- 1Department of Psychiatry, Severance Hospital, Yonsei University College of Medicine, Seoul, South Korea
- 2Institute of Behavioral Science in Medicine, Yonsei University College of Medicine, Seoul, South Korea
- 3Institute for Innovation in Digital Healthcare, Yonsei University, Seoul, South Korea
- 4Department of Psychiatry, Yongin Severance Hospital, Yonsei University College of Medicine, Seoul, South Korea
The excessive use of smartphones is associated with various medical complications and mental health problems. However, existing research findings on neurobiological mechanisms behind problematic smartphone use are limited. In this study, we investigated functional connectivity in problematic smartphone users, focusing on the default mode network (DMN) and attentional networks. We hypothesized that problematic smartphone users would have alterations in functional connectivity between the DMN and attentional networks and that such alterations would correlate with the severity of problematic smartphone use. This study included 30 problematic smartphone users and 35 non-problematic smartphone users. We carried out group independent component analysis (group ICA) to decompose resting-state functional magnetic resonance imaging (fMRI) data into distinct networks. We examined functional connectivity using seed-to-seed analysis and identified the nodes of networks in group ICA, which we used as region of interest. We identified greater functional connectivity of the dorsal anterior cingulate cortex (dACC) with the ventral attention network (VAN) and with the DMN in problematic smartphone users. In seed-to-seed analysis, problematic smartphone users showed atypical dACC-VAN functional connectivity which correlated with the smartphone addiction proneness scale total scores. Our resting-state fMRI study found greater functional connectivity between the dACC and attentional networks in problematic smartphone users. Our findings suggest that increased bottom-up and interoceptive attentional processing might play an important role in problematic smartphone use.
Introduction
Smartphone use has increased greatly over the last decade, and has become an integral part of our daily lives. Whilst a smartphone has brought about great convenience, its excessive use has also brought many adverse health effects on its user (1–3). Problematic smartphone use has been associated with depression and anxiety (4). It has also been associated with psychological distress mediated by emotional dysregulation (5), with higher impulsivity and higher alcohol use disorder symptoms (6).
Recent studies have demonstrated similarities between excessive smartphone usage and behavioral addiction (7, 8). The Diagnostic and Statistical Manual of Mental Disorders-5 (DSM-5) diagnostic criteria for behavioral addiction are similar to that of substance use disorder and include symptoms of withdrawal and tolerance, continued use despite negative consequences and loss of control over the activity (9). Researchers observed that problematic smartphone use share common symptomatology with other behavioral addiction, leading to have a negative impact on functioning in occupational, social, and daily life (10). As Kardefelt-Winther et al. proposed, two important components in behavioral addictions are: (a) significant functional impairment or distress as a direct consequence of the behavior and (b) persistence over time. Accordingly, functional impairment from excessive smartphone use has been demonstrated through academic problems, interpersonal problems, impairment of cognitive function leading to reduced inhibitory control, impaired attention or in reduced numerical processing capacity (11–13).
Researchers have attempted to elucidate neurobiological mechanisms behind behavioral addictions. Between behavioral addiction and substance addiction, shared alteration in neurocircuitry, especially in the frontal and striatal regions has been demonstrated in neuroimaging studies (14–16). Mesolimbic pathway has been shown to be associated with both substance addiction and behavioral addiction (17, 18). Attentional control, which is a key factor in exerting executive function, has been associated with the severity of gambling in problem gamblers (19). Attentional control involves two systems, namely top-down factors and bottom-up factors. The dorsal attention network (DAN) is in charge of top-down attentional control and takes into account knowledge, expectation and current goals (20). The DAN includes the intraparietal sulcus and frontal eye field (20). The ventral attention network (VAN) consists of the ventral frontal cortex and temporoparietal junction and is in charge of bottom-up attentional control (21). Hypoconnectivity within the VAN has also been shown in subjects with internet addiction (22). Moreover, the default mode network (DMN) is a large-scale network that is activated during resting state but deactivated when performing task. The DMN comprises of the medial prefrontal cortex, posterior cingulate cortex and precuneus in the midline of the brain and the bilateral inferior parietal lobule (23). Altered functional connectivity in the DMN has been demonstrated in internet addiction and internet gaming disorder (24, 25).
There are a small number of studies that examine the neurobiological mechanisms behind problematic smartphone use. To consider a number of existing studies – structural magnetic resonance imaging (MRI) studies have demonstrated that problematic smartphone users exhibit smaller gray matter volume in the right orbitofrontal cortex (26). Hovarth et al. in their amplitude of low frequency fluctuation (ALFF) study, demonstrated that intrinsic activity in the right anterior cingulate cortex was negatively correlated with the smartphone addiction inventory (SPAI) (27). Subjects with smartphone addiction also showed significantly smaller gray matter volume in the insula compared to controls (27). Chun et al. in resting-state functional magnetic resonance imaging (fMRI) study using region of interest (ROI) to ROI analysis, observed that adolescents with excessive smartphone use showed lower functional connectivity between the orbitofrontal cortex and nucleus accumbens, and between the orbitofrontal cortex and middle cingulate cortex (28).
The majority of the existing functional connectivity studies on problematic smartphone users are based on a priori selection of ROIs, which may introduce potential bias and limit studies from having a global view of the brain connectivity (29). While it is still an open question to decide which method is superior, previous studies demonstrated that when researchers define ROI using brain atlas, the selected ROIs may not correspond to the real functional boundaries and that using data-driven independent component analysis (ICA) would be more appropriate (30).
In this study, we investigated alterations in functional connectivity in male problematic smartphone users by using group independent component analysis (group ICA), a data-driven technique, which can discover hidden factors from a set of measurements without a priori selection of ROIs (31), focusing on the DMN and attentional networks. We defined ROIs from group ICA results, as it may better correspond to the real functional boundaries for the data collected in our study (30). We hypothesized that problematic smartphone users would have alterations in functional connectivity in the DMN and in attention networks, and that such alterations will correlate with the severity of problematic smartphone use.
Materials and methods
Participants
A total of 65 male smartphone users participated in the study. As previous studies demonstrated gender differences in the neurobiology underlying addictions (32–36), and as brain imaging studies have shown structural and functional differences between male and female participants with addictive disorders (37), we have only gathered male participants, in an effort to collect a more homogenous sample, especially considering the small number of participants in our study.
We recruited participants from the local community through announcements, flyers or word of mouth. We administered the Structured Clinical Interview for DSM-5 to evaluate major psychiatric illness (38). The Korean version of the Wechsler Adult Intelligence Scale IV was administered on all participants to assess intelligence quotient (IQ) (39). Exclusion criteria were: participants with major psychiatric disorder, intellectual disability, neurological illness or contraindication to MRI. Participants included in the study had no history of receiving psychiatric treatment or taking psychiatric medication. Participants were divided into two groups: problematic smartphone users and non-problematic smartphone users.
Psychometric measures
Participants were assessed for excessive smartphone use using the Korean Smartphone Addiction Proneness Scale (SAPS) (40). The SAPS, developed by the Korean National Information Society Agency, is used to evaluate excessive smartphone use (it shows high reliability with Cronbach’s alpha of 0.880) (40). The scale consists of four subdomains: disturbance of adaptive functions, virtual life orientation, withdrawal and tolerance. The scale also consists of 15 items, rated by the 4-point Likert scale (1 = strongly disagree; 2 = disagree; 3 = agree; 4 = strongly agree) ranging from 15 to 60. Participants with a total SAPS score of ≥44 or disturbance of adaptive functions, withdrawal and tolerance subscale scores of ≥15, ≥13, and ≥13, respectively were classified as high-risk smartphone users. At-risk smartphone users were defined as participants with the SAPS score of 40–43 or disturbance of adaptive functions subscale score of ≥14. In our study, we classified both high-risk and at-risk smartphone users as problematic smartphone users, following what was proposed during the SAPS development (41), which is also in line with the classification criteria used in previous studies (26, 28). To examine internet addiction status, the Internet Addiction Test (IAT) was administered (42). To assess impulsivity, the Barratt Impulsiveness Scale (BIS) was administered (43). All subjects were assessed with the Beck Depression Inventory (BDI) (44), Beck Anxiety Inventory (BAI) (45), Alcohol Use Disorder Identification Test (AUDIT) (46), Pittsburgh Sleep Quality Index (PSQI) (47), Conners’ Adult ADHD Rating Scale-Short Version (CAARS) (48) and Wender Utah Rating Score-Short Version (WURS) (49).
Image acquisition and pre-processing
We used a 3T MRI scanner (Magnetom; Siemens, Munich, Germany) equipped with an eight-channel head coil to acquire brain MRI data. To acquire structured MRI data, we used T1-weighed spoiled gradient echo sequence (echo time = 2.19 ms, repetition time = 1,780 ms, flip angle = 9°, field of view = 256 mm × 256 mm, matrix = 256 × 256, transverse slice thickness = 1 mm). We obtained fMRI data through T2-weighed gradient echo planar pulse sequence (echo time = 30 ms, repetition time = 2,500 ms, flip angle = 90°, field of view = 240 mm × 240 mm, matrix = 64 × 64, slice thickness = 3.5 mm). T2-weighed sequence was used for this fMRI experiment because the transverse relaxation time (T2) is dependent on blood oxygenation level (50, 51). We instructed participants to fixate on a white cross on a black background for 6 min, without any motor, cognitive or lingual activities.
We used CONN-fMRI functional connectivity toolbox, version 20.b1 for preprocessing functional connectivity analysis. We applied CONN preprocessing pipeline. Using the Statistical Parametric Mapping (SPM12; Wellcome Trust Centre for Neuroimaging)2 realign and unwarp procedure (52), we realigned the functional data. We also applied unwarping and slice-timing correction.
We then ran Artifact Detection Tool (ART)-based automatic outlier detection. If functional volume signal intensity was outside five standard deviations from the mean, or if there was any evidence that they were displaced for more than 0.9 mm compared to the preceding volume, they were considered outliers. If we needed to censor more than 15% of frames for scrubbing, we excluded the relevant participant. All participants were included in the functional connectivity analysis as they did not meet the exclusion criteria. SPM12 unified segmentation and normalization procedure (53) was used to normalize functional and anatomical data into standard Montreal Neurological Institute (MNI) space and to segment them into gray matter, white matter and cerebrospinal fluid. In this process, we iteratively performed tissue classification, estimating the posterior tissue probability maps (TPMs) from the intensity values of the reference image and registration, estimating the non-linear spatial transformation best approximating posterior and prior TPMs until convergence. We re-sliced images to a 2-mm isotropic resolution. Images were smoothed with an 8-mm full-width at half maximum kernel.
Functional connectivity analysis
We obtained functional connectivity maps of seed-to-seed analysis using the CONN-fMRI functional connectivity toolbox version 20.b. For functional connectivity analysis, seed regions were selected from results of group ICA. The seed regions were selected from the largest clusters of each components of interest with peak z-values. ROIs were defined as a 6-mm radium sphere centered on coordinates acquired from spatial maps of targeted functional network.
Group independent component analysis
We carried out group ICA to investigate spatially independent network using group ICA of fMRI toolbox (GIFT ver 4.0c).3 Two stages of principal component analysis were performed to reduce the preprocessed data. Dimensionality estimation to determine the number of independent components from fMRI data was performed using the modified minimal description length criteria (54). This method has been widely used in ICA studies using fMRI data (55–57). We repeated ICA analysis 20 times and obtained 38 independent functional spatial maps for every participant.
We selected ICA components through stepwise estimation. Firstly, we used probabilistic maps of white matter, cerebrospinal fluid within standardized brain space in MNI template in SPM12 and correlated it with each component. Spatial correlation greater than r2 = 0.025 in both white matter and cerebrospinal fluid were considered artifacts and were therefore removed from the analysis (Supplementary Figures 4, 5). Secondly, we selected candidates of large-scale intrinsic networks, and remaining components were correlated with network atlas of the DMN (58). To analyze within and between group differences, we performed one-sample t-tests and two-sample t-tests using SPM12 with “SPM stat” function in the GIFT toolbox. We converted spatial maps of selected components to Z-values to assess correlations among components.
Statistical analysis
We performed independent t-test to compare demographic and clinical variables between problematic smartphone users and non-problematic smartphone users. We examined the relationship between clinical assessments and functional connectivity using the Pearson correlation analysis. The Statistical Package for the Social Sciences (SPSS), version 26.0 (SPSS Inc., Chicago, IL, USA) was used for statistical analysis with p-value < 0.05 (two-tailed).
Ethics
The Institutional Review Board at Severance Hospital, Yonsei University approved all protocols for this study. Written informed consent was obtained from all participants prior to participation.
Results
Demographic and clinical assessment
There were no differences between the two groups in age and in IQ. The SAPS score was significantly higher in problematic smartphone users. The total score in BIS did not show any significant difference between problematic smartphone users and non-problematic smartphone users, while non-planning impulsiveness subscale was higher in problematic smartphone users. The total score in CAARS did not differ between problematic smartphone users and non-problematic smartphone users, while hyperactivity, restlessness subscale score was higher in problematic smartphone users. The PSQI score was significantly higher in problematic smartphone users (Table 1).
Group independent component analysis
Among the 38 independent components, 7 components passed our selection criteria. The selected components showed a low correlation with white matter and cerebrospinal fluid, and were highly correlated with gray matter and pre-existing templates (58, 59) (Supplementary Figures 1, 2).
The DMN (IC 21) consists of posterior cingulate cortex, angular gyrus and medial prefrontal cortex. The VAN (IC 37) consists of inferior frontal gyrus and temporoparietal junction. The right DAN (IC 32) and the left DAN (IC 20) consist of intraparietal sulcus and frontal eye field. In two-sample t-test of the DMN and VAN, the left dorsal anterior cingulate cortex (dACC) was hyperactivated in problematic smartphone user group compared to non-problematic smartphone user group. We attempted to find out whether the activation of the dACC was associated with proneness to smartphone addiction by examining the correlation between the SAPS score and beta weights of the dACC ROI from the DMN and VAN. Beta weights of the left dACC ROI selected from the DMN showed a negative correlation with the SAPS score (Pearson’s r: −0.254, p-value = 0.041) (Figure 1A). Beta weights of the left dACC ROI from the VAN also showed a negative correlation with the SAPS score (Pearson’s r = −0.282, p-value = 0.023) (Figure 1B).
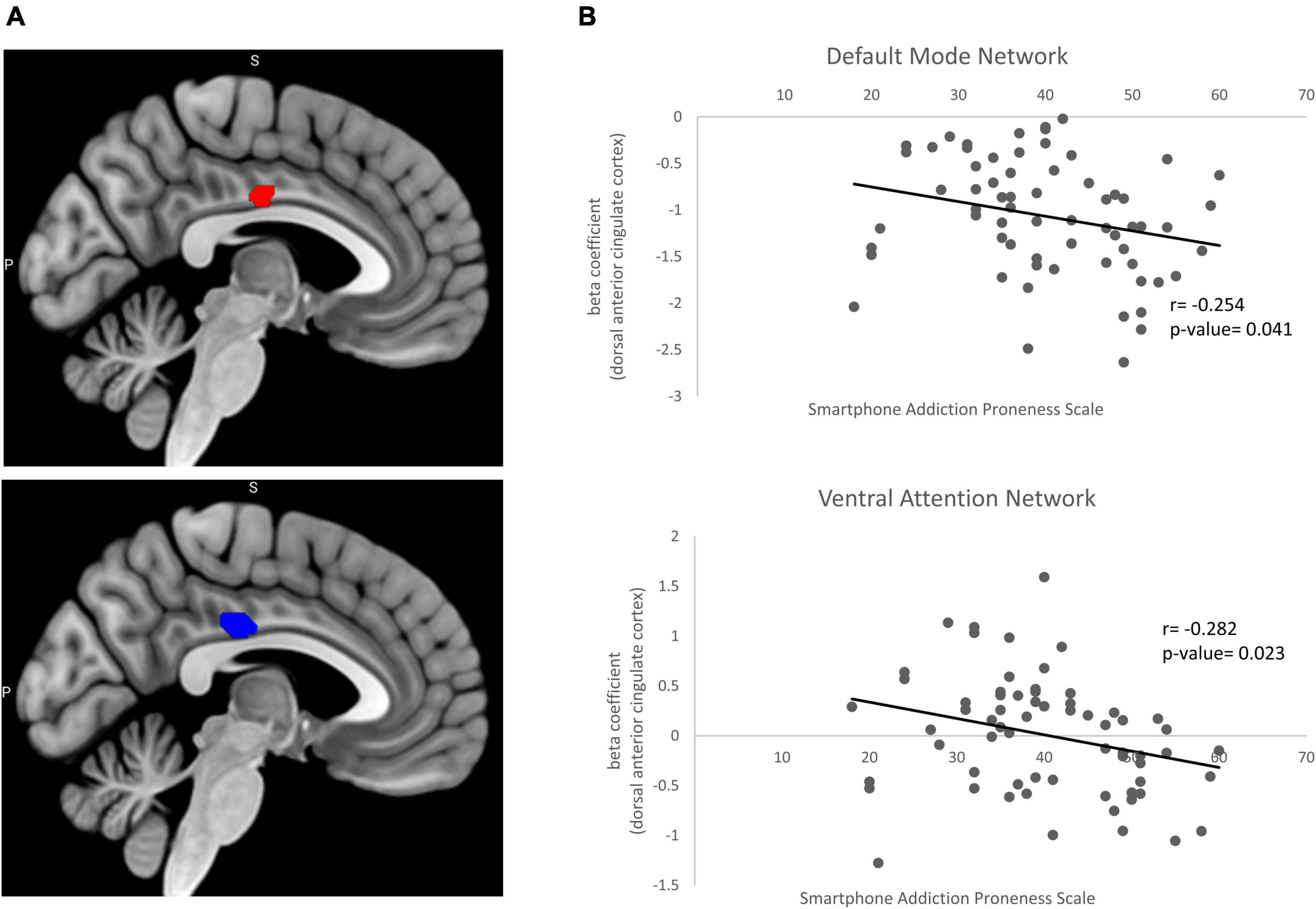
Figure 1. Beta coefficient from two sample t-test and correlation with clinical variable. (A) Significant increases in the resting state functional connectivity of the default mode network (red) and ventral attention network (blue) in problematic smartphone users. Problematic smartphone users displayed increased resting state functional connectivity in dorsal anterior cingulate cortex in both default mode network and ventral attention network. (B) Pearson correlation analysis for correlation of beta coefficient in dorsal anterior cingulate cortex and smartphone addiction proneness scale. In default mode network, beta coefficient in dorsal anterior cingulate cortex showed negative correlation (Pearsons’s r = –0.254, p-value = 0.041). In ventral attention network dorsal anterior cingulate cortex also showed negative correlation with smartphone addiction proneness scale (Pearson’s r = –0.282, p-value = 0.023).
Functional connectivity analysis
For seed-to-seed analysis, seeds were determined by the peak z-values in the spatial maps of independent components of interest. Posterior cingulate cortex (−6, −54, 16) was selected as the seed for the DMN. The left inferior frontal gyrus (−52, 20, 10), left temporoparietal junction (−56, −44, 32), right inferior frontal gyrus (40, 18, −6) and right temporoparietal junction (62, −36, 38) were selected as the seeds for the VAN. The left frontal eye field (−22, −6, 62) and right frontal eye field (24, 2, 64) were selected as the seeds for the DAN. When we used two-sample t-test to compare brain activation in the DMN (independent component 21) between problematic smartphone users and non-problematic smartphone users, we found that the dACC (−4, −16, 32) was hyperactivated in the problematic smartphone user group. This coordinate for the dACC (−4, −16, 32) was selected as a seed. In seed-to-seed analysis, problematic smartphone users showed a different connectivity pattern from the non-problematic smartphone users. For problematic smartphone users, the dACC showed additional functional connectivity with the left temporoparietal junction. Moreover, a negative connectivity from the posterior cingulate cortex to the frontal eye field (both left and right) was only observed in problematic smartphone users but not in non-problematic smartphone users (Figure 2A and Supplementary Figure 3).
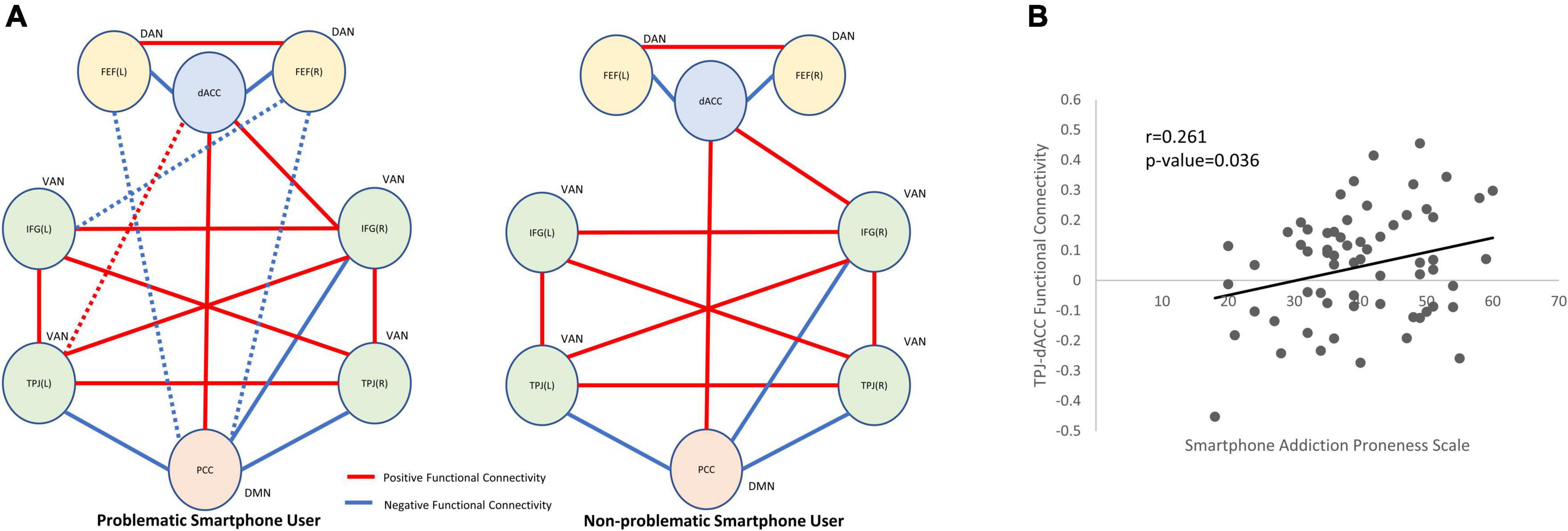
Figure 2. Between-network connectivity and functional connectivity correlation with clinical variables. (A) Seed-to-seed within-group analysis (red line: positive functional connectivity; blue line: negative functional connectivity). The statistical inferences were thresholded using a p-value < 0.05. In problematic smartphone users, functional connectivity of dACC to left TPJ was identified which were not observed in non-problematic smartphone users. Functional connectivity of PCC to FEF was found in problematic smartphone user group, which were not observed in non-problematic smartphone user group. (B) Pearson correlation analysis for clinical correlation of functional connectivity. We identified positive correlation between TPJ-dACC functional connectivity and smartphone addiction proneness scale (Pearson’s r = 0.261, p-value = 0.036). DAN, dorsal attention network; VAN, ventral attention network; DMN, default mode network; dACC, dorsal anterior cingulate cortex; IFG, inferior frontal gyrus; TPJ, temporoparietal junction, PCC, posterior cingulate cortex; FEF, frontal eye field.
Correlation between functional connectivity strength and psychometric measures
The functional connectivity strength between the dACC and left temporoparietal junction also correlated with the SAPS (Pearson’s r = 0.261, p-value = 0.036) (Figure 2B). There was no significant correlation between the dACC and temporoparietal junction functional connectivity strength and any psychometric measures.
Discussion
We identified aberrant functional connectivity of the dACC with the VAN and with the DMN in problematic smartphone users. Moreover, both functional connectivities were associated with proneness to smartphone addiction. In seed-to-seed analysis, problematic smartphone users showed atypical dACC functional connectivity with ROIs of the VAN which is associated with the SAPS total scores, suggesting the importance of excessive exposure to smartphone use on brain functional connectivity. Bearing in mind that the dACC is crucial in executive control process and that the VAN is crucial in bottom-up control of attention, our findings imply that alterations in attention to external stimuli mediated by the VAN may be associated with problematic smartphone use.
One of the important functions of the dACC has been considered to be self-control and persistence, which is associated with choosing an option that is in line with one’s long-term benefit (60, 61). The importance of the dACC in self-control has been demonstrated repeatedly in its association with successful self-control in response inhibition tasks (62), intertemporal choice tasks (63) and delay tasks (64). Furthermore, the dACC is also important in cognitive control and is involved with adjustment of behavior so that one’s behavior aligns with internally maintained goals and limiting behaviors that do not align with achieving such goals (65). This is consistent with the finding that activation of the dACC produce intense feelings of will power that allows one to persevere against challenges. One theory suggest that the dACC carries value signal, which allows for decision-makers to overcome a tendency to succumb to temptations (66). In addiction studies, aberration in the dACC was associated with problems in inhibitory control for drug addicts (67, 68). Considering the role of the dACC in cognitive control, the difference in functional connectivity between resting state networks, such as the VAN and DMN with the dACC may be related to problems in self-control and difficulties in avoiding behaviors, such as using of smartphones excessively even when such behavior interferes with the users’ daily lives negatively. More importantly, the dACC is an important hub of the salience network, modulating switch between the executive control network and DMN, allowing access to attention and working memory when salient stimuli is detected (69, 70). Therefore, change in functional connectivity to salience network via the dACC may interfere with appropriate detection and filtering of salient stimuli, such as smartphone, when using it does not align with one’s goal at the time of use.
The VAN is activated while reorienting to an unattended object that is behaviorally relevant, but is not activated when reorientation simply occurs without behavioral relevance (71). A previous study has shown that top-down signal from anterior cingulate which forms a putative task-control network may send top-down signal to the VAN, which may be deactivated when unimportant objects appear (72). Increased functional connectivity between the dACC and VAN may suggest aberration in bottom up process through the VAN in processing reorientation to external relevant stimuli. While the VAN works as a circuit breaker to interrupt signal to the DAN, thereby changing the locus of orientation, top down signal from the dACC restricts its activation only to relevant but unattended stimuli (73). Aberration in functional connectivity between the dACC and VAN may interfere with the VAN’s selective activation to relevant stimuli, which may lead to maladaptive and excessive reorientation to a smartphone even when it is not relevant to one’s current goal or task.
Increased functional connectivity between the dACC, a crucial node in salience network, with the DMN may suggest that more resource is allocated to the DMN to facilitate internal mental processes against cognitive control. Previous findings demonstrate that the salience network typically shows a positive correlation with the executive control network and a negative correlation with the DMN, which helps allocate attention to external vs. internal stimuli (70, 74). We found that the dACC activity was increased in the DMN of problematic smartphone users compared to non-problematic smartphone users. This suggest that increased resource was allocated to the DMN, which promote internal mental process, rather than cognitive control mediated by executive control network. In line with our finding, subjects with internet gaming disorder had higher functional connectivity between the central node of salience network and areas of the DMN (75).
There are several potential limitations to our study. First, our study does not reveal whether the difference in functional connectivity in problematic smartphone users are vulnerability factors or whether they reflect an outcome of excessive smartphone use. Future studies may include longitudinal studies that involve early childhood and adolescence periods. Second, our study only includes male participants. Gender differences in addiction-like behaviors and neurobiology underlying addiction has been previously demonstrated (32, 33, 36, 37). While the results from our study may provide insight into underlying neurobiology of problematic smartphone use in male participants, it is difficult to generalize it to female participants. It would be meaningful to conduct a follow up study with female participants, which will help us identify similarities and differences between the sexes, which may explain gender differences in addictive disorders. Third, as our study included relatively small number of participants and because group ICA results are noisy, it is possible that ROIs defined from our study may not effectively overcome the limitations of ROIs defined from atlases. Follow up studies with large sample size may be helpful.
Conclusion
In conclusion, our resting-state fMRI study found stronger functional connectivity in the dACC with the DMN and VAN of problematic smartphone users compared with non-problematic smartphone users. Furthermore, we found that the dACC showed additional functional connectivity with the VAN in problematic smartphone users. Future studies on network topology and local fMRI signal fluctuation, such as ALFF, will be necessary to further discern differences between problematic smartphone users and non-problematic smartphone users. Our findings suggest that altered attentional processing and internal mental process may play an important role in problematic smartphone use in light of the differences in functional connectivity in the VAN and DMN, which may share similar abnormalities with other addictive disorders.
Data availability statement
The datasets presented in this article are not readily available because includes potential personal information. Requests to access the datasets should be directed to JL, aHVtYW5qaGxAZ21haWwuY29t.
Ethics statement
The studies involving human participants were reviewed and approved by the Institutional Review Board at Severance Hospital, Yonsei University. The patients/participants provided their written informed consent to participate in this study.
Author contributions
MK, Y-CJ, DL, and JL: conceptualization. MK and JL: data curation. Y-CJ, DL, and JL: methodology and writing – review and editing. Y-CJ and JL: supervision. MK: writing – original draft. All authors contributed to the article and approved the submitted version.
Funding
This study was supported by a grant from the Korean Mental Health Technology R&D Project, Ministry of Health and Welfare, South Korea (HM14C2578).
Conflict of interest
The authors declare that the research was conducted in the absence of any commercial or financial relationships that could be construed as a potential conflict of interest.
Publisher’s note
All claims expressed in this article are solely those of the authors and do not necessarily represent those of their affiliated organizations, or those of the publisher, the editors and the reviewers. Any product that may be evaluated in this article, or claim that may be made by its manufacturer, is not guaranteed or endorsed by the publisher.
Supplementary material
The Supplementary Material for this article can be found online at: https://www.frontiersin.org/articles/10.3389/fpsyt.2022.1008557/full#supplementary-material
Supplementary Figure 1 | Independent components. (A) Default mode network (IC21). (B) Ventral Attention Network (IC37). (C) Dorsal Attention Network, Left (IC20). (D) Dorsal Attention Network, Right (IC32).
Supplementary Figure 2 | Independent components. (A) Executive control network, left (IC28). (B) Executive control network, right (IC34). (C) Salience network (IC23).
Supplementary Figure 3 | Results of seed-to-seed functional connectivity analysis. Statistical inferences were thresholded using an FDR-corrected p-value < 0.05. Values of each functional connectivity are presented on figure.
Supplementary Figure 4 | Independent components considered to be artifacts (cerebrospinal fluid or white matter). (A) Independent Component 5. (B) Independent Component 6. (C) Independent Component 8. (D) Independent Component 10.
Supplementary Figure 5 | Independent components considered to be artifacts (cerebrospinal fluid or white matter). (A) Independent Component 11. (B) Independent Component 19.
Footnotes
- ^ http://www.nitrc.org/projects/conn
- ^ http://www.fil.ion.ucl.ac.uk/spm
- ^ http://mialab.mrn.org/software/gift
References
1. Jiang Z, Shi M. Prevalence and co-occurrence of compulsive buying, problematic Internet and mobile phone use in college students in Yantai, China: relevance of self-traits. BMC Public Health. (2016) 16:1211. doi: 10.1186/s12889-016-3884-1
2. de-Sola J, Talledo H, de Fonseca FR, Rubio G. Prevalence of problematic cell phone use in an adult population in Spain as assessed by the mobile phone problem use scale (MPPUS). PLoS One. (2017) 12:e0181184. doi: 10.1371/journal.pone.0181184
3. Lopez-Fernandez O, Honrubia-Serrano L, Freixa-Blanxart M, Gibson W. Prevalence of problematic mobile phone use in British adolescents. Cyberpsychol Behav Soc Netw. (2014) 17:91–8. doi: 10.1089/cyber.2012.0260
4. Lee J, Ahn JS, Min S, Kim MH. Psychological characteristics and addiction propensity according to content type of smartphone use. Int J Environ Res Public Health. (2020) 17:2292. doi: 10.3390/ijerph17072292
5. Squires LR, Hollett KB, Hesson J, Harris N. Psychological distress, emotion dysregulation, and coping behaviour: a theoretical perspective of problematic smartphone use. Int J Mental Health Addict. (2021) 19:1284–99. doi: 10.1007/s11469-020-00224-0
6. Grant JE, Lust K, Chamberlain SR. Problematic smartphone use associated with greater alcohol consumption, mental health issues, poorer academic performance, and impulsivity. J Behav Addict. (2019) 8:335–42. doi: 10.1556/2006.8.2019.32
7. Kwon M, Lee JY, Won WY, Park JW, Min JA, Hahn C, et al. Development and validation of a smartphone addiction scale (SAS). PLoS One. (2013) 8:e56936. doi: 10.1371/journal.pone.0056936
8. Lin YH, Chang LR, Lee YH, Tseng HW, Kuo TB, Chen SH. Development and validation of the smartphone addiction inventory (SPAI). PLoS One. (2014) 9:e98312. doi: 10.1371/journal.pone.0098312
9. American Psychiatric Association. Diagnostic and Statistical Manual of Mental Disorders: DSM-5. Washington, DC: American Psychiatric Association (2013). doi: 10.1176/appi.books.9780890425596
10. Ting CH, Chen YY. Smartphone Addiction. Amsterdam: Elsevier (2020). p. 215–40. doi: 10.1016/B978-0-12-818626-8.00008-6
11. Chen J, Liang Y, Mai C, Zhong X, Qu C. General deficit in inhibitory control of excessive smartphone users: evidence from an event-related potential study. Front Psychol. (2016) 7:511. doi: 10.3389/fpsyg.2016.00511
12. Hadar A, Hadas I, Lazarovits A, Alyagon U, Eliraz D, Zangen A. Answering the missed call: initial exploration of cognitive and electrophysiological changes associated with smartphone use and abuse. PLoS One. (2017) 12:e0180094. doi: 10.1371/journal.pone.0180094
13. Kardefelt-Winther D, Heeren A, Schimmenti A, van Rooij A, Maurage P, Carras M, et al. How can we conceptualize behavioural addiction without pathologizing common behaviours? Addiction. (2017) 112:1709–15. doi: 10.1111/add.13763
14. Balodis IM, Kober H, Worhunsky PD, Stevens MC, Pearlson GD, Potenza MN. Diminished frontostriatal activity during processing of monetary rewards and losses in pathological gambling. Biol Psychiatry. (2012) 71:749–57. doi: 10.1016/j.biopsych.2012.01.006
15. Choi JS, Shin YC, Jung WH, Jang JH, Kang DH, Choi CH, et al. Altered brain activity during reward anticipation in pathological gambling and obsessive-compulsive disorder. PLoS One. (2012) 7:e45938. doi: 10.1371/journal.pone.0045938
16. Tanabe J, Thompson L, Claus E, Dalwani M, Hutchison K, Banich MT. Prefrontal cortex activity is reduced in gambling and nongambling substance users during decision-making. Hum Brain Mapp. (2007) 28:1276–86. doi: 10.1002/hbm.20344
17. Reuter J, Raedler T, Rose M, Hand I, Glascher J, Buchel C. Pathological gambling is linked to reduced activation of the mesolimbic reward system. Nat Neurosci. (2005) 8:147–8. doi: 10.1038/nn1378
18. Van Holst RJ, van den Brink W, Veltman DJ, Goudriaan AE. Brain imaging studies in pathological gambling. Curr Psychiatry Rep. (2010) 12:418–25. doi: 10.1007/s11920-010-0141-7
19. Spada MM, Roarty A. The relative contribution of metacognitions and attentional control to the severity of gambling in problem gamblers. Addict Behav Rep. (2015) 1:7–11. doi: 10.1016/j.abrep.2015.02.001
20. Ptak R, Schnider A. The dorsal attention network mediates orienting toward behaviorally relevant stimuli in spatial neglect. J Neurosci. (2010) 30:12557–65. doi: 10.1523/JNEUROSCI.2722-10.2010
21. Frank DW, Sabatinelli D. Stimulus-driven reorienting in the ventral frontoparietal attention network: the role of emotional content. Front Hum Neurosci. (2012) 6:116. doi: 10.3389/fnhum.2012.00116
22. Wang Y, Qin Y, Li H, Yao D, Sun B, Li Z, et al. Abnormal functional connectivity in cognitive control network, default mode network, and visual attention network in internet addiction: a resting-state fMRI study. Front Neurol. (2019) 10:1006. doi: 10.3389/fneur.2019.01006
23. Raichle ME, MacLeod AM, Snyder AZ, Powers WJ, Gusnard DA, Shulman GL. A default mode of brain function. Proc Natl Acad Sci USA. (2001) 98:676–82. doi: 10.1073/pnas.98.2.676
24. Lee D, Lee J, Lee JE, Jung Y-C. Altered functional connectivity in default mode network in Internet gaming disorder: influence of childhood ADHD. Prog Neuro Psychopharmacol Biol Psychiatry. (2017) 75:135–41. doi: 10.1016/j.pnpbp.2017.02.005
25. Wang L, Shen H, Lei Y, Zeng L-L, Cao F, Su L, et al. Altered default mode, fronto-parietal and salience networks in adolescents with Internet addiction. Addict Behav. (2017) 70:1–6. doi: 10.1016/j.addbeh.2017.01.021
26. Lee D, Namkoong K, Lee J, Lee BO, Jung Y-C. Lateral orbitofrontal gray matter abnormalities in subjects with problematic smartphone use. J Behav Addict. (2019) 8:404–11. doi: 10.1556/2006.8.2019.50
27. Horvath J, Mundinger C, Schmitgen MM, Wolf ND, Sambataro F, Hirjak D, et al. Structural and functional correlates of smartphone addiction. Addict Behav. (2020) 105:106334. doi: 10.1016/j.addbeh.2020.106334
28. Chun J-W, Choi J, Cho H, Choi M-R, Ahn K-J, Choi J-S, et al. Role of frontostriatal connectivity in adolescents with excessive smartphone use. Front Psychiatry. (2018) 9:437. doi: 10.3389/fpsyt.2018.00437
29. Vargas C, López-Jaramillo C, Vieta E. A systematic literature review of resting state network—functional MRI in bipolar disorder. J Affect Disord. (2013) 150:727–35. doi: 10.1016/j.jad.2013.05.083
30. Yu Q, Du Y, Chen J, He H, Sui J, Pearlson G, et al. Comparing brain graphs in which nodes are regions of interest or independent components: a simulation study. J Neurosci Methods. (2017) 291:61–8. doi: 10.1016/j.jneumeth.2017.08.007
31. Calhoun VD, Liu J, Adali T. A review of group ICA for fMRI data and ICA for joint inference of imaging, genetic, and ERP data. Neuroimage. (2009) 45(1 Suppl.):S163–72. doi: 10.1016/j.neuroimage.2008.10.057
32. Bobzean SA, DeNobrega AK, Perrotti LI. Sex differences in the neurobiology of drug addiction. Exp Neurol. (2014) 259:64–74. doi: 10.1016/j.expneurol.2014.01.022
33. Tavares H, Martins SS, Lobo DS, Silveira CM, Gentil V, Hodgins DC. Factors at play in faster progression for female pathological gamblers: an exploratory analysis. J Clin Psychiatry. (2003) 64:433–8. doi: 10.4088/JCP.v64n0413
34. Kosten TA, Gawin FH, Kosten TR, Rounsaville BJ. Gender differences in cocaine use and treatment response. J Subst Abuse Treat. (1993) 10:63–6. doi: 10.1016/0740-5472(93)90100-G
35. Hudson A, Stamp JA. Ovarian hormones and propensity to drug relapse: a review. Neurosci Biobehav Rev. (2011) 35:427–36. doi: 10.1016/j.neubiorev.2010.05.001
36. Becker JB, Perry AN, Westenbroek C. Sex differences in the neural mechanisms mediating addiction: a new synthesis and hypothesis. Biol Sex Diff. (2012) 3:1–35. doi: 10.1186/2042-6410-3-14
37. Zhang X, Liang M, Qin W, Wan B, Yu C, Ming D. Gender differences are encoded differently in the structure and function of the human brain revealed by multimodal MRI. Front Hum Neurosci. (2020) 14:244. doi: 10.3389/fnhum.2020.00244
38. First MB, Williams JBW, Karg RS, Spitzer RL. Structured Clinical Interview for DSM-5 Disorders, Clinician Version (SCID-5-CV). Arlington, VA: American Psychiatric Association (2016).
39. Wechsler D. Wechsler Adult Intelligence Scale–Fourth Edition (WAIS–IV). (Vol. 22). San Antonio, TX: NCS Pearson (2008). p. 816–27. doi: 10.1037/t15169-000
40. Kim D, Lee Y, Lee J, Nam JK, Chung Y. Development of Korean Smartphone addiction proneness scale for youth. PLoS One. (2014) 9:e97920. doi: 10.1371/journal.pone.0097920
41. Shin K, Kim D, Jung Y, Lee J, Lee Y, Kim M. Development of Korean smart phone addiction proneness scale for youth and adults. Seoul Korean Natl Inf Soc Agency. (2011) 24:231–8.
42. Young KS. Caught in the Net: How to Recognize the Signs of Internet Addiction–and a Winning Strategy for Recovery. Hoboken, NJ: John Wiley & Sons (1998).
43. Patton JH, Stanford MS, Barratt ES. Factor structure of the Barratt impulsiveness scale. J Clin Psychol. (1995) 51:768–74. doi: 10.1002/1097-4679(199511)51:6<768::AID-JCLP2270510607>3.0.CO;2-1
44. Beck A, Ward C, Mendelson M, Mock J, Erbaugh J. An inventory for measuring depression. Arch Gen Psychiatry. (1961) 4:561–71. doi: 10.1001/archpsyc.1961.01710120031004
45. Yook S, Kim Z. A clinical study on the Korean version of beck anxiety inventory: comparative study of patient and non-patient. Korean J Clin Psychol. (1997) 16:185–97.
46. Saunders JB, Aasland OG, Babor TF, De la Fuente JR, Grant M. Development of the alcohol use disorders identification test (AUDIT): WHO collaborative project on early detection of persons with harmful alcohol consumption-II. Addiction. (1993) 88:791–804. doi: 10.1111/j.1360-0443.1993.tb02093.x
47. Buysse DJ, Reynolds IIICF, Monk TH, Berman SR, Kupfer DJ. The Pittsburgh sleep quality index: a new instrument for psychiatric practice and research. Psychiatry Res. (1989) 28:193–213. doi: 10.1016/0165-1781(89)90047-4
48. Steer RA, Kumar G, Beck AT. Use of the Conners-wells’ adolescent self-report scale: short form with psychiatric outpatients. J Psychopathol Behav Assess. (2001) 23:231–9.
49. Ward MF. The Wender Utah rating scale: an aid in the retrospective diagnosis of childhood attention deficit hyperactivity disorder. Am J Psychiatry. (1993) 150:885. doi: 10.1176/ajp.150.6.885
50. Ogawa S, Lee T-M, Kay AR, Tank DW. Brain magnetic resonance imaging with contrast dependent on blood oxygenation. Proc Natl Acad Sci USA. (1990) 87:9868–72. doi: 10.1073/pnas.87.24.9868
51. Thulborn KR, Waterton JC, Matthews PM, Radda GK. Oxygenation dependence of the transverse relaxation time of water protons in whole blood at high field. Biochim Biophys Acta Gen Subj. (1982) 714:265–70. doi: 10.1016/0304-4165(82)90333-6
52. Andersson JL, Hutton C, Ashburner J, Turner R, Friston K. Modeling geometric deformations in EPI time series. Neuroimage. (2001) 13:903–19. doi: 10.1006/nimg.2001.0746
53. Ashburner J, Friston KJ. Unified segmentation. Neuroimage. (2005) 26:839–51. doi: 10.1016/j.neuroimage.2005.02.018
54. Li YO, Adali T, Calhoun VD. Estimating the number of independent components for functional magnetic resonance imaging data. Hum Brain Mapp. (2007) 28:1251–66. doi: 10.1002/hbm.20359
55. Garrity AG, Pearlson GD, McKiernan K, Lloyd D, Kiehl KA, Calhoun VD. Aberrant “default mode” functional connectivity in schizophrenia. Am J Psychiatry. (2007) 164:450–7. doi: 10.1176/ajp.2007.164.3.450
56. Sorg C, Riedl V, Mühlau M, Calhoun VD, Eichele T, Läer L, et al. Selective changes of resting-state networks in individuals at risk for Alzheimer’s disease. Proc Natl Acad Sci USA. (2007) 104:18760–5. doi: 10.1073/pnas.0708803104
57. Assaf M, Jagannathan K, Calhoun VD, Miller L, Stevens MC, Sahl R, et al. Abnormal functional connectivity of default mode sub-networks in autism spectrum disorder patients. Neuroimage. (2010) 53:247–56. doi: 10.1016/j.neuroimage.2010.05.067
58. Shirer WR, Ryali S, Rykhlevskaia E, Menon V, Greicius MD. Decoding subject-driven cognitive states with whole-brain connectivity patterns. Cereb Cortex. (2012) 22:158–65. doi: 10.1093/cercor/bhr099
59. Yeo BT, Krienen FM, Sepulcre J, Sabuncu MR, Lashkari D, Hollinshead M, et al. The organization of the human cerebral cortex estimated by intrinsic functional connectivity. J Neurophysiol. (2011) 106:1125–65. doi: 10.1152/jn.00338.2011
60. Heilbronner SR, Hayden BY. Dorsal anterior cingulate cortex: a bottom-up view. Annu Rev Neurosci. (2016) 39:149–70. doi: 10.1146/annurev-neuro-070815-013952
61. Ainslie G. Specious reward: a behavioral theory of impulsiveness and impulse control. Psychol Bull. (1975) 82:463–96. doi: 10.1037/h0076860
62. Floden D, Stuss DT. Inhibitory control is slowed in patients with right superior medial frontal damage. J Cogn Neurosci. (2006) 18:1843–9. doi: 10.1162/jocn.2006.18.11.1843
63. Peters J, Büchel C. Episodic future thinking reduces reward delay discounting through an enhancement of prefrontal-mediotemporal interactions. Neuron. (2010) 66:138–48. doi: 10.1016/j.neuron.2010.03.026
64. Narayanan NS, Laubach M. Top-down control of motor cortex ensembles by dorsomedial prefrontal cortex. Neuron. (2006) 52:921–31. doi: 10.1016/j.neuron.2006.10.021
65. Botvinick MM, Cohen JD. The computational and neural basis of cognitive control: charted territory and new frontiers. Cogn Sci. (2014) 38:1249–85. doi: 10.1111/cogs.12126
66. Hillman KL, Bilkey DK. Neurons in the rat anterior cingulate cortex dynamically encode cost–benefit in a spatial decision-making task. J Neurosci. (2010) 30:7705–13. doi: 10.1523/JNEUROSCI.1273-10.2010
67. Goldstein RZ, Tomasi D, Rajaram S, Cottone LA, Zhang L, Maloney T, et al. Role of the anterior cingulate and medial orbitofrontal cortex in processing drug cues in cocaine addiction. Neuroscience. (2007) 144:1153–9. doi: 10.1016/j.neuroscience.2006.11.024
68. Hester R, Garavan H. Executive dysfunction in cocaine addiction: evidence for discordant frontal, cingulate, and cerebellar activity. J Neurosci. (2004) 24:11017–22. doi: 10.1523/JNEUROSCI.3321-04.2004
69. Menon V, Uddin LQ. Saliency, switching, attention and control: a network model of insula function. Brain Struct Funct. (2010) 214:655–67. doi: 10.1007/s00429-010-0262-0
70. Sridharan D, Levitin DJ, Menon V. A critical role for the right fronto-insular cortex in switching between central-executive and default-mode networks. Proc Natl Acad Sci USA. (2008) 105:12569–74. doi: 10.1073/pnas.0800005105
71. Corbetta M, Patel G, Shulman GL. The reorienting system of the human brain: from environment to theory of mind. Neuron. (2008) 58:306–24. doi: 10.1016/j.neuron.2008.04.017
72. Dosenbach NU, Visscher KM, Palmer ED, Miezin FM, Wenger KK, Kang HC, et al. A core system for the implementation of task sets. Neuron. (2006) 50:799–812. doi: 10.1016/j.neuron.2006.04.031
73. Shulman GL, McAvoy MP, Cowan MC, Astafiev SV, Tansy AP, d’Avossa G, et al. Quantitative analysis of attention and detection signals during visual search. J Neurophysiol. (2003) 90:3384–97. doi: 10.1152/jn.00343.2003
74. Menon V. Large-scale brain networks and psychopathology: a unifying triple network model. Trends Cogn Sci. (2011) 15:483–506. doi: 10.1016/j.tics.2011.08.003
Keywords: attention network, default mode network, problematic smartphone use, functional MRI, dorsal anterior cingulate cortex
Citation: Kwon M, Jung Y-C, Lee D and Lee J (2022) Altered resting-state functional connectivity of the dorsal anterior cingulate cortex with intrinsic brain networks in male problematic smartphone users. Front. Psychiatry 13:1008557. doi: 10.3389/fpsyt.2022.1008557
Received: 31 July 2022; Accepted: 16 September 2022;
Published: 03 October 2022.
Edited by:
Aviv M. Weinstein, Ariel University, IsraelReviewed by:
Yuncong Ma, University of Pennsylvania, United StatesShuoqiu Gan, Hefei Comprehensive National Science Center, China
Copyright © 2022 Kwon, Jung, Lee and Lee. This is an open-access article distributed under the terms of the Creative Commons Attribution License (CC BY). The use, distribution or reproduction in other forums is permitted, provided the original author(s) and the copyright owner(s) are credited and that the original publication in this journal is cited, in accordance with accepted academic practice. No use, distribution or reproduction is permitted which does not comply with these terms.
*Correspondence: Junghan Lee, aHVtYW5qaGxAZ21haWwuY29t