- 1Institut d'Investigacions Biomèdiques August Pi I Sunyer (IDIBAPS), Barcelona, Spain
- 2Centro de Investigación Biomédica en Red de Salud Mental (CIBERSAM), Instituto de Salud Carlos III, Madrid, Spain
- 3Department of Medicine, University of Barcelona, Barcelona, Spain
- 4Department of Education and Psychology, Freie Universität Berlin, Berlin, Germany
- 5Einstein Center for Neurosciences Berlin, Charité - Universitätsmedizin Berlin, Berlin, Germany
- 6Berlin School of Mind and Brain, Humboldt-Universität zu Berlin, Berlin, Germany
- 7Bipolar and Depressive Disorders Unit, Hospital Clinic, Barcelona, Spain
- 8Clinical Psychology PhD Program, California School of Professional Psychology, San Diego, CA, United States
- 9Department of Psychiatry, University of California San Diego, La Jolla, CA, United States
- 10Fundació Clínic per a la Recerca Biomèdica (FCRB), Barcelona, Spain
- 11Psychiatric and Psychology Service, Hospital Clinic, Barcelona, Spain
- 12Schizophrenia Unit, Hospital Clinic, Barcelona, Spain
- 13Department of Psychiatry and Forensic Medicine, Autonomous University of Barcelona, Barcelona, Spain
- 14Department of Psychology, Abat Oliba CEU (“Centro de Estudios Universitarios”) University, Barcelona, Spain
- 15Programa E-Health ICOnnecta't, Institut Català d'Oncologia, Barcelona, Spain
- 16Department of Neuroimaging, Institute of Psychiatry, Psychology, and Neuroscience, King's College London, London, United Kingdom
- 17Department of Child and Adolescent Psychiatry, Institute of Psychiatry, Psychology, and Neuroscience, King's College London, London, United Kingdom
- 18Live and Health Sciences Research Institute (ICVS), University of Minho, Braga, Portugal
- 19ICVS/3B's, PT Government Associate Laboratory, Braga, Portugal
- 20Clinical Academic Center - Braga, Braga, Portugal
- 21Division of Psychology and Language Sciences, University College London, London, United Kingdom
- 22State Key Laboratory of Cognitive Neuroscience and Learning and IDG/McGovern Institute for Brain Research, Beijing Normal University, Beijing, China
- 23Department of Neurology, Department of Addictive Behavior and Addiction Medicine, Central Institute of Mental Health, Affiliated Yancheng Hospital of Southeast University, Yancheng, China
- 24Medical Faculty Mannheim, University of Heidelberg, Mannheim, Germany
- 25Department of Psychological Medicine, Institute of Psychiatry, Psychology, and Neuroscience, King's College London, London, United Kingdom
- 26Department of Psychiatry and Behavioral Science, College of Medicine and Health Sciences, United Arab Emirates University (UAEU), Al Ain, United Arab Emirates
- 27Department of Psychosis Studies, Institute of Psychiatry, Psychology & Neuroscience, King's College London, London, United Kingdom
- 28Psychiatry and Mental Health Group, Neuroscience Program, Bellvitge Biomedical Research Institute (IDIBELL), L'Hospitalet de Llobregat, Barcelona, Spain
- 29Department of Psychobiology and Methodology of Health Sciences, Universitat Autònoma de Barcelona, Barcelona, Spain
- 30Department of Psychiatry, University of Michigan, Ann Arbor, MI, United States
- 31The Social and Behavioral Research Branch, National Human Genome Research Institute, National Institute of Health, Bethesda, MD, United States
- 32Early Psychosis: Interventions and Clinical-Detection (EPIC) Lab, Department of Psychosis Studies, Institute of Psychiatry, Psychology, London, United Kingdom
- 33Department of Brain and Behavioral Sciences, University of Pavia, Pavia, Italy
- 34Outreach and Support in South London (OASIS) Service, South London and Maudsley NHS Foundation Trust, London, United Kingdom
- 35Department of Clinical Neuroscience, Center for Psychiatry Research, Karolinska Institutet, Stockholm, Sweden
- 36Stockholm Health Care Services, Stockholm County Council, Stockholm, Sweden
- 37Child and Adolescent Psychiatry and Psychology Department, Hospital Sant Joan de Déu, Barcelona, Spain
- 38Child and Adolescent Mental Health Research Group, Institut de Recerca Sant Joan de Déu, Barcelona, Spain
- 39Mental Health Department, Hospital Universitari Parc Taulí, Institut d'Investigació i Innovació Parc Taulí (I3PT), Sabadell, Spain
- 40Department of Psychiatry, University of Ottawa, Ottawa, ON, Canada
- 41Department of Mental Health, The Ottawa Hospital, Ottawa, ON, Canada
- 42Clinical Epidemiology Program, Ottawa Hospital Research Institute, Ottawa, ON, Canada
- 43Department of Pediatrics, Yonsei University College of Medicine, Seoul, South Korea
Background: In mental health, comorbidities are the norm rather than the exception. However, current meta-analytic methods for summarizing the neural correlates of mental disorders do not consider comorbidities, reducing them to a source of noise and bias rather than benefitting from their valuable information.
Objectives: We describe and validate a novel neuroimaging meta-analytic approach that focuses on comorbidities. In addition, we present the protocol for a meta-analysis of all major mental disorders and their comorbidities.
Methods: The novel approach consists of a modification of Seed-based d Mapping—with Permutation of Subject Images (SDM-PSI) in which the linear models have no intercept. As in previous SDM meta-analyses, the dependent variable is the brain anatomical difference between patients and controls in a voxel. However, there is no primary disorder, and the independent variables are the percentages of patients with each disorder and each pair of potentially comorbid disorders. We use simulations to validate and provide an example of this novel approach, which correctly disentangled the abnormalities associated with each disorder and comorbidity. We then describe a protocol for conducting the new meta-analysis of all major mental disorders and their comorbidities. Specifically, we will include all voxel-based morphometry (VBM) studies of mental disorders for which a meta-analysis has already been published, including at least 10 studies. We will use the novel approach to analyze all included studies in two separate single linear models, one for children/adolescents and one for adults.
Discussion: The novel approach is a valid method to focus on comorbidities. The meta-analysis will yield a comprehensive atlas of the neuroanatomy of all major mental disorders and their comorbidities, which we hope might help develop potential diagnostic and therapeutic tools.
Introduction
Authors have reported potential brain anatomical abnormalities for different mental disorders since the 1980s (1). At present, the neuroscientific community has enough data (thousands of studies) to create an atlas of these abnormalities, but this is not yet a reality due to the heterogeneity in the findings across studies investigating the same disorder. For instance, a meta-analysis of structural brain alterations of social anxiety disorder (SAD) found that studies presented contradictory findings, such as increases and decreases in gray matter (GM) volume in the hippocampus and other brain regions (2). Similarly, whereas several meta-analyses had reported significant SAD-related abnormalities in GM in the amygdala-hippocampal, prefrontal, and parietal regions (3–5), an ENIGMA study only found a significant larger GM volume in the right putamen (6). Another example could be the case of obsessive-compulsive disorder (OCD) neuroanatomical findings, where although the abnormalities of the corticostriatal-thalamocortical circuits have been consistently reported (7–9), recent evidence has been accumulating to other regions outside these circuits with less agreement among meta- and mega-analysis reports (7, 10, 11). Moreover, the exact direction of increases and decreases in GM volume in certain areas, such as the orbitofrontal cortex (OFC), has been unclear (12). For example, some reports show reduced GM in bilateral (13) or right (14) OFC, whereas there are also studies reporting increased bilateral (15) or left (16) OFC.
The found heterogeneity may be partly related to the use of magnetic resonance imaging (MRI) devices with varying field strengths (17) and head coils (18) or to the techniques or software used to process the images (18, 19). In addition, several clinical parameters might moderate the findings, such as the age at disease onset and the duration of disease (20, 21), the current age (22), symptom heterogeneity (23), the gender distribution (24), the medication status (25, 26), or clinical stage (27). For example, in a meta-analysis of attention-deficit hyperactivity disorder (ADHD) (28), we found that samples with more medicated patients showed less decreased GM volume in the right caudate.
Another relevant but little explored source of heterogeneity may be the varying presence of comorbidities, which are the rule in psychiatry (29). About half of people with mental disorders have more than one comorbid mental disorder (30, 31). Some authors claim that “there are no patients without comorbidity” (32). We must acknowledge that some studies exclude individuals with specific comorbid mental disorders. Still, this exclusion is frequently limited to very few entities such as psychosis and substance use disorders. Similarly, we must acknowledge that some comorbidities are conceptually impossible, e.g., a patient with bipolar disorder (BD) cannot be diagnosed with comorbid major depressive disorder (MDD), but again, the list of impossible comorbidities is limited. Therefore, most patients included in a study of a given mental disorder might also have other mental disorders. For example, in a meta-analysis of neuroimaging studies in OCD, patients had comorbid depression or anxiety disorders in 65% of the studies. The percentage of patients with comorbid disorders reached 50% (for depression) and 85% (for anxiety disorders) in some included studies (33). This common presence of abnormalities from other mental disorders might thus confound the results of case-control studies.
Relevantly, previous studies have found that some brain abnormalities associated with different mental disorders are non-specific. For example, in several meta-analyses, we observed similar decreases of GM volume in the anterior cingulate/medial frontal cortex in disorders as different as psychosis, anxiety disorders (AD), ADHD, and autism spectrum disorders (ASD) (33–37). Similarly, an ENIGMA study reported a high similarity of brain structural abnormalities between MDD, BD, OCD, and schizophrenia (38, 39). We fully acknowledge that some of these non-specific abnormalities may be transdiagnostic, i.e., associated with two or more mental disorders. However, there is also the possibility that some others are related to the confounding effects of comorbidities.
This study aims to describe and validate a novel neuroimaging meta-analytic approach that focuses on comorbidities and presents the protocol for a meta-analysis of all major mental disorders and their potential comorbidities. We exemplify this protocol for voxel-based morphometry (VBM) studies investigating GM volume differences between patients with mental disorders and healthy controls. However, it could be similarly applied to any neuroimaging modality compatible with SDM (e.g., functional MRI or diffusion tensor imaging). Specifically, we will meta-analyze brain abnormalities from different disorders with different comorbidities, with a single meta-linear model (though separately for children/adolescents and adults). This analysis will yield an MRI-based atlas that dissects the specific brain anatomical abnormalities of each mental disorder and comorbidity. In complementary analyses and depending on data availability reported in the studies, we will explore the potentially confounding or moderating effects of age, sex, medication, age of onset, duration of illness, and symptom severity.
Methods and Analysis
The Novel Approach
The new approach is conceptually novel, but it involves only a minor modification of the “seed-based d mapping-permutation of subject images” (SDM-PSI) (www.sdmproject.com) (11, 40–42), an already validated and widely used brain imaging meta-analytic method (43–51). The main advantage of this method is that it directly tests whether there are differences between patients and controls, rather than conducting indirect tests such as whether peaks tend to converge in some regions more than in others (52).
It first creates maps of the lower and upper bounds of possible effect sizes for each study based on the available statistical information and the anisotropic covariance between adjacent voxels (53). Second, it uses maximum likelihood estimation techniques to impute several effect sizes maps for each study, assuming that the effect size follows a truncated normal distribution within the lower and upper bounds. Third, it fits a random-effects meta-analytic linear model separately for each voxel. Fourth, it combines the meta-analytic maps resulting from the different imputations using Rubin's rules. Finally, it conducts a permutation test to yield threshold-free cluster enhancement (TFCE)-based (54) familywise error rates (FWER, i.e., corrected p-values).
In this paper, we will first describe the new concept, then report the small methodological changes, and finally report a validation of the approach using simulations.
Description
Commonly, the primary (random-effects meta-analytic) linear model of a meta-analysis is just a (weighted) mean:
where Yi is the effect size of the ith study, β is the meta-analytic effect size, and εi is the residual for the ith study. It is important to remember that this model is conducted separately for each voxel.
In some previous meta-analyses, we attempted to control for comorbidity via meta-regression (covariation) by the percentage of patients with a comorbid disorder:
where the intercept βA is the effect size for patients with only disorder A, the coefficient βAB−A is the difference between patients with both disorders and patients with only the disorder A, and Xi,AB is the proportion of patients with both disorders in the ith study.
However, these attempts had two relevant limitations. First, βAB−A (the difference between patients with both disorder A and disorder B and patients with only disorder A) mixed the effects of disorder B and the effects of the comorbidity AB. Thus, we could not know which part of βAB−A was shared by any patient with disorder B (with or without disorder A) and which part of βAB−A was specific to those patients with both disorder A and disorder B. For instance, imagine that patients with only disorder A show decreased amygdala, patients with only disorder B show decreased cerebellum, and patients with both disorder A and disorder B show decreased amygdala, cerebellum, and prefrontal cortex. The decrease in the cerebellum is shared by any patient with disorder B, and the reduction in the prefrontal cortex is specific to those with both disorder A and disorder B, but βAB−A would mix both abnormalities. Second, we could only include studies on disorder A in which a variable proportion of patients had disorder B. Conversely, we could not include studies on disorder B in which a variable proportion of patients had the disorder A, with the subsequent loss in precision.
Here, we propose to use the following model to study two disorders A and B:
where βA is the effect size for patients with only disorder A, XiA is the proportion of patients with disorder A in the ith study, βB is the effect size for patients with only disorder B, XiB is the proportion of patients with disorder B in the ith study, βAB is the effect size of the comorbidity AB, and XiAB is the proportion of patients with both disorders in the ith study.
This model overcomes the limitations of the previous attempts because it separates the effects of disorder B and the effects of the comorbidity AB and can accept both studies on disorder A and studies on disorder B because it treats all disorders equally. Indeed, we can extend the model to as many disorders and comorbidities as wished. Still, considering the complexity of the analysis and the likely poor reporting of co-occurring comorbidities, we will only consider pairs of comorbid mental disorders that are possible (e.g., anxiety and MDD) and have been studied by at least ten studies.
Validation
To validate the novel approach, we simulated 64 studies on disorder A, 64 on disorder B, and 64 on disorder C, with varying levels of comorbidity, and then meta-analyzed them using the novel approach. The reason to simulate 64 studies for each disorder is that we simulated eight levels of comorbidity (including no comorbidity) for each of the two comorbid disorders, e.g., for disorder A, we simulated eight levels of comorbid disorder B X 8 levels of comorbid disorder C.
Specifically, we first simulated for each study that a varying proportion of the patients had one of the other disorders or both. We then created the subjects' gray matter maps as white noise following a standard normal distribution. Still, for each patient, we added or subtracted 0.5 in four brain regions depending on the disorders he/she had and the rules in Figure 1. We thus created abnormalities with a medium effect size (Cohen's d around 0.5). Finally, we conducted the voxelwise t-test between patients and controls to derive the study t-map.
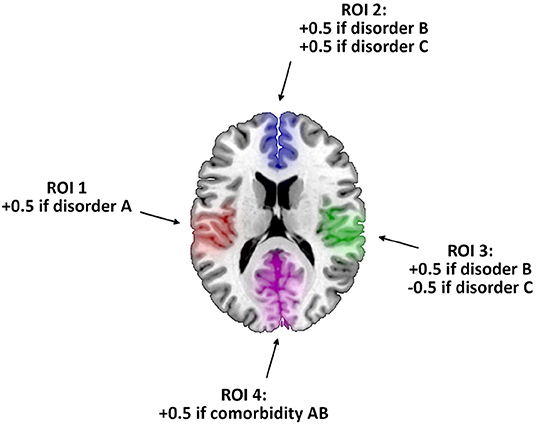
Figure 1. Rules for creating the gray matter maps of a simulated patient. After creating a map using white noise, we added or subtracted 0.5 in the colored brain regions of interest (ROI) depending on the disorders he/she had.
To meta-analyze the studies' t-maps with the novel SDM-PSI approach (with default values and defining statistical significance as TFCE permutation-based FWER < 0.05), we modeled:
For example, imagine that disorder A was OCD, disorder B was MDD, and disorder C referred to anxiety disorders. Studies on OCD would be coded as XiA = 1, XiAB = [proportion of patients with comorbid MDD], and XiAC = [proportion of patients with comorbid anxiety disorders]. Studies on MDD would be coded as XiB = 1, XiAB = [proportion of patients with comorbid OCD], and XiBC = [proportion of patients with comorbid anxiety disorders]. Finally, studies on anxiety disorders would be coded as XiC = 1, XiAC = [proportion of patients with comorbid OCD], and XiBC = [proportion of patients with comorbid MDD]. Although studies seldom report the proportion of patients with multiple comorbidities (e.g., in studies on OCD, the proportion of patients with both comorbid MDD and anxiety disorders), our new approach could easily account for them if reported. Meta-analysts should add a regressor for each reported combination of comorbid mental disorders.
For comparison purposes, we also conducted three meta-regressions using the previous SDM-PSI approach (again, with default values and defining statistical significance as TFCE permutation-based FWER < 0.05):
Results of the Validation
The novel SDM-PSI approach detected all the simulated abnormalities with the correct effect size and did not yield any falsely positive findings (Table 1, left column; and Figure 2).
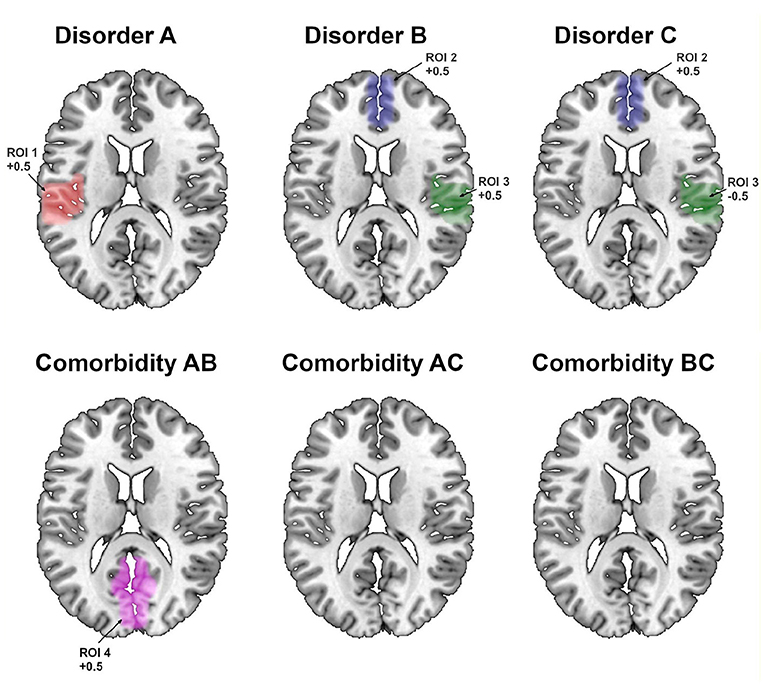
Figure 2. Regions showing statistically significant effects using the novel SDM-PSI approach. ROI, region of interest.
The previous SDM-PSI reported a list of findings very similar to the simulated factual data (Table 1, right columns; and Figure 3). However, the results showed the two main limitations we had expected. First, they mixed the abnormalities of the comorbid disorder and the comorbidity. For instance, in the meta-regression using studies on disorder A, the coefficient βAB−A mixes the anomalies simulated for disorder B and comorbidity AB. We acknowledge that this limitation could be potentially overcome by looking at the meta-regression using studies on disorder B. However, this strategy would be confusing in this example because we would still not know whether abnormality in the region of interest (ROI) 4 is due to disorder A or comorbidity AB. The second limitation was a slight loss of accuracy, as shown by that some Hedges' g are slightly different from 0.5, and there are a few falsely positiveresults.
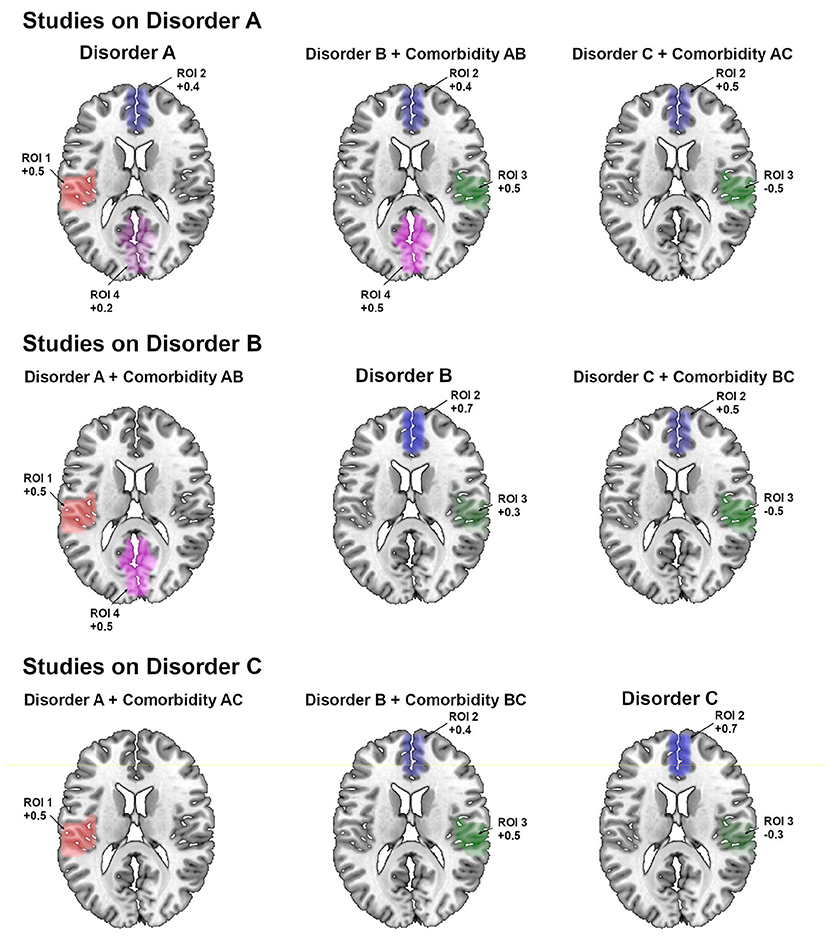
Figure 3. Regions showing statistically significant effects using the previous SDM-PSI approach (separate results for meta-analysis of studies on disorder A, meta-analysis of studies on disorder B, and meta-analysis of studies on disorder C). ROI, region of interest.
Therefore, while the novel SDM-PSI approach does not invalidate the previous version, it better disentangles the specific abnormalities of comorbid disorders.
Results were similar when we created the simulated data with double error, thus expecting Cohen's d around 0.25 (Table 2). That said, one can expect poorer estimations with smaller effect sizes or in meta-analyses with few studies. Thus, we would not recommend the new approach when the number of studies for each regressor is too small for the expected effect sizes.
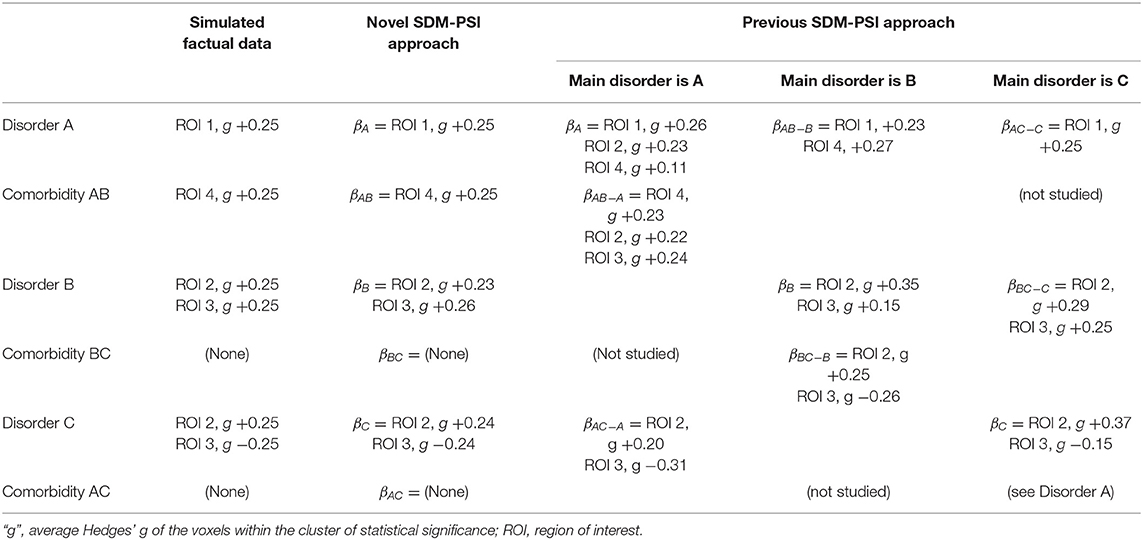
Table 2. Results of the novel approach validation after creating the simulated data with double error.
Protocol for the -Meta-Analysis
We pre-registered this protocol to PROSPERO (CRD42021245098).
Design
Meta-regression of case-control VBM studies of GM volume abnormalities in all major mental disorders. The dependent variable will be the brain anatomical differences between patients and controls in a voxel. The independent variables will be the percentages of patients with each mental disorder and each pair of potentially comorbid mental disorders.
Systematic Search
With few exceptions (see below), we will include all whole-brain VBM studies in any mental disorder listed in the mental, behavioral, or neurodevelopmental disorders classification of ICD-11 (International Classification of Diseases 11th Revision). Note that we will use ICD-11 to select the major disorders to investigate. Still, as we clarify later, we will include studies using any standard clinical assessment beyond ICD (e.g., DSM). Our search will have two steps.
First Step: Search and Inclusion of Meta-Analyses
In the first step, we will search for the most recent SDM meta-analysis (if any) in the PubMed and Scopus databases for each mental disorder listed in ICD-11 classification (excluding nicotine use disorder, substance-induced specific disorders, neurocognitive disorders, and mental or behavioral disorders associated with pregnancy, childbirth, or the puerperium). The keywords will be the mental disorder (e.g., “major depression,” “anxiety disorders,” “bipolar disorders,” etc.) AND (“meta-analysis”) AND (“voxel-based morphometry” OR “VBM” OR “gray matter” OR “grey matter”). We will first screen all the results by the title/abstract and afterward by full-text reading.
The inclusion criterion will be meta-analyses of studies that employed VBM to investigate whole-brain GM volume differences between patients with the above disorders and healthy controls. The exclusion criterion will be meta-analyses from which we can include <10 studies even after adding new studies as described in the second step. We will select the most recent meta-analysis conducted with SDM if more than one meta-analysis meets our inclusion/exclusion criteria. Suppose the inclusion/exclusion criteria of a meta-analysis led to the exclusion of studies that we would include according to our second-step study inclusion/exclusion criteria (see next). In that case, we will look for these potentially includable studies (e.g., a meta-analysis may have excluded studies in children/adolescents while we will include them). Conversely, suppose the inclusion/exclusion criteria of a meta-analysis led to the inclusion of studies that we would exclude according to our second-step inclusion/exclusion criteria. In that case, we would exclude these studies (e.g., a meta-analysis may have included studies with fewer than 10 participants per group while we will exclude them). Suppose during our search, a new meta-analysis is published after we have included a meta-analysis for the same mental disorder. In that case, we will include both analyses (but we will include the duplicated studies only once.
Second Step: Search and Inclusion of Individual Studies
In the second step, we will search in PubMed and Scopus databases for the studies published since the search date of the selected meta-analysis. The keywords used in this search will be [Title/Abstract]: (selected mental disorder) and (“VBM” OR “morphometry” OR “voxel-based” OR “voxelwise” OR “gray matter” OR “grey matter”). We will first screen all the results by the title/abstract and then by full-text reading.
Inclusion criteria will be: (1) studies reporting whole-brain regional GM volume differences between individuals with the included mental disorders, diagnosed by standard clinical assessments (DSM or ICD), and matched healthy controls; (2) employing VBM to conduct the comparisons, (3) reporting the peaks of the clusters of statistically significant voxels or null findings, or availability of statistical parametric map; (4) using a constant statistical threshold throughout the whole gray matter; (5) published as peer-reviewed original articles in English in indexed journals. Exclusion criteria will be: (1) sample size smaller than 10 participants in either the patient or the control group; (2) no case-control comparisons; (3) disorders' subtypes with a known organic origin (e.g., pediatric autoimmune neuropsychiatric disorders associated with streptococci); (4) coordinates of the peaks of the clusters cannot be obtained after contacting the authors (unless maps are available, in which case we will not need the coordinates); (5) ROI or small volume correction (SVC) analyses; (6) ANOVA analysis without whole-brain t-test post hoc analyses; (7) case reports, conferences abstracts, editorials, non-scientific letters, and research protocols; (8) duplicated datasets (we will only include the largest sample size); (9) studies that only analyze the correlation of GM volume with any other measure or that use GM volume features to predict diagnosis unless they specify an additional VBM case-control comparison in the abstract. Special cases will be: (1) longitudinal studies: we will only include the baseline comparison; (2) studies reporting different subgroup analyses: we will include the combined analysis of all subgroups if available. Otherwise, we will include them as different studies if they use different control groups and provide demographic and clinical data for both subgroups separately. If they share the control group, we will divide the control sample size between the number of subgroups.
Two researchers will conduct the systematic search independently, and we will resolve any discrepancies by consensus with a third researcher.
Data Collection
For each study separately, we will extract the sample sizes, demographic and clinical data, methodologic details, and the original statistical parametric map (when available) or the coordinates and t-values (or equivalent statistics when available) of the peaks of the clusters of statistically significant voxels (or null findings).
Demographic data will include age distribution (mean and standard deviation) and percentage of males and females.
Clinical data will consist of the percentages of patients with different mental disorders, the percentage of patients receiving each medication group (antipsychotics, antidepressants, anxiolytics -other than hypnotic-, mood stabilizers, and stimulants), the severity of the primary disorder assessed by standard measures [e.g., Hamilton Depression Rating Scale (HDRS) (55)], the age of onset or illness duration of the primary disorder (mean and standard deviation), and the different subtypes for the primary disorder as reported in the included meta-analyses (e.g., inattentive, hyperactive, or combined type for ADHD, type I or II for BD, etc.).
Methodological details will include the pre-processing analysis software (e.g., FSL, SPM) and their version, stereotactic space (e.g., MNI, Talairach space, or MNI coordinates converted to Talairach using the old Brett transform), and the statistical significance threshold (e.g., FWER < 0.05). For studies reporting peaks obtained using two or more whole-brain statistical significance levels (e.g., uncorrected p < 0.001 and corrected FWER p < 0.05), we will include all peaks obtained using the less conservative threshold. We will also record the information required for the quality assessment (see below).
Given the magnitude of data, we will store them in a database and a well-organized file system with automatic daily backups.
Quality Assessment
We will use the Newcastle-Ottawa Scale (NOS) for case-control studies to assess each study's quality (56). The NOS assesses three characteristics of the studies: the selection of the study groups, the comparability of the groups, and the ascertainment of the exposure for case-control studies. The “selection of the study groups” evaluates the adequate definition of case and control, as well as the representativity of the cases (e.g., selection of all eligible cases with the outcome of interest over a defined period, in a defined catchment area or hospital, etc.), and the controls (community controls or hospital controls). The “comparability of the groups” evaluates if researchers matched cases and controls and/or adjusted for confounders (e.g., age, sex, handedness). Here, statements of no differences between groups or non-statistically significant differences are insufficient for establishing comparability. Finally, we will not evaluate the “ascertainment of the exposure for case-control studies” because both groups underwent a structural MRI in our studies.
We will also assess how much demographic or clinical data each independent study reports.
Imputation of Missing Comorbidity Data
Not all studies report the percentage of patients with specific comorbid disorders. For instance, in the meta-analysis of OCD mentioned earlier, 12% of studies had not excluded comorbid MDD but did not report how many patients had this diagnosis. To impute these unreported data, we will assume they are missing at random. In other words, the proportion of patients with no information about comorbid MDD should follow a similar distribution than in studies reporting this information.
The proportion of patients with a comorbid disorder likely follows a zero-inflated distribution. For example, the percentage of patients with MDD might follow some statistical distribution, but this distribution probably has excess zeroes due to the studies that excluded patients with MDD. However, as fitting zero-inflated distributions with the small data available would be unfeasible, we will use a more straightforward, distribution-free approach. Specifically, the imputation will consist of assigning to each study not reporting the proportion of patients with comorbid MDD, the proportion from another random OCD study. Thus, for example, we may estimate that the missing proportion in a given study is the same as in the study by van den Heuvel et al. (57), or the same as in the study by Pujol et al. (58), or the same as in any other random study (including studies that excluded MDD).
We will repeat these imputations 50 times. We want to remark that his number is commonly considered more than adequate for multiple imputation (Rubin recommended 3 to 10 imputations (59). Additionally, we have checked that the histogram of the imputed proportions of patients with MDD is similar to the histogram of known proportions of patients with MDD after only ten imputations and nearly identical after twenty imputations.
These imputations will be conducted separately for each comorbid disorder. Thus, for instance, in studies with OCD, we will impute comorbid MDD and comorbid anxiety disorders separately.
Statistical Analyses
We will carry out the data pre-processing and the statistical analysis with the SDM-PSI 6.21 software (https://www.sdmproject.com/) (11, 40–42). We will conduct two independent analyses, one for adults and one for children/adolescents.
The pre-processing of statistical parametric maps is the straightforward conversion into images of effect sizes. For studies with only peak information available, the pre-processing consists of estimating the 3D images of the lower and higher bounds of potential effect sizes. The software will later impute the effect sizes multiple times within these bounds.
We will include all major mental disorders in one single linear model described earlier. Then, we will estimate the effects related to each disorder and comorbidity by testing different contrasts within the model. The steps will be those of standard SDM-PSI (11, 40–42) unless otherwise specified:
1. Estimation of the 3D images of maximum likely effect sizes for each model coefficient.
2. Multiple imputation of the study 3D images of effect size adding spatially realistic noise to the expected effect size according to the estimated distribution within the bounds. Following SDM-PSI default parameters, we will conduct this process 50 times, resulting in 50 imputed datasets covering the imputations' uncertainty.
3. Separately for each imputation dataset, random-effects meta-linear model. The dependent variable will be the effect size of the voxel. The independent variables will be the percentages of patients with each mental disorder and the percentages of patients with each pair of potentially comorbid disorders (as far as they involve at least ten studies). In case that at least studies reported the percentage of patients with more than three or more comorbid disorders (e.g., OCD, MDD, and anxiety), we will also include these percentages as an independent variable in the model.
4. Using Rubin's rules, combination of the meta-analytic 3D images of effect size from the different imputation datasets.
To assess the statistical significance, the software converts the 3D image of z-values into a 3D image of threshold-free cluster enhancement (TFCE) statistics and finds the p-value of the TFCE statistics using a Freedman-Lane-based permutation test (60). We will consider statistically significant those voxels with family-wise error-rate (FWER, i.e., corrected p-values) <0.05. For comprehensive reporting, we will also publish supplementary results using significance thresholds of FWER < 0.01, uncorrected p < 0.001, and uncorrected p < 0.005.
In the complementary analyses, we will add additional independent variables to the linear model to explore potential interactions with mean sample age and percentage of males and control the medication's possible confounding effects. Depending on our final dataset, we will try to assess the effects of the age of onset or illness duration and the severity of the primary disorder, as reported in the original studies. Furthermore, we will perform a subgroup analysis excluding those disorders for which we cannot safely collect whether they are comorbidities for other disorders (e.g., we expect that we will not have information on comorbid personality disorders in many studies).
We will use the I2 statistic to quantify heterogeneity and conduct meta-regression by the standard error [similar to an Egger-test (41)] to detect potential publication bias. Conventionally, I2 values above 50% are interpreted as an indication of significant heterogeneity (61).
Discussion
This paper first presents and validates a neuroimaging meta-analytic approach that focuses on comorbidities in mental disorders. Then, using simulations, we show that the new method may detect all GM volume differences with the correct effect size and without falsely positive findings. Finally, we describe the protocol for a meta-analysis of all major mental disorders and their comorbidities, separately for adult and pediatric groups. We will also assess the potentially confounding effects of medication, age of onset or illness duration and the symptom severity of the primary disorder, and the moderator effects of sex on GM volume.
We broadly expect some findings according to previous literature, though a significant part of these findings might change due to the improvements of the new approach. For example, for chronic schizophrenia, previous meta-analyses have detected reduced GM volume in the bilateral insula/ superior temporal gyrus, dorsal, and rostral anterior cingulate cortex (ACC) / medial frontal gyrus, and the thalamus (62, 63). Similarly, for the first episode of psychosis, we expect a reduced GM volume in the right dorsal ACC and the right posterior insula/superior temporal gyrus (35, 62, 63). In OCD, previous meta-analyses have detected both increased GM volumes, mainly located in subcortical regions (e.g., bilateral putamen, left cerebellum, and left hippocampus), and decreased GM volumes, located primarily on prefrontal and cingulate areas (e.g., bilateral ACC/ventromedial prefrontal cortex, bilateral inferior frontal gyrus) (9, 33, 34). For BD and MDD, previous meta-analyses have detected a commonly reduced GM volume in the medial prefrontal system and ACC, regions strongly implicated in mood regulation (64). However, smaller hippocampus and parahippocampal gyrus volumes have been more reported in MDD (26, 37, 65–69). For ADHD, previous meta-analyses have reported a reduced GM volume in ventromedial orbitofrontal cortex/ventromedial prefrontal cortex/rostral ACC, and the right basal ganglia/anterior and posterior insula (34, 70). For ASD, previous meta-analyses have reported reduced GM volume in dorsal ACC/dorsomedial prefrontal cortex, left cerebellum, and increased GM volume in the left middle superior anterior lobe and middle frontal gyrus (33, 70). For anxiety disorders, previous meta-analyses have reported a reduced GM volume in the right ventral ACC and inferior frontal gyrus (71). These areas have been primarily reported in panic disorder, together with the prefrontal cortex (72, 73). However, some of these findings may have been influenced by comorbidity. Thus, we need to conduct the new meta-analysis to know which results remain, which do not, and which had not been detected due to the confounding effects of comorbidities.
We hope that this improved atlas of the anatomical localization of the brain abnormalities associated with each mental disorder will help improve our understanding of their physio-pathological processes. In addition, we hope that this atlas could be the base for developing MRI-based diagnostic tools that help earlier diagnoses and, therefore, more targeted treatments. For instance, when complex psychotic symptoms hamper the assessment of other symptoms needed for the diagnosis, the “opinion” of an MRI-based diagnostic tool could provide timely extra information to establish an affective vs. non-affective diagnosis and thus a more focused treatment earlier. Or similarly, in depressed individuals at risk of manic shift, the “opinion” of an MRI-based diagnostic tool may help the clinician better evaluate the probability of bipolar vs. unipolar disorder and thus design a more personalized preventive strategy. Indeed, we have found elsewhere that diagnostic labels are among the variables that best predict the future recurrence of an episodic disorder.
Better knowledge about the disorder-specific abnormalities could also increase the efficacy of therapies to modify specific brain regions' activity [e.g., deep brain stimulation or non-invasive brain stimulations such as repetitive transcranial magnetic stimulation (74)]. Improved knowledge about the spatial distribution of these abnormalities may help localize the brain targets better. Indeed, previous studies have already shown how the efficacy of such therapies depends on the exact position of the brain target (75).
We acknowledge that the novel approach has several limitations. The first relates to the debatable nosology of current mental disorders, based on clinical consensus rather than known biological underpinnings. We know, for example, that major psychiatric disorders share some genetic risk factors, and there are high percentages of comorbidity and diagnostic change. However, this does not mean that there are no disorder-specific brain correlates. As noted above, diagnostic labels are among the best predictors of future outcomes, highlighting their clinical relevance. The second relates to the commonly poor reporting of some comorbid disorders in the literature and the subthreshold disorder-specific symptoms that we will not consider due to the complexity of the analysis and the expected large amount of missing data. For instance, studies may check for schizophrenia but not for personality disorders. For this reason, we will conduct subgroup analyses excluding the disorders under-reported as comorbidities. A third limitation is that, as stated earlier, some studies may not report the proportion of patients with specific comorbidities. Thus, we will have to use multiple imputation. We will use a simplistic imputation algorithm without considering whether the proportion of patients with a given comorbid disorder may depend on the age or symptom severity of the sample. We preferred this simple algorithm because we anticipate that we would not be able to collect the necessary data for robustly using more complex imputation algorithms. Another limitation is that, as in any other meta-analysis, a potential drawback may be the heterogeneity across studies. Considering comorbidity, age, sex, and medication, we aim to explain the heterogeneity more than previous meta-analyses, but we anticipate that there will still be unexplained heterogeneity. A significant source of heterogeneity may be due to differences in the MRI equipment (e.g., varying field strength or head coils) and acquisition parameters (76) and the VBM processing method employed by the different studies, such as software and version, normalization, statistical correction, or the size of the smoothing kernel (19). Another relevant source of heterogeneity may be different subject-specific artifacts such as head motion (77), body mass index (78), drops in signal-to-noise ratio due to susceptibility artifacts, the symptom severity of the disorders, or the different phases present in some disorders (e.g., the various episodes in BD) (79, 80). Also, we will only study those mental disorders for which a meta-analysis has already been published and examined by at least ten studies. Last but not least, we must highlight that even when, for simplicity, we talk about GM volume abnormalities, we should more appropriately refer to differences in T1-MRI signal, given that the acquired MRI data are not a direct measure of brain structure (81).
Author Contributions
LF, AA-E, and JR conceived the study, modified the SDM-PSI software, and validated the novel approach. All authors participated in the redaction of the manuscript, including minor or major modifications of the protocol.
Funding
This work was supported by several grants from the Instituto de Salud Carlos III - Subdirección General de Evaluación y Fomento de la Investigación: Research Project Grant (PI19/00394 to JR), Miguel Servet Research Contract (CPII19/0009 to JR), and the PFIS contract (FI20/00047 to LF). NV thanks the BITRECS project, which has received funding from the European Union's Horizon 2020 research and innovation programme under the Marie Skłodowska-Curie Grant Agreement No 754550 and from La Caixa Foundation (ID 100010434), under the agreement LCF/PR/GN18/50310006.
Conflict of Interest
NV has received financial support for CME activities and travel funds from the following entities (unrelated to the present work): Angelini, Janssen-Cilag, Lundbeck, Otsuka. EVia is a co-investigator in a Janssen-Cilag, S.A., clinical trial with the esketamine molecule (unrelated to the present work). KR received a grant from TAKEDA pharmaceutical for another project and consulting fees from Lundbeck and Supernus (unrelated to the present work).
The remaining authors declare that the research was conducted in the absence of any commercial or financial relationships that could be construed as a potential conflict of interest.
Publisher's Note
All claims expressed in this article are solely those of the authors and do not necessarily represent those of their affiliated organizations, or those of the publisher, the editors and the reviewers. Any product that may be evaluated in this article, or claim that may be made by its manufacturer, is not guaranteed or endorsed by the publisher.
Acknowledgments
We thank all the participants of the studies that we will include in the meta-analysis.
References
1. DeLisi LE, Goldin LR, Hamovit JR, Maxwell ME, Kurtz D, Nurnberger JI, et al. Cerebral ventricular enlargement as a possible genetic marker for schizophrenia. Psychopharmacol Bull. (1985) 21:365–7.
2. Brühl AB, Delsignore A, Komossa K, Weidt S. Neuroimaging in social anxiety disorder—a meta-analytic review resulting in a new neurofunctional model. Neurosci Biobehav Rev. (2014) 47:260–80. doi: 10.1016/j.neubiorev.2014.08.003
3. Brühl AB, Hänggi J, Baur V, Rufer M, Delsignore A, Weidt S, et al. Increased cortical thickness in a frontoparietal network in social anxiety disorder: increased cortical thickness in SAD. Hum Brain Mapp. (2014) 35:2966–77. doi: 10.1002/hbm.22378
4. Irle E. Reduced amygdalar and hippocampal size in adults with generalized social phobia. J Psychiatry Neurosci. (2010) 35:126–31. doi: 10.1503/jpn.090041
5. Irle E, Barke A, Lange C, Ruhleder M. Parietal abnormalities are related to avoidance in social anxiety disorder: a study using voxel-based morphometry and manual volumetry. Psychiatry Res Neuroimaging. (2014) 224:175–83. doi: 10.1016/j.pscychresns.2014.08.013
6. Bas-Hoogendam JM, van Steenbergen H, Nienke Pannekoek J, Fouche J-P, Lochner C, Hattingh CJ, et al. Voxel-based morphometry multi-center mega-analysis of brain structure in social anxiety disorder. Neuroimage Clin. (2017) 16:678–88. doi: 10.1016/j.nicl.2017.08.001
7. Rotge J-Y, Langbour N, Guehl D, Bioulac B, Jaafari N, Allard M, et al. Gray matter alterations in obsessive–compulsive disorder: an anatomic likelihood estimation meta-analysis. Neuropsychopharmacol. (2010) 35:686–91. doi: 10.1038/npp.2009.175
8. Tang W, Zhu Q, Gong X, Zhu C, Wang Y, Chen S. Cortico-striato-thalamo-cortical circuit abnormalities in obsessive-compulsive disorder: a voxel-based morphometric and fMRI study of the whole brain. Behav Brain Res. (2016) 313:17–22. doi: 10.1016/j.bbr.2016.07.004
9. Picó-Pérez M, Moreira PS, de Melo Ferreira V, Radua J, Mataix-Cols D, Sousa N, et al. Modality-specific overlaps in brain structure and function in obsessive-compulsive disorder: multimodal meta-analysis of case-control MRI studies. Neurosci Biobehav Rev. (2020) 112:83–94. doi: 10.1016/j.neubiorev.2020.01.033
10. de Wit SJ, Alonso P. Multicenter voxel-based morphometry mega-analysis of structural brain scans in obsessive-compulsive disorder. Am J Psychiatry. (2014) 171:340–9. doi: 10.1176/appi.ajp.2013.13040574
11. Radua J, Mataix-Cols D. Voxel-wise meta-analysis of grey matter changes in obsessive–compulsive disorder. Br J Psychiatry. (2009) 195:393–402. doi: 10.1192/bjp.bp.108.055046
12. Eng GK, Sim K, Chen S-HA. Meta-analytic investigations of structural grey matter, executive domain-related functional activations, and white matter diffusivity in obsessive compulsive disorder: an integrative review. Neurosci Biobehav Rev. (2015) 52:233–57. doi: 10.1016/j.neubiorev.2015.03.002
13. Hoexter MQ, de Souza Duran FL, D'Alcante CC, Dougherty DD, Shavitt RG, Lopes AC, et al. Gray matter volumes in obsessive-compulsive disorder before and after fluoxetine or cognitive-behavior therapy: a randomized clinical trial. Neuropsychopharmacology. (2012) 37:734–45. doi: 10.1038/npp.2011.250
14. Togao O, Yoshiura T, Nakao T, Nabeyama M, Sanematsu H, Nakagawa A, et al. Regional gray and white matter volume abnormalities in obsessive–compulsive disorder: a voxel-based morphometry study. Psychiatry Res Neuroimaging. (2010) 184:29–37. doi: 10.1016/j.pscychresns.2010.06.011
15. Szeszko PR, Christian C, MacMaster F, Lencz T, Mirza Y, Taormina SP, et al. Gray matter structural alterations in psychotropic drug-naive pediatric obsessive-compulsive disorder: An optimized voxel-based morphometry study. AJP. (2008) 165:1299–307. doi: 10.1176/appi.ajp.2008.08010033
16. Christian CJ, Lencz T, Robinson DG, Burdick KE, Ashtari M, Malhotra AK, et al. Gray matter structural alterations in obsessive–compulsive disorder: relationship to neuropsychological functions. Psychiatry Res Neuroimaging. (2008) 164:123–31. doi: 10.1016/j.pscychresns.2008.03.005
17. Pardoe H, Pell GS, Abbott DF, Berg AT, Jackson GD. Multi-site voxel-based morphometry: methods and a feasibility demonstration with childhood absence epilepsy. Neuroimage. (2008) 42:611–6. doi: 10.1016/j.neuroimage.2008.05.007
18. Focke NK, Helms G, Kaspar S, Diederich C, Tóth V, Dechent P, et al. Multi-site voxel-based morphometry — not quite there yet. Neuroimage. (2011) 56:1164–70. doi: 10.1016/j.neuroimage.2011.02.029
19. Henley SMD, Ridgway GR, Scahill RI, Klöppel S, Tabrizi SJ, Fox NC, et al. Pitfalls in the use of voxel-based morphometry as a biomarker: examples from Huntington disease. AJNR Am J Neuroradiol. (2010) 31:711–9. doi: 10.3174/ajnr.A1939
20. Truong W, Minuzzi L, Soares CN, Frey BN, Evans AC, MacQueen GM, et al. Changes in cortical thickness across the lifespan in major depressive disorder. Psychiatry Res Neuroimaging. (2013) 214:204–11. doi: 10.1016/j.pscychresns.2013.09.003
21. Liloia D, Brasso C, Cauda F, Mancuso L, Nani A, Manuello J, et al. Updating and characterizing neuroanatomical markers in high-risk subjects, recently diagnosed and chronic patients with schizophrenia: a revised coordinate-based meta-analysis. Neurosci Biobehav Rev. (2021) 123:83–103. doi: 10.1016/j.neubiorev.2021.01.010
22. Schmaal L, Hibar DP, Sämann PG, Hall GB, Baune BT, Jahanshad N, et al. Cortical abnormalities in adults and adolescents with major depression based on brain scans from 20 cohorts worldwide in the ENIGMA Major Depressive Disorder Working Group. Mol Psychiatry. (2017) 22:900–9. doi: 10.1038/mp.2016.60
23. Koutsouleris N, Davatzikos C, Borgwardt S, Gaser C, Bottlender R, Frodl T, et al. Accelerated brain aging in schizophrenia and beyond: a neuroanatomical marker of psychiatric disorders. Schizophr Bull. (2014) 40:1140–53. doi: 10.1093/schbul/sbt142
24. Bora E, Fornito A, Yücel M, Pantelis C. The effects of gender on grey matter abnormalities in major psychoses: a comparative voxelwise meta-analysis of schizophrenia and bipolar disorder. Psychol Med. (2012) 42:295–307. doi: 10.1017/S0033291711001450
25. Torres US, Portela-Oliveira E, Borgwardt S, Busatto GF. Structural brain changes associated with antipsychotic treatment in schizophrenia as revealed by voxel-based morphometric MRI: an activation likelihood estimation meta-analysis. BMC Psychiatry. (2013) 13:342. doi: 10.1186/1471-244X-13-342
26. Zhao Y-J, Du M-Y, Huang X-Q, Lui S, Chen Z-Q, Liu J, et al. Brain grey matter abnormalities in medication-free patients with major depressive disorder: a meta-analysis. Psychol Med. (2014) 44:2927–37. doi: 10.1017/S0033291714000518
27. Fusar-Poli P, Salazar de Pablo G, Correll CU, Meyer-Lindenberg A, Millan MJ, Borgwardt S, et al. Prevention of psychosis: advances in detection, prognosis, and intervention. JAMA Psychiatry. (2020) 77:755. doi: 10.1001/jamapsychiatry.2019.4779
28. Nakao T, Radua J, Rubia K, Mataix-Cols D. Gray matter volume abnormalities in ADHD: voxel-based meta-analysis exploring the effects of age and stimulant medication. AJP. (2011) 168:1154–63. doi: 10.1176/appi.ajp.2011.11020281
29. Fusar-Poli P, Solmi M, Brondino N, Davies C, Chae C, Politi P, et al. Transdiagnostic psychiatry: a systematic review. World Psychiatry. (2019) 18:192–207. doi: 10.1002/wps.20631
30. Kessler RC, Chiu WT, Demler O, Walters EE. Prevalence, Severity, and Comorbidity of Twelve-month DSM-IV disorders in the national comorbidity survey replication (NCS-R). Arch Gen Psychiatry. (2005) 62:617–27. doi: 10.1001/archpsyc.62.6.617
31. Caspi A, Houts RM, Ambler A, Danese A, Elliott ML, Hariri A, et al. Longitudinal assessment of mental health disorders and comorbidities across 4 decades among participants in the dunedin birth cohort study. JAMA Netw Open. (2020) 3:e203221. doi: 10.1001/jamanetworkopen.2020.3221
32. Perugi G, Barbuti M. There are no patients without comorbidity. Eur Neuropsychopharmacol. (2021) 50:104–6. doi: 10.1016/j.euroneuro.2021.05.002
33. Carlisi CO, Norman LJ, Lukito SS, Radua J, Mataix-Cols D, Rubia K. Comparative multimodal meta-analysis of structural and functional brain abnormalities in autism spectrum disorder and obsessive-compulsive disorder. Biol Psychiatry. (2017) 82:83–102. doi: 10.1016/j.biopsych.2016.10.006
34. Norman LJ, Carlisi C, Lukito S, Hart H, Mataix-Cols D, Radua J, et al. Structural and functional brain abnormalities in attention-deficit/hyperactivity disorder and obsessive-compulsive disorder: a comparative meta-analysis. JAMA Psychiatry. (2016) 73:815. doi: 10.1001/jamapsychiatry.2016.0700
35. Radua J, Borgwardt S, Crescini A, Mataix-Cols D, Meyer-Lindenberg A, McGuire PK, et al. Multimodal meta-analysis of structural and functional brain changes in first episode psychosis and the effects of antipsychotic medication. Neurosci Biobehav Rev. (2012) 36:2325–33. doi: 10.1016/j.neubiorev.2012.07.012
36. Radua J, van den Heuvel OA, Surguladze S, Mataix-Cols D. Meta-analytical comparison of voxel-based morphometry studies in obsessive-compulsive disorder vs other anxiety disorders. Arch Gen Psychiatry. (2010) 67:701–11. doi: 10.1001/archgenpsychiatry.2010.70
37. Wise T, Radua J, Via E, Cardoner N, Abe O, Adams TM, et al. Common and distinct patterns of grey-matter volume alteration in major depression and bipolar disorder: evidence from voxel-based meta-analysis. Mol Psychiatry. (2017) 22:1455–63. doi: 10.1038/mp.2016.72
38. Opel N, Goltermann J, Hermesdorf M, Berger K, Baune BT, Dannlowski U. Cross-disorder analysis of brain structural abnormalities in six major psychiatric disorders: a secondary analysis of mega- and meta-analytical findings from the ENIGMA consortium. Biol Psychiatry. (2020) 88:678–86. doi: 10.1016/j.biopsych.2020.04.027
39. Arnone D, Cavanagh J, Gerber D, Lawrie SM, Ebmeier KP, McIntosh AM. Magnetic resonance imaging studies in bipolar disorder and schizophrenia: meta-analysis. Br J Psychiatry. (2009) 195:194–201. doi: 10.1192/bjp.bp.108.059717
40. Radua J, Mataix-Cols D, Phillips ML, El-Hage W, Kronhaus DM, Cardoner N, et al. A new meta-analytic method for neuroimaging studies that combines reported peak coordinates and statistical parametric maps. Eur Psychiatry. (2012) 27:605–11. doi: 10.1016/j.eurpsy.2011.04.001
41. Albajes-Eizagirre A, Solanes A, Vieta E, Radua J. Voxel-based meta-analysis via permutation of subject images (PSI): theory and implementation for SDM. Neuroimage. (2019) 186:174–84. doi: 10.1016/j.neuroimage.2018.10.077
42. Albajes-Eizagirre A, Solanes A, Fullana MA, Ioannidis JPA, Fusar-Poli P, Torrent C, et al. Meta-analysis of voxel-based neuroimaging studies using Seed-based d Mapping with Permutation of Subject Images (SDM-PSI). J Vis Exp. (2019) 153. doi: 10.3791/59841
43. Webler RD, Berg H, Fhong K, Tuominen L, Holt DJ, Morey RA, et al. The neurobiology of human fear generalization: meta-analysis and working neural model. Neurosci Biobehav Rev. (2021) 128:421–36. doi: 10.1016/j.neubiorev.2021.06.035
44. Pezzoli S, Sánchez-Valle R, Solanes A, Kempton MJ, Bandmann O, Shin JI, et al. Neuroanatomical and cognitive correlates of visual hallucinations in Parkinson's disease and dementia with Lewy bodies: voxel-based morphometry and neuropsychological meta-analysis. Neurosci Biobehav Rev. (2021) 128:367–82. doi: 10.1016/j.neubiorev.2021.06.030
45. Del Fabro L, Schmidt A, Fortea L, Delvecchio G, D'Agostino A, Radua J, et al. Functional brain network dysfunctions in subjects at high-risk for psychosis: a meta-analysis of resting-state functional connectivity. Neurosci Biobehav Rev. (2021) 128:90–101. doi: 10.1016/j.neubiorev.2021.06.020
46. Jauhar S, Fortea L, Solanes A, Albajes-Eizagirre A, McKenna PJ, Radua J. Brain activations associated with anticipation and delivery of monetary reward: a systematic review and meta-analysis of fMRI studies. PLoS ONE. (2021) 16:e0255292. doi: 10.1371/journal.pone.0255292
47. Fortea A, Batalla A, Radua J, van Eijndhoven P, Baeza I, Albajes-Eizagirre A, et al. Cortical gray matter reduction precedes transition to psychosis in individuals at clinical high-risk for psychosis: a voxel-based meta-analysis. Schizophr Res. (2021) 232:98–106. doi: 10.1016/j.schres.2021.05.008
48. Gharehgazlou A, Freitas C, Ameis SH, Taylor MJ, Lerch JP, Radua J, et al. Cortical gyrification morphology in individuals with ASD and ADHD across the lifespan: a systematic review and meta-analysis. Cerebral Cortex. (2021) 31:2653–69. doi: 10.1093/cercor/bhaa381
49. Sheng L, Zhao P, Ma H, Radua J, Yi Z, Shi Y, et al. Cortical thickness in Parkinson's disease: a coordinate-based meta-analysis. Aging. (2021) 13:4007–23. doi: 10.18632/aging.202368
50. Soldevila-Matías P, Schoretsanitis G, Tordesillas-Gutierrez D, Cuesta MJ, de Filippis R, Ayesa-Arriola R, et al. Neuroimaging correlates of insight in non-affective psychosis: a systematic review and meta-analysis. Rev Psiquiatr Salud Mental. (in press). doi: 10.1016/j.rpsm.2021.07.001
51. Dugré JR, Radua J, Carignan-Allard M, Dumais A, Rubia K, Potvin S. Neurofunctional abnormalities in antisocial spectrum: a meta-analysis of fMRI studies on Five distinct neurocognitive research domains. Neurosci Biobehav Rev. (2020) 119:168–83. doi: 10.1016/j.neubiorev.2020.09.013
52. Albajes-Eizagirre A, Radua J. What do results from coordinate-based meta-analyses tell us? NeuroImage. (2018) 176:550–3. doi: 10.1016/j.neuroimage.2018.04.065
53. Radua J, Rubia K, Canales-Rodríguez EJ, Pomarol-Clotet E, Fusar-Poli P, Mataix-Cols D. Anisotropic kernels for coordinate-based meta-analyses of neuroimaging studies. Front Psychiatry. (2014) 5:13. doi: 10.3389/fpsyt.2014.00013
54. Smith S, Nichols T. Threshold-free cluster enhancement: addressing problems of smoothing, threshold dependence and localisation in cluster inference. Neuroimage. (2009) 44:83–98. doi: 10.1016/j.neuroimage.2008.03.061
55. Hamilton M. A rating scale for depression. J Neurol Neurosurg Psychiatry. (1960) 23:56–62. doi: 10.1136/jnnp.23.1.56
56. Wells G, Shea B, O'Connell J. The Newcastle-Ottawa Scale (NOS) for Assessing The Quality of Nonrandomised Studies in Meta-analyses. Ottawa Health Research Institute Web site (2014).
57. van den Heuvel OA, Remijnse PL, Mataix-Cols D, Vrenken H, Groenewegen HJ, Uylings HBM, et al. The major symptom dimensions of obsessive-compulsive disorder are mediated by partially distinct neural systems. Brain. (2008) 132:853–68. doi: 10.1093/brain/awn267
58. Pujol J, Soriano-Mas C, Alonso P, Cardoner N, Menchón JM, Deus J, et al. Mapping structural brain alterations in obsessive-compulsive disorder. Arch Gen Psychiatry. (2004) 61:720. doi: 10.1001/archpsyc.61.7.720
59. Rubin DB. Multiple Imputation for Nonresponse in Surveys. New York: John Wiley & Sons Inc. (1987). doi: 10.1002/9780470316696
60. Winkler AM, Ridgway GR, Webster MA, Smith SM, Nichols TE. Permutation inference for the general linear model. NeuroImage. (2014) 92:381–97. doi: 10.1016/j.neuroimage.2014.01.060
61. Ioannidis JPA, Patsopoulos NA, Evangelou E. Uncertainty in heterogeneity estimates in meta-analyses. BMJ. (2007) 335:914–6. doi: 10.1136/bmj.39343.408449.80
62. Bora E, Fornito A, Radua J, Walterfang M, Seal M, Wood SJ, et al. Neuroanatomical abnormalities in schizophrenia: a multimodal voxelwise meta-analysis and meta-regression analysis. Schizophr Res. (2011) 127:46–57. doi: 10.1016/j.schres.2010.12.020
63. Ellison-Wright I, Glahn DC, Laird AR, Thelen SM, Bullmore E. The anatomy of first-episode and chronic schizophrenia: an anatomical likelihood estimation meta-analysis. AJP. (2008) 165:1015–23. doi: 10.1176/appi.ajp.2008.07101562
64. Rive MM, van Rooijen G, Veltman DJ, Phillips ML, Schene AH, Ruhé HG. Neural correlates of dysfunctional emotion regulation in major depressive disorder. A systematic review of neuroimaging studies. Neurosci Biobehav Rev. (2013) 37:2529–53. doi: 10.1016/j.neubiorev.2013.07.018
65. Kempton MJ, Salvador Z, Munafò MR, Geddes JR, Simmons A, Frangou S, et al. Structural neuroimaging studies in major depressive disorder: meta-analysis and comparison with bipolar disorder. Arch Gen Psychiatry. (2011) 68:675. doi: 10.1001/archgenpsychiatry.2011.60
66. Arnone D, McIntosh AM, Ebmeier KP, Munafò MR, Anderson IM. Magnetic resonance imaging studies in unipolar depression: systematic review and meta-regression analyses. Eur Neuropsychopharmacol. (2012) 22:1–16. doi: 10.1016/j.euroneuro.2011.05.003
67. Arnone D, Job D, Selvaraj S, Abe O, Amico F, Cheng Y, et al. Computational meta-analysis of statistical parametric maps in major depression: computational meta-analysis of parametric maps in major depression. Hum Brain Mapp. (2016) 37:1393–404. doi: 10.1002/hbm.23108
68. Selvaraj S, Arnone D, Job D, Stanfield A, Farrow TF, Nugent AC, et al. Grey matter differences in bipolar disorder: a meta-analysis of voxel-based morphometry studies: grey matter differences in bipolar disorder. Bipolar Disord. (2012) 14:135–45. doi: 10.1111/j.1399-5618.2012.01000.x
69. Pezzoli S, Emsell L, Yip SW, Dima D, Giannakopoulos P, Zarei M, et al. Meta-analysis of regional white matter volume in bipolar disorder with replication in an independent sample using coordinates, T-maps, and individual MRI data. Neurosci Biobehav Rev. (2018) 84:162–70. doi: 10.1016/j.neubiorev.2017.11.005
70. Lukito S, Norman L, Carlisi C, Radua J, Hart H, Simonoff E, et al. Comparative meta-analyses of brain structural and functional abnormalities during cognitive control in attention-deficit/hyperactivity disorder and autism spectrum disorder. Psychol Med. (2020) 50:894–919. doi: 10.1017/S0033291720000574
71. Shang J, Fu Y, Ren Z, Zhang T, Du M, Gong Q, et al. The common traits of the ACC and PFC in anxiety disorders in the DSM-5: meta-analysis of voxel-based morphometry studies. PLoS ONE. (2014) 9:e93432. doi: 10.1371/journal.pone.0093432
72. Wang X, Cheng B, Wang S, Lu F, Luo Y, Long X, et al. Distinct grey matter volume alterations in adult patients with panic disorder and social anxiety disorder: a systematic review and voxel-based morphometry meta-analysis. J Affect Disord. (2021) 281:805–23. doi: 10.1016/j.jad.2020.11.057
73. Wu Y, Zhong Y, Ma Z, Lu X, Zhang N, Fox PT, et al. Gray matter changes in panic disorder: a voxel-based meta-analysis and meta-analytic connectivity modeling. Psychiatry Res Neuroimaging. (2018) 282:82–9. doi: 10.1016/j.pscychresns.2018.09.009
74. Padberg F, Bulubas L, Mizutani-Tiebel Y, Burkhardt G, Kranz GS, Koutsouleris N, et al. The intervention, the patient and the illness – personalizing non-invasive brain stimulation in psychiatry. Exp Neurol. (2021) 341:113713. doi: 10.1016/j.expneurol.2021.113713
75. Puigdemont D, Pérez-Egea R, Portella MJ, Molet J, de Diego-Adeliño J, Gironell A, et al. Deep brain stimulation of the subcallosal cingulate gyrus: further evidence in treatment-resistant major depression. Int J Neuropsychopharmacol. (2012) 15:121–33. doi: 10.1017/S1461145711001088
76. Chiò A, Pagani M, Agosta F, Calvo A, Cistaro A, Filippi M. Neuroimaging in amyotrophic lateral sclerosis: insights into structural and functional changes. Lancet Neurol. (2014) 13:1228–40. doi: 10.1016/S1474-4422(14)70167-X
77. Zaitsev M, Maclaren J, Herbst M. Motion artifacts in MRI: a complex problem with many partial solutions: motion artifacts and correction. J Magn Reson Imaging. (2015) 42:887–901. doi: 10.1002/jmri.24850
78. McWhinney SR, Abé C, Alda M, Benedetti F, Bøen E, del Mar Bonnin C, et al. Association between body mass index and subcortical brain volumes in bipolar disorders–ENIGMA study in 2735 individuals. Mol Psychiatry. (in press). doi: 10.1038/s41380-021-01098-x
79. Carvalho AF, Firth J, Vieta E. Bipolar disorder. N Engl J Med. (2020) 383:58–66. doi: 10.1056/NEJMra1906193
80. Burdick KE, Millett CE. Cognitive heterogeneity is a key predictor of differential functional outcome in patients with bipolar disorder. Eur Neuropsychopharmacol. (2021) 53:4–6. doi: 10.1016/j.euroneuro.2021.06.008
Keywords: meta-analysis, magnetic resonance imaging (MRI), seed-based d mapping (SDM), gray matter (GM), mental disorder, comorbidity, medication
Citation: Fortea L, Albajes-Eizagirre A, Yao Y-W, Soler E, Verdolini N, Hauson AO, Fortea A, Madero S, Solanes A, Wollman SC, Serra-Blasco M, Wise T, Lukito S, Picó-Pérez M, Carlisi C, Zhang J, Pan P, Farré-Colomés Á, Arnone D, Kempton MJ, Soriano-Mas C, Rubia K, Norman L, Fusar-Poli P, Mataix-Cols D, Valentí M, Via E, Cardoner N, Solmi M, Shin JI, Vieta E and Radua J (2022) Focusing on Comorbidity—A Novel Meta-Analytic Approach and Protocol to Disentangle the Specific Neuroanatomy of Co-occurring Mental Disorders. Front. Psychiatry 12:807839. doi: 10.3389/fpsyt.2021.807839
Received: 02 November 2021; Accepted: 13 December 2021;
Published: 18 January 2022.
Edited by:
Stefan Borgwardt, University of Lübeck, GermanyReviewed by:
Felix Brandl, Technical University of Munich, GermanyAndreea Oliviana Diaconescu, Krembil Centre for Neuroinformatics, Centre for Addiction and Mental Health, Canada
Copyright © 2022 Fortea, Albajes-Eizagirre, Yao, Soler, Verdolini, Hauson, Fortea, Madero, Solanes, Wollman, Serra-Blasco, Wise, Lukito, Picó-Pérez, Carlisi, Zhang, Pan, Farré-Colomés, Arnone, Kempton, Soriano-Mas, Rubia, Norman, Fusar-Poli, Mataix-Cols, Valentí, Via, Cardoner, Solmi, Shin, Vieta and Radua. This is an open-access article distributed under the terms of the Creative Commons Attribution License (CC BY). The use, distribution or reproduction in other forums is permitted, provided the original author(s) and the copyright owner(s) are credited and that the original publication in this journal is cited, in accordance with accepted academic practice. No use, distribution or reproduction is permitted which does not comply with these terms.
*Correspondence: Joaquim Radua, cmFkdWEmI3gwMDA0MDtjbGluaWMuY2F0