- 1Institute of Cognitive and Clinical Neuroscience, Central Institute of Mental Health, Medical Faculty Mannheim, Heidelberg University, Mannheim, Germany
- 2Department of Psychiatry and Psychotherapy, University of Regensburg, Regensburg, Germany
Representational similarity analysis (RSA) is a popular multivariate analysis technique in cognitive neuroscience that uses functional neuroimaging to investigate the informational content encoded in brain activity. As RSA is increasingly being used to investigate more clinically-geared questions, the focus of such translational studies turns toward the importance of individual differences and their optimization within the experimental design. In this perspective, we focus on two design aspects: applying individual vs. averaged behavioral dissimilarity matrices to multiple participants' neuroimaging data and ensuring the congruency between tasks when measuring behavioral and neural representational spaces. Incorporating these methods permits the detection of individual differences in representational spaces and yields a better-defined transfer of information from representational spaces onto multivoxel patterns. Such design adaptations are prerequisites for optimal translation of RSA to the field of precision psychiatry.
Introduction
Over the past decade, the multivariate method of Representational Similarity Analysis (RSA) (1) has become a popular means of investigating the informational content represented within patterns of activity in the brain. This technique allows researchers to test hypotheses regarding the relative high-dimensional structure of information, represented by multivariate activity patterns, in different regions of the brain. Since its development, RSA has been used with functional neuroimaging to explore topics such as object categorization (2), semantics (3), object recognition (4), affect (5), action observation (6), and fear-conditioning (7). Studies employing such multivariate analyses have provided the field with an improved understanding of how information is encoded in local activity patterns and how such high-dimensional information can be altered through processes such as attention (8) or aversive-learning (9–11). Recently, researchers have begun translating these methods to psychiatry in an attempt to explore differences in representational spaces between individuals with and without disorders (12–16).
While the discipline of cognitive neuroscience has tended to use functional magnetic resonance imaging (fMRI) to explore group-level effects in mapping functions to brain structures, there has been a recent push to investigate individual differences using fMRI (17–19) and to incorporate advanced analysis methods into precision psychiatry (20, 21). As such, individualizing neuroimaging analyses is a critical step for studying individual differences in human behavior, which can be facilitated by (a) preserving a given individual's behavioral data prior to carrying out RSA and (b) increasing the congruency between the conditions under which the behavioral and neuroimaging data are acquired. Below we provide a brief overview of RSA, describe these two strategies in further detail, and discuss aspects of the experimental design that researchers and clinicians can implement in (translational) work that focuses on individual differences.
Representational Similarity Analysis: An Overview
Representational similarity analysis falls within the framework of multivariate pattern analysis (22–24), which investigates the activity from many distributed neurons as a function of mental representations. Rather than using the average activity of such neurons (i.e., the classic univariate approach) as the proxy for mental representations, multivariate analyses take advantage of the pattern of activity across voxels, thereby providing a more informative approach to exploring and making inferences about representational spaces [i.e., abstract spaces of mental representations within the cognitive architecture whose structures are defined by some feature(s)]. Extending this logic, RSA specifically operationalizes dissimilarities in representational spaces as the dissimilarities between evoked multi-voxel patterns and then compares this dissimilarity structure (i.e., the set of neural dissimilarities in question) to a behavioral or theoretical dissimilarity structure. As this approach depends on relative differences between voxels (rather than differences within voxels), RSA provides researchers with a richer means of uncovering higher-dimensional information within the structure of mental representations.
To carry out RSA with fMRI, researchers design a task-based study, in which stimuli are usually presented to participants in a trial-by-trial manner. During data analysis, one generally performs the classic mass-univariate analysis of fitting stimulus-specific models of the hemodynamic response to time course data evoked by those stimuli using the general linear model, and the resulting beta-weights (or t-scores) serve as the input for the multivariate analysis. One of the most common uses of RSA involves employing models that describe the relationship between stimuli, according to some organizing principle, and observing the extent to which a given model explains differences between the observed stimulus-evoked multivariate patterns in the brain.
For example, if presenting participants with 10 pictures (five pleasant pictures and five unpleasant pictures), a researcher could develop a model of emotional valence in which the five pleasant pictures are considered similar to each other, the five unpleasant pictures are considered similar to each other, and the pleasant and unpleasant pictures are considered dissimilar to one other. This hypothesis can be visualized as a representational dissimilarity matrix (RDM), which is the relative dissimilarity structure reflecting the relationship between all pairs of stimuli under investigation (Figure 1A). An alternate model using the same images could see the stimuli organized according to their animacy. If some of the pleasant and unpleasant pictures contain people in them, these stimuli could be considered similar, and perhaps the remaining stimuli contain only inanimate objects, which could also be considered similar. The animate and inanimate stimuli would then be considered dissimilar to one another (Figure 1B). Pitting these two model RDMs against each other allows a researcher to determine whether the activity patterns in some brain region are better explained by the “valence” model and whether the activity patterns in some other brain region are better explained by the “animacy” model. The pairwise relationships between stimuli at the neural level are generally operationalized by considering their evoked multivoxel patterns (e.g., within a local neighborhood, a given brain region, or a set of brain regions) and computing a distance measure, such as Euclidean or correlation distance (25), between them. It is this neural RDM that the model RDMs seek to explain. Comparing the explanatory power of each model (or a combination thereof) with that of the other models allows researchers to determine which of the tested models best explains the representational space underlying the activity patterns and, consequently, interpret information processing at some level of the cognitive architecture.
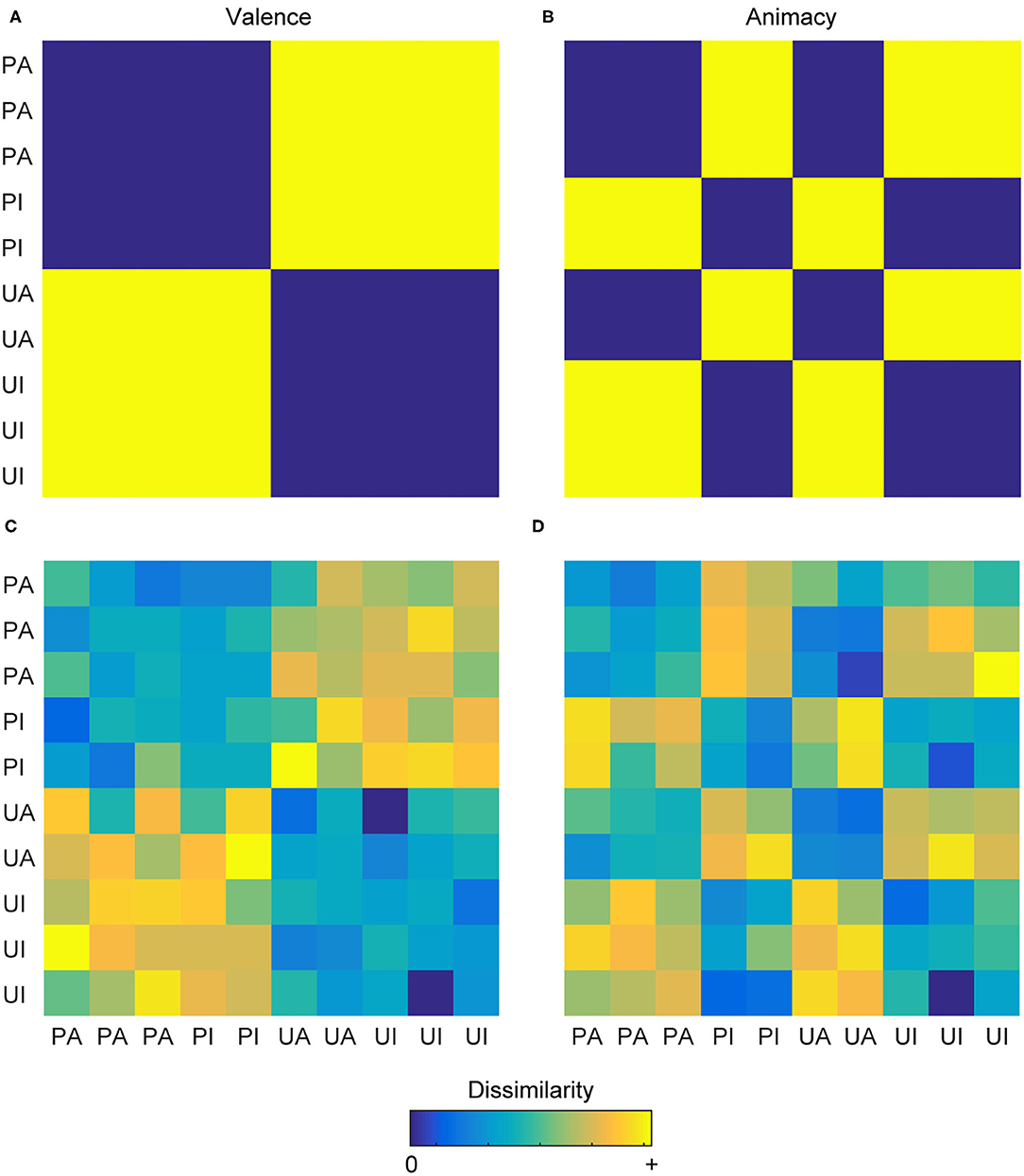
Figure 1. Representational dissimilarity matrices. (A) A theoretical RDM depicting the binary relationship between pairs of images classified as pleasant-animate (PA), pleasant-inanimate (PI), unpleasant-animate (UA), and unpleasant-inanimate (UI) according to their valence. (B) A theoretical RDM depicting the binary relationship between these same images according to their animacy. These two models are effectively uncorrelated (r = −0.08) and can be used to explore the hypotheses of whether a particular brain region encodes valence information or animacy information. Cool colors depict decreasing dissimilarity, and warm colors depict increasing dissimilarity. (C,D) The same conventions as the upper row but depicted here are two RDMs that might result from a participant having carried out the multi-arrangement method, yielding a similar information structure as the theoretical RDMs but additionally containing graded, individualized information.
Recently, RDMs have been generated through behavioral experiments, for example, by combining the multi-arrangement method (26) with inverse multidimensional scaling (27), rather than directly hypothesized by the researcher. During the multi-arrangement method, participants drag-and-drop stimuli into a 2D arena on a computer screen, in which the distances between stimuli reflect the participants' dissimilarity judgments of the stimuli, according to some organizing principle. Inverse-multidimensional scaling then estimates the RDM from the 2D inter-stimulus distances (Figures 1C,D). For additional details, see (27). These methods provide the benefit of yielding high-dimensional representational spaces that contain nuanced, idiosyncratic, and continuous information and have been used to generate behavioral RDMs in combination with RSA to investigate domains such as similarity of body parts (28), emotions (29), tool-related features (30), action observation (31), and face perception (32). A variety of tutorials, reviews, and methodological guides on RSA have been compiled over the past years for researchers seeking more extensive explanations of these concepts and current practices (25, 33–38).
Among these various domains, one of the manners in which RSA has proven to be particularly powerful is that it can yield comparable RDMs across participants, even when the evoked patterns themselves are not comparable [i.e., a second-order isomorphism (38)], thereby revealing a similar underlying representational structure across different individuals. On the other hand, also the differences between individuals' RDMs may be indicative of meaningful individual differences when interpretable through an additional variable (e.g., personality traits). As such, similar brain regions among participants can be investigated in terms of the individual differences in their underlying representational spaces. In the next sections we expand further on these points with respect to methodological strategies.
Averaging vs. Preserving Individual RDMs
One approach to RSA involves researchers averaging behavioral RDMs from many participants and then comparing this averaged behavioral RDM to distinct individuals' neural RDMs. This approach yields one behavioral RDM per condition for all participants in the study, which fits the central paradigm of general psychology to test for commonalities among samples: i.e., that the average representation of one sample is equivalent to the average representation of another sample, as long as both samples originate from the same population. As such, researchers have often used one sample to generate the behavioral RDMs and a different sample to obtain the neuroimaging data. As generally valid (and in some cases necessary) as this method is, such an approach is not sensitive to individual-level information, the importance of which has been frequently addressed (17, 39–42), especially with respect to translational neuroscience (21, 43, 44).
On the other hand, recent studies have demonstrated that individualized RDMs can reveal meaningful individual-level information in cortical activity patterns (29, 45–47). Levine et al. explicitly compared the results between individualized emotion RDMs and a sample-averaged emotion RDM (29). This analysis yielded an interaction: effects based on the individualized RDMs were present in the insula but not in ventral temporal cortex, while the effects based on the averaged RDM were present in ventral temporal cortex but not in the insula. Such results suggest that the insula may be a common location for individuals' distinct emotion information: a finding that one cannot reveal when using a single RDM based on averaged behavioral data. This approach provides researchers the ability to interpret between-subject differences of neural RDMs [i.e., in the absence of second-order isomorphisms (38)] as meaningful individual differences in the underlying mental representations, which nevertheless share a common neural substrate (in this case, the insula).
Moreover, recent studies have shown that individuals' behavioral representational spaces correlate with other individualized factors [e.g., facial categorization with distractibility (46), semantics with fluid and crystallized intelligence (48), and affect with personality factors (49)]. Given these findings, it is likely that critical information is removed when averaging individuals' RDMs. Instead, by retaining such individualized information, these methods will benefit translational neuroscientists and clinicians in developing imaging-based biomarkers that address the cognitive level of mental disorders.
Task-Congruency When Acquiring Behavioral and Neural Data
Behavioral similarity of concepts is task dependent. Recent work has seen participants perform the multi-arrangement task following different organizing principles; consequently, the underlying representational spaces differed as a function of the organizing principle that participants followed (29, 31, 50, 51). Such findings are in line with prior neuroimaging studies that have shown prominent changes in the neural representational space when participants engaged differently with the stimuli. For example, compared to a distractor task, a color-naming task increased the categorical nature of the representational space of colors underlying visual areas (52). Relatedly, having participants attend to either the behavior or the taxonomy of animals depicted in video clips shifted the representational space to emphasize the attended feature (8). Given these findings, having participants not attend to the features-of-interest (with which the behavioral RDMs were generated), for example via passive-viewing or distractor tasks, likely results in decreased sensitivity, as the neural RDMs contain a different underlying structure from the behavioral RDMs. Thus, such approaches uncover some form of automatic processing of the stimuli that is the least common denominator between the neural data and the behavioral data.
Additionally, one recent and intriguing finding came to light when different groups of participants were tasked with arranging a set of images according to their background, color, function, or shape (51), while a fifth group was instructed to freely arrange the images on the computer screen. The resulting averaged behavioral RDM from the “free-arrangement” sample resembled the behavioral RDM from the sample that arranged the images according to the similarity of their functions. This finding raises the question of what “default” information underlies representations in the absence of specific task demands, and how not controlling for this default processing may lead to neural representations that contain (possibly fluctuating) subjective biases toward the stimuli (53), thereby reducing the statistical power of the RSA. This issue is especially pronounced given that individuals have different attentional biases (54–58) and fluctuate between different mental states in an individualized manner (59–62). As such, during passive-viewing or unrelated tasks, the computational goal of the neural transfer function may not be sufficiently specified, resulting in the underlying structure of information present in the behavioral representational spaces differing from that in the neural representational spaces.
This approach still yields interesting group-level information regarding automatic processing or common attentional biases (and maintains a level of methodological simplicity by only needing to carry out one MR scan to which multiple distinct behavioral RDMs can be applied). However, since the nature of these automatic processes is undetermined, future studies that seek to investigate differential aspects of cognitive processes should consider controlling for mental operations during the scanning session. Addressing this issue will be especially pertinent to researchers and clinicians interested in applying such methods to patient populations, given the attentional and state-dependent biases of psychopathology (63–68).
Possible Adaptations
Consider Multiple Sessions Per Participant
One of the most straightforward ways to avoid needing to use averaged behavioral RDMs involves planning an experiment that includes RSA and the multi-arrangement method to consist of (at least) two sessions (i.e., one behavioral and one neuroimaging) per subject (29, 45). This way, every subject would produce at least one individualized behavioral RDM, allowing for an individuals' own behavioral data to be applied to their own neural data, thereby taking advantage of the individual-level information.
An additional consideration is that it is certainly possible that some representational spaces are more homogeneous across participants than others (e.g., perceived shape of a stimulus vs. emotion evoked by a stimulus). Relatedly, Hebart et al. recently revealed that similarity judgments between objects can be described by 49 dimensions (69). As such, depending on the dimension under investigation, using individualized RDMs may provide only minor benefits, possibly rendering the increased complexity of the experimental design unwarranted. Nevertheless, even slight differences in seemingly homogeneous representational spaces may be meaningful in particular research domains (e.g., color perception in synesthesia).
Engage Participants With a Feature-Related Task
For the purposes of individual differences, addressing task-incongruency can involve having participants performing a task in the scanner that relates to the stimulus feature that also drove the organization of the stimuli during the behavioral session (or the theoretically motivated RDM). A step in this direction is seen in two recent studies that combined behavioral RDMs from the multi-arrangement method with fMRI: Bracci et al. asked participants to indicate whether a stimulus looked like (i.e., appearance condition) or depicted (i.e., animacy condition) an animal (70), and Tucciarelli et al. asked participants to indicate when they observed the same action on two consecutive trials (31).
Along these lines, the goal is to at least turn the participants' attention toward the features that underlie their related behavioral representational spaces. For example, if during the behavioral task participants organized pictures based on how the images made them feel, a simple task in the scanner could involve having participants press a button with their index finger if they like the picture presented to them and press a button with their middle finger if they dislike it (of course counterbalancing the stimulus-response mapping across experimental runs). A color-based task could ask participants to indicate if the colors in the stimulus tend to be brighter or darker; likewise a function-based task could ask participants to indicate if they use the object depicted in the stimulus on a regular basis. Although these tasks yield a binary outcome (while the behavioral data are continuous), participants are nevertheless turning their attention toward the same aspects of the stimuli as during the behavioral task, thereby ensuring an increased similarity between the computational goal of the neural transfer function of different participants, such that observed differences between participants' neural and behavioral RDMs should reflect related differences between their mental representations.
The downside to this approach is that scanning sessions would necessarily require more time, as one would need multiple in-scanner tasks to account for each behavioral model generated by the participants. However, this drawback may be warranted, if it results in higher sensitivity and accuracy of the analysis, which could improve the detection of individual-level information or permit subtyping of participants.
Concluding Remarks
As representational similarity analysis is increasingly being used to investigate questions in cognitive and clinical neuroscience, it is necessary to address a few ways in which this method can be optimized for investigating both individual differences and commonalities in the context of individual differences. While the current approaches have revealed many interesting findings from a general or group-level perspective, we hold that taking advantage of individualized behavioral RDMs and controlling for task-related idiosyncrasies will ultimately allow for more informative neuroimaging investigations of differences between individuals' mental representations. These two methods work together toward this goal in that individualized behavioral RDMs permit the discovery of between-subject differences in mental representations, while task-congruency ensures that said differences can be interpreted within the context of the computational goal of individuals' neural transfer functions. Such an approach will allow researchers to test a variety of hypotheses that pertain to mental health disorders. For example, one could investigate whether individuals with emotion dysregulation have altered emotion spaces that are specific to particular brain regions, and, moreover, whether idiosyncrasies in these neural emotion spaces can assist in differential diagnosis of psychopathology, especially given the transdiagnostic nature of emotion dysregulation (71). Additionally, one could examine whether individual differences in behavioral and neural similarity of trigger stimuli are prognostic of treatment success in patients with anxiety disorders. As such, we find these methodological changes to be essential for optimal translation of task-based functional neuroimaging into fields where individual-level information is crucial, such as precision psychiatry.
Data Availability Statement
The original contributions presented in the study are included in the article/supplementary material; further inquiries can be directed to the corresponding authors.
Author Contributions
SL drafted the manuscript. Both authors devised the argumentation, revised, and approved the final version of the manuscript.
Conflict of Interest
The authors declare that the research was conducted in the absence of any commercial or financial relationships that could be construed as a potential conflict of interest.
Publisher's Note
All claims expressed in this article are solely those of the authors and do not necessarily represent those of their affiliated organizations, or those of the publisher, the editors and the reviewers. Any product that may be evaluated in this article, or claim that may be made by its manufacturer, is not guaranteed or endorsed by the publisher.
Acknowledgments
The authors would like to thank Angelika Lingnau for comments on an earlier version of this manuscript.
References
1. Kriegeskorte N, Mur M, Bandettini P. Representational similarity analysis - connecting the branches of systems neuroscience. Front Syst Neurosci. (2008) 2:1–28. doi: 10.3389/neuro.06.004.2008
2. Kriegeskorte N, Mur M, Ruff DA, Kiani R, Bodurka J, Esteky H, et al. Matching categorical object representations in inferior temporal cortex of man and monkey. Neuron. (2008) 60:1126–41. doi: 10.1016/j.neuron.2008.10.043
3. Devereux BJ, Clarke A, Marouchos A, Tyler LK. Representational similarity analysis reveals commonalities and differences in the semantic processing of words and objects. J Neurosci. (2013) 33:18906–16. doi: 10.1523/JNEUROSCI.3809-13.2013
4. Cichy RM, Pantazis D, Oliva A. Resolving human object recognition in space and time. Nat Neurosci. (2014) 17:455–62. doi: 10.1038/nn.3635
5. Skerry AE, Saxe R. Neural representations of emotion are organized around abstract event features. Curr Biol. (2015) 25:1945–54. doi: 10.1016/j.cub.2015.06.009
6. Wurm MF, Caramazza A, Lingnau A. Action categories in lateral occipitotemporal cortex are organized along sociality and transitivity. J Neurosci. (2017) 37:562–75. doi: 10.1523/JNEUROSCI.1717-16.2017
7. Levine SM, Kumpf M, Rupprecht R, Schwarzbach J V. Supracategorical fear information revealed by aversively conditioning multiple categories. Cogn Neurosci. (2021) 12:28–39. doi: 10.1080/17588928.2020.1839039
8. Nastase SA, Connolly AC, Oosterhof NN, Halchenko YO, Guntupalli JS, Visconti di Oleggio Castello M, et al. Attention selectively reshapes the geometry of distributed semantic representation. Cereb Cortex. (2017) 27:4277–91. doi: 10.1093/cercor/bhx138
9. Visser RM, Scholte HS, Kindt M. Associative learning increases trial-by-trial similarity of BOLD-MRI patterns. J Neurosci. (2011) 31:12021–8. doi: 10.1523/JNEUROSCI.2178-11.2011
10. Dunsmoor JE, Kragel PA, Martin A, La Bar KS. Aversive learning modulates cortical representations of object categories. Cereb Cortex. (2014) 24:2859–72. doi: 10.1093/cercor/bht138
11. Levine SM, Pfaller M, Reichenberger J, Shiban Y, Mühlberger A, Rupprecht R, et al. Relating experimentally-induced fear to pre-existing phobic fear in the human brain. Soc Cogn Affect Neurosci. (2018) 13:164–72. doi: 10.1093/scan/nsx147
12. Zhu J, Lowen SB, Anderson CM, Ohashi K, Khan A, Teicher MH. Association of prepubertal and postpubertal exposure to childhood maltreatment with adult amygdala function. JAMA Psychiatry. (2019) 76:843. doi: 10.1001/jamapsychiatry.2019.0931
13. Hennings AC, McClay M, Lewis-Peacock JA, Dunsmoor JE. Contextual reinstatement promotes extinction generalization in healthy adults but not PTSD. Neuropsychologia. (2020) 147:107573. doi: 10.1016/j.neuropsychologia.2020.107573
14. O'Hearn K, Larsen B, Fedor J, Luna B, Lynn A. Representational similarity analysis reveals atypical age-related changes in brain regions supporting face and car recognition in autism. Neuroimage. (2020) 209:116322. doi: 10.1016/j.neuroimage.2019.116322
15. Morey RA, Haswell CC, Stjepanović D, Dunsmoor JE, LaBar KS. Neural correlates of conceptual-level fear generalization in posttraumatic stress disorder. Neuropsychopharmacology. (2020) 45:1380–9. doi: 10.1038/s41386-020-0661-8
16. Richardson H, Gweon H, Dodell-Feder D, Malloy C, Pelton H, Keil B, et al. Response patterns in the developing social brain are organized by social and emotion features and disrupted in children diagnosed with autism spectrum disorder. Cortex. (2020) 125:12–29. doi: 10.1016/j.cortex.2019.11.021
17. Dubois J, Adolphs R. Building a science of individual differences from fMRI. Trends Cogn Sci. (2016) 20:425–43. doi: 10.1016/j.tics.2016.03.014
18. Foulkes L, Blakemore S-J. Studying individual differences in human adolescent brain development. Nat Neurosci. (2018) 21:315–23. doi: 10.1038/s41593-018-0078-4
19. Hawco C, Dickie EW, Jacobs G, Daskalakis ZJ, Voineskos AN. Moving beyond the mean: subgroups and dimensions of brain activity and cognitive performance across domains. Neuroimage. (2021) 231:117823. doi: 10.1016/j.neuroimage.2021.117823
20. Huys QJM, Maia T V, Frank MJ. Computational psychiatry as a bridge from neuroscience to clinical applications. Nat Neurosci. (2016) 19:404–13. doi: 10.1038/nn.4238
21. Gratton C, Kraus BT, Greene DJ, Gordon EM, Laumann TO, Nelson SM, et al. Defining individual-specific functional neuroanatomy for precision psychiatry. Biol Psychiatry. (2020) 88:28–39. doi: 10.1016/j.biopsych.2019.10.026
22. Haxby JV, Gobbini MI, Furey ML, Ishai A, Schouten JL, Pietrini P. Distributed and overlapping representations of faces and objects in ventral temporal cortex. Science. (2001) 293:2425–30. doi: 10.1126/science.1063736
23. Kamitani Y, Tong F. Decoding the visual and subjective contents of the human brain. Nat Neurosci. (2005) 8:679–85. doi: 10.1038/nn1444
24. Haynes J-D, Rees G. Predicting the orientation of invisible stimuli from activity in human primary visual cortex. Nat Neurosci. (2005) 8:686–91. doi: 10.1038/nn1445
25. Walther A, Nili H, Ejaz N, Alink A, Kriegeskorte N, Diedrichsen J. Reliability of dissimilarity measures for multi-voxel pattern analysis. Neuroimage. (2016) 137:188–200. doi: 10.1016/j.neuroimage.2015.12.012
26. Goldstone R. An efficient method for obtaining similarity data. Behav Res Methods Instr Comput. (1994) 26:381–6. doi: 10.3758/BF03204653
27. Kriegeskorte N, Mur M. Inverse MDS: inferring dissimilarity structure from multiple item arrangements. Front Psychol. (2012) 3:245. doi: 10.3389/fpsyg.2012.00245
28. Bracci S, Caramazza A, Peelen MV. Representational similarity of body parts in human occipitotemporal cortex. J Neurosci. (2015) 35:12977–85. doi: 10.1523/JNEUROSCI.4698-14.2015
29. Levine SM, Wackerle A, Rupprecht R, Schwarzbach JV. The neural representation of an individualized relational affective space. Neuropsychologia. (2018) 120:35–42. doi: 10.1016/j.neuropsychologia.2018.10.008
30. Wang X, Zhuang T, Shen J, Bi Y. Disentangling representations of shape and action components in the tool network. Neuropsychologia. (2018) 117:199–210. doi: 10.1016/j.neuropsychologia.2018.05.026
31. Tucciarelli R, Wurm M, Baccolo E, Lingnau A. The representational space of observed actions. Elife. (2019) 8:e47686. doi: 10.7554/eLife.47686
32. Dobs K, Isik L, Pantazis D, Kanwisher N. How face perception unfolds over time. Nat Commun. (2019) 10:1258. doi: 10.1038/s41467-019-09239-1
33. Popal H, Wang Y, Olson IR. A guide to representational similarity analysis for social neuroscience. Soc Cogn Affect Neurosci. (2019) 14:1243–53. doi: 10.1093/scan/nsz099
34. Dimsdale-Zucker HR, Ranganath C. Representational similarity analyses: a practical guide for functional MRI applications. In: Manahan-Vaughan D, editor. Handbook of Behavioral Neuroscience. Amsterdam: Elsevier BV (2018). p. 509–25.
35. Haynes JD. A primer on pattern-based approaches to fMRI: principles, pitfalls, and perspectives. Neuron. (2015) 87:257–70. doi: 10.1016/j.neuron.2015.05.025
36. Nili H, Wingfield C, Walther A, Su L, Marslen-Wilson W, Kriegeskorte N. A toolbox for representational similarity analysis. PLoS Comput Biol. (2014). 10:e1003553. doi: 10.1371/journal.pcbi.1003553
37. Kriegeskorte N, Kievit RA. Representational geometry: integrating cognition, computation, and the brain. Trends Cogn Sci. (2013) 17:401–12. doi: 10.1016/j.tics.2013.06.007
38. Weaverdyck ME, Lieberman MD, Parkinson C. Tools of the trade multivoxel pattern analysis in fMRI: a practical introduction for social and affective neuroscientists. Soc Cogn Affect Neurosci. (2020) 15:487–509. doi: 10.1093/scan/nsaa057
39. de Haas B, Iakovidis AL, Schwarzkopf DS, Gegenfurtner KR. Individual differences in visual salience vary along semantic dimensions. Proc Natl Acad Sci. (2019) 16:11687–92. doi: 10.1073/pnas.1820553116
40. Gordon EM, Laumann TO, Gilmore AW, Newbold DJ, Greene DJ, Berg JJ, et al. Precision functional mapping of individual human brains. Neuron. (2017) 95:791–807.e7. doi: 10.1016/j.neuron.2017.07.011
41. Charest I, Kriegeskorte N. The brain of the beholder: honouring individual representational idiosyncrasies. Lang Cogn Neurosci. (2015) 30:367–79. doi: 10.1080/23273798.2014.1002505
42. Miller MB, Donovan C-L, Bennett CM, Aminoff EM, Mayer RE. Individual differences in cognitive style and strategy predict similarities in the patterns of brain activity between individuals. Neuroimage. (2012) 59:83–93. doi: 10.1016/j.neuroimage.2011.05.060
43. Matthews PM, Honey GD, Bullmore ET. Applications of fMRI in translational medicine and clinical practice. Nat Rev Neurosci. (2006) 7:732–44. doi: 10.1038/nrn1929
44. Drysdale AT, Grosenick L, Downar J, Dunlop K, Mansouri F, Meng Y, et al. Resting-state connectivity biomarkers define neurophysiological subtypes of depression. Nat Med. (2017) 23:28–38. doi: 10.1038/nm.4246
45. Charest I, Kievit RA, Schmitz TW, Deca D, Kriegeskorte N. Unique semantic space in the brain of each beholder predicts perceived similarity. Proc Natl Acad Sci. (2014) 111:14565–70. doi: 10.1073/pnas.1402594111
46. Lee J, Geng JJ. Idiosyncratic patterns of representational similarity in prefrontal cortex predict attentional performance. J Neurosci. (2017) 37:1257–68. doi: 10.1523/JNEUROSCI.1407-16.2016
47. Gagnepain P, Vallée T, Heiden S, Decorde M, Gauvain J-L, Laurent A, et al. Collective memory shapes the organization of individual memories in the medial prefrontal cortex. Nat Hum Behav. (2020) 4:189–200. doi: 10.1038/s41562-019-0779-z
48. Ichien N, Lu H, Holyoak KJ. Individual differences in judging similarity between semantic relations. In: Goel A, Seifert C, Freksa C, editors. Proceedings of the 41st Annual Meeting of the Cognitive Science Society. Austin, TX: Cognitive Science Society (2019). p. 464–70.
49. Levine SM, Alahäivälä ALI, Wechsler TF, Wackerle A, Rupprecht R, Schwarzbach JV. Linking personality traits to individual differences in affective spaces. Front Psychol. (2020) 11:448. doi: 10.3389/fpsyg.2020.00448
50. Bracci S, Op de. Beeck H. Dissociations and associations between shape and category representations in the two visual pathways. J Neurosci. (2016) 36:432–44. doi: 10.1523/JNEUROSCI.2314-15.2016
51. Cichy RM, Kriegeskorte N, Jozwik KM, van den Bosch JJF, Charest I. The spatiotemporal neural dynamics underlying perceived similarity for real-world objects. Neuroimage. (2019) 194:12–24. doi: 10.1016/j.neuroimage.2019.03.031
52. Brouwer GJ, Heeger DJ. Categorical clustering of the neural representation of color. J Neurosci. (2013) 33:15454–65. doi: 10.1523/JNEUROSCI.2472-13.2013
53. Levine SM, Wackerle A. Investigating emotional similarity: a comment on riberto, pobric, and talmi (2019). Brain Topogr. (2020) 33:285–7. doi: 10.1007/s10548-020-00766-5
54. Wingenfeld K, Mensebach C, Driessen M, Bullig R, Hartje W, Beblo T. Attention bias towards personally relevant stimuli: the individual emotional stroop task. Psychol Rep. (2006) 99:781–93. doi: 10.2466/PR0.99.3.781-793
55. Tomer R. Attentional bias as trait: correlations with novelty seeking. Neuropsychologia. (2008) 46:2064–70. doi: 10.1016/j.neuropsychologia.2008.02.005
56. Taylor CT, Bomyea J, Amir N. Malleability of attentional bias for positive emotional information and anxiety vulnerability. Emotion. (2011) 11:127–38. doi: 10.1037/a0021301
57. Iacono V, Ellenbogen MA, Wilson AL, Desormeau P, Nijjar R. Inhibition of personally-relevant angry faces moderates the effect of empathy on interpersonal functioning. PLoS ONE. (2015) 10:e0112990. doi: 10.1371/journal.pone.0112990
58. Lichev V, Sacher J, Ihme K, Rosenberg N, Quirin M, Lepsien J, et al. Automatic emotion processing as a function of trait emotional awareness: an fMRI study. Soc Cogn Affect Neurosci. (2015) 10:680–9. doi: 10.1093/scan/nsu104
59. Mueller S, Wang D, Fox MD, Yeo BTT, Sepulcre J, Sabuncu MR, et al. Individual variability in functional connectivity architecture of the human brain. Neuron. (2013) 77:586–95. doi: 10.1016/j.neuron.2012.12.028
60. Shine JM, Bissett PG, Bell PT, Koyejo O, Balsters JH, Gorgolewski KJ, et al. The dynamics of functional brain networks: integrated network states during cognitive task performance. Neuron. (2016) 92:544–54. doi: 10.1016/j.neuron.2016.09.018
61. Nomi JS, Vij SG, Dajani DR, Steimke R, Damaraju E, Rachakonda S, et al. Chronnectomic patterns and neural flexibility underlie executive function. Neuroimage. (2017) 147:861–71. doi: 10.1016/j.neuroimage.2016.10.026
62. Donnelly-Kehoe P, Saenger VM, Lisofsky N, Kühn S, Kringelbach ML, Schwarzbach J, et al. Reliable local dynamics in the brain across sessions are revealed by whole-brain modeling of resting state activity. Hum Brain Mapp. (2019) 40:2967–80. doi: 10.1002/hbm.24572
63. MacLeod C, Mathews A, Tata P. Attentional bias in emotional disorders. J Abnorm Psychol. (1986) 95:15–20. doi: 10.1037/0021-843X.95.1.15
64. Holtzheimer PE, Mayberg HS. Stuck in a rut: rethinking depression and its treatment. Trends Neurosci. (2011) 34:1–9. doi: 10.1016/j.tins.2010.10.004
65. Swick D, Ashley V. Enhanced attentional bias variability in post-traumatic stress disorder and its relationship to more general impairments in cognitive control. Sci Rep. (2017) 7:14559. doi: 10.1038/s41598-017-15226-7
66. Shah D, Cameron C, Smith D, Jaworska N, Blais C, Fisher D, et al. An electrophysiological investigation of attentional bias in a PTSD population. Psychol Trauma Theory Res Pract Policy. (2018) 10:523–32. doi: 10.1037/tra0000339
67. Brewer J, Cortese A, de Wit H, Denys D, Hanlon CA, Holmes EA, et al. Interventions and implications. In: Kalivas PW, Paulus MP, editors. Intrusive Thinking: From Molecules to Free Will Strüngmann. Cambridge, MA: MIT Press (2020).
68. Price RB, Beltz AM, Woody ML, Cummings L, Gilchrist D, Siegle GJ. Neural connectivity subtypes predict discrete attentional-bias profiles among heterogeneous anxiety patients. Clin Psychol Sci. (2020) 8:491–505. doi: 10.1177/2167702620906149
69. Hebart MN, Zheng CY, Pereira F, Baker CI. Revealing the multidimensional mental representations of natural objects underlying human similarity judgements. Nat Hum Behav. (2020) 4:1173–85. doi: 10.1038/s41562-020-00951-3
70. Bracci S, Ritchie JB, Kalfas I, Op de. Beeck HP. The ventral visual pathway represents animal appearance over animacy, unlike human behavior and deep neural networks. J Neurosci. (2019) 39:6513–25. doi: 10.1523/JNEUROSCI.1714-18.2019
Keywords: fMRI, individual differences, multivariate pattern analysis, precision psychiatry, representational similarity analysis, task-based imaging
Citation: Levine SM and Schwarzbach JV (2021) Individualizing Representational Similarity Analysis. Front. Psychiatry 12:729457. doi: 10.3389/fpsyt.2021.729457
Received: 23 June 2021; Accepted: 10 September 2021;
Published: 11 October 2021.
Edited by:
Stefan Borgwardt, University of Lübeck, GermanyReviewed by:
Amin Zandvakili, Warren Alpert Medical School of Brown University, United StatesRichard Bruce Bolster, University of Winnipeg, Canada
Copyright © 2021 Levine and Schwarzbach. This is an open-access article distributed under the terms of the Creative Commons Attribution License (CC BY). The use, distribution or reproduction in other forums is permitted, provided the original author(s) and the copyright owner(s) are credited and that the original publication in this journal is cited, in accordance with accepted academic practice. No use, distribution or reproduction is permitted which does not comply with these terms.
*Correspondence: Seth M. Levine, c2V0aC5sZXZpbmVAemktbWFubmhlaW0uZGU=; Jens V. Schwarzbach, amVucy5zY2h3YXJ6YmFjaEB1a3IuZGU=