- 1Centre for Psychiatry Research, Department of Clinical Neuroscience, Karolinska Institutet, & Region Stockholm Health Care Services, Region Stockholm, Stockholm, Sweden
- 2Centre for Dependency Disorders, Region Stockholm, Stockholm, Sweden
Background: Numerous trials have demonstrated the efficacy of internet interventions targeting alcohol or cannabis use, yet a substantial proportion of users do not benefit from the format, warranting further research to identify moderators of treatment effects. Users' initial attitudes toward treatment is a potential moderator, yet no previous study has investigated users' attitudes in the context of internet interventions for addictive disorders.
Method: In this secondary analysis on two internet-based trials targeting harmful alcohol use (n = 1,169) and regular cannabis use (n = 303), respectively, we compared user groups' attitudes at the item level; explored within-group heterogeneity by submitting attitude scores to a k-means cluster analysis; and investigated whether latent subgroups in each user group moderated the treatment effects. Outcome models were run using generalized linear models with 10,000 bias-corrected bootstraps accounting for subject-level clustering.
Results: While substance groups and latent subgroups converged in enjoying the anonymity provided by the format, their interest toward treatment differed. Outcome analyses revealed a significant and negative time by subgroup effect on grams of cannabis consumed and screening test score (CAST), favoring the subgroup with positive treatment attitudes. There were not any significant effects of subgroup on alcohol consumption. Despite initial treatment reluctance, participants in the neutral subgroup decreased their cannabis use (gram) significantly when receiving the intervention vs. control.
Conclusions: This first, exploratory study revealed key differences between substance groups' attitudes, but more importantly that within-group heterogeneity appear to affect cannabis outcomes. Assessing attitudes could be key in patient-treatment matching, yet more research is needed.
Introduction
Alcohol and cannabis are among the most used psychoactive substances globally (1). Every third user is likely to experience a transition from recreational use to addiction at some point in their life (2), entailing substantial psychosocial and monetary costs for the affected individual, significant others and society at large (3). Even though there are effective, evidence-based interventions only an estimated 19.8% with alcohol use disorder (AUD) and 13.2% with cannabis use disorder (CUD) will ever seek treatment (4, 5). Perceived and institutional barriers to seeking and entering treatment for alcohol use include treatment unavailability (6, 7), and attitudinal factors such as fear of stigma and shame (8–11) and finding traditional treatment services unattractive (10). In addition to these hinders, treatment-seeking for cannabis use is associated with particular challenges: many users view cannabis as an important part of their identity that demonstrates independence and free-thinking (12, 13). Congruently, a common top reported reason for not seeking cannabis treatment is the desire for self-reliance (14, 15). This likely contributes to the considerably low treatment uptake for individuals with CUD (14).
In addition to resolving many of the institutional barriers to disseminating evidence-based treatments at scale and at a low cost (16), internet interventions also have the potential to overcome many of the attitudinal barriers to treatment seeking: past research has revealed that service users (hereafter labeled users) experience internet interventions targeting alcohol use as accessible (6) and safekeeping privacy (6, 17). The interventions typically involve cognitive behavioral skill building exercises (identifying high-risk situations, craving management, etc.), formulated according to the principles of motivational interviewing, with or without therapist guidance through asynchronous text communication. It has been proposed that internet interventions may be particularly suitable for satisfying cannabis-dependent individuals' desire for self-reliance (15), yet it has not been empirically examined. Indeed, little is known how users perceive internet interventions for regular cannabis use, and it is unclear how their views converge and differ from those of users of alcohol internet interventions. Increased knowledge about the user groups' views toward features and components of internet-based treatments, as well as factors that facilitate treatment-seeking, would be of great utility in developing transdiagnostic designs, tailoring the interventions to each target group, and validly translating insights from each respective treatment group.
A growing number of trials support the efficacy of internet interventions in reducing alcohol and cannabis consumption (16–19), yet a substantial proportion of users do no benefit from the interventions and the effect sizes for interventions targeting regular cannabis use tend to be small (18). A potential moderator of treatment effects that has been overlooked in the extant literature is the attitudes that users hold toward internet interventions. Attitudes, referring to the degree to which a person evaluates a behavior or an entity as favorable or not (20, 21), is a key concept in health and social psychology that is hypothesized to be a determinant of intention and behavior change—a claim that has gained empirical support (22). The concept of attitudes has been adopted to explain acceptance toward emerging digital technologies, such in the original Technology Acceptance Model (23), and has during the last decade been recognized to be a specifically important research target for the field of internet interventions (24). Several studies have investigated the general public's (25, 26), stakeholders' (27), clinicians' (28–30) and different patient populations' (31, 32) attitudes toward internet interventions, yet only a handful of studies have investigated whether users' attitudes at treatment entry affect outcome.
A recent study on a 1-month transdiagnostic intervention targeting anxiety symptoms found that initial attitudes moderated the treatment effect with a small effect size relative to care as usual, such that users with positive initial attitudes who received the intervention reported greater symptom decrease over time (33). Similarly, it has been demonstrated that the effect of an online intervention for depression was contingent on initial attitudes, also in favor of positive initial attitudes with a small effect size (34). Importantly, subscale analysis revealed that participants with greater negative baseline-attitudes as measured by the scale “technologization threat,” which covers reluctance to participating in computerized treatment instead of in-person contact, experienced increased depression symptom over time compared to the control group (34). Although not relying on direct moderation analyses, another study on depression showed that positive initial attitudes toward online treatment predicted better outcomes and that users with greater negative baseline attitudes were more likely to discontinue their online treatment (35).
The extant literature thus suggests that baseline attitudes are associated with differential treatment effects. Apart from some subscale analyses, previous research has used unidimensional sum scores which may not capture the full complexity of attitudes. Specifically, this may disregard attitudinal ambivalence: holding both positive and negative appraisals toward an attitude object (36). Moreover, considering that internet trials typically have lower threshold for entry (e.g., liberal inclusion criteria, minimal waiting time) than traditional services, and recruit participants beyond professional help systems, it is likely that the trials include a more heterogeneous sample with respect to attitudes. Such heterogeneity can be explored with analytical approaches that attempt to cluster individuals into latent subgroups that share similar patterns of attributes (37), in this specific case attitudes, going beyond aggregated scores by identifying specific constellations of responses. To our knowledge, no previous study has explored the impact of users' attitudes toward internet interventions targeting any addictive disorder or whether latent subgroups of users, derived from patterns of attitudes, moderate outcomes.
The aim of the current study was three-fold: (a) to examine difference in specific attitudes toward internet interventions among users of programs for alcohol vs. cannabis use; (b) to explore latent subgroups based on attitudes in each of the two groups of users; and (c), to explore whether such subgroups moderated the respective treatment effect.
Method
Sample and Procedure
This study is a secondary analysis of data from two randomized controlled trials of internet interventions for harmful alcohol use or dependence (38) and regular cannabis use (39), respectively. See each respective publication for details on participant recruitment, procedure, treatments and full outcomes reporting. In brief, the trial targeting alcohol use (N = 1,169) compared a 12-week therapist-guided intervention and self-help intervention with an information-only control (1:1:1) at 3- and 6-months follow-ups. Users of the therapist-led intervention had greater decrease in alcohol consumption at 3 months compared to the control, although other time by group effects were not significant (38). The trial targeting regular cannabis use (N = 303) compared a guided self-help intervention with a wait-list control (WLC) in a ratio of (1:1), showing no significant time by group effects on cannabis use at the 3-month follow-up, yet such effects were revealed in the subset of participants that had not sought other professional help during the trial (39). In both trials, all participants were asked to rate their attitudes toward internet interventions (see full description of measure below) before entering the program, of which n = 1,037 + 256 participants answered the form, implying a coverage rate of 89% and 84% in the alcohol and cannabis program, respectively. The current study uses these ratings, along with baseline variables and outcome measures from each trial. Missing data in attitude items were handled using either case-wise omission (if percentage missing data exceeded 10%) or imputed using the case-wise median. Following this criterion, 4 of 1,293 subjects were omitted and 47 values imputed. The final sample consisted of n = 1,289 participants: n = 1,037 users of the alcohol program and n = 252 users of the cannabis program. Both the alcohol (2014/1758-31/2) and cannabis trials (2014/1374-31/5) were approved by the Swedish Ethical Review Authority, as were the specific analyses described herein (2021-02322). All participants provided informed consent.
Measures
To measure users' attitudes toward web-based programs, both studies included a newly developed 33-item form (see Supplementary Material) that covered three aspects of believed importance: reasons for choosing internet-based treatment (7 items), views on features of the program (16 items) and views on the specific treatment components (10 items). The form included three additional items in the alcohol trial that were excluded to enable user-group comparisons. All items were rated on a 11-point scale ranging from 0-10, with verbal anchors at 0 (“Completely unimportant, does not need to be included”) and 10 (“Very important, I would not consider using the service without this feature”). The items were identical across the studies except for terms referring to each user group. For presentation purposes herein, substance-specific terms were substituted with the generic term “substance” and items were translated from Swedish by DR, followed by independent back-translation by PL to ensure validity. The questionnaire showed satisfactory internal consistency in each substance group, as measured by McDonald's Omega (Alcohol: ω = 0.849, 95% Wald CI = 0.832-−0.867; Cannabis: ω = 0.852, 95% Wald CI = 0.817 −0.887) (40). Readiness to change substance use was measured in both trials using the 100-point Visual Analog Scale (41).
Primary outcome measures in the alcohol and cannabis trials were past-week number of standard drinks and past-week number of days of cannabis non-use, respectively. Both these measures were extracted from data that was collected using the Timeline Follow-Back method (42). Secondary outcome measures included past-week binge-drinking (the original count response format was dichotomized in this study due to severe overdispersion: ϕ = 2.367), the Swedish 10-item version of the Alcohol Use Disorder Identification Test (43), past-week consumption of cannabis in gram, and the Swedish 12-item version of the Cannabis Abuse Screening Test (CAST) (44), in the respective trials, as well as self-reported alcohol or cannabis use disorder criteria (45).
Analyses
All analyses were performed using the R (version 4.1.0) statistical environment (46). Data was analyzed in three steps, corresponding to the three research questions. First, means and parametric 95% confidence intervals were calculated for each item, and then compared between the alcohol and cannabis groups. Second, to explore latent subgroups in each respective group, raw item-level scores were submitted to a k-means cluster analysis for each group separately. K-means is a learning algorithm that partitions a group of observations into clusters, given a specified parameter k (47, 48). The algorithm starts by establishing k centers randomly, and then alternates between assigning each observation to the closest cluster center and repositioning the cluster centers to the temporary mean of the affiliated observations, in a process that iterates until the centers become fixed. We set the distance metric to Manhattan (L1 norm), as it has been suggested to perform better than the default Euclidean metric (L2 norm) in high-dimensional data sets that features 20 or more variables (49). A central issue in clustering is specifying the parameter k (50). Numerous indices exist and these often generate competing suggestions. To not rely on a single index, we evaluated possible cluster solutions (ranging 2–10 clusters) using 26 fit indices provided by the NbClust R package (51). We selected the cluster solution that was suggested by most indices. Next, we used item-level means and parametric 95% confidence intervals to explore attitudinal differences between the subgroups. To assess the effects of user groups and latent subgroups on treatment attitudes, above and beyond the effect of age and readiness to change, respectively, we ran separate multiple linear regressions at the item level.
Finally, having explored latent subgroups in each respective group, we investigated whether attitude cluster moderated treatment effects in each substance group, by including cluster affiliation as a predictor with three-way interactions in generalized linear models that additionally featured time and treatment predictors, along with full-factorial interaction terms and 2 × 2 × 3-factorial interaction terms for the cannabis-use and alcohol-use group, respectively. As there were no significant changes in outcomes between 3- and 6-months follow up in the alcohol-use group as well as high attrition (38), the follow-up time-points were collapsed to retain power. These models were run separately for each substance group using the relatively assumption-free cluster-bootstrap generalized linear modeling approach with subject-level clustering and 10 000 bias-corrected bootstraps (52).
Results
Comparison Between User Groups
Substance groups differed significantly in mean scores on more than a third of the items (13 of 33 items). On items covering possible reasons for seeking internet-based treatment, both substance groups saw great value in anonymity, but the aspect of not having to disclose treatment-seeking to other people was on average higher valued in subjects with CUD, as was the autonomy-emphasizing aspect that the program allow the user to set his or her own goal, whereas the alcohol-use group had a higher preference for being able to access treatment at any time. In general, both substance groups endorsed the treatment components that reinforce intra-individual skills, such as dealing with cravings, whereas treatment ingredients that target inter-individual skills (e.g., support in dealing with issues in close relationships) were considered less important. The core treatment components of goal-setting support, relapse-prevention and motivation-elicitation were higher valued by the alcohol-use group. The cannabis-use group reported no higher preference for any of the treatment components compared to the alcohol-use group. Regarding the format of the program, the alcohol-use group considered it more important to be able to have contact with a therapist through the internet and receive personal feedback. In contrast, the cannabis-use group had a higher preference for the informational content of the program, i.e., that the program included facts about how cannabis affects health and included references to scientific sources. See Figure 1 for item-level mean scores and 95% confidence intervals. Attitudinal differences between substance groups were significant above and beyond the effect of age in 9 of the 13 items that originally displayed between-group differences in means (see Supplementary Table 1).
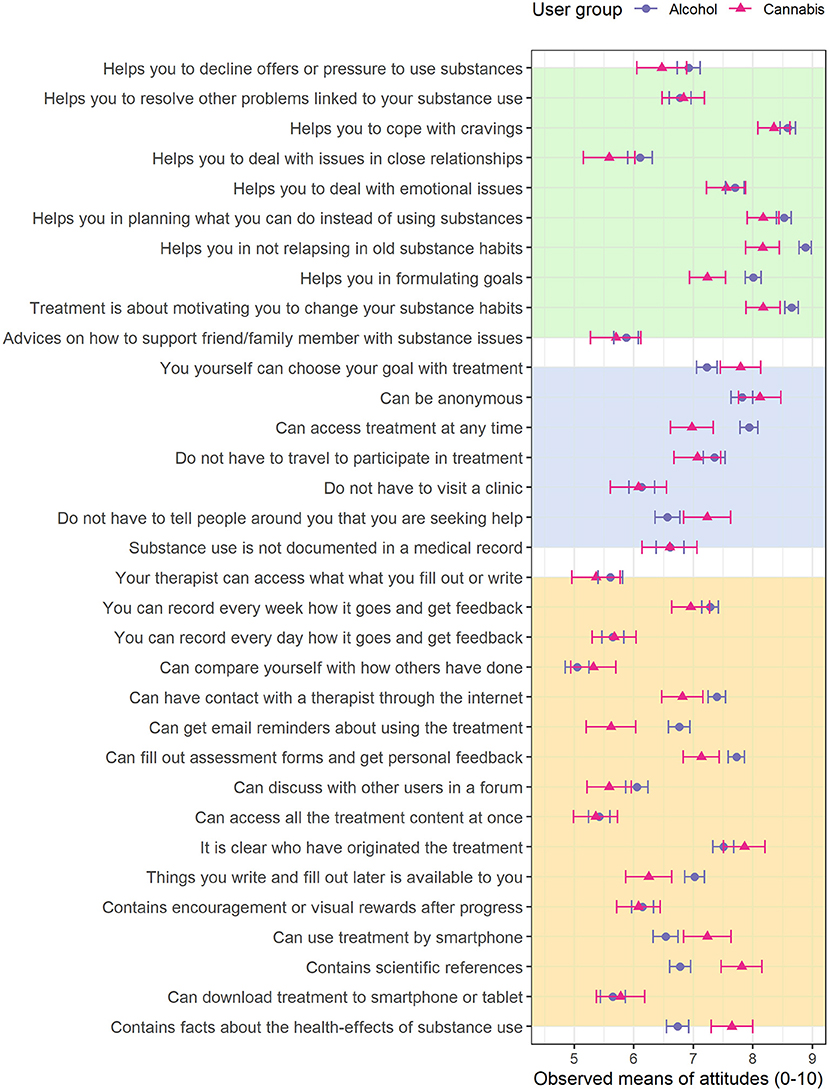
Figure 1. Observed means at the item level, grouped by substance group. The error-bars represent the parametric 95% confidence interval (CI). Background colors represent question category (Yellow: treatment features; Blue: reasons for seeking internet-based treatment; Green: treatment components).
Latent Subgroups
The optimal cluster solution for the attitude ratings was k = 2, favored by 10 of 26 indices in both user groups (see Supplementary Table 2 for model fit indices and Supplementary Figure 1 for the two graphical indices). Latent subgroups differed significantly on all but a few items in the alcohol-use group (30 of 33 items) and on most items in the cannabis-use group (25 of 33 items). The first subgroup gave consistently lower attitude ratings than the second subgroup (and vice versa) across all item-level differences in both substance groups (Figure 2). Grand mean differences between latent subgroups motivated the labels of “Neutral attitudes” for the first subgroup (Alcohol: M = 6.01, N = 525; Cannabis: M = 5.65, N = 100) and “Positive attitudes” for the second subgroup (Alcohol: M = 7.89, N = 512; Cannabis: M = 7.56, N = 152) in both user groups. The latent subgroups exhibited similar constellations of responses across substance groups: the neutral and positive subgroups in each substance group differed in appraisal of the treatments' components and features but converged in endorsing anonymity aspects. However, a closer comparison of the response patterns that constituted the neutral subgroup in each substance group revealed differences: the neutral subgroup in the alcohol trial seemed to view the treatment with overall uninterest, only approving of the anonymity aspects, whereas the neutral subgroup in the cannabis-use group additionally approved of goal-setting autonomy, availability and transparency aspects, suggesting a basic interest in engaging with the material but not necessarily for treatment purpose. Item-level mean differences between latent subgroups remained significant in most cases (Alcohol trial: 27 of 30 mean differences; Cannabis trial: 18 of 25 mean differences) when accounting for readiness to change (Supplementary Table 3).
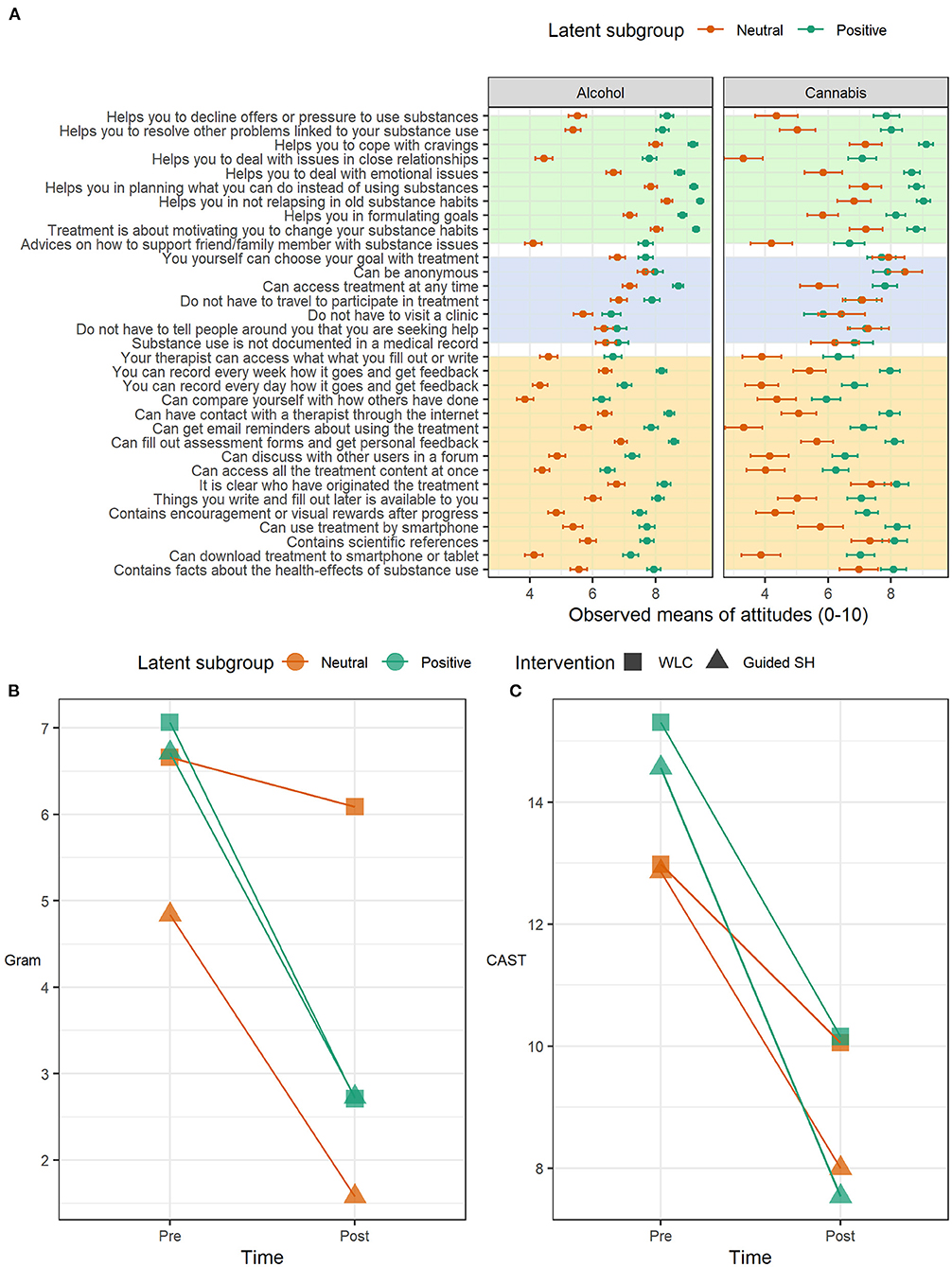
Figure 2. (A) Observed means at the item level, grouped by latent subgroup within each respective user group. The error-bars represent the parametric 95% confidence interval (CI). Background colors represent question category (Yellow: treatment features; Blue: reasons for seeking internet-based treatment; Green: treatment components). The lower panel contains outcome plots for observed means in cannabis consumption over time grouped by intervention (WLC, Wait-list control; SH, Self-help) and latent subgroup. (B) Self-reported grams used during the previous seven days; (C) 12-item version of the Cannabis Abuse Screening Test.
Associations With Outcomes
Internet Interventions for Harmful Alcohol Use
There was a main effect of time across all outcomes. There were not any significant effects of attitude subgroup on any outcome: neither main effects nor two- or three-ways interactions effects with time and/or treatment predictors (Table 1).
Guided Self-Help for Regular Cannabis Use
There were significant positive main effects of subgroup on cannabis consumption (grams), CAST and diagnostic criteria, implying higher baseline severity among users with positive treatment attitudes (Table 2). Significant negative time by subgroup effects on gram and CAST revealed that the positive subgroup experienced superior improvement over time relative to the neutral subgroup. Average changes in cannabis consumption (gram) and CAST over time grouped by treatment and latent subgroup are shown Figure 2. Time by subgroup interaction effects on gram and CAST-score remained significant (Gram: B = −0.73, CI = −1.464, −0.196; CAST: B = −2.46, CI = −4.815, −0.226) in post-hoc analysis excluding participants who had sought other professional help. Post-hoc analysis conducted on the subgroup with neutral attitudes revealed a statistically significant time by treatment interaction effect on the secondary outcome gram (B = −1.06, CI = −1.67, −0.266). For full report of post-hoc model parameters, see Supplementary Table 4. There was not any significant main effect of positive subgroup (B = 0.10, SE = 0.38, p = −0.788) or interaction effect between positive subgroup and WLC (B = 0.73, SE = 0.54, p = 0.175) on loss to follow up.
Discussion
The present study explored and compared users' attitudes toward anonymous internet interventions targeting alcohol use and cannabis use. While the substance groups generally converged in enjoying the unique aspects of internet interventions (e.g., anonymity, no transportation required), our results suggest that users of the alcohol program give higher priority to individual treatment components, such as relapse prevention, whereas users of the cannabis program to a greater extent value the informational and autonomy-emphasizing features of the program. Our data highlight the need to explore within-group heterogeneity in users' attitudes. We showed that the interest toward the cannabis program as an informational service was restricted to a subgroup of users rather than the overall sample, and that there was indeed an unobserved subgroup of users that put high value on the treatment components, signaling an intention to engage in treatment. Importantly, this interest toward treatment predicted decreased cannabis consumption over time, independent of treatment allocation. Latent subgroups in the alcohol trial were characterized by positive and neutral attitudes, respectively, but within-group heterogeneity was not significantly associated with treatment outcome.
In line with prior research on users' attitudes toward internet interventions for other disorders (33–35), positive attitudes predicted superior outcome for users of the cannabis trial. This suggests that attitudes toward treatment to some degree have a generalizable and transdiagnostic relevance in predicting outcome, yet the attitude-outcome association was not significant for users of the alcohol intervention. Noteworthy, as concerns the outcomes standard drinks and binge-drinking, the bias-corrected confidence intervals for the three-way interaction effects only slightly overlapped the null. Without implying that a larger sample size would prove these effects to be significant (53)—indeed the sample size in the alcohol trial was more than four times as large as the cannabis sample—further observations in independent samples are needed to fully reject the hypothesis that initial attitudes moderate alcohol treatment outcomes. Increased understanding about whether the attitude-outcome association differs across patient populations could be important for concentrating research efforts, and selectively translating findings into clinical practice. A possible explanation for the non-significant results could be that within-group differences in attitudes among users of alcohol interventions are not optimally represented as stemming from distinct subgroups of users, but rather are unidimensional. Our finding that subgroups in the alcohol trial differed in overall appraisal of the program, whereas subgroups in the cannabis trial demonstrated more complex response patterns—shared a basic interest in the program while differing in interest toward treatment—appear to support such an interpretation, yet it should be considered that the trials had unequal sample sizes and thereby unequal statistical power to detect subgroup differences.
Although there is a growing literature that positive attitudes moderate outcome, little is known about the symptom trajectories of users with negative or neutral attitudes. Our post-hoc analysis demonstrated that even the subgroup with neutral attitudes in the cannabis trial—the subgroup that initially had a basic interest in the material but little interest in using it for treatment purposes—benefitted from the intervention relative to WLC, as shown by a significantly greater decrease in consumption (grams of cannabis). If this finding can be replicated in independent samples, it would not only confirm the potential of guided self-help in attracting people who are reluctant to engage in treatment, but also its capability in eliciting motivation and inducing behavior change despite initial treatment reluctance. Considering the notable treatment gap among individuals with CUD, and the desire for self-reliance being the top attitudinal barrier to treatment-seeking, this would have large clinical and public health value. Importantly, individuals in the subgroup with neutral attitudes that received treatment had lower baseline consumption compared to WLC, indicating lower levels of addiction severity. It may be that guided self-help is particularly beneficial for individuals in the early stages of addiction progression that are reluctant to engage in treatment. These findings must however be interpreted with caution as they emerged from post-hoc analysis.
To our surprise, the attitude-outcome association was not restricted to treatment but also present in WLC in the cannabis trial. Importantly, participants in both conditions were blinded to when the other arm would receive treatment. As there neither was a main effect of subgroup nor a subgroup by WLC interaction effect on loss to follow up, the observed wait-list improvement is unlikely to spring from attrition bias. Further, the time by subgroup interaction effects remained significant when excluding subjects that had sought other help, thus, external interventions were not the source of wait-list improvement. However, it is possible that time by subgroup interaction effect was due to omitted variable bias: in specific, it is well-documented in the addiction field that novel, negative consequences related to the substance use simultaneously can increase the motivation to seek help (54–56) and induce sudden behavior change. Considering that heightened willingness to seek help may positively influence the attitudes toward the requested treatment, this bias is likely augmented for the positive subgroup. Interestingly though, the positive subgroup had high baseline levels of cannabis consumption, even significantly greater than the neutral subgroup (on three of four outcome measures), suggesting that treatment-seeking preceded behavior change. In view of these findings, we hypothesize that mere participation in baseline measurements had a positive influence on the subgroup of users with positive attitudes. More specifically, baseline measurements could have triggered increased awareness and self-monitoring (57), eliciting behavior change even in absence of the intervention (58). Positive assessment effects has been suggested to be a cause of wait-list improvement in psychological trials (57) and has also previously been observed in the addiction literature (59, 60). If these attitude-outcome findings in the cannabis trial replicate they could have substantial implications for patient-treatment matching: high-intensity therapist resources could be allocated to the subgroup with neutral attitudes, whereas the subgroup with initial positive attitudes could be offered automated or low-intensity therapist-led interventions, potentially optimizing the outcome of internet interventions targeting regular cannabis use.
In summary, our exploratory analyses revealed that there are key differences between substance groups' attitudes toward treatment but more importantly that within-group heterogeneity specifically matters for cannabis outcomes. Considering the low treatment utilization in addictive disorders, and the potential of internet interventions in overcoming some of the barriers to treatment, there is a need for further research for whom it works, with attitudes potentially being a moderator of large clinical value.
Limitations
This first study on users' attitudes toward internet interventions for addictive disorders has some limitations. First, although we evaluated possible cluster solutions using 26 fit indices, current applications of the k-means algorithm do not allow testing for and rejecting sample homogeneity (k = 1) in multivariate data. Second, the instrument used to measure attitudes was custom-made and had not been independently validated, nor were comprehensive psychometric analyses included in the current study. Internal consistency was however satisfactory, and the analytical approach focused on the item rather than scale level, rendering many standard psychometric evaluation indices superfluous. Third, the current study was not designed to evaluate the impact of a full set of potential differences (e.g., socio-economic status) that are likely to exists between cannabis and alcohol users; importantly, regardless of indirect attribution, our findings nonetheless have implications for the treatment of these groups as they present. Moreover, we did include age and readiness to change as covariates in some models. Future research should examine further constructs that potentially confounds between- and within-group differences in attitudes. Fourth, the survey underlying these secondary analyses was not mandatory, possibly introducing self-selection bias as users with greater positive attitudes could have been more inclined to answer the form. However, importantly, the survey had high coverage rates in both trials and within-group heterogeneity in attitudes was great regardless, indicating no sampling bias.
Data Availability Statement
The data that support the findings of this study are available from the corresponding author upon reasonable request.
Ethics Statement
The studies involving human participants were reviewed and approved by Swedish Ethical Review Authority. The patients/participants provided their written informed consent to participate in this study.
Author Contributions
DR, MJ, and PL: designed the study. MJ and UH: collected data, made substantial contributions to analysis, or interpretation of findings. DR and PL: analyses and drafted the manuscript. All authors contributed in revising the manuscript for important intellectual content and approved the final version to be published.
Funding
DR was funded by Region Stockholm and the Swedish Research Council for Health, Working Life and Welfare (2019-01642). The alcohol and cannabis trials were supported by separate grants.
Conflict of Interest
The authors declare that the research was conducted in the absence of any commercial or financial relationships that could be construed as a potential conflict of interest.
Publisher's Note
All claims expressed in this article are solely those of the authors and do not necessarily represent those of their affiliated organizations, or those of the publisher, the editors and the reviewers. Any product that may be evaluated in this article, or claim that may be made by its manufacturer, is not guaranteed or endorsed by the publisher.
Acknowledgments
We would like to thank all colleagues at the Addiction eClinic for delivering the guided interventions in the original trials and providing valuable input to the interpretation of this study. We would also like to thank Kristina Sinadinovic for her lead role in conducting the clinical trial of the cannabis program and assisting in the ethical application of this study, and Sven Andréasson for critically reviewing and providing valuable feedback on the draft of the manuscript.
Supplementary Material
The Supplementary Material for this article can be found online at: https://osf.io/SQ52M/
References
1. Peacock A, Leung J, Larney S, Colledge S, Hickman M, Rehm J, et al. Global statistics on alcohol, tobacco and illicit drug use: 2017 status report. Addiction. (2018) 113:1905–26. doi: 10.1111/add.14234
2. Marel C, Sunderland M, Mills KL, Slade T, Teesson M, Chapman C. Conditional probabilities of substance use disorders and associated risk factors: progression from first use to use disorder on alcohol, cannabis, stimulants, sedatives and opioids. Drug Alcohol Depend. (2019) 194:136–42. doi: 10.1016/j.drugalcdep.2018.10.010
3. Degenhardt L, Charlson F, Ferrari A, Santomauro D, Erskine H, Mantilla-Herrara A, et al. The global burden of disease attributable to alcohol and drug use in 195 countries and territories, 1990-2016: a systematic analysis for the Global Burden of Disease Study 2016. Lancet Psychiatry. (2018) 5:987–1012. doi: 10.1016/S2215-0366(18)30337-7
4. Grant BF, Goldstein RB, Saha TD, Chou SP, Jung J, Zhang H, et al. Epidemiology of DSM-5 alcohol use disorder. JAMA Psychiatry. (2015) 72:757. doi: 10.1001/jamapsychiatry.2015.0584
5. Hasin DS, Kerridge BT, Saha TD, Huang B, Pickering R, Smith SM, et al. Prevalence and correlates of DSM-5 cannabis use disorder, 2012-2013: findings from the national epidemiologic survey on alcohol and related conditions-III. Am J Psychiatry. (2016) 173:588–99. doi: 10.1176/appi.ajp.2015.15070907
6. Khadjesari Z, Stevenson F, Godfrey C, Murray E. Negotiating the ‘grey area between normal social drinking and being a smelly tramp': a qualitative study of people searching for help online to reduce their drinking. Health Expect. (2015) 18:2011–20. doi: 10.1111/hex.12351
7. Probst C, Manthey J, Martinez A, Rehm J. Alcohol use disorder severity and reported reasons not to seek treatment: a cross-sectional study in European primary care practices. Subst Abus Treat Prev policy. (2015) 10:32. doi: 10.1186/s13011-015-0028-z
8. Andréasson S, Danielsson AK, Wallhed-Finn S. Preferences regarding treatment for alcohol problems. Alcohol Alcohol. (2013) 48:694–9. doi: 10.1093/alcalc/agt067
9. Keyes KM, Hatzenbuehler ML, McLaughlin KA, Link B, Olfson M, Grant BF, et al. Stigma and treatment for alcohol disorders in the United States. Am J Epidemiol. (2010) 172:1364–72. doi: 10.1093/aje/kwq304
10. Wallhed Finn S, Bakshi AS, Andréasson S. Alcohol consumption, dependence, and treatment barriers: perceptions among nontreatment seekers with alcohol dependence. Subst Use Misuse. (2014) 49:762–9. doi: 10.3109/10826084.2014.891616
11. Saunders SM, Zygowicz KM, D'Angelo BR. Person-related and treatment-related barriers to alcohol treatment. J Subst Abuse Treat. (2006) 30:261–70. doi: 10.1016/j.jsat.2006.01.003
12. Sandberg S. Is cannabis use normalized, celebrated or neutralized? Analysing talk as action. Addict Res Theory. (2012) 20:372–81. doi: 10.3109/16066359.2011.638147
13. Dahl SL. Remaining a user while cutting down: the relationship between cannabis use and identity. Drugs Educ Prev Policy. (2015) 22:175–84. doi: 10.3109/09687637.2014.920765
14. Kerridge BT, Mauro PM, Chou SP, Saha TD, Pickering RP, Fan AZ, et al. Predictors of treatment utilization and barriers to treatment utilization among individuals with lifetime cannabis use disorder in the United States. Drug Alcohol Depend. (2017) 181:223–8. doi: 10.1016/j.drugalcdep.2017.09.032
15. Van Der Pol P, Liebregts N, de Graaf R, Korf DJ, van den Brink W, van Laar M. Facilitators and barriers in treatment seeking for cannabis dependence. Drug Alcohol Depend. (2013) 133:776–80. doi: 10.1016/j.drugalcdep.2013.08.011
16. Riper H, Hoogendoorn A, Cuijpers P, Karyotaki E, Boumparis N, Mira A, et al. Effectiveness and treatment moderators of internet interventions for adult problem drinking: an individual patient data meta-analysis of 19 randomised controlled trials. PLOS Med. (2020) 15:e1002714. doi: 10.1371/journal.pmed.1002714
17. Ekström V, Johansson M, Johansson M. Choosing internet-based treatment for problematic alcohol use - Why, when and how? Users' experiences of treatment online. Addict Sci Clin Pract. (2020) 15:22. doi: 10.1186/s13722-020-00196-5
18. Hoch E, Preuss UW, Ferri M, Simon R. Digital interventions for problematic cannabis users in non-clinical settings: findings from a systematic review and meta-analysis. Eur Addict Res. (2016) 22:233–42. doi: 10.1159/000445716
19. Olmos A, Tirado-Muñoz J, Farré M, Torrens M. The efficacy of computerized interventions to reduce cannabis use: a systematic review and meta-analysis. Addict Behav. (2018) 79:52–60. doi: 10.1016/j.addbeh.2017.11.045
20. Eagly AH, Chaiken S. The Psychology of Attitudes. Orlando, FL: Harcourt Brace Jovanovich College Publishers (1993). xxii, 794–xxii, 794.
21. Ajzen I. From intentions to actions: a theory of planned behavior. Action Control Springer Berlin Heidelberg. (2020) 1985:11–39. doi: 10.1007/978-3-642-69746-3_2
22. Sheeran P, Maki A, Montanaro E, Avishai-Yitshak A, Bryan A, Klein WMP, et al. The impact of changing attitudes, norms, and self-efficacy on health-related intentions and behavior: a meta-analysis. In: Paschal S. Health Psychology. Chapel Hill, NC: Department of Psychology and Neuroscience, University of North Carolina; American Psychological Association (2016). p. 1178–88.
23. Davis FD. A technology acceptance model for empirically testing new end-user information systems: theory and results. Management. (Ph.D.) (1985). p. 291.
24. Andersson G. Internet-delivered psychological treatments. Annu Rev Clin Psychol. (2016) 12:157–79. doi: 10.1146/annurev-clinpsy-021815-093006
25. Apolinário-Hagen J, Harrer M, Kählke F, Fritsche L, Salewski C, Ebert DD. Public attitudes toward guided internet-based therapies: web-based survey study. J Med Internet Res. (2018) 20:e10735. doi: 10.2196/preprints.10735
26. March S, Day J, Ritchie G, Rowe A, Gough J, Hall T, et al. Attitudes toward e-mental health services in a community sample of adults: online survey. J Med Internet Res. (2018) 20:e9109. doi: 10.2196/jmir.9109
27. Topooco N, Riper H, Araya R, Berking M, Brunn M, Chevreul K, et al. Attitudes towards digital treatment for depression: a European stakeholder survey. Internet Interv. (2017) 8:1–9. doi: 10.1016/j.invent.2017.01.001
28. Vigerland S, Ljótsson B, Bergdahl Gustafsson F, Hagert S, Thulin U, Andersson G, et al. Attitudes towards the use of computerized cognitive behavior therapy (cCBT) with children and adolescents: a survey among Swedish mental health professionals. Internet Interv. (2014) 1:111–7. doi: 10.1016/j.invent.2014.06.002
29. Schröder J, Berger T, Meyer B, Lutz W, Hautzinger M, Späth C, et al. Attitudes towards internet interventions among psychotherapists and individuals with mild to moderate depression symptoms. Cognit Ther Res. (2017) 41:745–56. doi: 10.1007/s10608-017-9850-0
30. Kivi M, Eriksson MCM, Hange D, Petersson EL, Björkelund C, Johansson B. Experiences and attitudes of primary care therapists in the implementation and use of internet-based treatment in Swedish primary care settings. Internet Interv. (2015) 2:248–56. doi: 10.1016/j.invent.2015.06.001
31. Mohr DC, Siddique J, Ho J, Duffecy J, Jin L, Fokuo JK. Interest in behavioral and psychological treatments delivered face-to-face, by telephone, and by internet. Ann Behav Med. (2010) 40:89–98. doi: 10.1007/s12160-010-9203-7
32. Hennemann S, Beutel ME, Zwerenz R. Drivers and barriers to acceptance of web-based aftercare of patients in inpatient routine care: a cross-sectional survey. J Med Internet Res. (2016) 18:e337. doi: 10.2196/jmir.6003
33. Schröder J, Jelinek L, Moritz S. A randomized controlled trial of a transdiagnostic Internet intervention for individuals with panic and phobias - One size fits all. J Behav Ther Exp Psychiatry. (2017) 54:17–24. doi: 10.1016/j.jbtep.2016.05.002
34. Schröder J, Berger T, Meyer B, Lutz W, Späth C, Michel P, et al. Impact and change of attitudes toward Internet interventions within a randomized controlled trial on individuals with depression symptoms. Depress Anxiety. (2018) (2020) 35:421–30. doi: 10.1002/da.22727
35. Lutz W, Arndt A, Rubel J, Berger T, Schröder J, Späth C, et al. Defining and predicting patterns of early response in a web-based intervention for depression. J Med Internet Res. (2017) 19:e206. doi: 10.2196/jmir.7367
36. Ajzen I. Nature and operation of attitudes. Annu Rev Psychol. (2001) 52:27–58. doi: 10.1146/annurev.psych.52.1.27
37. Muthén BO, Muthén LK. Integrating person-centered and variable-centered analyses: growth mixture modeling with latent trajectory classes. Alcohol Clin Exp Res. (2000) 24:882–91. doi: 10.1111/j.1530-0277.2000.tb02070.x
38. Johansson M, Berman AH, Sinadinovic K, Lindner P, Hermansson U, Andreasson S. The effects of internet-based cognitive behavioral therapy for harmful alcohol use and alcohol dependence as self-help or with therapist-guidance: a three-armed randomized trial. J Med Internet Res. (accepted). doi: 10.2196/29666
39. Sinadinovic K, Johansson M, Johansson AS, Lundqvist T, Lindner P, Hermansson U. Guided web-based treatment program for reducing cannabis use: a randomized controlled trial. Addict Sci Clin Pract. (2020) 15:1–13. doi: 10.1186/s13722-020-00185-8
40. McNeish D. Thanks coefficient alpha, We'll take it from here. Psychol Methods. (2018) 23:412–33. doi: 10.1037/met0000144
41. Bertholet N, Cheng DM, Palfai TP, Samet JH, Saitz R. Does readiness to change predict subsequent alcohol consumption in medical inpatients with unhealthy alcohol use? Addict Behav. (2009) 34:636–40. doi: 10.1016/j.addbeh.2009.03.034
42. Sobell LC, Sobell MB. Timeline follow-back. In: Measuring Alcohol Consumption. Totowa, NJ: Humana Press (1992). p. 41–72.
43. Bergman H, Källmén H. Alcohol use among Swedes and a psychometric evaluation of the alcohol use disorders identification test. Alcohol Alcohol. (2002) (2021) 37:245–51. doi: 10.1093/alcalc/37.3.245
44. Legleye S, Karila L, Beck F, Reynaud M. Validation of the CAST, a general population cannabis abuse screening Test. J Subst Use. (2007) 12:233–42. doi: 10.1080/14659890701476532
45. American Psychiatric Association. Diagnostic and Statistical Manual of Mental Disorders (DSM-5®). Washington: American Psychiatric Publishing (2013).
46. R Core Team. R: A Language and Environment for Statistical Computing. Vienna (2021). Available online at: https://www.r-project.org/
47. MacQueen J. Some methods for classification and analysis of multivariate observations. In: Le Cam LM, Neyman J, editors. Proceedings of the fifth Berkeley Symposium on Mathematical Statistics and Probability, Volume 1: Statistics. Los Angeles, CA: University of California Press (1967). p. 281–97.
48. Hartigan JA, Wong MA. Algorithm AS 136: a K-means clustering algorithm. Appl Stat. (1979) 28:100. doi: 10.2307/2346830
49. Aggarwal CC, Hinneburg A, Keim DA. On the surprising behavior of distance metrics in high dimensional space. In: Lecture Notes in Computer Science (including subseries Lecture Notes in Artificial Intelligence and Lecture Notes in Bioinformatics). Springer Verlag (2001). p. 420–34.
50. Steinley D. K-means clustering: a half-century synthesis. Br J Math Stat Psychol. (2006) 59:1–34. doi: 10.1348/000711005X48266
51. Charrad M, Ghazzali N, Boiteau V, Niknafs A. NbClust : an R Package for Determining the. J Stat Softw. (2014) 61:1–36. doi: 10.18637/jss.v061.i06
52. Deen M, de Rooij M. ClusterBootstrap: an R package for the analysis of hierarchical data using generalized linear models with the cluster bootstrap. Behav Res Methods. (2020) (2020) 52:572–90. doi: 10.3758/s13428-019-01252-y
53. Wood J, Freemantle N, King M, Nazareth I. Trap of trends to statistical significance: likelihood of near significant P value becoming more significant with extra data. BMJ. (2014) 348:1–6. doi: 10.1136/bmj.g2215
54. Roper L, McGuire J, Salmon P, Booth PG. Treatment-seeking for alcohol problems: the influence of mirroring events and windows of opportunity. Addict Res Theory. (2013) 21:479–88. doi: 10.3109/16066359.2012.746318
55. Årstad J, Nesvåg SM, Njå ALM, Biong SN. How enough becomes enough: processes of change prior to treatment for substance use disorder. J Subst Use. (2018) 23:429–35. doi: 10.1080/14659891.2018.1436608
56. Orford J, Kerr C, Copello A, Hodgson R, Alwyn T, Black R, et al. Why people enter treatment for alcohol problems: findings from UK alcohol treatment trial pre-treatment interviews. J Subst Use. (2006) 11:161–76. doi: 10.1080/14659890500246540
57. Arrindell W. Changes in waiting-list patients over time: data on some commonly-used measures. Beware! Behav Res Ther. (2001) 39:1227–47. doi: 10.1016/S0005-7967(00)00104-2
58. Miles LM, Rodrigues AM, Sniehotta FF, French DP. Asking questions changes health-related behavior: an updated systematic review and meta-analysis. J Clin Epidemiol Elsevier USA. (2021) 123:59–68. doi: 10.1016/j.jclinepi.2020.03.014
59. Clifford PR, Maisto SA, Davis CM. Alcohol treatment research assessment exposure subject reactivity effects: Part I. Alcohol use and related consequences. J Stud Alcohol Drugs. (2007) 68:519–28. doi: 10.15288/jsad.2007.68.519
Keywords: attitudes, internet interventions, latent subgroups, K-means (KM) clustering, alcohol, cannabis
Citation: Romero D, Johansson M, Hermansson U and Lindner P (2021) Impact of Users' Attitudes Toward Anonymous Internet Interventions for Cannabis vs. Alcohol Use: A Secondary Analysis of Data From Two Clinical Trials. Front. Psychiatry 12:730153. doi: 10.3389/fpsyt.2021.730153
Received: 24 June 2021; Accepted: 27 August 2021;
Published: 27 September 2021.
Edited by:
Máté Kapitány-Fövény, Semmelweis University, HungaryReviewed by:
Mauro Ceccanti, Sapienza University of Rome, ItalyGeorgios Demetrios Kotzalidis, Sapienza University of Rome, Italy
Copyright © 2021 Romero, Johansson, Hermansson and Lindner. This is an open-access article distributed under the terms of the Creative Commons Attribution License (CC BY). The use, distribution or reproduction in other forums is permitted, provided the original author(s) and the copyright owner(s) are credited and that the original publication in this journal is cited, in accordance with accepted academic practice. No use, distribution or reproduction is permitted which does not comply with these terms.
*Correspondence: Danilo Romero, danilo.romero@ki.se