- 1Institute of Neuroscience and Psychology, University of Glasgow, Glasgow, United Kingdom
- 2Tel-Aviv Sourasky Medical Center, Sagol Brain Institute Tel-Aviv, Wohl Institute for Advanced Imaging, Tel-Aviv, Israel
- 3Sagol School of Neuroscience, Tel-Aviv University, Tel-Aviv, Israel
- 4Departments of Comparative Medicine and Psychiatry, Yale School of Medicine, Yale University, New Haven, CT, United States
- 5The Clinical Neurosciences Division, VA Connecticut Healthcare System, United States Department of Veterans Affairs, National Center for Posttraumatic Stress Disorder, West Haven, CT, United States
- 6Department of Cognitive Science, Macquarie University, Sydney, NSW, Australia
Post-Traumatic Stress Disorder (PTSD) is a severe psychiatric disorder with profound public health impact due to its high prevalence, chronic nature, accompanying functional impairment, and frequently occurring comorbidities. Early PTSD symptoms, often observed shortly after trauma exposure, abate with time in the majority of those who initially express them, yet leave a significant minority with chronic PTSD. While the past several decades of PTSD research have produced substantial knowledge regarding the mechanisms and consequences of this debilitating disorder, the diagnosis of and available treatments for PTSD still face significant challenges. Here, we discuss how novel therapeutic interventions involving social robots can potentially offer meaningful opportunities for overcoming some of the present challenges. As the application of social robotics-based interventions in the treatment of mental disorders is only in its infancy, it is vital that careful, well-controlled research is conducted to evaluate their efficacy, safety, and ethics. Nevertheless, we are hopeful that robotics-based solutions could advance the quality, availability, specificity and scalability of care for PTSD.
1. Introduction
Stress occurs when our dynamic biological and/or psychological equilibrium is threatened or perceived to be threatened (1, 2). The feeling of stress is prevalent and ubiquitous in our everyday lives, significantly impacting the maintenance of both physical and mental health (3), with increasing social and economic costs (4). Critically, even a single stressful event, if perceived as life-threatening (i.e., traumatic), can lead to longstanding psychopathology as exemplified by Post-Traumatic Stress Disorder (PTSD) (5). PTSD is a prevalent and severe psychiatric disorder with profound public health impact due to its chronic nature, accompanying functional impairment, and highly common comorbidities (6, 7). Existing therapeutics for PTSD show limited efficacy, presumably because they do not meet minimal quality criteria or because they attempt to treat rather than prevent the disorder (8). Furthermore, many PTSD treatments were developed without directly targeting the specific underlying mechanisms (2, 9). As both PTSD diagnosis and treatment still face significant challenges, here we aim to highlight how a novel technological solution, namely, social robots, might be able to offer assistance in the diagnosis and treatment of PTSD.
Digitization in psychiatry is gaining momentum, providing those who suffer from low mental health with an increasing array of self-help solutions, many of which are available on users' mobile devices (see 10–12). PTSD diagnosis and treatment can take many different forms, ranging from traditional questionnaires (see 13, 14) to ecological momentary assessment (EMA) (e.g., 15, 16) and intervention (EMI) (e.g., 16–18), mobile applications, virtual agents (e.g., 19–22), and exposure treatments using virtual reality (VR) devices (e.g., 23, 24). However, in the following, we argue that social robots offer another promising approach for supporting PTSD diagnosis and treatment, due to their availability, autonomy, and embodiment. Hence, this perspective paper specifically focuses on the potential application of social robots for PTSD diagnosis and treatment.
The definition of a social robot in this work is an autonomous machine that interacts and communicates with humans or other agents by following social behaviors and rules relevant to their role (25). Furthermore, for the purposes of this paper, we further include in our social robots definition that these machines are autonomous, and function in physical and social spaces alongside humans (26) (see Figure 1 for examples). In this paper, we wish to particularly emphasize the relevance and value of social robots' physical embodiment, as we believe this offers additional benefits beyond other digital and AI-fuelled innovations that are screen or voice-based. Social robots are attracting increasing attention for their potential use in autonomous health interventions (26). Such robots are already being applied in psychosocial interventions (27, 28), in mental health settings (29), and deployed as supportive agents to aid in rehabilitation (30, 31). Due to many social robots features, including human-like design (32–35), autonomous abilities, and high mobility (26), we suggest that some of these machines could also be well-suited to helping overcome some of the challenges of PTSD diagnosis and treatment. In this paper, we propose a novel approach for the integration of social robots in PTSD clinical management, in order to improve diagnosis and early interventions aiming to prevent and/or treat post-traumatic psychopathology. In the following, we introduce some major challenges faced in PTSD diagnosis and treatment, present an overview of recent developments in social robotics research, and reflect on the potential of these robotics developments to overcome PTSD diagnosis and treatment challenges.
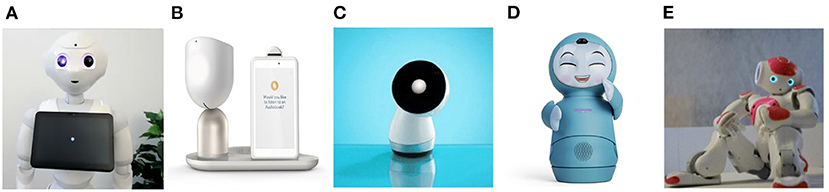
Figure 1. Examples of several social robotics platforms that are heavily used in research and/or have enjoyed commercial success, and are discussed in this perspective paper. (A) Pepper, a humanoid by SoftBank Robotics. (B) ElliQ, a household robot by Intuition Robotics. (C) Jibo, a personal home assistant robot by NTT Disruption. (D) Moxi, an animated household robot by Embodied. (E) Nao, a humanoid robot and Pepper's little sibling by SoftBank Robotics.
2. Challenges for PTSD Diagnosis and Treatment
Challenges for PTSD diagnosis and treatment include the short critical time-frame for intervention after trauma exposure (36, 37); the high dependency on available qualified medical teams and trauma specialists in emergency departments (38–40); and the limited efficacy and high dropout rates of current first line pharmacological and psychological treatments (41). Moreover, when patients overcome the physical consequences resulting from the traumatic event, they often end or limit their relationship with the medical team, resulting in diminished compliance with or cessation of mental health recommendations (42).
PTSD is the only mental disorder to have a salient onset, thus providing an excellent target for secondary prevention and the mapping of pathogenic processes (43). Symptom trajectories provide an observable dimension of PTSD development or remission. Prospective studies of PTSD resulting from single traumatic incidents consistently show a progressive reduction in the symptoms' prevalence and severity during the year following trauma exposure (44–46). These early observed symptoms, seen shortly after trauma, subside in most of those initially expressing them and persist in about 30 percent of those diagnosed with PTSD 1 month after trauma (47, 48). Given the importance of interventions in the early aftermath of traumatic events, we suggest that early introduction of technology-assisted and self-help interventions should be further explored to prevent and treat post-traumatic psychopathology. Technology-assisted clinical interventions are becoming increasingly common in the health care field and in combating mental health as these interventions are often aimed at improving access to and cost-effectiveness of care (49–51).
3. Supporting early diagnosis in emergency departments
In emergency departments (ED) of general hospitals, diagnosing acute PTSD symptoms in trauma survivors can be a long complicated process, highly dependent on qualified human resources (e.g. trauma teams)(38–40). Moreover, the ED medical staff often focus mainly on physical injuries, prioritized by the degree of severity, disregarding mental health symptoms such as acute stress symptoms after trauma exposure. This results in patients not being diagnosed for Acute Stress Disorder (ASD) symptoms early after trauma, and receiving no intervention or treatment. If these acute stress symptoms persist for over a month after trauma exposure, the individuals are given the diagnosis of PTSD (14). Hence, it is highly important to assess early clinical symptoms shortly after trauma, and to follow-up on these throughout this early critical time-frame (36, 37). Furthermore, PTSD diagnosis rarely relies on formal and objective biological indicators, and instead indicated merely by subjective symptoms reported in clinician-administered interviews (14, 52). The clinician-administered PTSD Scale for DSM-5 (CAPS-5) is the current gold standard of PTSD assessment (14). It was designed to be administered by clinicians or clinical researchers who have a working knowledge of PTSD, but can also be administered by appropriately trained para-professionals. When necessary, to make a provisional PTSD diagnosis, the PTSD Checklist for DSM-5 (PCL-5), a 20-item self-report measure that assesses the 20 symptoms of PTSD defined by the DSM-5, can also be used (14).
While PTSD diagnosis is highly dependent on a clinician interpretation and expertise (38), it could nonetheless benefit from automation in the administration phase. For example, surveys of trauma survivors using self-reported measures (e.g., PCL-5 (13)) could be administer by a social robot, supporting human trauma teams in EDs after large-scale traumatic events, moving between trauma survivors and collecting relevant health data for diagnosing PTSD in an early stage. The current diagnosis procedure imposes a high data registration workload on medical staff, including nurses and clinicians who often demonstrate high rates of burnout due to the intense nature of EDs (53). This has serious implications, especially since trauma medical teams are often called on beyond normal working hours (54), and in case of large-scale traumatic events (e.g., large scale industrial accidents, natural disasters, terror attacks) with many trauma survivors (39). Medical teams in EDs could use the support of automatic systems to diagnose trauma survivors' mental condition in a fast and efficient way. This would provide them with time and energy to focus on other emergency medical procedures and will reduce their already-heavy workload. Trauma teams are a fundamental component for improving trauma-related care (40), hence, reducing the workload where possible can support trauma teams' performance in emergency situations. These common challenges faced in EDs and on site at traumatic events world-over also limit PTSD diagnosis, with only 7% of trauma centers reporting to be screening for PTSD (55).
Measures like the PCL-20 are constructed as self-reporting instruments that can be filled individually by trauma survivors. However, automation of some aspects of early trauma screening should also ensure that trauma survivors start the diagnosis procedure for PTSD during their hospitalization and in an early stage shortly after being exposed to a traumatic event, which should in turn reduce the patient burden of completing self-administered questionnaires in this critical time frame. As the PCL-5 is a straight-forward self-report tool that is easy to administer (13), a social robot should be able to communicate the items to most trauma survivors, and calculate a score on the spot. Moreover, due to the social robot's communication abilities, human-like design, and physical presence (see 26, 56), we propose that these self-reported items could be communicated in a more natural way. Rather than administering a questionnaire, a social robot could converse with a patient and elicit the necessary information naturally following the instrument's items (e.g., 34, 57). Using the robot's interactivity features, the social robot can update the system and prioritize fast and personalized reactions from relevant medical professionals (e.g., a psychiatrist, social worker, psychologist) for further elaborate diagnosis and early intervention.
Previous studies and ecologically valid study reports positive evidence for the use of social robots in autonomous health data acquisition among hospitalized patients. Moreover, these studies reported for social robots administrating health data acquisition in a variety of settings such as hospitals, homes, schools, and nursing homes (58–60). A randomized controlled cross-over trial with a social robot (Pepper, SoftBank Robotics, see Figure 1) and a nurse administering three questionnaires (52 questions in total) showed minimal differences in health data acquisition effectiveness between the two conditions (the Pepper robot vs. the nurse). Moreover, the study results demonstrated that the social robot was accepted by the patients (older adults) (58). The study suggests that social robots may effectively collect health data autonomously in public settings, and assist medical teams in diagnosing patients. As mentioned earlier, using a social robot for surveying trauma survivors via a self-reported tool can reduce the load on the trauma medical teams and ensure that relevant data for the diagnosis will be collected and reported in the relevant medical system on time for early intervention.
It is important to highlight that while there is vast evidence in the social robotics and human—robot interaction research literature for the effect of social robots on human's behavior in health settings (e.g., 28, 29, 61), and on self-disclosure in particular (34, 62–66), eliciting information from trauma survivors (especially regarding the trauma and the associated affect) is substantially different and will impose different and new challenges. This will require further investigation via future empirical research, as it is vital to understand disclosure to a social robot (and how different it is from disclosure to a human or disembodied technologies) when people are in a hyper-vulnerable state.
4. Overcoming logistical barriers
Following the administration of acute medical treatment, immediately following a trauma, several further logistical barriers exist that can prevent trauma survivors from receiving proper mental health diagnosis and intervention. These can be personal (e.g., living in rural areas with limited local mental health providers, limited mobility, language barriers, legal status, poor relationships and communication with providers, fragmentation of care) or professional (e.g., lack of support from the employer, lack of time, high responsibility, isolated employment) (67). These logistical barriers can be crucial considering the shortage of mental health professionals, especially in rural and difficult-to-access regions (4, 68, 69). An example is the barriers experienced by active-duty and ex-serving military personnel who suffer from PTSD. Studies involving active-duty infantry US soldiers demonstrate that 28% of soldiers met self-reported criteria for PTSD or major depressive disorder in the post-deployment period (70). Nevertheless, less than 40% of soldiers with mental health problems utilize mental health services, and only 50% seek intervention following a clinical referral (70). Active-duty soldiers report for logistical barriers when seeking mental health. These include difficulties in arranging appointments, lack of mental health professionals and/or limited availability in remote military bases, and lack of opportunities to see mental health professionals in their limited time outside military basis (71, 72). Importantly, active-duty soldiers are not the only people who suffer from limited access to mental health services. People who live in rural areas, or in regions with limited mobility, also frequently report having limited access to mental health services (4, 67, 73).
While a social robot can not and would not replace a human clinician in these settings, it could possibly be situated in these unique hard-to-reach environments, aiming to expand some aspects of mental health service delivery. These aspects include, but not limited to, local clinics in rural areas, far military bases, community centers, and homes of people with limited mobility. A social robot could collect health-related data in one's home or another familiar environment, monitor and report symptoms, and potentially offer early intervention in familiar settings. Deploying social robots in such a way could provide cost-effective mental health support, offering solutions to those with limited opportunities to access mental health services in their everyday settings. Accordingly, clinical symptoms of trauma survivors could be monitored early after trauma exposure (6, 74), and clinical teams could prioritize those who are at high-risk for PTSD development. This in turn would allow employment of early interventions aiming to prevent the development of the chronic disorder, which is more efficient than trying to treat chronic PTSD (8). Furthermore, small accessible social robotic devices designed for the home—such as ElliQ (Intuition Robotics), Moxi (Embodied), and Jibo (NTT Disruption) (see Figure 1)—could be placed in people's homes to monitor symptoms of trauma survivors with limited mobility. These social robots are easy to operate, easy to transport, and can elicit meaningful responses from humans in relevant settings (26, 75, 76).
A social robot in these settings can also be remotely operated by a clinician from afar, serving as a telepresence medium to provide access to professional mental health care providers in isolated settings (see 77, 78). For example, SoftBank Robotics recently introduced a new telepresence feature for their Pepper robot (79, 80). In contrast to other telepresence robots that are merely an extension of the telepresenced human, here the human telepresenced through the social robot shares a body with another social entity - such as Pepper, the humanoid social robot. This feature could introduce valuable opportunities for using social robots for PTSD, where they can perform both autonomously and/or be controlled by proxy. Therefore, aided by their physical embodiment, social robots offer the potential to provide human-mediated care by proxy as well as by using their autonomous programming to administer clinical management tasks independently when needed.
5. Overcoming Social Barriers
Extending from logistical barriers, social robots can also help to overcome social barriers for those seeking mental health treatment for PTSD. Some trauma survivors consider their hospitalization to be traumatic, hence they tend to avoid visiting or consulting with clinicians (81). Others avoid seeking mental health treatment at all due to personal internal social barriers such as stigma, isolation, stress, prejudice and feelings of shame (67, 82) associated with traumatic experiences (70, 82–86). Indeed, evidence of active-duty members and veterans demonstrating an unwillingness to discuss their mental health or emotions with medical teams due to prejudice and stigma has been well-documented (70, 82). Individuals with combat-related PTSD often feel strong negative emotions (e.g., anger, guilt, shame) in relation to the trauma and their subsequent mental condition. For examples, feeling of shame were associated with worse clinical outcomes in veterans with PTSD, specifically increase in suicidal ideation (85). Furthermore, sexual assault victims exhibited difficulties to discuss their traumatic events in both formal and informal settings (87). Finally, other individuals are not willing to receive mental health assistance mainly due to lack of support from family, friends, and their community (67).
We suggest that a social robot can potentially bypass some of the above-mentioned social barriers, and encourage individuals to report and treat their post-traumatic symptoms. We see compelling evidence for people being willing to disclose sensitive information, including stressors and mental health symptoms, to avatars and virtual agents. For example, a study by Utami et al. (19) explored the reactions of older adults when having “end-of-life” conversations with a virtual agent. The study's results show that all study participants were comfortable discussing with the agent about death anxiety, last will and testament, providing compelling evidence for the potential utility of artificial agents in these complex socioemotional domains. Another study by Lucas et al. (20) employed a virtual agent that affords anonymity while building rapport to interview active-duty service members about their mental health symptoms after they returned from a year-long deployment in Afghanistan. The study reports that participants disclosed more symptoms to a virtual agent interviewer than on the official Post-Deployment Health Assessment (PDHA), and than on an anonymized PDHA. Moreover, the results of a larger sample experiment with active-duty and former service members reported a similar effect (20).
Furthermore, another recent study (34) examined the extent to which social robot and disembodied conversational agent (voice assistant) can elicit rich disclosures, and accordingly might be used to support people's psychological health through conversation. The study reported that a social robot (NAO, SoftBank Robotics, see Figure 1) was successful in eliciting rich disclosures from human users, evidenced in the information that was shared, people's vocal output, and their perceptions of the interaction (34). This is in line with additional works that report different behavioral and emotional effects when communicating with social robots, and increased willingness of participants to disclose information and emotions in the presence of embodied artificial agents (e.g., 63, 65, 88–91). While participants were aware of many of the obvious differences between speaking to a humanoid social robot (NAO, SoftBank Robotics) compared to a disembodied conversational agent (Google Nest Mini voice assistant), their verbal disclosures to both were similar in length and duration (34). Another study (92) demonstrated positive responses of human users to a humanoid robot taking the role of couples counselor, aiming to promote positive communication. It is of note that the robot also played a meaningful role in mediating positive responses (in terms of behavior and affect) within the couples' dyadic interaction in this same study. While social robots obviously can not offer the same opportunities for social interaction and engagement as humans (33), their cognitive architectures and embodied cognition can nonetheless elicit socially meaningful behaviors from humans (93). As such, they can afford valuable opportunities for engagement with humans when introduced in specific contexts, and in careful ethically responsible ways (94).
6. Discussion and Conclusions
Through this paper, we aimed to introduce several challenges related to PTSD diagnosis and treatment, and highlight suitable opportunities to address them by introducing social robots in PTSD diagnosis and treatment. As it is crucial to diagnose acute PTSD early after trauma, social robots can support clinical assessments of trauma survivors during the hospitalization phase. They may also aid trauma teams in EDs by reducing some of their stress and burden during busy times (39, 40). As social robots can support high fidelity data collection and on-line, on-going analysis of human behavior, emotions, and physiological reactions, they might have the potential to support early diagnosis of PTSD among trauma survivors. Finally, social robots can assist with overcoming several logistical and social barriers that trauma survivors face when required to monitor symptoms and when seeking mental health interventions (67, 73, 81, 82).
We clearly acknowledge that various screen-based (or virtual), non-embodied technologies can also assist with some of the challenges, for example, via the use of EMA and EMI methodologies (e.g., 15, 17), or through use of a virtual agent on one's mobile device (e.g., 19, 20). While these kinds of tools and instruments might be useful and widely available, social robots provide an additional benefit through their embodiment, in that they have the potential to communicate and interact with people in a more socially meaningful way by initiating interactions more naturally than mobile devices, and providing rapid and responsive ecological momentary interventions in users' natural physical settings. While mobile apps require the user to take a certain initiative to log information, take action, or respond to a notification, social robots can elicit interactions more naturally due to their design, animated behavior, and social roles (see 26, 95, 96). This would be extremely helpful when monitoring symptoms for trauma survivors since they often prefer to refrain from discussing the trauma (67, 82). In fact, most EMA and EMI mobile solutions for self-monitoring are highly dependent on users' initiative and responsibility (see 97–99), which can be very challenging after experiencing a traumatic event. Moreover, EMA's and EMI's repetitive nature could be further triggering when addressing aspects related to traumatic events (see 98). Accordingly, social robots might just fall at the ideal intersection between being an autonomous and physically present technology that can capture emotion and information while also being able to demonstrate social and cognitive cues that might help to elicit rich and valuable disclosures from these patients. We do not argue that social robots are a perfect solution, but rather that they could potentially help overcome some of the barriers that other solutions might still struggle with.
It should also be mentioned that while social robots are indeed more expensive and less readily available than smartphone devices, they remain reasonably employable in social spaces (see 100), and can take on a cost-effective and user-friendly embodiment of a household robot (see 26, 75). To sum up, while smartphone applications have clear benefits in terms of availability, cost and scalability, in our perspective social robots' physical embodiment and cognitive architectures could support richer interactions with human users, which in turn could potentially help to overcome some of the logistical and emotional barriers of PTSD diagnosis and treatment.
To the best of our knowledge, no social robotics studies to date have been conducted with trauma survivors or individuals diagnosed with PTSD. Nonetheless, a study by Nomura and colleagues (101) provides evidence for the benefits of employing social robots for minimizing social tensions and anxieties. Through this work, the authors showed that participants with higher social anxiety tended to feel less anxious and demonstrated lower tensions when knowing that they would interact with a robot, as opposed to a human, in a service interaction. In addition, the authors suggested that an interaction with a robot elicited lower tensions compared to an interaction with another person, regardless of one's level of social anxiety. Extrapolating to PTSD settings, it is reasonable to assume that social robots might support trauma survivors with overcoming their social barriers to disclose and monitor their symptoms over time. Whether these robotic agents are situated at home, the local clinic, community center or hospital, they hold the potential to reach patients and invoke authentic reactions that could be critical for early diagnosis and treatment of PTSD.
Due to the lack of empirical work on this issue, in this perspective paper, our primary aim was to address the potential for introducing social robots for PTSD diagnosis and treatment, based on evidence gathered from a variety of different applications and perspectives from both clinical and non-clinical contexts. As such, we would like to stress that the ideas presented in this perspective paper are at a very early stage, based on studies with a variety of populations, and will need to be carefully and ethically tested before applying them to interventions with people in a hyper vulnerable state (such as those who experienced traumatic events or have already been diagnosed with PTSD). Studies looking into this should start by testing participants after experiencing trauma but without demonstrating PTSD symptoms or with subthreshold symptoms. When seeing good and valid results, studies could then carefully and responsibly move on to being replicated with clinical populations carefully. These sorts of trials should be accompanied/supervised or monitored by a mental health professional to ensure that participants do not experience negative effects.
Most of the preventive therapies for PTSD to date have been developed without directly documented neurocognitive targets (9). The currently most effective treatment for PTSD (cognitive behavioral training (CBT)) (102) was conceived entirely on psychological grounds. Similarly, trials of the most effective drugs for PTSD, selective serotonin reuptake inhibitors (SSRIs) (103), were based on these drugs' observed antidepressant effect; the recognition that the serotoninergic system is involved in the biology of PTSD only came afterward. Despite the abundance of biological insights into PTSD that have been achieved, the development of treatments for PTSD has not been different from the history of much of medicine: effective agents are discovered by serendipity, and their biological mechanisms of action are only clarified later. As social robots could potentially hold out the prospect of providing a nuanced and novel solution to some of the challenges that PTSD diagnosis and treatment are facing, future research should be appropriately conducted to test this premise.
Data Availability Statement
The original contributions presented in the study are included in the article/supplementary material, further inquiries can be directed to the corresponding author.
Author Contributions
GL and ZB-Z conceptualized the paper and wrote the first draft. EC supervised and revised. All authors edited and approved the final version.
Funding
The authors gratefully acknowledge funding from the European Union's Horizon 2020 Research and Innovation Programme under the Marie Sklodowska-Curie to ENTWINE, the European Training Network on Informal Care (Grant agreement no. 814072), the European Research Council (ERC) under the European Union's Horizon 2020 Research and Innovation Programme (Grant agreement no. 677270 to EC), and the Leverhulme Trust (PLP-2018-152 to EC).
Conflict of Interest
The authors declare that the research was conducted in the absence of any commercial or financial relationships that could be construed as a potential conflict of interest.
Publisher's Note
All claims expressed in this article are solely those of the authors and do not necessarily represent those of their affiliated organizations, or those of the publisher, the editors and the reviewers. Any product that may be evaluated in this article, or claim that may be made by its manufacturer, is not guaranteed or endorsed by the publisher.
References
1. Chrousos GP. Stress and disorders of the stress system. Nat Rev Endocrinol. (2009) 5:374–81. doi: 10.1038/nrendo.2009.106
2. McEwen BS. The brain on stress: toward an integrative approach to brain, body, and behavior. Perspect Psychol Sci. (2013) 8:673–5. doi: 10.1177/1745691613506907
3. de Kloet ER, Joëls M, Holsboer F. Stress and the brain: from adaptation to disease. Nat Rev Neurosci. (2005) 6:463–75. doi: 10.1038/nrn1683
4. Kazlauskas E. Challenges for providing health care in traumatized populations: barriers for PTSD treatments and the need for new developments. Glob Health Action. (2017) 10:1322399. doi: 10.1080/16549716.2017.1322399
5. Yehuda R, Hoge CW, McFarlane AC, Vermetten E, Lanius RA, Nievergelt CM, et al. Post-traumatic stress disorder. Nat Rev Dis Primers. (2015) 1:15057. doi: 10.1038/nrdp.2015.57
6. Kessler RC, Sonnega A, Bromet E, Hughes M, Nelson CB. Posttraumatic stress disorder in the National Comorbidity Survey. Arch Gen Psychiatry. (1995) 52:1048–60. doi: 10.1001/archpsyc.1995.03950240066012
7. Kessler RC. Posttraumatic stress disorder: the burden to the individual and to society. J Clin Psychiatry. (2000) 61(Suppl. 5):4. doi: 10.4088/JCP.v61n0713e
8. Kalisch R, Baker DG, Basten U, Boks MP, Bonanno GA, Brummelman E, et al. The resilience framework as a strategy to combat stress-related disorders. Nat Hum Behav. (2017) 1:784–90. doi: 10.1038/s41562-017-0200-8
9. Pitman RK, Rasmusson AM, Koenen KC, Shin LM, Orr SP, Gilbertson MW, et al. Biological studies of post-traumatic stress disorder. Nat Rev Neurosci. (2012) 13:769–87. doi: 10.1038/nrn3339
10. Hirschtritt ME, Insel TR. Digital technologies in psychiatry: present and future. Focus. (2018) 16:251. doi: 10.1176/appi.focus.20180001
11. Doherty K, Balaskas A, Doherty G. The design of ecological momentary assessment technologies. Interact Comput. (2020) 32:257–278. doi: 10.1093/iwcomp/iwaa019
12. Mohr DC, Burns MN, Schueller SM, Clarke G, Klinkman M. Behavioral Intervention Technologies: Evidence review and recommendations for future research in mental health. Gen Hosp Psychiatry. (2013) 35:332–8. doi: 10.1016/j.genhosppsych.2013.03.008
13. Blanchard EB, Jones-Alexander J, Buckley TC, Forneris CA. Psychometric properties of the PTSD Checklist (PCL). Behav Res Ther. (1996) 34:669–73. doi: 10.1016/0005-7967(96)00033-2
14. Weathers FW, Bovin MJ, Lee DJ, Sloan DM, Schnurr PP, Kaloupek DG, et al. The Clinician-Administered PTSD Scale for DSM-5 (CAPS-5): development and initial psychometric evaluation in military veterans. Psychol Assess. (2018) 30:383–95. doi: 10.1037/pas0000486
15. Lorenz P, Schindler L, Steudte-Schmiedgen S, Weidner K, Kirschbaum C, Schellong J. Ecological momentary assessment in posttraumatic stress disorder and coping. An eHealth study protocol. Eur J Psychotraumatol. (2019) 10:1654064. doi: 10.1080/20008198.2019.1654064
16. McDevitt-Murphy ME, Luciano MT, Zakarian RJ. Use of ecological momentary assessment and intervention in treatment with adults. Focus. (2018) 16:370–375. doi: 10.1176/appi.focus.20180017
17. Balaskas A, Schueller SM, Cox AL, Doherty G. Ecological momentary interventions for mental health: a scoping review. PLoS ONE. (2021) 16:e0248152. doi: 10.1371/journal.pone.0248152
18. Schueller SM, Aguilera A, Mohr DC. Ecological momentary interventions for depression and anxiety. Depress Anxiety. (2017) 34:540–545. doi: 10.1002/da.22649
19. Utami D, Bickmore T, Nikolopoulou A, Paasche-Orlow M. Talk about death: end of life planning with a virtual agent. Lecture Notes Comput Sci. (2017) 10498:441–50. doi: 10.1007/978-3-319-67401-8_55
20. Lucas GM, Rizzo A, Gratch J, Scherer S, Stratou G, Boberg J, et al. Reporting mental health symptoms: breaking down barriers to care with virtual human interviewers. Front Rob AI. (2017) 4:51. doi: 10.3389/frobt.2017.00051
21. Rizzo A, Lange B, Buckwalter JG, Forbell E, Kim J, Sagae K, et al. SimCoach: an intelligent virtual human system for providing healthcare information and support. Int J Disabil Human Dev. (2011) 10:277–81. doi: 10.1515/IJDHD.2011.046
22. Scherer S, Lucas GM, Gratch J, Skip Rizzo A, Morency L. Self-Reported symptoms of depression and PTSD are associated with reduced vowel space in screening interviews. IEEE Trans Affect Comput. (2016) 7:59–73. doi: 10.1109/TAFFC.2015.2440264
23. Rizzo AS, Shilling R. Clinical virtual reality tools to advance the prevention, assessment, and treatment of PTSD. Eur J Psychotraumatol. (2017) 8(Suppl. 5): 1414560. doi: 10.1080/20008198.2017.1414560
24. Kothgassner OD, Goreis A, Kafka JX, Van Eickels RL, Plener PL, Felnhofer A. Virtual reality exposure therapy for posttraumatic stress disorder (PTSD): a meta-analysis. Eur J Psychotraumatol. (2019) 10:1654782. doi: 10.1080/20008198.2019.1654782
25. Breazeal C. Toward sociable robots. Rob Auton Syst. (2003) 42:167–75. doi: 10.1016/S0921-8890(02)00373-1
26. Henschel A, Laban G, Cross ES. What makes a robot social? A review of social robots from science fiction to a home or hospital near you. Curr Rob Rep. (2021) 2:9–19. doi: 10.1007/s43154-020-00035-0
27. Cifuentes CA, Pinto MJ, Céspedes N, Múnera M. Social robots in therapy and care. Curr Rob Rep. (2020) 1:59–74. doi: 10.1007/s43154-020-00009-2
28. Robinson NL, Cottier TV, Kavanagh DJ. Psychosocial health interventions by social robots: systematic review of randomized controlled trials. J Med Internet Res. (2019) 21:1–20. doi: 10.2196/13203
29. Scoglio AAJ, Reilly ED, Gorman JA, Drebing CE. Use of social robots in mental health and well-being research: systematic review. J Med Internet Res. (2019) 21:e13322. doi: 10.2196/13322
30. Mohebbi A. Human-robot interaction in rehabilitation and assistance: a review. Curr Rob Rep. (2020) 1:131–44. doi: 10.1007/s43154-020-00015-4
31. Feingold Polak R, Tzedek SL. Social robot for rehabilitation: expert clinicians and post-stroke patients' evaluation following a long-term intervention. In: Proceedings of the 2020 ACM/IEEE International Conference on Human-Robot Interaction. HRI '20. New York, NY, USA: Association for Computing Machinery (2020). p. 151–60.
32. Cross ES, Ramsey R, Liepelt R, Prinz W, de C Hamilton F A. The shaping of social perception by stimulus and knowledge cues to human animacy. Philos Trans R Soc Lond B, Biol Sci. (2016) 371:20150075. doi: 10.1098/rstb.2015.0075
33. Cross ES, Hortensius R, Wykowska A. From social brains to social robots: applying neurocognitive insights to human-robot interaction. Philos Trans R Soc B. (2019) 374:20180024. doi: 10.1098/rstb.2018.0024
34. Laban G, George JN, Morrison V, Cross ES. Tell me more! Assessing interactions with social robots from speech. Paladyn J Behav Rob. (2021) 12:136–59. doi: 10.1515/pjbr-2021-0011
35. Hortensius R, Hekele F, Cross ES. The perception of emotion in artificial agents. IEEE Trans Cogn Dev Syst. (2018) 10:852–64. doi: 10.1109/TCDS.2018.2826921
36. Ben-Zion Z, Fine NB, Keynan NJ, Admon R, Halpern P, Liberzon I, et al. Neurobehavioral moderators of post-traumatic stress disorder (PTSD) trajectories: study protocol of a prospective MRI study of recent trauma survivors. Eur J Psychotraumatol. (2019) 10:1683941. doi: 10.1080/20008198.2019.1683941
37. Ben-Zion Z, Artzi M, Niry D, Keynan NJ, Zeevi Y, Admon R, et al. Neuroanatomical risk factors for posttraumatic stress disorder in recent trauma survivors. Biol Psychiatry. (2020) 5:311–9. doi: 10.1016/j.bpsc.2019.11.003
38. Bauer MR, Ruef AM, Pineles SL, Japuntich SJ, Macklin ML, Lasko NB, et al. Psychophysiological assessment of PTSD: a potential research domain criteria construct. Psychol Assess. (2013) 25:1037–43. doi: 10.1037/a0033432
39. Hirshberg A, Holcomb JB, Mattox KL. Hospital trauma care in multiple-casualty incidents: a critical view. Ann Emerg Med. (2001) 37:647–52. doi: 10.1067/mem.2001.115650
40. Georgiou A, Lockey DJ. The performance and assessment of hospital trauma teams. Scand J Trauma Resusc Emerg Med. (2010) 18:66. doi: 10.1186/1757-7241-18-66
41. Berger W, Mendlowicz MV, Marques-Portella C, Kinrys G, Fontenelle LF, Marmar CR, et al. Pharmacologic alternatives to antidepressants in posttraumatic stress disorder: a systematic review. Progress Neuropsychopharmacol Biol Psychiatry. (2009) 33:169–80. doi: 10.1016/j.pnpbp.2008.12.004
42. Cusack K, Jonas DE, Forneris CA, Wines C, Sonis J, Middleton JC, et al. Psychological treatments for adults with posttraumatic stress disorder: a systematic review and meta-analysis. Clin Psychol Rev. (2016) 43:128–41. doi: 10.1016/j.cpr.2015.10.003
43. Qi W, Gevonden M, Shalev A. Prevention of post-traumatic stress disorder after trauma: current evidence and future directions. Curr Psychiatry Rep. (2016) 18:20. doi: 10.1007/s11920-015-0655-0
44. Brewin CR, Andrews B, Valentine JD. Meta-analysis of risk factors for posttraumatic stress disorder in trauma-exposed adults. J Consult Clin Psychol. (2000) 68:748–766. doi: 10.1037/0022-006X.68.5.748
45. Hepp U, Moergeli H, Buchi S, Bruchhaus-Steinert H, Kraemer B, Sensky T, et al. Post-traumatic stress disorder in serious accidental injury: 3-year follow-up study. Br J Psychiatry. (2008) 192:376–83. doi: 10.1192/bjp.bp.106.030569
46. Ozer EJ, Best SR, Lipsey TL, Weiss DS. Predictors of posttraumatic stress disorder and symptoms in adults: a meta-analysis. Psychol Bull. (2003) 129:52–73. doi: 10.1037/0033-2909.129.1.52
47. Perkonigg A, Pfister H, Stein MB, Höfler M, Lieb R, Maercker A, et al. Longitudinal course of posttraumatic stress disorder and posttraumatic stress disorder symptoms in a community sample of adolescents and young adults. Am J Psychiatry. (2005) 162:1320–7. doi: 10.1176/appi.ajp.162.7.1320
48. Peleg T, Shalev AY. Longitudinal studies of PTSD: overview of findings and methods. CNS Spectr. (2006) 11:589–602. doi: 10.1017/S109285290001364X
49. Heapy AA, Higgins DM, Cervone D, Wandner L, Fenton BT, Kerns RD. A systematic review of technology-assisted self-management interventions for chronic pain: looking across treatment modalities. Clin J Pain. (2015) 31:470–92. doi: 10.1097/AJP.0000000000000185
50. Kampmann IL, Emmelkamp PMG, Morina N. Meta-analysis of technology-assisted interventions for social anxiety disorder. J Anxiety Disord. (2016) 42:71–84. doi: 10.1016/j.janxdis.2016.06.007
51. Newman MG, Szkodny LE, Llera SJ, Przeworski A. A review of technology-assisted self-help and minimal contact therapies for anxiety and depression: is human contact necessary for therapeutic efficacy? Clin Psychol Rev. (2011) 31:89–103. doi: 10.1016/j.cpr.2010.09.008
52. Ben-Zion Z, Zeevi Y, Keynan NJ, Admon R, Kozlovski T, Sharon H, et al. Multi-domain potential biomarkers for post-traumatic stress disorder (PTSD) severity in recent trauma survivors. Transl Psychiatry. (2020) 10:208. doi: 10.1038/s41398-020-00898-z
53. Moukarzel A, Michelet P, Durand AC, Sebbane M, Bourgeois S, Markarian T, et al. Burnout syndrome among emergency department staff: prevalence and associated factors. Biomed Res Int. (2019) 2019:6462472. doi: 10.1155/2019/6462472
54. Pape-Köhler CIA, Simanski C, Nienaber U, Lefering R. External factors and the incidence of severe trauma: time, date, season and moon. Injury. (2014) 45:S93–S99. doi: 10.1016/j.injury.2014.08.027
55. Love J, Zatzick D. Screening and intervention for comorbid substance disorders, PTSD, depression, and suicide: a trauma center survey. Psychiatr Serv. (2014) 65:918–923. doi: 10.1176/appi.ps.201300399
56. Broadbent E. Interactions with robots: the truths we reveal about ourselves. Annu Rev Psychol. (2017) 68:627–52. doi: 10.1146/annurev-psych-010416-043958
57. Laban G, Kappas A, Morrison V, Cross ES. Protocol for a mediated long-term experiment with a social robot. PsyArXiv. (2021). doi: 10.31234/osf.io/4z3aw
58. Boumans R, van Meulen F, Hindriks K, Neerincx M, Olde Rikkert MGM. Robot for health data acquisition among older adults: a pilot randomised controlled cross-over trial. BMJ Q Safety. (2019) 28:793–9. doi: 10.1136/bmjqs-2018-008977
59. van der Putte D, Boumans R, Neerincx M, Rikkert MO, de Mul M. A social robot for autonomous health data acquisition among hospitalized patients: an exploratory field study. In: 2019 14th ACM/IEEE International Conference on Human-Robot Interaction (HRI). Daegu: IEEE (2019). p. 658–9.
60. Vitanza A, D'Onofrio G, Ricciardi F, Sancarlo D, Greco A, Giuliani F. Assistive robots for the elderly: innovative tools to gather health relevant data. In: Consoli S, Reforgiato Recupero D, Petković M, editors. Data Science for Healthcare: Methodologies and Applications. Cham: Springer International Publishing (2019). p. 195–215.
61. Robinson NL, Connolly J, Hides L, Kavanagh DJ. Social robots as treatment agents: pilot randomized controlled trial to deliver a behavior change intervention. Internet Intervent. (2020) 21:100320. doi: 10.1016/j.invent.2020.100320
62. Shiomi M, Nakata A, Kanbara M, Hagita N. Robot reciprocation of hugs increases both interacting times and self-disclosures. Int J Soc Rob. (2020) 13:353–61. doi: 10.1007/s12369-020-00644-x
63. Ling H, Björling E. Sharing stress with a robot: what would a robot say? Human Mach Commun. (2020) 1:133–58. doi: 10.30658/hmc.1.8
64. Aroyo AM, Rea F, Sandini G, Sciutti A. Trust and social engineering in human robot interaction: will a robot make you disclose sensitive information, conform to its recommendations or gamble? IEEE Rob Autom Lett. (2018) 3:3701–8. doi: 10.1109/LRA.2018.2856272
65. Birnbaum GE, Mizrahi M, Hoffman G, Reis HT, Finkel EJ, Sass O. Machines as a source of consolation: robot responsiveness increases human approach behavior and desire for companionship. In: 2016 11th ACM/IEEE International Conference on Human-Robot Interaction (HRI). Christchurch: IEEE (2016). p. 165–72.
66. De Groot JJ, Barakova E, Lourens T, van Wingerden E, Sterkenburg P. Game-Based Human-Robot Interaction Promotes Self-disclosure in People with Visual Impairments and Intellectual Disabilities BT - Understanding the Brain Function and Emotions. Cham: Springer International Publishing (2019). p. 262–72.
67. Blixen CE, Kanuch S, Perzynski AT, Thomas C, Dawson NV, Sajatovic M. Barriers to self-management of serious mental illness and diabetes. Am J Health Behav. (2016) 40:194–204. doi: 10.5993/AJHB.40.2.4
68. Merwin E, Hinton I, Dembling B, Stern S. Shortages of rural mental health professionals. Arch Psychiatr Nurs. (2003) 17:42–51. doi: 10.1053/apnu.2003.1
70. Quartana PJ, Wilk JE, Thomas JL, Bray RM, Rae Olmsted KL, Brown JM, et al. Trends in mental health services utilization and stigma in US soldiers from 2002 to 2011. Am J Public Health. (2014) 104:1671–9. doi: 10.2105/AJPH.2014.301971
71. Naifeh JA, Colpe LJ, Aliaga PA, Sampson NA, Heeringa SG, Stein MB, et al. Barriers to initiating and continuing mental health treatment among soldiers in the army study to assess risk and resilience in servicemembers (Army STARRS). Mil Med. (2016) 181:1021–32. doi: 10.7205/MILMED-D-15-00211
72. Hines LA, Goodwin L, Jones M, Hull L, Wessely S, Fear NT, et al. Factors affecting help seeking for mental health problems after deployment to Iraq and Afghanistan. Psychiatr Serv (Washington, DC). (2014) 65:98–105. doi: 10.1176/appi.ps.004972012
73. Kantor V, Knefel M, Lueger-Schuster B. Perceived barriers and facilitators of mental health service utilization in adult trauma survivors: a systematic review. Clin Psychol Rev. (2017) 52:52–68. doi: 10.1016/j.cpr.2016.12.001
74. Shalev AY, Freedman S. PTSD following terrorist attacks: a prospective evaluation. Am J Psychiatry. (2005) 162:1188–91. doi: 10.1176/appi.ajp.162.6.1188
75. Deutsch I, Erel H, Paz M, Hoffman G, Zuckerman O. Home robotic devices for older adults: opportunities and concerns. Comput Human Behav. (2019) 98:122–33. doi: 10.1016/j.chb.2019.04.002
76. Wiederhold BK. Can robots help us manage the caregiving crisis? Cyberpsychol Behav Soc Network. (2018) 21:533–4. doi: 10.1089/cyber.2018.29123.bkw
77. Hilty DM, Randhawa K, Maheu MM, McKean AJS, Pantera R, Mishkind MC, et al. A review of telepresence, virtual reality, and augmented reality applied to clinical care. J Technol Behav Sci. (2020) 5:178–205. doi: 10.1007/s41347-020-00126-x
78. Pavaloiu IB, Vasilateanu A, Popa R, Scurtu D, Hang A, Goga N. Healthcare robotic telepresence. In: Proceedings of the 13th International Conference on Electronics, Computers and Artificial Intelligence, ECAI 2021. Pitesti: IEEE (2021).
79. Lou S. Feature Update: Telepresence Enabled by Pepper | SoftBank Robotics; (2020). Available online at: https://www.softbankrobotics.com/emea/en/blog/news-trends/feature-update-telepresence-enabled-pepper
80. SoftBank Robotics Labs. Pepper Telepresence Toolkit: A toolkit to build telepresence or teleoperation applications for Pepper QiSDK: talk through Pepper, drive Pepper around, etc. (2020). Available online at: https://github.com/softbankrobotics-labs/peppertelepresence-toolkit
81. Fletcher KE, Steinbach S, Lewis F, Hendricks M, Kwan B. Hospitalized medical patients with posttraumatic stress disorder (PTSD): review of the literature and a roadmap for improved care. J Hosp Med. (2020) 19:2020. doi: 10.12788/jhm.3409
82. Blais RK, Renshaw KD, Jakupcak M. Posttraumatic stress and stigma in active-duty service members relate to lower likelihood of seeking support. J Trauma Stress. (2014) 27:116–119. doi: 10.1002/jts.21888
83. Wilson JP, Droždek B, Turkovic S. Posttraumatic shame and guilt. Trauma Violence Abuse. (2006) 7:122–41. doi: 10.1177/1524838005285914
84. Saraiya T, Lopez-Castro T. Ashamed and afraid: a scoping review of the role of shame in post-traumatic stress disorder (PTSD). J Clin Med. (2016) 5:94. doi: 10.3390/jcm5110094
85. Cunningham KC, LoSavio ST, Dennis PA, Farmer C, Clancy CP, Hertzberg MA, et al. Shame as a mediator between posttraumatic stress disorder symptoms and suicidal ideation among veterans. J Affect Disord. (2019) 243:216–9. doi: 10.1016/j.jad.2018.09.040
86. Cândea DM, Szentagotai-Tăta A. Shame-proneness, guilt-proneness and anxiety symptoms: a meta-analysis. J Anxiety Disord. (2018) 58:78–106. doi: 10.1016/j.janxdis.2018.07.005
87. Starzynski LL, Ullman SE, Filipas HH, Townsend SM. Correlates of women's sexual assault disclosure to informal and formal support sources. Violence Vict. (2005) 20:417–32. doi: 10.1891/vivi.2005.20.4.417
88. Birnbaum GE, Mizrahi M, Hoffman G, Reis HT, Finkel EJ, Sass O. What robots can teach us about intimacy: the reassuring effects of robot responsiveness to human disclosure. Comput Human Behav. (2016) 63:416–23. doi: 10.1016/j.chb.2016.05.064
89. Björling EA, Rose E, Davidson A, Ren R, Wong D. Can we keep him forever? Teen's engagement and desire for emotional connection with a social robot. Int J Soc Rob. (2019) 12:65–77. doi: 10.1007/s12369-019-00539-6
90. Hoffman G, Birnbaum GE, Vanunu K, Sass O, Reis HT. Robot responsiveness to human disclosure affects social impression and appeal. In: Proceedings of the 2014 ACM/IEEE International Conference on Human-Robot Interaction. HRI â '14. New York, NY: Association for Computing Machinery (2014). p. 1–8.
91. Ghazali AS, Ham J, Barakova E, Markopoulos P. Assessing the effect of persuasive robots interactive social cues on users' psychological reactance, liking, trusting beliefs and compliance. Adv Rob. (2019) 33:325–37. doi: 10.1080/01691864.2019.1589570
92. Utami D, Bickmore TW, Kruger LJ. A robotic couples counselor for promoting positive communication. In: RO-MAN 2017-26th IEEE International Symposium on Robot and Human Interactive Communication. Lisbon: IEEE (2017). p. 248–55. doi: 10.1109/ROMAN.2017.8172310
93. Sandini G, Mohan V, Sciutti A, Morasso P. Social cognition for human-robot symbiosis - challenges and building blocks. Front Neurorobot. (2018) 12:34. doi: 10.3389/fnbot.2018.00034
94. Wullenkord R, Eyssel F. Societal and ethical issues in HRI. Curr Rob Rep. (2020) 1:85–96. doi: 10.1007/s43154-020-00010-9
95. Cross ES, Ramsey R. Mind meets machine: towards a cognitive science of human–machine interactions. Trends Cogn Sci. (2021) 25:200–12. doi: 10.1016/j.tics.2020.11.009
96. Hortensius R, Cross ES. From automata to animate beings: the scope and limits of attributing socialness to artificial agents. Ann N Y Acad Sci. (2018) 1426:93–110. doi: 10.1111/nyas.13727
97. Burke LE, Shiffman S, Music E, Styn MA, Kriska A, Smailagic A, et al. Ecological momentary assessment in behavioral research: addressing technological and human participant challenges. J Med Internet Res. (2017) 19:e77. doi: 10.2196/jmir.7138
98. Chandler KD, Hodge CJ, McElvaine K, Olschewski EJ, Melton KK, Deboeck P. Challenges of ecological momentary assessments to study family leisure: Participants' perspectives 2021. J Leisure Res. (2021) 1:1–7. doi: 10.1080/00222216.2021.2001398
99. Dao KP, De Cocker K, Tong HL, Kocaballi AB, Chow C, Laranjo L. Smartphone-Delivered ecological momentary interventions based on ecological momentary assessments to promote health behaviors: systematic review and adapted checklist for reporting ecological momentary assessment and intervention studies. JMIR Mhealth Uhealth. (2021) 9:e22890. doi: 10.2196/22890
100. Mubin O, Ahmad MI, Kaur S, Shi W, Khan A. Social robots in public spaces: a meta-review. In: Ge SS, Cabibihan JJ, Salichs MA, Broadbent E, He H, Wagner AR, et al., editors. Social Robotics. Cham: Springer International Publishing (2018). p. 213–20.
101. Nomura T, Kanda T, Suzuki T, Yamada S. Do people with social anxiety feel anxious about interacting with a robot? AI Soc. (2020) 35:381–90. doi: 10.1007/s00146-019-00889-9
102. Smith P, Yule W, Perrin S, Tranah T, Dalgleish T, Clark DM. Cognitive-behavioral therapy for PTSD in children and adolescents: a preliminary randomized controlled trial. J Am Acad Child Adolesc Psychiatry. (2007) 46:1051–1061. doi: 10.1097/CHI.0b013e318067e288
Keywords: post-traumatic stress disorder, social robots, trauma, mental health, human-robot interaction, affective computing, affective science, emotion
Citation: Laban G, Ben-Zion Z and Cross ES (2022) Social Robots for Supporting Post-traumatic Stress Disorder Diagnosis and Treatment. Front. Psychiatry 12:752874. doi: 10.3389/fpsyt.2021.752874
Received: 03 August 2021; Accepted: 27 December 2021;
Published: 04 February 2022.
Edited by:
Hanna Karakula-Juchnowicz, Medical University of Lublin, PolandReviewed by:
Paweł Krukow, Medical University of Lublin, PolandJohanna Seibt, Aarhus University, Denmark
Copyright © 2022 Laban, Ben-Zion and Cross. This is an open-access article distributed under the terms of the Creative Commons Attribution License (CC BY). The use, distribution or reproduction in other forums is permitted, provided the original author(s) and the copyright owner(s) are credited and that the original publication in this journal is cited, in accordance with accepted academic practice. No use, distribution or reproduction is permitted which does not comply with these terms.
*Correspondence: Emily S. Cross, emily.cross@mq.edu.au
†These authors have contributed equally to this work and share first authorship