- 1The Affiliated Cancer Hospital of Zhengzhou University, Henan Cancer Hospital, Zhengzhou, China
- 2Henan Engineering Research Center for Tumor Precision Medicine and Comprehensive Evaluation, Henan Cancer Hospital, Zhengzhou, China
- 3Henan Provincial Key Laboratory of Anticancer Drug Research, Henan Cancer Hospital, Zhengzhou, China
- 4Department of Magnetic Resonance Imaging, The First Affiliated Hospital of Zhengzhou University, Zhengzhou, China
- 5Department of Breast Disease, Henan Breast Cancer Center, The Affiliated Cancer Hospital of Zhengzhou University, Henan Cancer Hospital, Zhengzhou, China
- 6Nanyang Institute of Technology, Nanyang, China
As a highly heterogeneous disorder, schizophrenia shows notable interindividual variation in clinical manifestations. On that account, an increasing number of studies begin to examine the interindividual variability in neuroimaging characterization in schizophrenia. However, whether schizophrenia demonstrates higher interindividual morphological variability than health controls (HCs) remains unknown. T1-weighted anatomical images were obtained from patients with schizophrenia (n = 61) and matched HCs (n = 73). For each subject, voxel-wise gray matter volume was obtained using voxel-based morphometry analysis. We first inquired whether patients with schizophrenia showed higher interindividual structural variation than HCs using the person based similarity index (PBSI). Then, we examined differences of voxel-wise morphological coefficient of variation (CV) between schizophrenia and HCs. To further associate identified regions showing higher variability in schizophrenia with cognitive/functional processes, functional annotation was performed. Patients with schizophrenia exhibited lower PBSIs than matched HCs, suggesting higher interindividual morphological variability in schizophrenia. The following results showed that patients with schizophrenia exhibited higher CVs than HCs in distributed brain regions including the striatum, hippocampus, thalamus, parahippocampa gyrus, frontal gyrus, and amygdala. Brain regions showing higher CVs in schizophrenia were significantly implicated in affective, incentive and reward related terms. These results provide a new insight into the high clinical heterogeneity and facilitate personalized diagnose and treatment in schizophrenia.
Introduction
As one of common mentaldisorders, schizophrenia affects more than 20 million people worldwide (1). Although many attempts have been done, the etiology of schizophrenia remains unknown. One of reasons is that schizophrenia is a highly heterogeneous disorder, reflected in diverse clinical presentations (2), complex genetic architectures (3), and various treatment responses (4). The heterogeneity hampers uncovering pathophysiology and discovering validated biomarkers indicative of precision diagnosis and treatment (5) in schizophrenia. Although researchers have consistently found structural aberrance in distributed brain regions with help of modern neuroimaging tools like MRI (6). The interindividual heterogeneity usually leads to discordant findings (7). An increasing number of studies begin to focus on the interindividual heterogeneity in schizophrenia.
The notion that schizophrenia is a heterogeneous disorder is widely accepted and patients with schizophrenia demonstrate considerable interindividual variation in terms of clinical characteristics and neuroimaging abnormalities. For example, although patients with schizophrenia are usually reported to have deficits in intellectual decline after illness onset, there is a fair proportion of patients do not (8). Distinct subtypes of schizophrenia usually demonstrate different or even totally opposite patterns of structural/functional abnormalities (7, 9–11). The heterogeneity is thought to be one of the leading causes that lead to conflicting findings in neuroimaging studies (12, 13). Recently, Brugger and Howes (6) perform a meta-analysis on 108 studies and find that patients with schizophrenia show significantly greater interindividual variation in the volumes of disturbed brain regions including the thalamus, putamen and temporal gyrus than healthy controls. One further research reveals that the high interindividual variation in brain structure is associated with polygenic risk in schizophrenia (14). After these studies, Sun et al. (5) reveal higher interindividual variability of functional connectome in schizophrenia. These results shed lights on the heterogeneity and provide new insights into the high clinical heterogeneity and facilitate personalized clinical decision in schizophrenia. However, whether patients with schizophrenia demonstrate higher interindividual morphological variability than health normal is still unknown.
In this study, we aimed to investigate interindividual morphological variation in schizophrenia. Sixty one patients with schizophrenia and matched healthy controls (HCs, n = 73) were included. Voxel-based morphometry analysis (VBM) (15) was performed to obtain voxel-wise gray matter volume for each subject from T1-weighted anatomical image. We first inquired whether schizophrenia showed higher interindividual morphological variation than HCs. Considering the multifarious clinical characteristics and findings of previous studies, we hypothesized that schizophrenia would show higher interindividual morphological variation than matched HCs. Then, we calculated voxel-wise morphological coefficient of variation (CV) measuring the degree of dispersion for each group (16) and obtained voxel-wise differences of interindividual morphological variability between schizophrenia and HCs. Finally, we performed functional annotation to associate identified regions showing higher variability in patients.
Materials and methods
Sample
All subjects (both patients with schizophrenia and HCs) come from the Centers of Biomedical Research Excellence available on the Internet1 (17). This dataset included 61 patients with schizophrenia and matched 73 HCs. Patients with schizophrenia were diagnosed with the Diagnostic and Statistical Manual of Mental Disorders (DSM-IV, Fourth Edition) for schizophrenia. All patients were on antipsychotic medications and no medication changes in 1 month. None of the patients had a history of neurological disorder, active substance dependence or abuse (except for nicotine) within the past year. Seventy-three healthy controls matched for sex and age were also recruited. All HCs completed a structured clinical interview for DSM-IV axis I disorders-non-patient edition to rule out axis I conditions. The exclusion criteria of HCs included: (1) current or past psychiatric disorder, (2) family history of a psychotic disorder in a first-degree relative, (3) history of more than one lifetime depressive episode, (4) history of depression or antidepressant use within the last 6 months, and (5) history of lifetime antidepressant use for more than 1 year, (6) recent history of substance abuse or dependence, (7) head trauma with a loss of consciousness greater than 5 min. In addition, all subjects refrained from smoking for at least 1 h prior to scanning.
This study was approved by Human Subjects Research Review Committee. Informed consent was obtained from the subjects according to the institutional guidelines at the University of New Mexico.
Data acquisition
T1-weighted anatomical images of all participants were acquired using Siemens Trio scanner. The main scanning parameters were as followed: echo time = 1.64 ms, repetition time = 2,530 ms, field of view = 256 mm, resolution matrix = 256 × 256, inversion time = 1,200 ms, flip angle = 7 degrees, thickness = 1.0 mm, and voxel size = 1 × 1 × 1 mm3.
Voxel based morphometry analysis
All scans were processed using the CAT12 toolbox.2 The standard pipeline steps were adopted mainly including bias-field correction, segmentation gray and white matter and cerebrospinal fluid, adjustment for partial volume effects, normalization into Montreal Neurological Institute space, resampled to 1.5 mm × 1.5 mm × 1.5 mm and non-linear modulation (18–20). Finally, the gray matter (GM) maps were smoothed using 6 mm full width at half maximum Gaussian kernel (FWHM) (21).
Calculation of the person based similarity index
First, we inquired whether schizophrenia showed higher interindividual morphological variation than HCs by comparing the person based similarity indexes (PBSIs) between them. PBSIs measured the degree of structural similarity between subjects where the higher PBSIs suggested higher similarity in one group (16). There were two main steps to obtain the PBSI. First, the spatial Spearman’s correlations of voxel-wise morphometry between subject k with other subjects in one group were calculated. This step would yield n-1 coefficients for each subject where n is the total number of subjects in the group. Second, averaging the n-1 coefficients to generate the PBSI score for subject k Figure 1. We compared PSBIs between patients with schizophrenia and HCs using two sample t-test controlling age and sex.
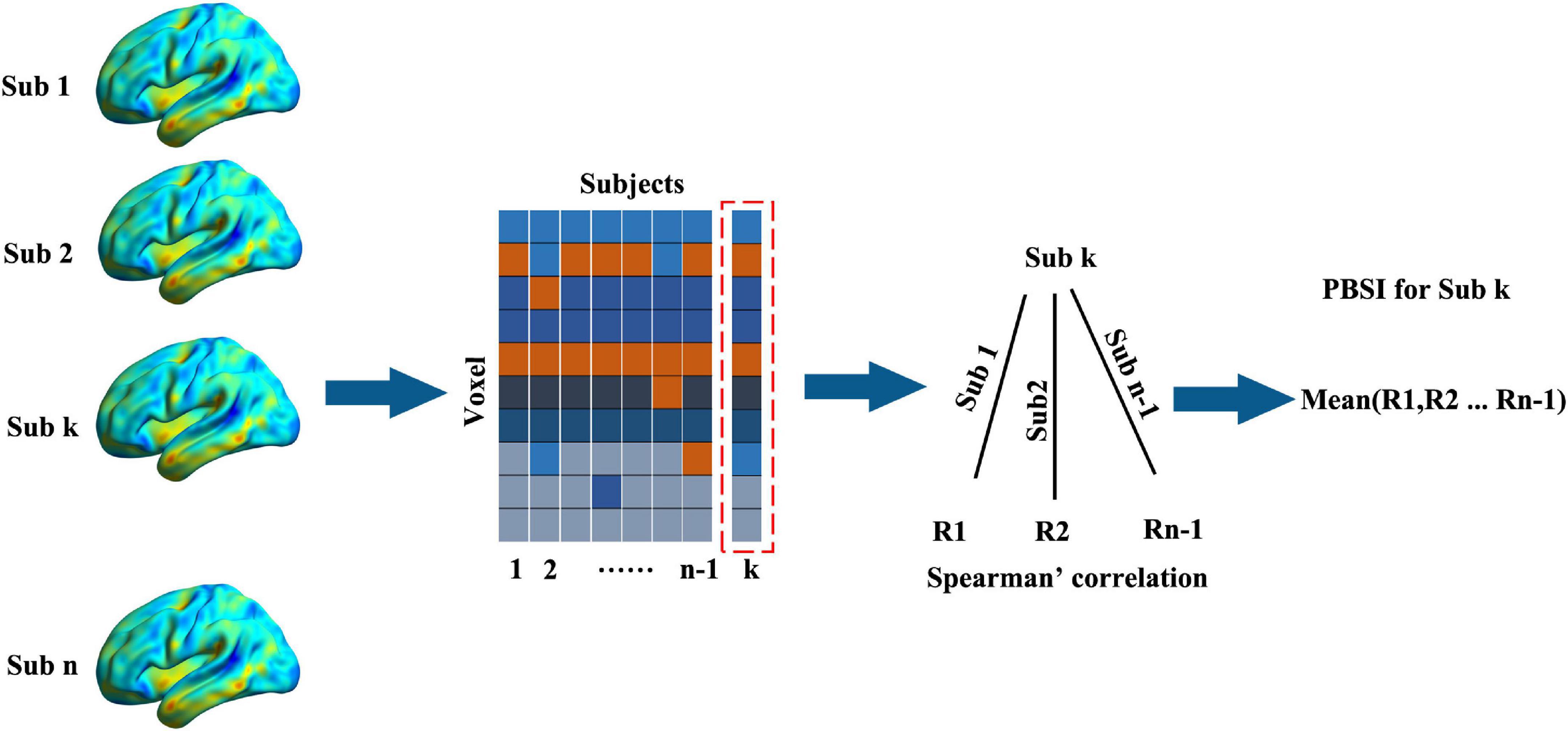
Figure 1. The work flow of calculating the person-based similarity index. The voxel-wise gray matter volumes were concatenated into vectors (voxel × subjects) for one group (the number of subjects was n). For subject k (sub k), the person-based similarity index (PBSI) was the mean Spearman’s correlations (the number was n-1) between it with other subjects.
Variability of voxel-wise morphometry
The coefficient of variation (CV) for voxel-wise gray matter volume (GMV) was obtained for each group separately according to the following formula: CV = SD/Mean. Mean and SD denoted the mean and the standard deviation of voxel-wise GMVs across subjects in each group. The difference significance was evaluated using the asymptotic test, yielding the statistic value (D) and its p-value (22). This procedure was done using cvequality in R-cran. The results were controlled for false positive with p < 0.05 (FDR corrected).
Functional annotation
To associate identified regions showing higher variability in schizophrenia with cognitive/functional processes, functional annotation was performed using brain annotation toolbox (BAT3) (23). This procedure used 217 cognitive/functional terms boring clear biological significance (24), that were available on Neurosynth4 (25). The significance was determined using permutation test (1000 times) (23) and the permutation p-values were corrected for FDR (p < 0.05).
Results
Demographic information
The demographic information of patients with schizophrenia and HCs was listed in Table 1. More details Information of PANSS was showed in Mayer et al. (17). Patient with schizophrenia exhibited no significant difference with HCs with regards to age and gender.
Lower person based similarity index in schizophrenia
As we hypothesized, schizophrenia exhibited significant lower PBSIs than HCs (t =−9.28, p < 0.001, cohen’s d =−1.61) Figure 2. These results suggested that patients with schizophrenia exhibited higher morphological heterogeneity than that in matched HCs.
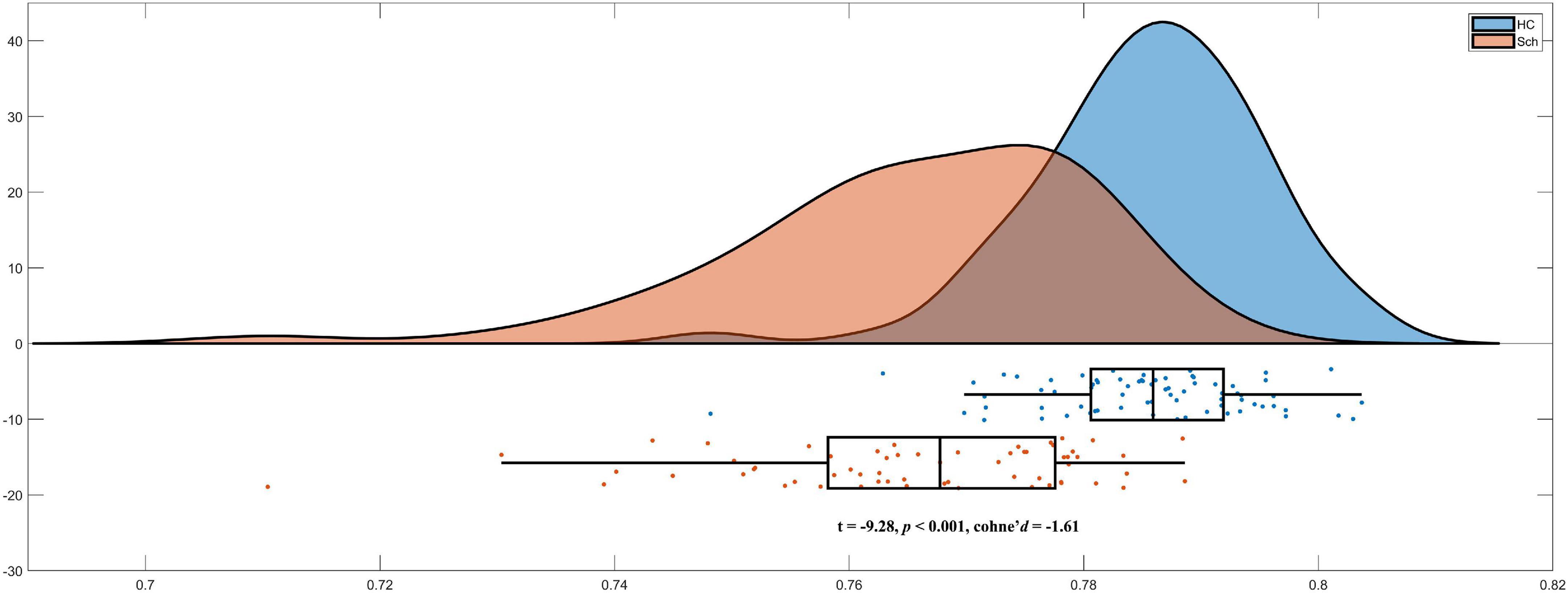
Figure 2. Lower person-based similarity indexes (PBSIs) in schizophrenia compared with healthy controls (HCs).
Voxel-wise morphological variability in schizophrenia
Then, we further investigated difference between schizophrenia and HCs in variability of voxel-wise morphometry. Patients with schizophrenia exhibited significant higher CVs (p < 0.05, FDR corrected) in distributed brain regions including the striatum, hippocampus, thalamus, parahippocampa gyrus, frontal gyrus and amygdala (Figure 3 and Table 2).
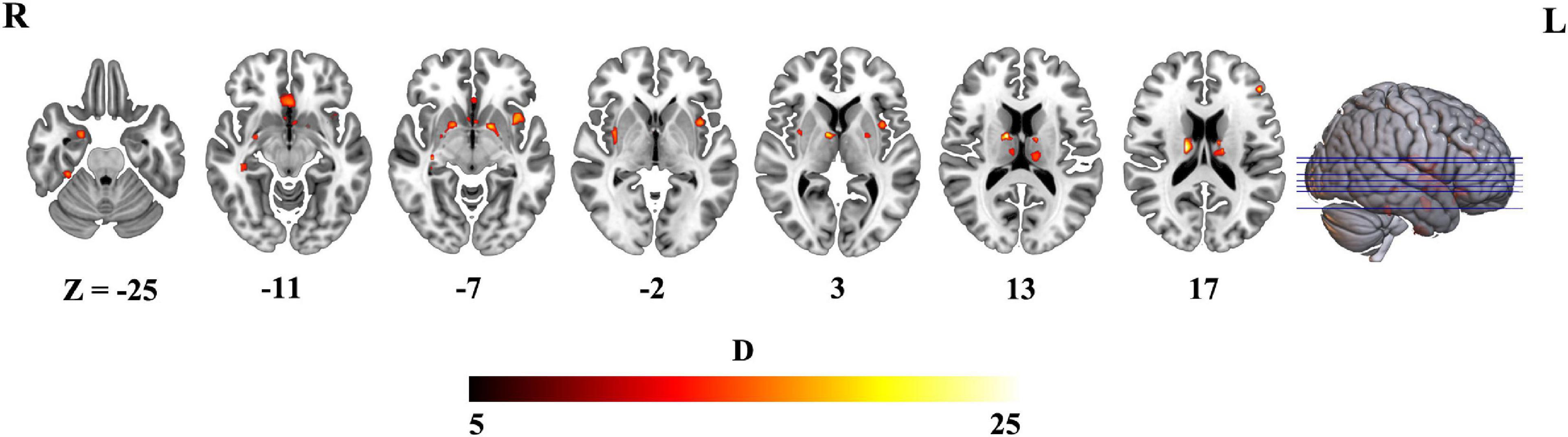
Figure 3. Brain regions exhibiting higher the coefficient of variation (CV) in schizophrenia (p < 0.05, FDR corrected).
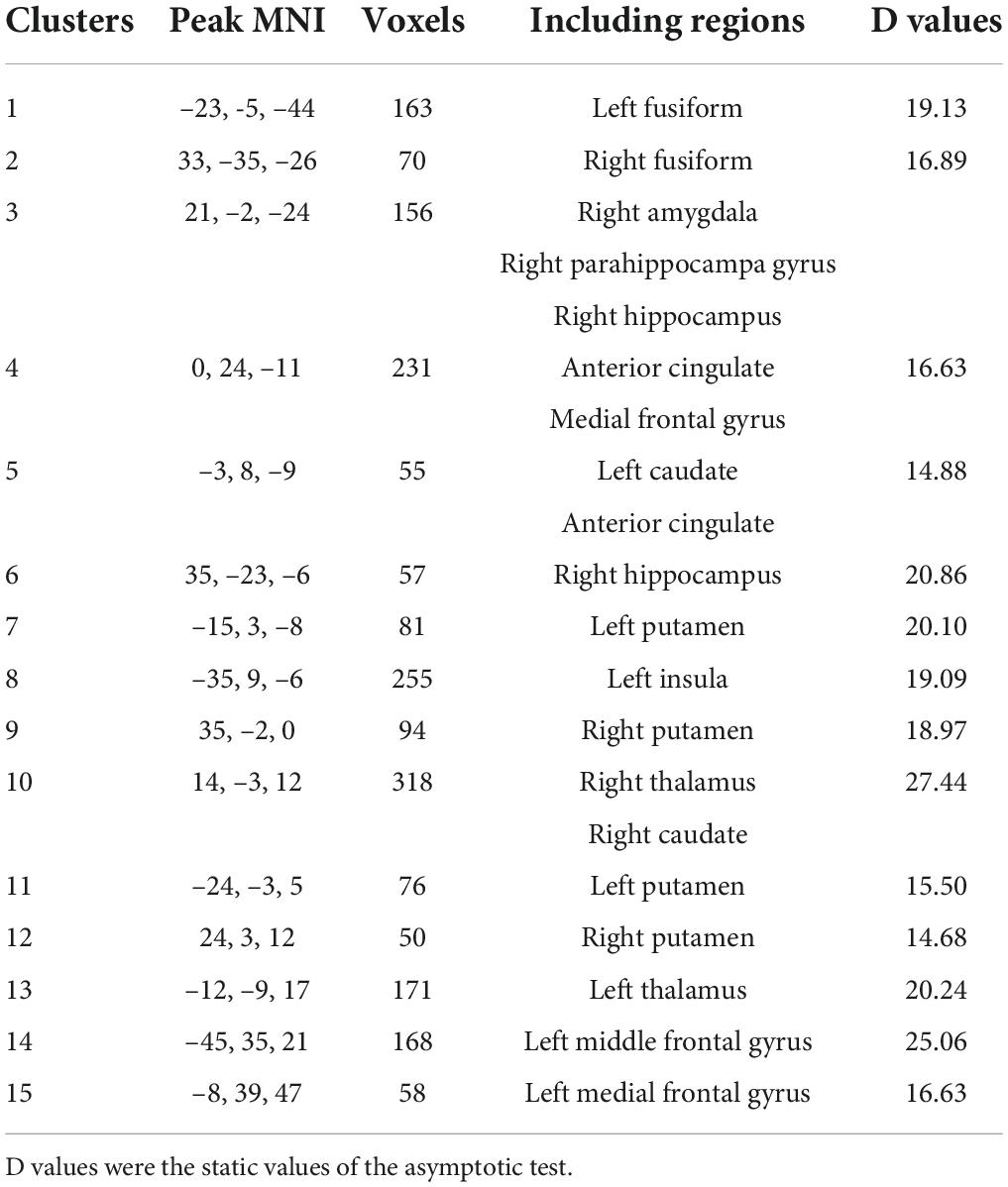
Table 2. Brain regions exhibiting higher coefficient of variation (CV) in schizophrenia (p < 0.05, FDR corrected).
Functional annotation results
We further performed functional annotation to associate identified brain regions and cognitive/functional processes. Brain regions showing higher CVs in schizophrenia were significantly associated with 14 cognitive/functional processes (permutation p < 0.05, FDR corrected), such as affective, incentive and reward related terms (details were included in Table 3).
Discussion
In this study, we investigated voxel-wise morphological heterogeneity measured with CV in schizophrenia. As we expected, we found significant lower PBSIs in patients with schizophrenia than matched HCs suggesting higher interindividual morphological heterogeneity in schizophrenia. Then, we identified that patients with schizophrenia exhibited higher CVs than HCs in distributed reward-related brain regions. The following functional annotation results suggested that these brain regions were significantly implicated in cognitive/functional processes, such as affective, incentive and reward related terms. These results provided new insights into the heterogeneity of schizophrenia.
The notion that schizophrenia was a highly heterogeneous disorder was increasingly accepted and the heterogeneity was expressed in complex genetic architectures, multifarious phenotypic expressions (3) and variable treatment responses (26). Accordingly, patients with schizophrenia were found to exhibit tremendous interindividual heterogeneity in neuroimaging representations. In a recent meta-analysis, the authors systematically reviewed 108 studies and found that patients with schizophrenia showed higher variability in the volume of distributed brain regions including the amygdala, thalamus and putamen than healthy controls (6). After this study, a series of studies emerged to investigate functional and structural heterogeneity in schizophrenia. For example, Sun et al. (5) identified higher inter-subject variability of the functional connectome in patients with schizophrenia and that the functional variability was associated with clinical heterogeneity. Antoniades et al. (16) found that both patients with schizophrenia and clinical high-risk individuals demonstrated higher brain morphological variation than normal persons. The greater variation aligning with neurobiological heterogeneity was representative of the core feature of the disorder and reflected gene-environment interactions (14). In accordance with these studies, we observed lower PBSIs indicating greater dissimilarity in voxel-wise morphological profiles in schizophrenia. The greater dissimilarity might not be resulted from factors such as medication exposure (16) and suggested that higher interindividual morphological variability was inherent in schizophrenia.
We further identified that brain regions encompassing the striatum, hippocampus, thalamus and parahippocampa gyrus, frontal gyrus, and amygdala exhibited higher CVs in patients than HCs. The higher structural and functional heterogeneity were consistently found in previous studies (5, 6, 14). The higher morphological variation in these regions explained discordant findings in these regions and reflected heterogeneity indicative of putative subtypes. Chand et al. (7) uncovered two subtypes of schizophrenia using a novel semi-supervised machine learning method. These two subtypes exhibited exactly opposite morphological differences in widespread brain regions including brain regions we reported. Consistent with these studies, we observed higher CVs in reward-related brain regions in schizophrenia, reflecting various deficits in reward processing in schizophrenia (27). Notably, Antoniades et al. (16) did not observed significant different in terms of CVs in regional volume between patients with schizophrenia and healthy controls. The reason might be different methodologies used in these two studies. Antoniades et al. (16) measured CVs at regional measures while we focused on voxel-wise CV aberrance. This could be tested in the future. The functional annotation results further revealed that the interindividual morphological variation was related to multifarious reward related deficits in schizophrenia (27).
There were some limitations should be mentioned. First, our results were obtained from one single dataset, future studies could use another independent dataset to confirm our results. Second, we did not have enough clinical data, such as cognitive performance and behavior scores, to establish a relationship between the interindividual variations with clinical characteristics. Third, factors such as cigarette and alcohol were not controlled in this study. Future studies should examine their effects on the morphological variation.
Conclusion
In this study, we examined the voxel-wise morphological variation in schizophrenia and matched HCs. Patients with schizophrenia exhibited higher interindividual variation than matched HCs. Further analysis showed that schizophrenia demonstrated distributed brain regions had higher morphological CVs than HCs. In addition, the high interindividual variation in these regions was related to multifarious reward related deficits in schizophrenia. Our results provided new insights into the high clinical heterogeneity and facilitated personalized diagnose and treatment in schizophrenia.
Data availability statement
The original contributions presented in this study are included in the article/supplementary material, further inquiries can be directed to the corresponding author.
Ethics statement
The studies involving human participants were reviewed and approved by Human Subjects Research Review Committee. The patients/participants provided their written informed consent to participate in this study.
Author contributions
KF and WZ designed the study. BaW and LN collected the data. KF, BaW, and BoW analyzed the data and drafted the work. LN and WZ revised the draft. All authors contributed to the article and approved the submitted version.
Funding
This research study was supported by the soft science project of medical science and technology of Henan province (RKX202002011), Medical Science and Technology Project of Henan Province (LHGJ20210196), and Henan Province Key R&D and Promotion Project (222102310317).
Conflict of interest
The authors declare that the research was conducted in the absence of any commercial or financial relationships that could be construed as a potential conflict of interest.
Publisher’s note
All claims expressed in this article are solely those of the authors and do not necessarily represent those of their affiliated organizations, or those of the publisher, the editors and the reviewers. Any product that may be evaluated in this article, or claim that may be made by its manufacturer, is not guaranteed or endorsed by the publisher.
Footnotes
- ^ http://fcon_1000.projects.nitrc.org/indi/retro/cobre.html
- ^ http://dbm.neuro.uni-jena.de/cat12/
- ^ http://123.56.224.61/softwares
- ^ https://neurosynth.org/
References
1. Gbd 2017 Disease and Injury Incidence and Prevalence Collaborators. Global, regional, and national incidence, prevalence, and years lived with disability for 354 diseases and injuries for 195 countries and territories, 1990-2017: a systematic analysis for the global burden of disease study 2017. Lancet. (2018) 392:1789–858. doi: 10.1016/S0140-6736(18)32279-7
2. Owen MJ, Sawa A, Mortensen PB. Schizophrenia. Lancet. (2016) 388:86–97. doi: 10.1016/S0140-6736(15)01121-6
3. Rodriguez-Murillo L, Gogos JA, Karayiorgou M. The genetic architecture of schizophrenia: new mutations and emerging paradigms. Ann Rev Med. (2012) 63:63–80. doi: 10.1146/annurev-med-072010-091100
4. Leucht S, Tardy M, Komossa K, Heres S, Kissling W, Salanti G, et al. Antipsychotic drugs versus placebo for relapse prevention in schizophrenia: a systematic review and meta-analysis. Lancet. (2012) 379:2063–71. doi: 10.1016/S0140-6736(12)60239-6
5. Sun X, Liu J, Ma Q, Duan J, Wang X, Xu Y, et al. Disrupted intersubject variability architecture in functional connectomes in schizophrenia. Schizophr Bull. (2021) 47:837–48. doi: 10.1093/schbul/sbaa155
6. Brugger SP, Howes OD. Heterogeneity and homogeneity of regional brain structure in schizophrenia: a meta-analysis. JAMA Psychiatry. (2017) 74:1104–11. doi: 10.1001/jamapsychiatry.2017.2663
7. Chand GB, Dwyer DB, Erus G, Sotiras A, Varol E, Srinivasan D, et al. Two distinct neuroanatomical subtypes of schizophrenia revealed using machine learning. Brain. (2020) 143:1027–38. doi: 10.1093/brain/awaa025
8. Weickert TW, Goldberg TE, Gold JM, Bigelow LB, Egan MF, Weinberger DR. Cognitive impairments in patients with schizophrenia displaying preserved and compromised intellect. Arch Gen Psychiatry. (2000) 57:907–13. doi: 10.1001/archpsyc.57.9.907
9. Voineskos AN, Foussias G, Lerch J, Felsky D, Remington G, Rajji TK, et al. Neuroimaging evidence for the deficit subtype of schizophrenia. JAMA Psychiatry. (2013) 70:472–80. doi: 10.1001/jamapsychiatry.2013.786
10. Lim K, Smucny J, Barch DM, Lam M, Keefe RSE, Lee J. Cognitive subtyping in schizophrenia: a latent profile analysis. Schizophr Bull. (2021) 47:712–21. doi: 10.1093/schbul/sbaa157
11. Han S, Xu Y, Guo HR, Fang K, Wei Y, Liu L, et al. Two distinct subtypes of obsessive compulsive disorder revealed by heterogeneity through discriminative analysis. Hum Brain Mapp. (2022) 43:3037–46. doi: 10.1002/hbm.25833
12. Saxena S, Rauch SL. Functional neuroimaging and the neuroanatomy of obsessive-compulsive disorder. Psychiatr Clin North Am. (2000) 23:563–86. doi: 10.1016/S0193-953X(05)70181-7
13. Boedhoe PSW, Schmaal L, Abe Y, Alonso P, Ameis SH, Anticevic A, et al. Cortical abnormalities associated with pediatric and adult obsessive-compulsive disorder: findings from the ENIGMA obsessive-compulsive disorder working group. Am J Psychiatry. (2018) 175:453–62. doi: 10.1176/appi.ajp.2017.17050485
14. Alnæs D, Kaufmann T, van der Meer D, Córdova-Palomera A, Rokicki J, Moberget T, et al. Brain heterogeneity in schizophrenia and its association with polygenic risk. JAMA Psychiatry. (2019) 76:739–48. doi: 10.1001/jamapsychiatry.2019.0257
15. Ashburner J, Friston KJ. Voxel-based morphometry–the methods. Neuroimage. (2000) 11:805–21. doi: 10.1006/nimg.2000.0582
16. Antoniades M, Haas SS, Modabbernia A, Bykowsky O, Frangou S, Borgwardt S, et al. Personalized estimates of brain structural variability in individuals with early psychosis. Schizophr Bull. (2021) 47:1029–38. doi: 10.1093/schbul/sbab005
17. Mayer AR, Ruhl D, Merideth F, Ling J, Hanlon FM, Bustillo J, et al. Functional imaging of the hemodynamic sensory gating response in schizophrenia. Hum Brain Mapp. (2013) 34:2302–12. doi: 10.1002/hbm.22065
18. Ashburner J. Computational anatomy with the SPM software. Magn Reson Imaging. (2009) 27:1163–74. doi: 10.1016/j.mri.2009.01.006
19. Han S, Xu Y, Guo HR, Fang K, Wei Y, Liu L, et al. Resolving heterogeneity in obsessive-compulsive disorder through individualized differential structural covariance network analysis. Cereb Cortex. (2022). doi: 10.1093/cercor/bhac163 [Epub ahead of print].
20. Fang K, Han S, Li Y, Ding J, Wu J, Zhang W. The vital role of central executive network in brain age: evidence from machine learning and transcriptional signatures. Front Neurosci. (2021) 15:733316. doi: 10.3389/fnins.2021.733316
21. Han S, Zheng R, Li S, Liu L, Wang C, Jiang Y, et al. Progressive brain structural abnormality in depression assessed with MR imaging by using causal network analysis. Psychol Med. (2021). doi: 10.1017/S0033291721003986 [Epub ahead of print].
22. Feltz CJ, Miller GE. An asymptotic test for the equality of coefficients of variation from k populations. Stat Med. (1996) 15:646–58. doi: 10.1002/(sici)1097-0258(19960330)15:6<647::aid-sim184>3.0.co;2-p
23. Liu Z, Rolls ET, Liu Z, Zhang K, Yang M, Du J, et al. Brain annotation toolbox: exploring the functional and genetic associations of neuroimaging results. Bioinformatics. (2019) 35:3771–8. doi: 10.1093/bioinformatics/btz128
24. Cheng W, Rolls ET, Zhang J, Sheng W, Ma L, Wan L, et al. Functional connectivity decreases in autism in emotion, self, and face circuits identified by knowledge-based enrichment analysis. Neuroimage. (2017) 148:169–78. doi: 10.1016/j.neuroimage.2016.12.068
25. Yarkoni T, Poldrack RA, Nichols TE, Van Essen DC, Wager TD. Large-scale automated synthesis of human functional neuroimaging data. Nat Methods. (2011) 8:665–70. doi: 10.1038/nmeth.1635
26. Malhotra AK. Dissecting the heterogeneity of treatment response in first-episode schizophrenia. Schizophr Bull. (2015) 41:1224–6. doi: 10.1093/schbul/sbv117
Keywords: schizophrenia, gray matter volume, heterogeneity, coefficient of variation, personalized
Citation: Fang K, Wen B, Niu L, Wan B and Zhang W (2022) Higher brain structural heterogeneity in schizophrenia. Front. Psychiatry 13:1017399. doi: 10.3389/fpsyt.2022.1017399
Received: 12 August 2022; Accepted: 26 August 2022;
Published: 23 September 2022.
Edited by:
Xiao Wang, Chengdu University of Traditional Chinese Medicine, ChinaReviewed by:
Changchun He, Chongqing University of Posts and Telecommunications, ChinaGuo Jing, University of Electronic Science and Technology of China, China
Copyright © 2022 Fang, Wen, Niu, Wan and Zhang. This is an open-access article distributed under the terms of the Creative Commons Attribution License (CC BY). The use, distribution or reproduction in other forums is permitted, provided the original author(s) and the copyright owner(s) are credited and that the original publication in this journal is cited, in accordance with accepted academic practice. No use, distribution or reproduction is permitted which does not comply with these terms.
*Correspondence: Wenzhou Zhang, hnzzzwz@hotmail.com