- 1Department of Pediatrics, First Affiliated Hospital, Zhengzhou University, Zhengzhou, China
- 2Department of Child Healthcare, Children's Hospital Affiliated to Zhengzhou University, Zhengzhou, China
Purpose: This study aimed to explore alterations in functional connectivity (FC) within and between default mode network (DMN), central executive network, and salience network in autism spectrum disorder (ASD) with co-occurring attention deficit hyperactivity disorder (ADHD).
Method: A total of 135 individuals' date of the Autism Brain Imaging Data Exchange II was used to compare the ASD+ADHD group with the ASD group in relation to the abnormal within-network and between-network connectivity of the ASD group relative to the TD group; consequently, the correlation analysis between abnormal FC and behavior was performed.
Results: The ASD+ADHD group exhibited decreased within-network connectivity in the precuneus of the ventral DMN compared with the ASD group. Among the three groups, the ASD+ADHD group showed lower connectivity, whereas the ASD group had higher connectivity than the TD group, although the effect of the separate post hoc test was not significant. Meanwhile, the ASD+ADHD group showed increased between-network connectivity between the ventral DMN and dorsal DMN and between the ventral DMN and left executive control network, compared with the ASD and TD groups.
Conclusion: Dysfunction of DMN in the “triple-network model” is the core evidence for ASD with co-occurring ADHD.
Introduction
Autism spectrum disorder (ASD) and attention deficit hyperactivity disorder (ADHD) are defined by symptom-based classification. As described in the Diagnostic and Statistical Manual of Mental Disorders (DSM-5), ASD exhibits abnormal behavioral symptoms of social/communication deficits and restricted and repetitive behaviors, whereas ADHD is defined by attentional and/or hyperactive/impulsive traits (1). The new edition of the DSM-5 allows the diagnosis of comorbid ASD and ADHD in clinical practice (2). Both ASD and ADHD display few clear links between diagnostic criteria and specific neurobiological alterations (3, 4). There are few publications describing consistent phenotypic variations in people with ASD and co-occurring ADHD.
In recent years, the development of functional magnetic resonance imaging (fMRI), a common neuroimaging technique, has provided a promising tool for investigating cognitive dysfunction. Several resting-state fMRI (rs-fMRI) studies have demonstrated that ASD or ADHD is related to atypical patterns of functional connectivity (FC) in large-scale brain networks (5–9). Of the many stable intrinsic brain networks, Menon proposed a triple-network model, which consists of three core neurocognitive networks: the default mode network (DMN), central executive network (CEN), and salience network (SN) as the three most important intrinsic networks for human brain activation. The DMN, considered a task-negative network, includes a collection of brain regions that deactivate reliably during cognitive task performance; its nodes are the medial prefrontal cortex and posterior cingulate cortex (PCC) (10). The CEN, often characterized as a task-positive network, encompasses a frontoparietal system anchored in the dorsolateral prefrontal cortex and posterior parietal cortex, which shows strong co-activation during the performance of cognitively demanding tasks (11). Traditionally, the SN, with key nodes in the dorsal anterior cingulate cortex and frontoinsular cortex, is thought to be involved in the processes of information filtering, detection, and integration (12). The triple-network approach examines “core” brain networks supporting cognitive, perceptual, affective, and social functions and was thought to be abnormally organized in many psychiatric and neurological disorders (13). In recent years, separate research on the human brain functional networks of people with ADHD (14) or ASD (15), the triple-network model has been widely used, and some common brain connectivity issues have been discovered. Specifically, the dysfunction of the DMN plays an important role in the social impairments of people with ASD (16), and the abnormal state switching and cognitive control of people with ADHD are related to the aberrant within-connectivity of the DMN, SN, CEN, and attention networks (6, 17). In addition, ASD and ADHD share the common brain network dysfunction characterized by different coupling patterns of the temporoparietal cortices in the DMN with SN and the dorsal attention network (18). However, the number of current studies related to ASD with co-occurring ADHD—with regard to the FC of the triple-network model—is scarce.
In this study, we applied the triple-network model to investigate the specific alterations of both within-network and between-network FC of the three core neurocognitive networks in the ASD with co-occurring ADHD (ASD+ADHD group) and ASD without co-occurring ADHD (ASD group) using rs-fMRI while identifying the relationship to clinical symptoms. We used independent component analysis (ICA) to identify the brain regions of the DMN, SN, and CEN according to the Stanford Functional Imaging in Neuropsychiatric Disorders (FIND) Lab. We hypothesized that altered connectivity existed both within and between these three core brain networks and predicted that specific FCs would explain the brain-behavior relationship that contributed to explaining the phenotypes of the ASD with ADHD co-occurring, thus demonstrating the diagnosis and potential therapeutic biomarkers of such people.
Materials and Methods
Subjects
In our analyses, we included data from four sites of an open-access multi-site image database [Autism Brain Imaging Data Exchange II (ABIDE II), http://fcon_1000.projects.nitrc.org/indi/abide/abide_II.html] with most subjects meeting the following criteria: first, we included individuals with a full-scale IQ and near full-brain coverage structural and rs-fMRI scan data and excluded individuals with excessive head motion characterized by a mean framewise displacement (FD) of more than 0.30 mm; next, we divided the people into two groups (ASD+ADHD and ASD) on the basis of the psychiatric comorbidity information on the ABIDE II website; and finally, from each site, we selected from the included dataset the same number of individuals matching in terms of age- and IQ-matched typical development (TD) with the other two groups. These inclusion criteria and an additional step for matching the three groups at each site resulted in a cohort of 135 individuals (45 ASD+ADHD, 45 ASD, and 45 TD), with whom the three groups were matched by age, sex, and full IQ (FIQ) in the scanner (see Table 1). A selection flowchart for the participants is provided in Supplementary Figure 1.
Image Acquisition and Preparation
The acquisition parameters and protocol information of the MRI images are provided in Supplementary Table 1. On these rs-fMRI data, we performed preprocessing using the Data Processing Assistant for Resting-State fMRI toolbox (DPARSF version 2.3), implemented in the MATLAB 2014a platform (19). The preprocessing steps included discarding the first five volumes to allow for magnetization equilibration and participant adaptation to the scanning environment, interleaved slice-timing correction, and head motion correction. Participants without excessive head motion, which was defined by a mean FD of <0.3 mm, were included in further analysis. The images were warped to the Montreal Neurological Institute space, then resampled to 3.0-mm isotropic voxels, and finally spatially smoothed with a 5-mm full width half maximum Gaussian kernel to improve the signal-to-noise ratio and reduce inter-subject variability.
ICA and Identification of Networks-of-Interest
Group ICA was performed on the overall group preprocessed data (135 subjects) using the InfoMax algorithm, as implemented in the Group ICA of fMRI Toolbox (GIFT) version 4.0 (http://www.icatb.sourceforge.net). The aggregate preprocessed data were written and projected into 29 independent components (ICs), the optimal number of their dimensions estimated by the minimum description length criteria tool within the GIFT software (20). Principal components analysis reduced the dimensions of the data (21), followed by an IC estimation that produced spatial maps and time courses (22). Following this, 100 ICA (ICASSO) ensured the stability of the decomposition (23). Next, GICA3 back-constructed a set of mean group components, resulting from the previous steps into a single subject space (24). Finally, to reflect the measures of within-network connectivity, we converted the intensity values of the spatial z-map of each subject to the z value (25).
After all these steps, 29 spatiotemporal components were obtained (Supplementary Figure 2). To select components reflecting each network of interest (the triple-network model), we statistically compared the spatial map of each IC with a group of covered six of 14 major networks consisting of 90 regions of interest (ROIs) [left executive control network (LECN), right executive control network (RECN), anterior_SN, post_SN, dorsal_DMN, and ventral_DMN] described by a previous study (26). We then calculated Pearson's correlation coefficients for each pairwise relationship with Sort Components in GIFT software and retained the ICs with the highest correlation coefficients as networks of interest for our triple-network model (26). This procedure identified nine ICs corresponding, respectively, to the LECN, RECN, anterior_SN, post_SN, dorsal_DMN, and ventral_DMN; the remaining ICs were eliminated from further analysis.
Within-Network and Between-Network Connectivity
The z-map of each subject-level component was indexed within-network connectivity measurements. Rs-fMRI data were band-pass–filtered (0.008–0.15 Hz) before the functional network connectivity (FNC) analysis (27), and the FNC software (http://mialab.mrn.org/sofware) was used to examine specific temporal correlations in a nonparametric pairwise manner, with a maximal lagged correlation approach, and calculated lags that were correlated to each other. Thus, all nine RSNs were paired with one another among the triple-networks to obtain 36 pairwise combinations, and the coefficients were then transformed into z-scores using Fisher's z-transformation. The transformed z-scores were indexed the between-network connectivity of each network pair.
Statistical Analysis
Between-group differences in demographic and clinical assessments were appropriately conducted with MANOVA (age, FIQ, ADOS_score, and SRS_score) or the chi-squared test (sex) using Statistical Product and Service Solutions software (version 25.0). The statistical module in DPARSF was used to perform statistical analysis on the spatial z-map (19). In each network, two-sample t-tests with age, site, sex, FIQ, and mean FD as covariates were used to analyze the group differences (ASD+ADHD and ASD) of within-network connectivity. The comparisons were limited to the network mask generated by composing the results of the one-sample t-test of each group [q < 0.01, false discovery rate (FDR) correction] (see Figure 2A). The significance criterion for between-group comparison was P < 0.05, correcting by threshold-free cluster enhancement (TFCE) (5,000 permutations) (28). We then used multivariate analysis of variance (MANOVA) to explore differences between FC values extracted from the above-detected clusters between the three groups with sex, age, FIQ, and mean FD as covariates.
To explore the differences in between-network connectivity, the resulting pairwise correlation coefficients were transformed to Fisher's z-values and then extracted into MANOVA while regressing out site, age, sex, FIQ, and mean FD. In addition, we further explored the differences in aberrant between-network connectivity between the ASD+ADHD and ASD groups using a post hoc test.
To examine whether there was a relationship between behavioral problems and abnormal within/between-network connections showing group differences, we used multiple regression analyses to estimate the associations after controlling for the site, age, sex, FIQ, and mean FD. The clinical characteristics of ASD were reflected by the z-scores of the Autism Diagnostic Observation Schedule, Second Edition (ADOS-2)_Social Affect (SA) subscale (29), the Social Responsiveness Scale (SRS), and the subscales (30). Our analysis was limited to the availability of ADOS_2_SA data for 95.6% of the ASD (n = 86) subjects (Table 1).
Results
Clinical Characteristics
As shown in Table 1, the age, sex and FIQ scores in the three groups were well matched. As expected, compared with the ASD and TD groups, the ASD+ADHD group had significantly higher SRS_total_T scores and higher subscale scores.
Identification of Network of Interests
In this study, the nine selected ICs were subsets of the large six major resting-state networks (RSNs) based on 90 ROIs relating to the triple-network model identified by a previous study (31): ICs 2, 12, and 25 were correlated to the dorsal DMN (dDMN); ICs 23 and 29 were correlated to the ventral DMN (vDMN); IC 24 was correlated to the LECN, IC 28 was correlated to the RECN; IC 20 was correlated to ther anterior SN; and IC 21 was correlated to the posterior SN (Figure 1A). We further compared the nine selected ICs in the current study with templates of the triple-network model (Figure 1B).
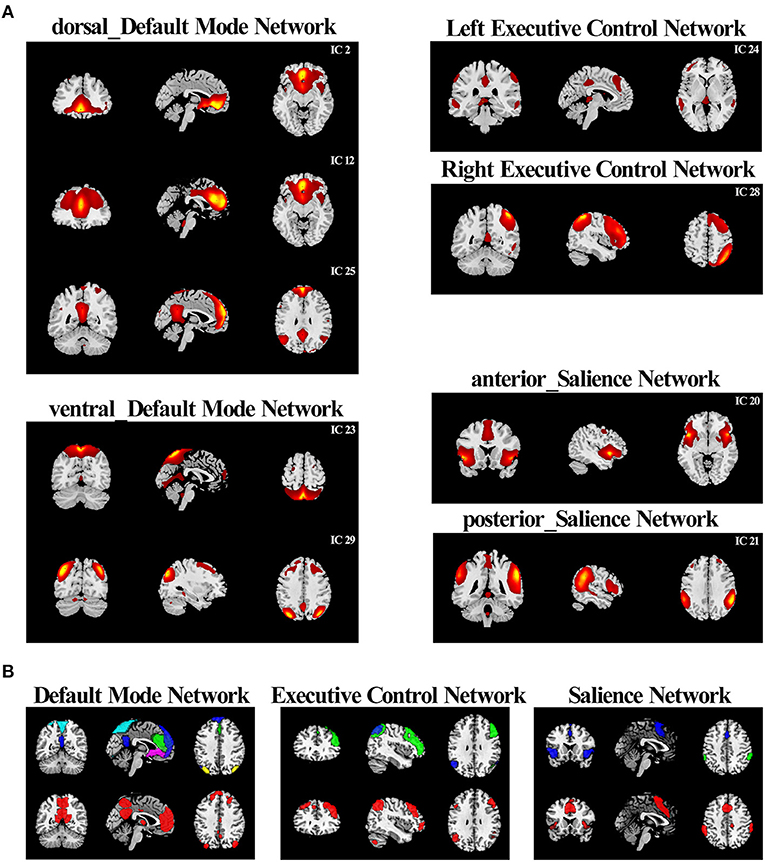
Figure 1. Comparison between the “triple network” of this study and the template networks (A) According to the correlation with the triple-network model network templates, the resting-state networks of the current study were divided into six categories. (B) The comparison between resting-state networks in current research and the triple-network model templates. RSN combinations of the same category in the current study were drawn in contrasting colors and displayed on the top. The corresponding reference template was drawn in red and displayed on the bottom.
Within-Network Dysfunction
Group comparisons of the network z-maps showed that, compared with the ASD group (TFCE-corrected), the ASD+ADHD group demonstrated significantly decreased connectivity in the left and inter-hemispheric precuneus of the IC 23, which was identified as the vDMN (Figure 2B, Table 2). There were no significant group differences in the within-network connectivity of the other ICs. Among the three groups, the ASD+ADHD group showed lower connectivity, whereas the ASD group had higher connectivity than the TD group. MANOVA revealed a significant main effect on the group, but the effect of the separate post hoc test was not significant (Figure 2B). Therefore, the difference in within-network connectivity observed between the ASD+ADHD group and ASD group is probably due to the sum of two contributions: (1) a decrease in connectivity in the ASD+ADHD group and (2) an increase in connectivity in the ASD group. This increases the delta within-network connectivity between the two groups.
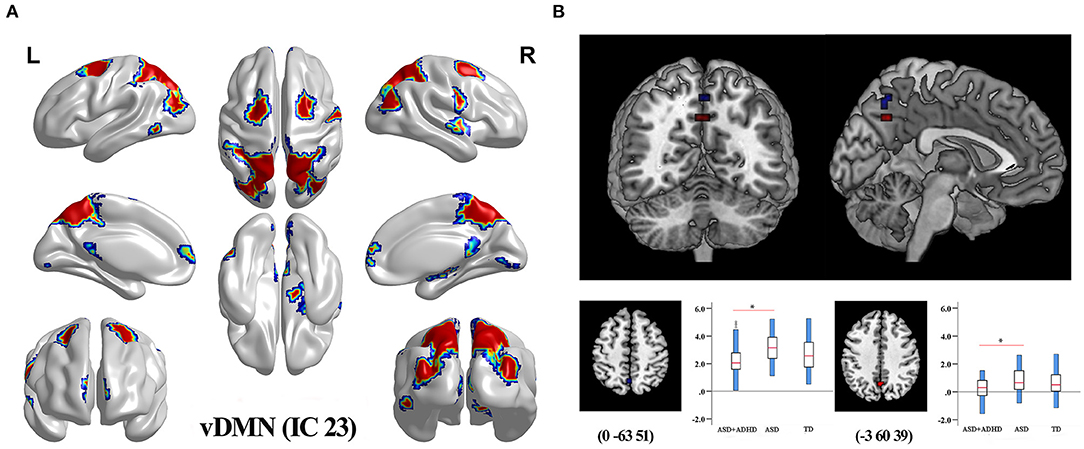
Figure 2. Differences in within-network connectivity in the triple-network model (A) The mask of IC 23 belonging to vDMN generated by composing the results of one-sample t-test of each group (q < 0.01, FDR correction). (B) ASD+ADHD group had lower FC in the left and inter-hemispheric precuneus of the vDMN compared with the ASD group.

Table 2. Brain regions showing significant within-network connectivity differences between ASD+ADHD and ASD groups.
Between-Network Dysfunction
The FNC analysis conducted on the between-network connectivity indicated significant differences between the four pairs of correlation coefficients among the three groups (P < 0.05; Figure 3B, Table 3). Of the four pairs with altered between-network connectivity, post hoc tests showed that ASD+ADHD group had significantly higher dDMN (IC 12)–vDMN (IC 23) and LECN (IC 24)–vDMN (IC 23) between-network connectivity than the ASD and TD groups, which indicated dysfunctional FC between the vDMN and dDMN and between the DMN and ECN; meanwhile, the ASD group had higher connectivity than the TD group, but the effect was not significant (Figure 3A, Table 3).
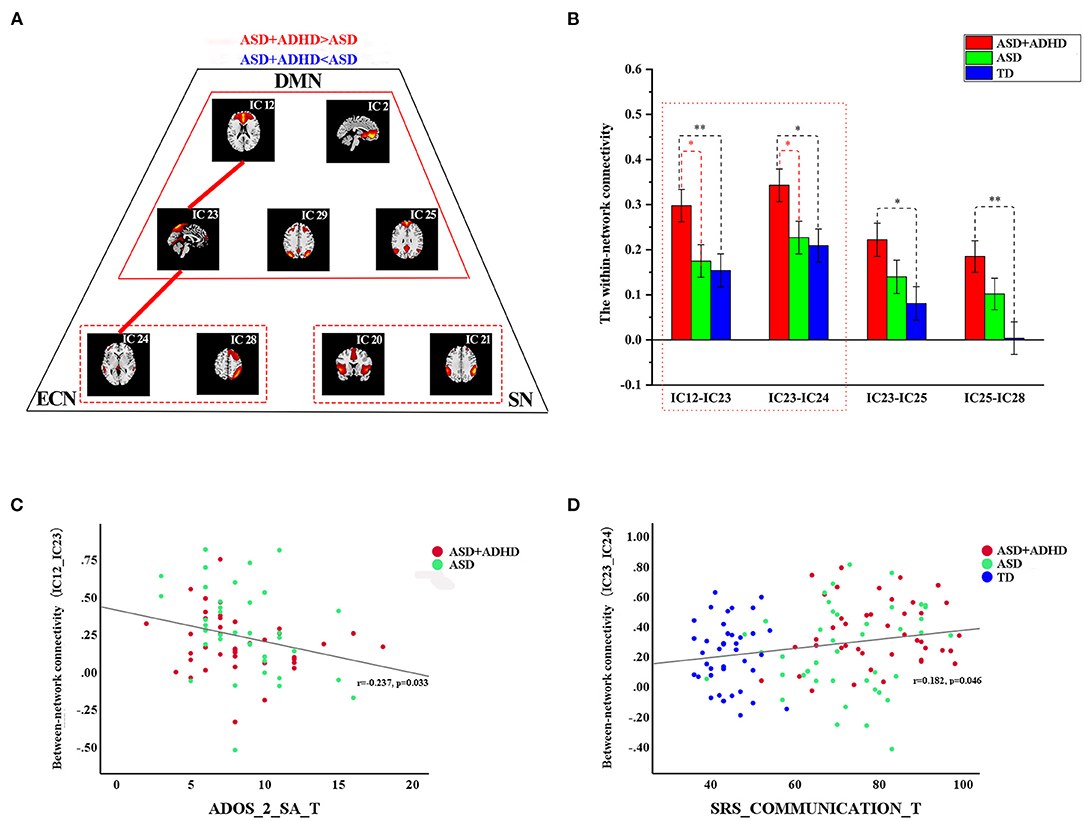
Figure 3. Differences in between-network connectivity and the correlations of clinical measures with abnormal connectivity (A) The red line indicates increased FNC strength in ASD+ADHD and the blue line displays the reverse situation. Further details are shown in Table 3. (B) Extracted altered between-network connectivity, ASD+ADHD group showed increased dDMN (IC 12)–vDMN (IC 23) connectivity and LECN (IC 24)–vDMN (IC 23) connectivity. (C) The altered dDMN (IC 12)–vDMN (IC 23) between-network connectivity by ADOS_2_SA scores. (D) The altered dDMN (IC 12)–vDMN (IC 23) between-network connectivity by SRS_communication scores.
Abnormalities in Network Connectivity Related to Symptoms Severity
As shown in Figures 3C,D, the altered dDMN (IC12)–vDMN (IC23) between-network connectivity was negatively correlated with ADOS_2_SA scores in all people with ASD (r = −0.237, p = 0.033, n = 77), and the altered vDMN (IC23)–LECN (IC24) between-network connectivity was positively correlated with SRS_communication T-scores in all samples (r = 0.182, p = 0.046, n = 126). However, the results were not corrected for multiple comparisons. There was no significant correlation between the abnormal within-network connectivity of the vDMN, and clinical characteristics measured by SRS and ADOS_2_SA scores were not detected.
Discussion
In this study, we demonstrated the dysconnectivity within and between the SN, DMN, and ECN in ASD with and without co-occurring ADHD, on the basis of the triple-network model. ASD+ADHD group showed decreased within-network connectivity in the left and inter-hemispheric precuneus of the vDMN compared with ASD group. Notably, ASD+ADHD group also demonstrated increased between-network connectivity between the dDMN and vDMN and between the vDMN and LCEN, which suggested the presence of atypical dynamic interactions of the triple network in the ASD with co-occurring ADHD. These results provide evidence of aberrant connectivity patterns across the core neurocognitive networks of triple-network models in ASD with co-occurring ADHD.
Social functioning is a major and central domain of impairment in both disorders, which significantly affects prognosis. Studies have reported that social awareness, social cognition, emotion recognition, and social communication may be poorer in children with ASD+ADHD than those with ASD alone (32). In this study, ASD+ADHD group showed more serious social deficits than those with ASD (Table 1). The networks of interest in the current study were informed by the triple-network hypothesis (13), according to which many psychiatric and neurological conditions were characterized by disorder-specific patterns of increased or reduced function and connectivity in the SN, DMN, and ECNs. The overall pattern observed in our study, showing an underconnectivity within the DMN accompanied by complex patterns of over connectivity between DMN and ECN and between vDMN and dDMN, was relevant to this hypothesis in several respects. As a major functional brain system, the DMN has been proven to play an important role in several psychiatric disorders including ASD (16) and ADHD (33). Previous studies indicate that the precuneus plays a core role not only in the DMN but also more broadly through its engagement under a variety of processing states (34–36). Its role in the DMN has been of particular interest because it shows the highest resting metabolic rate within the network, requiring ~35% more glucose than any other region in the human brain (37). Abnormalities of the precuneus in people with ASD (38) and ADHD (33, 39) have been revealed in numerous studies, one of which suggested that the right calcarine, left superior frontal gyrus (SFG), and DMN nodes (including the precuneus) play important roles in children with ASD and co-occurring anxiety and ADHD (40). The fMRI meta-analyses of cognitive control including 60 fMRI datasets revealed ASD-differentiating medial prefrontal under activation but overactivation in the bilateral ventrolateral prefrontal cortices and precuneus (41); our study also found that the ASD group showed increased within-network connectivity in the precuneus compared with the TD group. A previous study showed that there was diffused hypoconnectivity within the default network in people with ADHD (42), and the lack of overactivation in the PCC/precuneus in people with ADHD during cognitive control was apparent in previous meta-analyses (43) and could potentially be related to psychostimulant exposure, which has been shown to normalize DMN functioning (44). Compared with the TD group, comorbid ADHD may cause hypoconnectivity within the DMN in people with ASD, which increases the delta within-network connectivity between ASD+ADHD and ASD groups. In addition, the ASD+ADHD group showed increased between-network connectivity between the vDMN and dDMN.
New achievements have been made relative to the triple-network aberrant connectivity in other psychiatric conditions, such as schizophrenia. In 2020, Luo et al. (45) found an aberrant task-evoked increase in the influence of the right anterior insula (rAI) on the middle frontal gyrus (MFG) and precuneus. Control signals from the rAI (salience signaling) were abnormally elevated and directed toward both task-positive (ECN) and task-negative brain (DMN) regions, when task-related demands arise in schizophrenia, contributing to working memory deficiency. In our study, we mainly determined the aberrant interaction between the DMN and ECN, but whether it was caused by abnormal salience signals from specific brain regions is still unclear. Further study is needed to identify if there is an abnormality in sensory-salience circuity in the process system in children with ADHD and ASD symptoms. There has also been updated research on dynamic FNC (dFNC) in triple networks of psychiatric conditions. In 2019, Wang et al. (46) found that people with bipolar disorder and major depressive disorder spent more time in sparse connections with decreased dFNC variability between the posterior DMN and the right CEN. It revealed more common but less specific dFNC alterations in both conditions: whether it was the same in ADHD with and without ASD needs to be clarified, which may help us understand their abnormal cognitive functions clinically (46). Supervised convex non-negative matrix factorization was utilized to distinguish different psychiatric conditions in a latent low-dimensional space of the triple brain network, with high classification accuracy on the basis of the extracted structural and functional abnormalities, which inspired our further study (47).
The DMN participates in evaluative social processes (16), mentalizing and theory of mind (48, 49), which is important for the social understanding of others (50). Rather than reflecting the DMN as a unitary, homogenous system, recent imaging studies have tended to show DMN dissociation, with the anterior DMN being more engaged in self-referential and emotional processes and with the posterior DMN being involved in episodic memory and perceptual processing (51, 52). Previous reports have pointed out that disrupted intrinsic DMN organization in children and adults with ASD is related to social deficits (53–55), which is consistent with our study and further suggests that comorbid ADHD may aggravate hyperconnectivity between the dDMN and vDMN and lead to the aggravation of social dysfunction in people with ASD.
As in the proposed triple-network model, the SN, DMN, and ECN did not function independently. The DMN represented the task-negative processing mode for the human brain, whereas the ECN characterized the somewhat contrary task-positive processing mode. Increasingly, research has revealed the important role of cooperation between the SN, ECN, and DMN in maintaining cognitive functions (56, 57). In this study, the levels of DMN-ECN interaction in the ASD+ADHD group were higher than those in the ASD group. In addition, a previous study showed that people with ASD displayed increased connectivity between the DMN and ECN, and the strength of FC decreased with age (58). Overconnectivity in the ASD group observed for DMN–ECN pairings thus partly reflected reduced anticorrelations (rather than robust positive correlations), indicating a reduction in the typical segregation between networks, consistent with some previous reports (59, 60). Notably, the dysfunction of the DMN in the triple-network may be a neurobiological feature of ASD with ADHD co-occurrence and a potential image trait marker to help identify such subgroups of people with ASD. The SN is involved in monitoring behaviorally relevant salient stimuli and interrupting ongoing activity when appropriate, playing a dynamic switching role between the DMN and the ECN, which support self-related (or internally directed) and goal-oriented (or externally directed) cognition, respectively, to guide appropriate responses to salient stimuli (61). So far, we have not found statistically significant differences between the ASD+ADHD and ASD groups.
This study has some limitations. First, our analyses were performed on people with ASD who had comorbid ADHD and people with ASD who did not have comorbid ASD based on the dataset; however, we did not have ADHD symptom scores for the participants. Further research including both ASD and ADHD symptom scores is required. Moreover, this study ignored the diagnoses of other psychiatric comorbidities.
Conclusions
In conclusion, our data show that, in addition to impaired FC within the DMN in people with ASD and comorbid ADHD, they also demonstrate aberrant functional interactions between the vDMN and dDMN and between the vDMN and LCEN. This triple-network model provides a new and powerful framework for understanding the dysfunctional brain architecture of ASD with co-occurring ADHD.
Data Availability Statement
The original contributions presented in the study are included in the article/Supplementary Material, further inquiries can be directed to the corresponding authors.
Ethics Statement
Ethical review and approval was not required for the study on human participants in accordance with the local legislation and institutional requirements. Written informed consent to participate in this study was provided by the participants' legal guardian/next of kin.
Author Contributions
KW designed this study. KW and KL performed data preprocessing and analysis. XN and KL drafted the manuscript. All authors approved the final version for publication.
Conflict of Interest
The authors declare that the research was conducted in the absence of any commercial or financial relationships that could be construed as a potential conflict of interest.
Publisher's Note
All claims expressed in this article are solely those of the authors and do not necessarily represent those of their affiliated organizations, or those of the publisher, the editors and the reviewers. Any product that may be evaluated in this article, or claim that may be made by its manufacturer, is not guaranteed or endorsed by the publisher.
Acknowledgments
We would like to thank the numerous contributors to the ABIDE_II database for collecting, organizing, and sharing the datasets. Meanwhile, we thank the NeuroImaging Tools and Resources Collaboratory (http://www.nitrc.org) for providing the data sharing platform.
Supplementary Material
The Supplementary Material for this article can be found online at: https://www.frontiersin.org/articles/10.3389/fpsyt.2021.736755/full#supplementary-material
References
1. American Psychiatric Association. Diagnostic and Statistical Manual of Mental Disorders: DSM-5. Arlington, TX: American Psychiatric Association (2013).
2. Taurines R, Schwenck C, Westerwald E, Sachse M, Siniatchkin M, Freitag C, et al. ADHD and autism: differential diagnosis or overlapping traits? a selective review. Atten Defic Hyperact Disord. (2012) 4:115–39. doi: 10.1007/s12402-012-0086-2
3. Ameis SH. Heterogeneity within and between autism spectrum disorder and attention-deficit/hyperactivity disorder: challenge or opportunity? JAMA Psychiatry. (2017) 74:1093–4. doi: 10.1001/jamapsychiatry.2017.2508
4. Mueller A, Hong DS, Shepard S, Moore T. Linking ADHD to the neural circuitry of attention. Trends Cogn Sci. (2017) 21:474–88. doi: 10.1016/j.tics.2017.03.009
5. Gao Y, Shuai D, Bu X, Hu X, Tang S, Zhang L, et al. Impairments of large-scale functional networks in attention-deficit/hyperactivity disorder: a meta-analysis of resting-state functional connectivity. Psychol Med. (2019) 49:2475–85. doi: 10.1017/S003329171900237X
6. Sidlauskaite J, Sonuga-Barke E, Roeyers H, Wiersema JR. Altered intrinsic organisation of brain networks implicated in attentional processes in adult attention-deficit/hyperactivity disorder: a resting-state study of attention, default mode and salience network connectivity. Eur Arch Psychiatry Clin Neurosci. (2016) 266:349–57. doi: 10.1007/s00406-015-0630-0
7. Bos DJ, Oranje B, Achterberg M, Vlaskamp C, Ambrosino S, Reus MAde, et al. Structural and functional connectivity in children and adolescents with and without attention deficit/hyperactivity disorder. J Child Psychol Psychiatry. (2017) 58:810–8. doi: 10.1111/jcpp.12712
8. Hull JV, Dokovna LB, Jacokes ZJ, Torgerson CM, Irimia A, Van Horn JD. Resting-state functional connectivity in autism spectrum disorders: a review. Front Psychiatry. (2016) 7:205. doi: 10.3389/fpsyt.2016.00205
9. Tyszka JM, Kennedy DP, Paul LK, Adolphs R. Largely typical patterns of resting-state functional connectivity in high-functioning adults with autism. Cereb Cortex. (2014) 24:1894–905. doi: 10.1093/cercor/bht040
10. Raichle ME, Snyder AZ. A default mode of brain function: a brief history of an evolving idea. NeuroImage. (2007) 37:1083–90. doi: 10.1016/j.neuroimage.2007.02.041
11. Sridharan D, Levitin DJ, Menon V. A critical role for the right fronto-insular cortex in switching between central-executive and default-mode networks. Proc Natl Acad Sci U S A. (2008) 105:12569–74. doi: 10.1073/pnas.0800005105
12. Menon V, Uddin LQ. Saliency, switching, attention and control: a network model of insula function. Brain Struct Funct. (2010) 214:655–67. doi: 10.1007/s00429-010-0262-0
13. Menon V. Large-scale brain networks and psychopathology: a unifying triple network model. Trends Cogn Sci. (2011) 15:483–506. doi: 10.1016/j.tics.2011.08.003
14. Qian X, Castellanos FX, Uddin LQ, Loo BRY, Liu S, Koh HL, et al. Large-scale brain functional network topology disruptions underlie symptom heterogeneity in children with attention-deficit/hyperactivity disorder. NeuroImage Clinical. (2019) 21:101600. doi: 10.1016/j.nicl.2018.11.010
15. Abbott AE, Nair A, Keown CL, Datko M, Jahedi A, Fishman I, et al. Patterns of atypical functional connectivity and behavioral links in autism differ between default, salience, and executive networks. Cereb Cortex. (2016) 26:4034–45. doi: 10.1093/cercor/bhv191
16. Padmanabhan A, Lynch CJ, Schaer M, Menon V. The default mode network in autism. Biol Psychiatry Cogn Neurosci Neuroimaging. (2017) 2:476–86. doi: 10.1016/j.bpsc.2017.04.004
17. Sidlauskaite J, Sonuga-Barke E, Roeyers H, Wiersema JR. Default mode network abnormalities during state switching in attention deficit hyperactivity disorder. Psychol Med. (2016) 46:519–28. doi: 10.1017/S0033291715002019
18. Kernbach JM, Satterthwaite TD, Bassett DS, Smallwood J, Margulies D, Krall S, et al. Shared endo-phenotypes of default mode dsfunction in attention deficit/hyperactivity disorder and autism spectrum disorder. Transl Psychiatry. (2018) 8:133. doi: 10.1038/s41398-018-0179-6
19. Yan CG, Wang XD, Zuo XN, Zang YF. DPABI: data processing & analysis for (resting-state) brain imaging. Neuroinformatics. (2016) 14:339–51. doi: 10.1007/s12021-016-9299-4
20. Rissanen J. Modelling by the shortest data description. Automatica. (1978) 14:465–71. doi: 10.1016/0005-1098(78)90005-5
21. Calhoun VD, Adali T, Pearlson GD, Pekar JJ. A method for making group inferences from functional MRI data using independent component analysis. Hum Brain Mapp. (2001) 14:140–51. doi: 10.1002/hbm.1048
22. Calhoun VD, Adali T, Pearlson GD, Pekar JJ. Spatial and temporal independent component analysis of functional MRI data containing a pair of task-related waveforms. Hum Brain Mapp. (2001) 13:43–53. doi: 10.1002/hbm.1024
23. Himberg J, Hyvarinen A, Esposito F. Validating the independent components of neuroimaging time series via clustering and visualization. Neuroimage. (2004) 22:1214–22. doi: 10.1016/j.neuroimage.2004.03.027
24. Erhardt EB, Rachakonda S, Bedrick EJ, Allen EA, Adali T, Calhoun VD. Comparison of multi-subject ICA methods for analysis of fMRI data. Hum Brain Mapp. (2011) 32:2075–95. doi: 10.1002/hbm.21170
25. Stevens MC, Kiehl KA, Pearlson GD, Calhoun VD. Brain network dynamics during error commission. Hum Brain Mapp. (2009) 30:24–37. doi: 10.1002/hbm.20478
26. Shirer WR, Ryali S, Rykhlevskaia E, Menon V, Greicius MD. Decoding subject-driven cognitive states with whole-brain connectivity patterns. Cereb Cortex. (2012) 22:158–65. doi: 10.1093/cercor/bhr099
27. Meda SA, Gill A, Stevens MC, Lorenzoni RP, Glahn DC, Calhoun VD, et al. Differences in resting-state functional magnetic resonance imaging functional network connectivity between schizophrenia and psychotic bipolar probands and their unaffected first-degree relatives. Biol Psychiat. (2012) 71:881–9. doi: 10.1016/j.biopsych.2012.01.025
28. Smith SM, Nichols TE. Threshold-free cluster enhancement: addressing problems of smoothing, threshold dependence and localisation in cluster inference. Neuroimage. (2009) 44:83–98. doi: 10.1016/j.neuroimage.2008.03.061
29. Lord RMC, DiLavore PC, Risi S, Gotham K, Bishop SL. Autism Diagnostic Observation Schedule. 2nd ed. (ADOS-2). Torrance, CA: Western Psychological Services (2012).
30. Constantino JN, Davis SA, Todd RD, Schindler MK, Gross MM, Brophy SL, et al. Validation of a Brief Quantitative Measure of Autistic Traits: Comparison of the Social Responsiveness Scale with the Autism Diagnostic Interview-Revised. J Autism Dev Disord. (2003) 33:427–33. doi: 10.1037/t17260-000
31. Choi EY, Yeo BTT, Buckner RL. The organization of the human striatum estimated by intrinsic functional connectivity. J Neurophysiol. (2012) 108:2242–63. doi: 10.1152/jn.00270.2012
32. Factor RS, Ryan SM, Farley JP, Ollendick TH, Scarpa A. Does the presence of anxiety and ADHD symptoms add to social impairment in children with autism spectrum disorder? J Autism Dev Disord. (2017) 47:1122–34. doi: 10.1007/s10803-016-3025-9
33. Castellanos FX, Margulies DS, Kelly C, Uddin LQ, Ghaffari M, Kirsch A, et al. Cingulate-precuneus interactions: a new locus of dysfunction in adult attention-deficit/hyperactivity disorder. Biol Psychiatry. (2008) 63:332–7. doi: 10.1016/j.biopsych.2007.06.025
34. Li R, Utevsky AV, Huettel SA, Braams BR, Peters S, Crone EA, et al. Developmental maturation of the precuneus as a functional core of the default mode network. J Cogn Neurosci. (2019) 31:1506–19. doi: 10.1162/jocn_a_01426
35. Utevsky AV, Smith DV, Huettel SA. Precuneus is a functional core of the default-mode network. J Neurosci. (2014) 34:932–40. doi: 10.1523/JNEUROSCI.4227-13.2014
36. Fransson P, Marrelec G. The precuneus/posterior cingulate cortex plays a pivotal role in the default mode network: Evidence from a partial correlation network analysis. Neuroimage. (2008) 42:1178–84. doi: 10.1016/j.neuroimage.2008.05.059
37. Gusnard DA, Raichle ME, Raichle ME. Searching for a baseline: functional imaging and the resting human brain. Nat Rev Neurosci. (2001) 2:685–94. doi: 10.1038/35094500
38. Cheng W, Rolls ET, Gu H, Zhang J, Feng J. Autism: reduced connectivity between cortical areas involved in face expression, theory of mind, and the sense of self. Brain. (2015) 138:1382–93. doi: 10.1093/brain/awv051
39. Tang C, Wei Y, Zhao J, Nie J. Different developmental pattern of brain activities in ADHD: a study of resting-state fMRI. Dev Neurosci. (2018) 40:246–57. doi: 10.1159/000490289
40. Wan B, Wang Z, Jung M, Lu Y, He H, Chen Q, et al. Effects of the co-occurrence of anxiety and attention-deficit/hyperactivity disorder on intrinsic functional network centrality among children with autism spectrum disorder. Autism Res. (2019) 12:1057–68. doi: 10.1002/aur.2120
41. Lukito S, Norman L, Carlisi C, Radua J, Hart H, Simonoff E, et al. Comparative meta-analyses of brain structural and functional abnormalities during cognitive control in attention-deficit/hyperactivity disorder and autism spectrum disorder. Psychol Med. (2020) 50:894–919. doi: 10.1017/S0033291720000574
42. Kelly C, Zuo X-N, Gotimer K, Cox CL, Lynch L, Brock D, et al. Reduced interhemispheric resting state functional connectivity in cocaine addiction. Biol Psychiatry. (2011) 69:684–92. doi: 10.1016/j.biopsych.2010.11.022
43. Norman LJ, Carlisi C, Lukito S, Hart H, Mataix-Cols D, Radua J, et al. Structural and functional brain abnormalities in attention-deficit/hyperactivity disorder and obsessive-compulsive disorder: a comparative meta-analysis. JAMA Psychiatry. (2016) 73:815–25. doi: 10.1001/jamapsychiatry.2016.0700
44. Rubia K, Alegria AA, Cubillo AI, Smith AB, Brammer MJ, Radua J. Effects of stimulants on brain function in attention-deficit/hyperactivity disorder: a systematic review and meta-analysis. Biol Psychiatry. (2014) 76:616–28. doi: 10.1016/j.biopsych.2013.10.016
45. Luo Q, Pan B, Gu H, Simmonite M, Francis S, Liddle PF, et al. Effective connectivity of the right anterior insula in schizophrenia: the salience network and task-negative to task-positive transition. NeuroImage Clin. (2020) 28:102377. doi: 10.1016/j.nicl.2020.102377
46. Wang J, Wang Y, Huang H, Jia Y, Zheng S, Zhong S, et al. Abnormal dynamic functional network connectivity in unmedicated bipolar and major depressive disorders based on the triple-network model. Psychol Med. (2020) 50:465–74. doi: 10.1017/S003329171900028X
47. Han W, Sorg C, Zheng C, Yang Q, Zhang X, Ternblom A, et al. Low-rank network signatures in the triple network separate schizophrenia and major depressive disorder. Neuroimage Clin. (2019) 22:101725. doi: 10.1016/j.nicl.2019.101725
48. Buckner RL, Andrews-Hanna JR, Schacter DL. The brain's default network - anatomy, function, and relevance to disease. Ann Ny Acad Sci. (2008) 1124:1–38. doi: 10.1196/annals.1440.011
49. Schilbach L, Eickhoff SB, Rotarska-Jagiela A, Fink GR, Vogeley K. Minds at rest? Social cognition as the default mode of cognizing and its putative relationship to the “default system” of the brain. Conscious Cogn. (2008) 17:457–67. doi: 10.1016/j.concog.2008.03.013
50. Li W, Mai X, Liu C. The default mode network and social understanding of others: what do brain connectivity studies tell us. Front Hum Neurosci. (2014) 8:74. doi: 10.3389/fnhum.2014.00074
51. Zhu X, Wang X, Xiao J, Liao J, Zhong M, Wang W, et al. Evidence of a dissociation pattern in resting-state default mode network connectivity in first-episode, treatment-naive major depression patients. Biol Psychiatry. (2012) 71:611–7. doi: 10.1016/j.biopsych.2011.10.035
52. Coutinho JF, Fernandesl SV, Soares JM, Maia L, Gonçalves ÓF, Sampaio A. Default mode network dissociation in depressive and anxiety states. Brain Imaging Behav. (2016) 10:147–57. doi: 10.1007/s11682-015-9375-7
53. Chen H, Duan XJ, Liu F, Lu FM, Ma XJ, Zhang YX, et al. Multivariate classification of autism spectrum disorder using frequency-specific resting-state functional connectivity-a multi-center study. Prog Neuro-Psychoph. (2016) 64:1–9. doi: 10.1016/j.pnpbp.2015.06.014
54. Lynch CJ, Uddin LQ, Supekar K, Khouzam A, Phillips J, Menon V. Default mode network in childhood autism: posteromedial cortex heterogeneity and relationship with social deficits. Biol Psychiat. (2013) 74:212–9. doi: 10.1016/j.biopsych.2012.12.013
55. Yerys BE, Gordon EM, Abrams DN, Satterthwaite TD, Weinblatt R, Jankowski KF, et al. Default mode network segregation and social deficits in autism spectrum disorder: evidence from non-medicated children DMN in children with ASD. Neuroimage Clin. (2015) 9:223–32. doi: 10.1016/j.nicl.2015.07.018
56. Kelly AM, Uddin LQ, Biswal BB, Castellanos FX, Milham MP. Competition between functional brain networks mediates behavioral variability. Neuroimage. (2008) 39:527–37. doi: 10.1016/j.neuroimage.2007.08.008
57. Pu W, Luo Q, Palaniyappan L, Xue Z, Yao S, Feng J, et al. Failed cooperative, but not competitive, interaction between large-scale brain networks impairs working memory in schizophrenia. Psychol Med. (2016) 46:1211–24. doi: 10.1017/S0033291715002755
58. Cerliani L, Mennes M, Thomas RM, Martino ADi, Thioux M, Keysers C. Increased functional connectivity between subcortical and cortical resting-state networks in autism spectrum disorder. JAMA Psychiatry. (2015) 72:767–77. doi: 10.1001/jamapsychiatry.2015.0101
59. Shih P, Keehn B, Oram JK, Leyden KM, Keown CL, Müller RA. Functional differentiation of posterior superior temporal sulcus in autism: a functional connectivity magnetic resonance imaging study. Biol Psychiatry. (2011) 70:270–7. doi: 10.1016/j.biopsych.2011.03.040
60. Rudie JD, Shehzad Z, Hernandez LM, Colich NL, Bookheimer SY, Iacoboni M, et al. Reduced functional integration and segregation of distributed neural systems underlying social and emotional information processing in autism spectrum disorders. Cereb Cortex. (2012) 22:1025–37. doi: 10.1093/cercor/bhr171
Keywords: autism, attention deficit hyperactivity disorder, co-occurring, triple-network model, default mode network
Citation: Wang K, Li K and Niu X (2021) Altered Functional Connectivity in a Triple-Network Model in Autism With Co-occurring Attention Deficit Hyperactivity Disorder. Front. Psychiatry 12:736755. doi: 10.3389/fpsyt.2021.736755
Received: 06 July 2021; Accepted: 04 November 2021;
Published: 02 December 2021.
Edited by:
Martine Hoogman, Radboud University Nijmegen, NetherlandsReviewed by:
Drozdstoy Stoyanov Stoyanov, Plovdiv Medical University, BulgariaPaola Valsasina, San Raffaele Scientific Institute (IRCCS), Italy
Copyright © 2021 Wang, Li and Niu. This is an open-access article distributed under the terms of the Creative Commons Attribution License (CC BY). The use, distribution or reproduction in other forums is permitted, provided the original author(s) and the copyright owner(s) are credited and that the original publication in this journal is cited, in accordance with accepted academic practice. No use, distribution or reproduction is permitted which does not comply with these terms.
*Correspondence: Kai Wang, wwwkkk8815@163.com
†These authors share first authorship