoea compared to polysomnography.
With the internet, mobile apps can enable users in the same community and share information and data. Being in the community can be achieved within users and also external identities. Users can share their sleep goals and get competed in the app presented by Rönkkö (12), and they can also export the data from mobile apps and transfer it by a network to other individuals such as health care providers (18).
The most significant limitation of sleep apps is the accuracy to monitor sleep (n = 5). Mansukhani and Kolla (31) evaluated the major shortcoming and limited utility of sleep apps in the clinical population. They concluded that sleep data gathered from tracking devices are less reliable in patients with insomnia and fragmented sleep problems. The tracking devices accommodated with sleep apps could not distinguish various sleep stages in different users (31). Several smartphone applications were developed for sleep–wake detection through sound and movement sensors (32). While comparing the performance of the apps, the sleep wake detection was found not sufficiently reliable compared with polysomnography. Wearable devices have been used with a mobile app to record sleep; however, they have shortcomings in measuring sleep problems (33). For instance, ActiGraph wGT3X-BT accelerometers have been used with the SleepBot app for people with schizophrenia to measure sedentary behavior and sleep. It was observed that the app's measure of sleep was inaccurate. Smartphone applications and associated wearable sleep tracking devices have limitations in detecting sleep durations, efficiency, and sleep cycle detection sleep compared to polysomnography (PSG) (34). Therefore, to improve the accuracy of sleep predictions, researchers suggest combining actigraphy-based sleep detection by using the data from movement sensors with the use of technology detected by smartphones (35).
The issue of poor sleep cycle detection by smart phone app has been reported in Bhat et al. (36) and the results suggest that current sleep apps need to have improved accuracy to be used as potential clinical utility tool.
While mobile apps collect and manage users' sleep data, the privacy of the data becomes a common concern. Leigh et al. (37) assessed the quality of apps designed for chronic insomnia disorders from the Android Google Play Store and evaluated their risk along with the privacy policy. Fino et al. (32) compared the performance of four existing sleep apps with polysomnography. The result showed that none of these four apps could detect rapid eye movement sleep, and the overall performance of sleep apps was worse than polysomnography. Short battery life is also a limitation of sleep apps, especially for those accommodated with small sensor devices (38).
Different user populations will have different characteristics regarding sleep, and each individual will further require unique demands from sleep apps. User-centered design is an efficient approach to developing clinical applications proved by Luna et al. (39) and McCurdie et al. (40). Aji et al. (41) applied a mixed-method study to explore and determine the end users' requirements and preferences for sleep applications. Users prefer the free app so that they can make a long-term commitment. Personalisation is an essential requirement for users to sleep apps. Nguyen (42) presented an approach to personalizing smart apps by personality traits and chorotype. Users are more likely to choose an app that provides a privacy policy with a high-security level (28).
Mobile apps can be used to support healthy sleep habits. Grigsby-Toussaint et al. (43) examined 35 apps and found that only a few apps included features to change behavior.
Theory-based, evidence-based and user-based are the three approaches to develop sleep apps as observed in those articles. Antezana et al. (44) evaluated thirty existing apps for physical activity, diet and sleep and determined whether they had followed theory-based behavior change techniques. All the 30 apps included at least one behavior change technique (BCT) in their design, and the most frequently used ones were goal setting and feedback.
User-centered is one of the basic design method for developing sleep apps (41). The preferences and social and cultural contextual factors of target populations should be considered when designing sleep apps (45). Evidence-based principles are also frequently included in app designs (46). Users preferred the apps that provide sleep tips based on empirical evidence (47). Personalized feedback (48), connection to other apps and multifunctional (49), and engaging content and easy-to-follow format (46) are some other design guidelines included in the articles. To engage the users, the app's content can be more interactive, such as a virtual pet (50).
The apps that automatically track sleep should enable users to edit the records manually, let the users take control of their data, and enable them to export the data (6). Hosszu et al. (51) stated that sleep apps should connect users with medical professionals and consider ethical issues when designing. Shin et al. (3) and Fino and Mazzetti (52) also suggested developing apps following design guidelines that are evidence-based and include behavior change techniques. Table 1 summarizes the key features of sleep apps and design considerations found in our included studies.
Mobile apps have become a popular way to support sleep, and many such apps exist in the market. The most frequently provided functions by the apps are sleep monitoring, measuring sleep, providing alarms, and recording sleep using a sleep diary. There is a lack of apps that support active medical therapy. Therefore, the role of sleep apps in supporting sleep disorder treatments still needs further investigation. Easy-to-use, low-cost, simple device, mobility and flexibility features make the sleep apps an excellent choice to support sleep. However, current sleep apps have some limitations, such as accuracy, privacy and security issues, short battery life and information quality. Frequently reported design guidelines for developing sleep apps are user-centered, evidence-based, theory-based, engagement, feedback, accessible format and social connectedness. We did not find much information about the user requirements of sleep apps for different populations. Future research is needed to determine the user requirements for diverse populations.
AA and OM conceived and designed the review. JW collected data and conducted the initial analysis with the help of AA. JW and AA wrote the manuscript, which was reviewed, and revised by OM. All authors contributed to the article and approved the submitted version.
The authors declare that the research was conducted in the absence of any commercial or financial relationships that could be construed as a potential conflict of interest.
All claims expressed in this article are solely those of the authors and do not necessarily represent those of their affiliated organizations, or those of the publisher, the editors and the reviewers. Any product that may be evaluated in this article, or claim that may be made by its manufacturer, is not guaranteed or endorsed by the publisher.
1. Adams R, Appleton S, Taylor A, McEvoy D, Antic N. Report to the Sleep Health Foundation 2016 Sleep Health Survey of Australian Adults. Adelaide: The Adelaide Institute for Sleep Health and The University of Adelaide (2016).
2. Baron KG, Duffecy J, Berendsen MA, Mason IC, Lattie EG, Manalo NC. Feeling validated yet? a scoping review of the use of consumer-targeted wearable and mobile technology to measure and improve sleep. Sleep Med Rev. (2018) 40:151–9. doi: 10.1016/j.smrv.2017.12.002
3. Shin JC, Kim J, Grigsby-Toussaint D. Mobile phone interventions for sleep disorders and sleep quality: systematic review. JMIR mHealth and uHealth. (2017) 5:e7244. doi: 10.2196/mhealth.7244
4. Stippig A, Hübers U, Emerich M. Apps in sleep medicine. Sleep Breath. (2015) 19:411–7. doi: 10.1007/s11325-014-1009-6
5. Cajita MI, Kline CE, Burke LE, Bigini EG, Imes CC. Feasible but not yet efficacious: a scoping review of wearable activity monitors in interventions targeting physical activity, sedentary behavior, and sleep. Curr Epidemiol Rep. (2020) 7:25–38. doi: 10.1007/s40471-020-00229-2
6. Choi YK, Demiris G, Lin S-Y, Iribarren SJ, Landis CA, Thompson HJ, et al. Smartphone applications to support sleep self-management: review and evaluation. J Clin Sleep Med. (2018) 14:1783–90. doi: 10.5664/jcsm.7396
7. Arksey H, O'Malley L. Scoping studies: towards a methodological framework. Int J Soc Res Methodol. (2005) 8:19–32. doi: 10.1080/1364557032000119616
8. Joanna Briggs Institute. (2015). Reviewers' Manual 2015 Methodology for JBI Scoping Reviews. Adelaide, Australia: Royal Adelaide Hospital.
9. Zhang Z, Guan C, Chan TE, Yu J, Wai AAP, Wang C, et al. Reduction in time-to-sleep through EEG based brain state detection and audio stimulation. In: Proceedings of the 2015 37th Annual International Conference of the IEEE Engineering in Medicine and Biology Society (EMBC). (Milan) (2015). p. 8050–3. doi: 10.1109/EMBC.2015.732026
10. Zaffaroni A, Coffey S, Dodd S, Kilroy H, Lyon G, O'Rourke D. Sleep staging monitoring based on sonar smartphone technology. In: Proceedings of the 2019 41st Annual International Conference of the IEEE Engineering in Medicine and Biology Society (EMBC) (Berlin). (2019). doi: 10.1109/EMBC.2019.8857033
11. Chen Z, Lin M, Chen F, Lane N, Cardone G, Wang R, et al. Unobtrusive sleep monitoring using smartphones. In: Proceedings of the 2013 7th International Conference on Pervasive Computing Technologies for Healthcare and Workshops (Venice). (2013). p. 145–52. doi: 10.4108/icst.pervasivehealth.2013.252148
12. Rönkkö, K. An activity tracker and its accompanying app as a motivator for increased exercise and better sleeping habits for youths in need of social care: field study. JMIR mHealth and uHealth. (2018) 6:e9286. doi: 10.2196/mhealth.9286
13. Fellendorf FT, Hamm C, Dalkner N, Platzer M, Sattler MC, Bengesser SA, et al. Monitoring sleep changes via a smartphone app in bipolar disorder: practical issues and validation of a potential diagnostic tool. Front Psychiatry. (2021) 12:641241. doi: 10.3389/fpsyt.2021.641241
14. Silva B, Marinheiro RN. Non-invasive monitoring with ballistocardiographic sensors for sleep management. 2021 Telecoms Conference (ConfTELE). Leiria: IEEE (2021). doi: 10.1109/ConfTELE50222.2021.9435481
15. Sieverdes JC, Treiber FA, Kline CE, Mueller M, Brunner-Jackson B, Sox L, et al. Ethnicity differences in sleep changes among prehypertensive adults using a smartphone meditation app: dose-response trial. JMIR Form Res. (2020) 4:20501. doi: 10.2196/20501
16. Ong AA, Gillespie MB. Overview of smartphone applications for sleep analysis. World J Otorhinolaryngol Head Neck Surg. (2016) 2:45–9. doi: 10.1016/j.wjorl.2016.02.001
17. Koffel E, Kuhn E, Petsoulis N, Erbes CR, Anders S, Hoffman JE, et al. A randomized controlled pilot study of CBT-I coach: feasibility, acceptability, and potential impact of a mobile phone application for patients in cognitive behavioral therapy for insomnia. Health Informatics J. (2018) 24:3–13. doi: 10.1177/1460458216656472
18. Kuhn E, Weiss BJ, Taylor KL, Hoffman JE, Ramsey KM, Manber R, et al. CBT-I coach: a description and clinician perceptions of a mobile app for cognitive behavioral therapy for insomnia. J Clin Sleep Med. (2016) 12:597–606. doi: 10.5664/jcsm.5700
19. Reilly ED, Robinson SA, Petrakis BA, Kuhn E, Pigeon WR, Wiener RS, et al. Mobile app use for insomnia self-management: pilot findings on sleep outcomes in veterans. Interact J Med Res. (2019) 8:e12408. doi: 10.2196/12408
20. Aji M, Glozier N, Bartlett D, Peters D, Calvo RA, Zheng Y, et al. A feasibility study of a mobile app to treat insomnia. Transl Behav Med. (2021) 11:604–12. doi: 10.1093/tbm/ibaa019
21. Chung K, Kim S, Lee E, Park JY. Mobile app use for insomnia self-management in urban community-dwelling older Korean adults: retrospective intervention study. JMIR mHealth and uHealth. (2020) 8:17755. doi: 10.2196/17755
22. Kuhn E, Miller KE, Puran D, Wielgosz J, Williams SLY, Owen JE, et al. A pilot randomized controlled trial of the insomnia coach mobile app to assess its feasibility, acceptability, and potential efficacy. Behav Ther. (2022) 53:440–57. doi: 10.1016/j.beth.2021.11.003
23. Philip P, Dupuy L, Sagaspe P, de Sevin E, Auriacombe M, Taillard J, et al. Efficacy of a smartphone-based virtual companion to treat insomniac complaints in the general population: sleep diary monitoring versus an internet autonomous intervention. J Clin Med. (2022) 11:4387. doi: 10.3390/jcm11154387
24. Kolla BP, Mansukhani S, Mansukhani MP. Consumer sleep tracking devices: a review of mechanisms, validity and utility. Expert Rev Med Devices. (2016) 13:497–506. doi: 10.1586/17434440.2016.1171708
25. Scott H, Lack L, Lovato N. A pilot study of a novel smartphone application for the estimation of sleep onset. J Sleep Res. (2018) 27:90–7. doi: 10.1111/jsr.12575
26. Tal A, Shinar Z, Shaki D, Codish S, Goldbart A. Validation of contact-free sleep monitoring device with comparison to polysomnography. J Clin Sleep Med. (2017) 13:517–22. doi: 10.5664/jcsm.6514
27. Shi C, Nourani M, Gupta G, Tamil L. Apnea MedAssist II: A smart phone based system for sleep apnea assessment. In: Proceedings of the 2013 IEEE International Conference on Bioinformatics and Biomedicine (Shanghai). (2013). p. 572–7.
28. Tseng M-H, Hsu H-C, Chang C-C, Ting H, Wu H-C, Tang P-H. Development of an intelligent app for obstructive sleep apnea prediction on android smartphone using data mining approach. In: 2012 9th International Conference on Ubiquitous Intelligence and Computing and 9th International Conference on Autonomic and Trusted Computing (Fukuoka). (2012). doi: 10.1109/UIC-ATC.2012.89
29. Behar J, Roebuck A, Shahid M, Daly J, Hallack A, Palmius N, et al. SleepAp: an automated obstructive sleep apnoea screening application for smartphones. IEEE J Biomed Health Inform. (2014) 19:325–31. doi: 10.1109/JBHI.2014.2307913
30. Tiron R, Lyon G, Kilroy H, Osman A, Kelly N, O'Mahony N, et al. Screening for obstructive sleep apnea with novel hybrid acoustic smartphone app technology. J Thorac Dis. (2020) 12:4476–95. doi: 10.21037/jtd-20-804
31. Mansukhani MP, Kolla BP. Apps and fitness trackers that measure sleep: are they useful. Cleve Clin J Med. (2017) 84:451–6. doi: 10.3949/ccjm.84a.15173
32. Fino E, Plazzi G, Filardi M, Marzocchi M, Pizza F, Vandi S, et al. (Not so) Smart sleep tracking through the phone: Findings from a polysomnography study testing the reliability of four sleep applications. J Sleep Res. (2020) 29:12935. doi: 10.1111/jsr.12935
33. Berry A, Drake RJ, Butcher I, Yung AR. Examining the feasibility, acceptability, validity and reliability of physical activity, sedentary behaviour and sleep measures in people with schizophrenia. Ment Health Phys Act. (2021) 21:100415. doi: 10.1016/j.mhpa.2021.100415
34. Kim K, Park D-Y, Song YJ, Han S, Kim HJ. Consumer-grade sleep trackers are still not up to par compared to polysomnography. Sleep Breath. (2021) 24:3–13. doi: 10.1007/s11325-021-02493-y
35. Martinez GJ, Mattingly SM, Young J, Faust L, Dey AK, Campbell AT, et al. Improved sleep detection through the fusion of phone agent and wearable data streams. In: 2020 IEEE International Conference on Pervasive Computing and Communications Workshops (PerCom Workshops) (Austin, TX) (2020). doi: 10.1109/PerComWorkshops48775.2020.9156211
36. Bhat S, Ferraris A, Gupta D, Mozafarian M, DeBari VA, Gushway-Henry N, et al. Is there a clinical role for smartphone sleep apps? comparison of sleep cycle detection by a smartphone application to polysomnography. J Clin Sleep Med. (2015) 11:709–15. doi: 10.5664/jcsm.4840
37. Leigh S, Ouyang J, Mimnagh C. Effective? engaging? secure? applying the ORCHA-24 framework to evaluate apps for chronic insomnia disorder. Evid Based Ment Health. (2017) 20:e20–e20. doi: 10.1136/eb-2017-102751
38. Lane ND, Lin M, Mohammod M, Yang X, Lu H, Cardone G, et al. Bewell: sensing sleep, physical activities and social interactions to promote wellbeing. Mob Netw Appl. (2014) 19:345–59. doi: 10.1007/s11036-013-0484-5
39. Luna D, Quispe M, Gonzalez Z, Alemrares A, Risk M, Otero C. User-centered design to develop clinical applications. Lit rev Stud Health Technol Inform. (2015) 216:967. doi: 10.3233/978-1-61499-564-7-967
40. McCurdie T, Taneva S, Casselman M, Yeung M, McDaniel C, Ho W, et al. mHealth consumer apps: the case for user-centered design. Biomed Instrum Technol. (2012) 46:49–56. doi: 10.2345/0899-8205-46.s2.49
41. Aji M, Gordon C, Peters D, Bartlett D, Calvo RA, Naqshbandi K, et al. Exploring user needs and preferences for mobile apps for sleep disturbance: mixed methods study. JMIR Mental Health. (2019) 6:e13895. doi: 10.2196/13895
42. Nguyen H. Personalizing a sleep health APP for college students using personality traits and chronotype. 2016 IEEE MIT Undergraduate Research Technology Conference (URTC) (Cambridge, MA). (2016). doi: 10.1109/URTC.2016.8284084
43. Grigsby-Toussaint DS, Shin JC, Reeves DM, Beattie A, Auguste E, Jean-Louis G. Sleep apps and behavioral constructs: a content analysis. Prev Med Rep. (2017) 6:126–9. doi: 10.1016/j.pmedr.2017.02.018
44. Antezana G, Venning A, Blake V, Smith D, Winsall M, Orlowski S, et al. An evaluation of behaviour change techniques in health and lifestyle mobile applications. Health Informatics J. (2020) 26:104–13. doi: 10.1177/1460458218813726
45. Quante M, Khandpur N, Kontos EZ, Bakker JP, Owens JA, Redline S. A qualitative assessment of the acceptability of smartphone applications for improving sleep behaviors in low-income and minority adolescents. Behav Sleep Med. (2019) 17:573–85. doi: 10.1080/15402002.2018.1432483
46. Cowie J, Bower JL, Gonzalez R, Alfano CA. Multimedia field test: digitalizing better sleep using the Sleepio Program. Cogn Behav Pract. (2018) 25:442–8. doi: 10.1016/j.cbpra.2017.09.005
47. Lee-Tobin PA, Ogeil RP, Savic M, Lubman DI. Rate my sleep: examining the information, function, and basis in empirical evidence within sleep applications for mobile devices. J Clin Sleep Med. (2017) 13:1349–54. doi: 10.5664/jcsm.6814
48. Yu JS, Kuhn E, Miller KE, Taylor K. Smartphone apps for insomnia: examining existing apps' usability and adherence to evidence-based principles for insomnia management. Transl Behav Med. (2019) 9:110–9. doi: 10.1093/tbm/iby014
49. Xu Z-f, Luo X, Shi J, Lai Y. Quality analysis of smart phone sleep apps in China: can apps be used to conveniently screen for obstructive sleep apnea at home? BMC Medical Inform Decis Mak. (2019) 19:1–8. doi: 10.1186/s12911-019-0916-7
50. Kalaiselvan V, Azman F, Cheng LK, Rahim FA. nocturnOWL: sleep-monitoring virtual pet mobile application. 2019 IEEE Conference on Open Systems (ICOS). (2019). doi: 10.1109/ICOS47562.2019.8975709
51. Hosszu A, Rosner D, Flaherty M. Sleep tracking apps' design choices: a review. 2019 22nd International Conference on Control Systems and Computer Science (CSCS) (2019). doi: 10.1109/CSCS.2019.00078
Keywords: mobile apps, human computer interaction, sleep disorder, sleep management, sleep apnea
Citation: Al Mahmud A, Wu J and Mubin O (2022) A scoping review of mobile apps for sleep management: User needs and design considerations. Front. Psychiatry 13:1037927. doi: 10.3389/fpsyt.2022.1037927
Received: 06 September 2022; Accepted: 28 September 2022;
Published: 18 October 2022.
Edited by:
Nina Christmann, Central Institute of Mental Health, GermanyReviewed by:
Soheila Saeedi, Hamadan University of Medical Sciences, IranCopyright © 2022 Al Mahmud, Wu and Mubin. This is an open-access article distributed under the terms of the Creative Commons Attribution License (CC BY). The use, distribution or reproduction in other forums is permitted, provided the original author(s) and the copyright owner(s) are credited and that the original publication in this journal is cited, in accordance with accepted academic practice. No use, distribution or reproduction is permitted which does not comply with these terms.
*Correspondence: Abdullah Al Mahmud, YWFsbWFobXVkQHN3aW4uZWR1LmF1; Omar Mubin, by5tdWJpbkB3ZXN0ZXJuc3lkbmV5LmVkdS5hdQ==
Disclaimer: All claims expressed in this article are solely those of the authors and do not necessarily represent those of their affiliated organizations, or those of the publisher, the editors and the reviewers. Any product that may be evaluated in this article or claim that may be made by its manufacturer is not guaranteed or endorsed by the publisher.
Research integrity at Frontiers
Learn more about the work of our research integrity team to safeguard the quality of each article we publish.