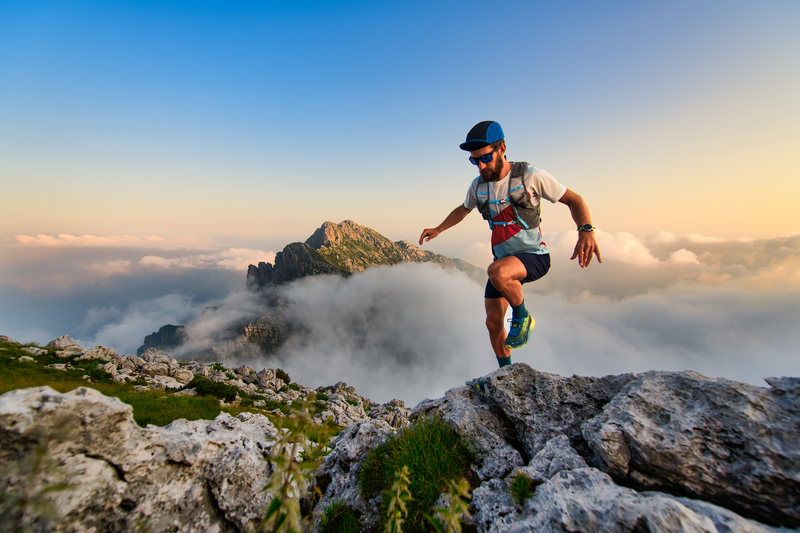
95% of researchers rate our articles as excellent or good
Learn more about the work of our research integrity team to safeguard the quality of each article we publish.
Find out more
MINI REVIEW article
Front. Psychiatry , 06 September 2021
Sec. Aging Psychiatry
Volume 12 - 2021 | https://doi.org/10.3389/fpsyt.2021.719125
This article is part of the Research Topic Artificial Intelligence in Geriatric Mental Health Research and Clinical Care View all 11 articles
Late-life depression (LLD) is a major public health concern. Despite the availability of effective treatments for depression, barriers to screening and diagnosis still exist. The use of current standardized depression assessments can lead to underdiagnosis or misdiagnosis due to subjective symptom reporting and the distinct cognitive, psychomotor, and somatic features of LLD. To overcome these limitations, there has been a growing interest in the development of objective measures of depression using artificial intelligence (AI) technologies such as natural language processing (NLP). NLP approaches focus on the analysis of acoustic and linguistic aspects of human language derived from text and speech and can be integrated with machine learning approaches to classify depression and its severity. In this review, we will provide rationale for the use of NLP methods to study depression using speech, summarize previous research using NLP in LLD, compare findings to younger adults with depression and older adults with other clinical conditions, and discuss future directions including the use of complementary AI strategies to fully capture the spectrum of LLD.
Depression is one of the leading causes of disability worldwide, affecting more than 264 million people of all ages (1). Although less prevalent among older adults (2), late-life depression (LLD), also referred to as geriatric depression, remains a major public health concern due to increased risk of morbidity, suicide, physical, cognitive, and social impairments, and self-neglect (3, 4). With a progressively aging population globally, the identification and treatment of LLD is critical (5).
LLD is generally defined as depression occurring in individuals aged 60 and over, though cutoffs vary in the literature. LLD can be further divided into early onset (first depressive episode before age 60) and late onset (first depressive episode after age 60) (6). For the purposes of this review, the definition of LLD includes both early and late onset episodes (5, 7, 8). As with younger individuals, LLD can be heterogeneous ranging from subthreshold changes in mood to major depression as outlined by the Diagnostic and Statistical Manual of Mental Disorders (DSM-5). However, diagnosing LLD is more challenging due to a different symptom profile compared to younger adults, and additional medical comorbidities (5, 9, 10). Misdiagnosis can occur if classic depressive symptoms (e.g., low mood) are not verbally expressed, and instead only somatic or cognitive symptoms are reported (11). Current depression treatment guidelines also recommend the use of standardized rating scales to gauge symptom severity (5), however, these scales may over or under emphasize the presence of somatic symptoms. One recent review of LLD scales suggested that the over-reliance on somatic items may result in a misdiagnosis of LLD due to the high prevalence of medical comorbidities in older adults (12). Additionally only a handful of assessments, including the Patient Health Questionnaire-9 (PHQ-9), Cornell Scale for Depression in Dementia, Geriatric Depression Scale, and the Hospital Anxiety and Depression Scale have specifically been validated in LLD (13–16). However, these validated scales can be susceptible to bias due to the subjective nature of scoring by the assessing clinician. These scales might also falsely identify individuals with cognitive impairment as depressed (10, 17).
To help overcome these limitations, there has been a growing interest in the development of objective measures of depression using artificial intelligence (AI) technologies such as natural language processing (NLP) (18–20). NLP approaches focus on the analysis of acoustic and linguistic aspects of human language derived from speech and text and can be integrated with machine learning approaches to classify depression and its severity (19, 21). Advantages of using these approaches to understand depression symptoms through speech include high ecological validity, low subjectivity, low cost of frequent assessments, and quicker administration of tasks compared to standard assessments. An added benefit of speech analysis using NLP is that speech data can be collected remotely, meeting a vital need for remote cognitive and behavioral assessments in the era of the coronavirus disease (COVID-19) pandemic (22). In this review we will provide rationale for the use of NLP approaches to study depression using speech, summarize previous research using NLP in LLD, compare findings to younger adults with depression and older adults with other clinical conditions, and discuss future directions including the use of complementary AI strategies to fully capture the spectrum of LLD symptoms.
To search for relevant literature related to speech analysis in individuals with depression or LLD, PubMed/MEDLINE, Web of Science, and Google Scholar databases were searched using terms including: “geriatric depression”, “older adult depression”, “late-life depression”, “major depressive disorder”, “natural language processing”, “speech analysis”, “speech”, “acoustics”, “linguistics”, “voice”. A sample search query used in the PubMed database is: [(“geriatric depression” OR “late-life depression”) AND (“speech” OR “linguistic” OR “acoustic” OR “language” or “voice”)]. While this mini review was not intended to be a systematic review of all literature related to NLP and depression, we used broad search terms to capture as many studies as possible specifically related to NLP in LLD. Only English language studies were included in the search strategy and no restrictions were placed on the year of publication.
Speech production is a complex process involving the communication of thoughts, ideas, and emotions by way of spoken words and phrases. Variations in physiology, cognition, and mood can produce noticeable changes in speech assessed by measures that capture what is being said through word selections and grammar usage (linguistic features) and how people sound based on acoustic waveforms (acoustic features) (Figure 1). For over a century, clinicians have documented subtle alterations in speech patterns in individuals with depression with early reports highlighting speech that was lower in pitch, more monotonous, slower, and more hesitant (23). These observations were most consistently seen in melancholic and psychotic depression, both of which are characterized by psychomotor retardation (24), a core feature of major depressive disorder (MDD). Other early studies investigating speech in the context of depression and psychomotor retardation reported paucity of speech, lower volume and tone, slowed responses, and monotonous speech (24, 25). Slowed speech or “speaking so slowly that other people could have noticed” is now routinely analyzed as part of self-report depression assessments such as the PHQ-9 (26).
Figure 1. Schematic representation showing how speech can be used to study linguistic and acoustic alterations in depression. To assess linguistic aspects of speech, audio samples are first transcribed using either manual or automatic speech recognition processes. Natural language processing methods can then be used to assess lexical, syntactic, and content measures of language. Audio waveforms can be directly analyzed to capture acoustic aspects of speech such as pause time, speech rate, and fundamental frequency. The right aspect of the figure shows examples of commonly reported speech alterations in the depression literature and the direction of these alterations compared to controls.
With regard to temporal characteristics of speech in depression, speech pause time or the amount of time between utterances, has been studied since the 1940s. During this period, timing devices could be used to measure speech pause times by manually pressing switches to indicate the start and end of a pause. In one study that included a broad group of individuals with psychiatric diagnoses, it was shown that patients with depression had more silent periods compared to those with hypomania (27). Later research improved study design by more precisely grouping patients according to clinical presentation (e.g., unipolar vs. bipolar, endogenous vs. neurotic) or by using specific tasks to elicit speech. In a small pilot study by Szabadi and colleagues, speech pause time was assessed in individuals with unipolar depression and in healthy controls during a counting task (28). The results showed that participants with depression had elongated speech pause time compared to controls, whose speech pause time remained consistent over a period of 2 months. Importantly, after recovery, pause time alterations normalized in patients, suggesting a role for speech pause time as a marker of clinical improvement. In a series of follow-up studies, Greden et al. replicated the elongated speech pause time findings in larger samples of individuals with depression (29, 30). Hardy and colleagues additionally showed that changes in speech pause time between baseline and final evaluations following a course of treatment for depression were associated with clinical changes on the Retardation Rating Scale for Depression (31). Others have not replicated this finding but have instead suggested that speech pause time abnormalities may be more evident during certain speech tasks (e.g., counting task) and/or only reflect certain depression symptoms or subtypes (32).
Focusing on the linguistic aspects of speech, early studies of depression used psycholinguistic approaches to manually encode measures characterizing lexical diversity, syntactic complexity, and speech content. In one study, comparing individuals with depression to those with mania, depressed participants used more modifying adverbs, first-person pronouns, and personal pronouns. In contrast, participants with mania used more action verbs, adjectives, and concrete nouns (33). Through content analysis, it was also shown that depressed patients used more words indicating self-preoccupation, in line with the large body of literature indicating a role for increased self-rumination in depression [e.g., (34, 35)].
While foundational to our understanding of speech alterations in depression, many early studies relied on traditional approaches such as expert opinion or manual linguistic annotation that have known limitations including subjectivity and limited application to large-scale studies or clinical settings (36). With improved technology, supported by advances in AI, the ability to detect and objectively quantify speech alterations in depression has drastically improved. NLP is a branch of AI that specifically focuses on understanding, interpreting, and manipulating large amounts of human language and speech data. It combines computational linguistics with statistical, machine learning, and deep learning models, to take unstructured, free-form data (e.g., a voice recording or writing sample) and produce structured, quantitative acoustic and linguistic outputs. NLP has the potential to capture the speech changes in depression that reflect both physiologic changes at the basic motor level and also higher-level cognitive processing.
A substantial literature now exists examining automated assessments for depression using speech analysis, with acoustic or paralinguistic speech properties being the focus of multiple in-depth reviews (19, 21). In general, a number of acoustic measures characterized by source features from the vocal folds [e.g., jitter, shimmer, harmonics-to-noise ratio (HNR)], filter features from the vocal tract (e.g., F1 and F2 formants), spectral features [e.g., Mel Frequency Cepstral Coefficients (MFCCs)], and prosodic/melodic features [e.g., fundamental frequency (F0), speech intensity, speed, and pause duration] have been shown to be altered in individuals with depression (19, 21). While some heterogeneity exists in the direction of the reported alterations for some features (e.g., loudness), several trends corroborating earlier studies are evident. For example, greater depression severity is frequently associated with decreased F0 or pitch, intensity variability, and speech rate reflecting slower, more monotonous speech patterns (19, 37–41). Other acoustic measures such as jitter, shimmer, and HNR tend to be higher in depressed patients (19), reflecting laryngeal muscle tension, typically perceived as breathy, rough, or hoarse voices (42). In classification models, MFCCs have been shown to discriminate depressed patients from controls with high sensitivity and specificity (43–45). MFCCs have also been shown to classify the speech of stressed individuals (46). Since depressed language can also be associated with stress (47), future research to disentangle how MFCCs are altered in various cognitive and emotional states is warranted.
Computerized analysis of linguistic speech measures in depression is becoming more common with methods such as Linguistic Inquiry and Word Count (LIWC) (48), which has advantages such as high inter-rater reliability, objectivity, and cost-effectiveness compared to earlier manual approaches. Using LIWC, a recent study showed that participants with depression used more verbal utterances related to sadness compared to individuals with anxiety or comorbid depression and anxiety; however, the groups did not differ in the use of first-person singular pronouns (49). Others have used LIWC to develop composite measures tapping into first-person singular pronoun use, negative affect words, and positive affect words to capture linguistic patterns of depressed affect in nonclinical samples (47). To best capture depression symptom heterogeneity, automated speech analysis methods combining acoustic and linguistic measures may prove to be the most informative (50).
Given previous work establishing the relationship between speech alterations in depression, researchers have started investigating how speech may be altered in LLD, specifically. Table 1 summarizes recent literature on the topic and highlights different approaches used to collect and analyze speech data. While it is difficult to draw conclusions based on heterogeneous samples and methods, automated speech analysis is proving to be a promising means to tap into cognitive and depressive symptoms in LLD and can be readily adapted for naturalistic settings (55). Encouraging findings indicate that vocal measures can predict high and low depression scores in LLD between 86 and 92% of the time (54). Others have shown that LLD can be classified with 77–86% accuracy compared to age-matched controls (56). In the latter study, acoustic features contributing to accuracy values differed between sexes, highlighting the importance of taking demographic factors such as age, sex, and education into account. Speech has been shown to differ based on these variables even in the absence of clinical conditions. For example, morphological differences in vocal fold length between males and females contribute variation in acoustic features such as F0 (58).
The use of NLP in LLD presents unique challenges with regard to understanding the specificity of identified speech alterations. Older adults are more likely to have additional medical comorbidities that may also cause speech changes (59, 60). For example, depression is both an independent risk factor and a prodrome for dementia (61), which makes an underlying neurocognitive disorder a potential confounding factor in detecting speech pattern changes (62). Second, older adults are more likely to be on multiple prescribed medications, which introduces the confounding factor of medication effects on the acoustic properties of speech (63). Compared to younger adults, older adults have been found to have a lower HNR, a marker of turbulent airflow generated at the glottis during phonation (64, 65), which may be partly attributable to the effects of medications (e.g., vocal tract dryness and thickened mucosal secretions). Finally, normal age-related hormonal and structural changes (e.g., cartilage ossification, muscle degeneration) (64, 65), may also contribute to lower HNR during phonation. These factors highlight the importance of including tightly matched control groups and large sample sizes to broadly examine how different factors impact speech measures in the older adult population.
Despite these challenges, the use of NLP approaches in older adults provides opportunities to improve our understanding of depression in the context of biological sex, medical comorbidities, and other clinical factors. For example, speech changes in mild cognitive impairment (MCI) and Alzheimer's disease (AD) have been well documented in recent years (66–68), and share commonalities with speech changes related to depression. Increased pause duration, increased pronoun use and reduced lexical and syntactic complexity have all been reported as occurring in MCI and AD (66, 67, 69, 70). Due to these similar changes in speech patterns, care must be taken to differentiate dementia from depression when using speech analysis tools, to avoid misdiagnosis. One study compared LLD to those with early AD on a picture description task, and found that those with AD had reduced informativeness of their descriptions, suggesting that measures of content may be useful in differentiating depression from AD (51). Two recent studies suggested that certain acoustic speech features may help differentiate depression and dementia, or dementia with and without comorbid depression (66, 71), but this topic requires further research.
Studies comparing the speech rate of LLD vs. Parkinson's disease (PD) consistently show that rate of speech is significantly reduced in LLD, whereas the finding is not consistent in PD. Individuals with PD may exhibit decreased, increased, or typical speech rates (41, 72). These findings reflect underlying pathophysiological changes in PD such as compensation for hypokinetic muscle tone, which is not seen in LLD. These speech differences may serve as useful markers to detect or monitor for depression in PD given the high degree of comorbidity between these two diagnoses.
Finally, applying NLP using a disease-agnostic or transdiagnostic approach may also play an important role in addressing the comorbidity seen in LLD. For example, apathy is a transdiagnostic symptom seen in MDD, schizophrenia, traumatic brain injuries, AD, PD, and other neuropsychiatric disorders. A study by Konig and colleagues found that the presence of apathy was associated with shorter speech, slower speech, and lower variance of prosody (lower F0 range) (73). Thus, transdiagnostic markers like apathy may be a helpful method of discriminating which speech features are unique to a disorder vs. those that may be shared across diseases and disorders.
While current NLP approaches to capture symptoms of LLD are promising, there remain new opportunities to improve our understanding and implementation of this important research area. Over the past decade, advances in computer and mobile phone technologies have improved the quality and quantity of audiovisual input and output, allowing internet-based video clinical assessments to become more commonplace (74). The COVID-19 pandemic in particular has further led to a dramatic shift to online virtual care (75). With these shifts, NLP can potentially be applied to speech signals in real-time or asynchronously in clinical contexts. Recent studies have used NLP to generate COVID-19 phenotypes (76), track emotional distress in online cancer support groups (77), diagnose PD (78, 79), predict driving risk in older adults (80), and predict binge-eating behaviors (81).
These advances offer the potential of solving many of the challenges described in previous sections. For example, speech could be unobtrusively measured during routine visits on virtual care platforms between a healthcare provider and a patient. Data from these visits could be captured longitudinally and monitored for signs of depression, cognitive changes, or comorbid conditions. Individuals with depression in remission could conversely be monitored for signs of relapse. Preliminary studies have shown that integration of real-time audiovisual analysis into telemedicine platforms may be a feasible method of detecting an individual's emotional state (82). Additionally, the use of smartphone and wearables technology to record these features have also demonstrated feasibility and acceptability in initial pilot studies (55, 83).
Beyond the clinician-patient interaction, NLP could also be implemented in non-clinical settings. Recent advances in smart speaker technology (e.g., Amazon Alexa, Google Assistant, Microsoft Cortana, Apple Siri) has resulted in significant consumer adoption, and these devices are currently being investigated as tools to support independent living and wellness in older adults (84). These technologies may provide an opportunity for naturalistic speech to be collected longitudinally over time and may be a more granular and accurate method of detecting speech changes seen in depression (37). Advantages of smart speaker technologies include increased ease of use compared to computers and smartphones. Overall, these devices may hold promise to help older adults maintain independence through the use of passive monitoring for both depression and mild cognitive impairment and could serve as an “early warning system” to alert caregivers or professionals to negative symptom changes (84–86). However, the translation of AI technologies for home use in elderly populations may be limited without explicitly addressing ethical and legal considerations for patients, caregivers, and healthcare providers (87).
Regardless of the technology implemented, it is important to recognize that most of these technologies have not been specifically designed with older adults in mind. Older adults have reported hesitation about using novel technologies due to limited experience, frustration with technology, and physical health limitations (e.g., visual impairment) (88). From a privacy perspective, concerns have been raised by participants who may be unsure about how their electronic health data may be used, processed, or stored (89). Preliminary studies suggest these concerns can be mitigated with detailed informed consent from participants and by outlining privacy protocols in place (55). Ensuring that these technologies are culturally-adapted is another important consideration that can affect use and adoption (90). Finally, older adults have been shown to respond better to digital assistants with a socially-oriented interaction style (e.g., embedding informal conversation, using small talk, and encouragement) rather than assistants with a task-oriented style (e.g., structured formal responses) (91). As a result, there has been greater focus on embedding these interactions into automated social chatbots and companion robots for older adults (92). Tailoring these technologies to older adults has the potential to reduce technology hesitancy, improve adoption, and potentially increase the reliability of data that is collected as well (93).
With an aging population globally, the identification and treatment of depression in older adults is critical. NLP approaches are proving to be a promising means to help assess, monitor, and detect depression and other comorbidities in older individuals based on speech. However, additional research is needed to fully characterize the spectrum of depression symptoms experienced by older individuals. Complementary speech collection and analysis strategies using AI, wearables, and other novel technologies may help further advance this important field.
DD and AY contributed to the conception, design of the study, and wrote the first draft of the manuscript. JR and MG wrote sections of the manuscript. All authors contributed to manuscript revision, read, and approved the submittedversion.
DD, JR, and MG are employees of Winterlight Labs. AY is a medical consultant for Winterlight Labs.
All claims expressed in this article are solely those of the authors and do not necessarily represent those of their affiliated organizations, or those of the publisher, the editors and the reviewers. Any product that may be evaluated in this article, or claim that may be made by its manufacturer, is not guaranteed or endorsed by the publisher.
1. James SL, Abate D, Abate KH, Abay SM, Abbafati C, Abbasi N, et al. Global, regional, and national incidence, prevalence, and years lived with disability for 354 diseases and injuries for 195 countries and territories, 1990–2017: a systematic analysis for the Global Burden of Disease Study 2017. Lancet. (2018) 392:1789–858. doi: 10.1016/S0140-6736(18)32279-7
2. Kessler RC, Birnbaum H, Bromet E, Hwang I, Sampson N, Shahly V. Age differences in major depression: results from the National Comorbidity Survey Replication (NCS-R). Psychol Med. (2010) 40:225–37. doi: 10.1017/S0033291709990213
3. Hodgetts S, Gallagher P, Stow D, Ferrier IN, O'Brien JT. The impact and measurement of social dysfunction in late-life depression: an evaluation of current methods with a focus on wearable technology. Int J Geriatr Psychiatry. (2017) 32:247–55. doi: 10.1002/gps.4632
4. Fiske A, Wetherell JL, Gatz M. Depression in older adults. Annu Rev Clin Psychol. (2009) 5:363–89. doi: 10.1146/annurev.clinpsy.032408.153621
5. Rodda J, Walker Z, Carter J. Depression in older adults. BMJ. (2011) 343:d5219–d5219. doi: 10.1136/bmj.d5219
6. Dols A, Bouckaert F, Sienaert P, Rhebergen D, Vansteelandt K. ten Kate M, et al. Early- and Late-Onset Depression in Late Life: A Prospective Study on Clinical and Structural Brain Characteristics and Response to Electroconvulsive Therapy. Am J Geriatric Psychiat. (2017) 25:178–89. doi: 10.1016/j.jagp.2016.09.005
7. Tedeschini E, Levkovitz Y, Iovieno N, Ameral VE, Nelson JC, Papakostas GI. Efficacy of antidepressants for late-life depression: a meta-analysis and meta-regression of placebo-controlled randomized trials. J Clin Psychiatry. (2011) 72:1660–8. doi: 10.4088/JCP.10r06531
8. Aziz R, Steffens DC. What are the causes of late-life depression? Psychiatric Clinics of North America. (2013) 36:497–516. doi: 10.1016/j.psc.2013.08.001
9. Kok RM, Reynolds CF. Management of depression in older adults: a review. JAMA. (2017) 317:2114. doi: 10.1001/jama.2017.5706
10. Andrews JA, Harrison RF, Brown LJE, MacLean LM, Hwang F, Smith T, et al. Using the NANA toolkit at home to predict older adults' future depression. J Affect Disord. (2017) 213:187–90. doi: 10.1016/j.jad.2017.02.019
11. Préville M, Mechakra Tahiri SD, Vasiliadis H-M, Quesnel L, Gontijo-Guerra S, Lamoureux-Lamarche C, et al. Association between perceived social stigma against mental disorders and use of health services for psychological distress symptoms in the older adult population: validity of the STIG scale. Aging Ment Health. (2015) 19:464–74. doi: 10.1080/13607863.2014.944092
12. Balsamo M, Cataldi F, Carlucci L, Padulo C, Fairfield B. Assessment of late-life depression via self-report measures: a review. CIA. (2018) 13:2021–44. doi: 10.2147/CIA.S178943
13. Alexopoulos GS, Abrams RC, Young RC, Shamoian CA. Cornell Scale for Depression in Dementia. BIOL PSYCHIATRY14. (1988) 23:271–84. doi: 10.1016/0006-3223(88)90038-8
14. Kenn C, Wood H, Kucyj M, Wattis J, Cunane J. Validation of the hospital anxiety and depression rating scale (HADS) in an elderly psychiatric population. Int J Geriatr Psychiatry. (1987) 2:189–93. doi: 10.1002/gps.930020309
15. Phelan E, Williams B, Meeker K, Bonn K, Frederick J, LoGerfo J, et al. study of the diagnostic accuracy of the PHQ-9 in primary care elderly. BMC Fam Pract. (2010) 11:63. doi: 10.1186/1471-2296-11-63
16. Yesavage JA, Brink TL, Rose TL, Lum O, Huang V, Adey M, et al. Development and validation of a geriatric depression screening scale: A preliminary report. J Psychiatr Res. (1982) 17:37–49. doi: 10.1016/0022-3956(82)90033-4
17. Brown LJE, Astell AJ. Assessing mood in older adults: a conceptual review of methods and approaches. Int Psychogeriatr. (2012) 24:1197–206. doi: 10.1017/S1041610212000075
18. Dai H-J, Su C-H, Lee Y-Q, Zhang Y-C, Wang C-K, Kuo C-J, et al. Deep learning-based natural language processing for screening psychiatric patients. Front Psychiatry. (2021) 11:533949. doi: 10.3389/fpsyt.2020.533949
19. Low DM, Bentley KH, Ghosh SS. Automated assessment of psychiatric disorders using speech: A systematic review. Laryngoscope Investigative Otolaryngology. (2020) 5:96–116. doi: 10.1002/lio2.354
20. Huang K-L, Duan S-F, Lyu X. Affective Voice Interaction and Artificial Intelligence: A research study on the acoustic features of gender and the emotional states of the PAD model. Front Psychol. (2021) 12:664925. doi: 10.3389/fpsyg.2021.664925
21. Cummins N, Scherer S, Krajewski J, Schnieder S, Epps J, Quatieri TF, et al. review of depression and suicide risk assessment using speech analysis. Speech Commun. (2015) 71:10–49. doi: 10.1016/j.specom.2015.03.004
22. Geddes MR, O'Connell ME, Fisk JD, Gauthier S, Camicioli R, Ismail Z. For the alzheimer society of Canada task force on dementia care best practices for COVID-19. Remote cognitive and behavioral assessment: Report of the Alzheimer Society of Canada Task Force on dementia care best practices for COVID-19. Alzheimer's Dement. (2020) 12: e12111. doi: 10.1002/dad2.12111
23. Kraepelin E. Manic Depressive Insanity and Paranoia. The Journal of Nervous and Mental Disease. (1921) 53:350. Available online at: https://journals.lww.com/jonmd/Fulltext/1921/04000/Manic_Depressive_Insanity_and_Paranoia.57.aspx (accessed May 30, 2021).
24. Buyukdura JS, McClintock SM, Croarkin PE. Psychomotor retardation in depression: Biological underpinnings, measurement, and treatment. Progress in Neuro-Psychopharmacology and Biological Psychiatry. (2011) 35:395–409. doi: 10.1016/j.pnpbp.2010.10.019
25. Sobin C, Sackeim HA. Psychomotor Symptoms of Depression. Am J Psychiatry. (1997)154:4–17. doi: 10.1176/ajp.154.1.4
26. Kroenke K, Spitzer RL, Williams JBW. The PHQ-9: Validity of a brief depression severity measure. J Gen Intern Med. (2001) 16:606–13. doi: 10.1046/j.1525-1497.2001.016009606.x
27. Chapple ED, Lindemann E. Clinical Implications of Measurements of Interaction Rates in Psychiatric Interviews. Hum Organ. (1942) 1:1–11. doi: 10.17730/humo.1.2.3r61twt687148g1j
28. Szabadi E, Bradshaw CM, Besson JA. Elongation of pause-time in speech: a simple, objective measure of motor retardation in depression. Br J Psychiatry. (1976) 129:592–7. doi: 10.1192/bjp.129.6.592
29. Greden JF, Albala AA, Smokler IA, Gardner R, Carroll BJ. Speech pause time: a marker of psychomotor retardation among endogenous depressives. Biol Psychiatry. (1981) 16:851–9.
30. Greden JF. Biological markers of melancholia and reclassification of depressive disorders. Encephale. (1982) 8:193–202.
31. Hardy P, Jouvent R, Widl D. Speech Pause Time and the Retardation Rating Scale for Depression (ERD) Towards a Reciprocal Validation.5. J Affect Disord. (1984) 6:123–7. doi: 10.1016/0165-0327(84)90014-4
32. Nilsonne Å. Acoustic analysis of speech variables during depression and after improvement. Acta Psychiatr Scand. (1987) 76:235–45. doi: 10.1111/j.1600-0447.1987.tb02891.x
33. Andreasen NJC. Linguistic analysis of speech in affective disorders. Arch Gen Psychiatry. (1976) 33:1361. doi: 10.1001/archpsyc.1976.01770110089009
34. Takano K, Tanno Y. Self-rumination, self-reflection, and depression: Self-rumination counteracts the adaptive effect of self-reflection. Behav Res Ther. (2009) 47:260–4. doi: 10.1016/j.brat.2008.12.008
35. Nolen-Hoeksema S, Wisco BE, Lyubomirsky S. Rethinking rumination. Perspect Psychol Sci. (2008) 3:400–24. doi: 10.1111/j.1745-6924.2008.00088.x
36. Corcoran CM, Mittal VA, Bearden CE, Gur ER, Hitczenko K, Bilgrami Z, et al. Language as a biomarker for psychosis: A natural language processing approach. Schizophrenia Res. (2020) S0920996420302474. doi: 10.1016/j.schres.2020.04.032
37. Mundt JC, Snyder PJ, Cannizzaro MS, Chappie K, Geralts DS. Voice acoustic measures of depression severity and treatment response collected via interactive voice response (IVR) technology. J Neurolinguistics. (2007) 20:50–64. doi: 10.1016/j.jneuroling.2006.04.001
38. Horwitz R, Quatieri TF, Helfer BS, Yu B, Williamson JR, Mundt J. On the relative importance of vocal source, system, and prosody in human depression. In: 2013 IEEE International Conference on Body Sensor Networks. Cambridge, MA, USA: IEEE. (2013) p. 1–6.
39. Alpert M, Pouget ER, Silva RR. Reflections of depression in acoustic measures of the patient's speech. J Affect Disord. (2001) 66:59–69. doi: 10.1016/S0165-0327(00)00335-9
40. Mundt JC, Vogel AP, Feltner DE, Lenderking WR. Vocal acoustic biomarkers of depression severity and treatment response. Biol Psychiatry. (2012) 72:580–7. doi: 10.1016/j.biopsych.2012.03.015
41. Cannizzaro M, Harel B, Reilly N, Chappell P, Snyder PJ. Voice acoustical measurement of the severity of major depression. Brain Cogn. (2004) 56:30–5. doi: 10.1016/j.bandc.2004.05.003
42. Farrús M, Hernando J, Ejarque P. Jitter and Shimmer Measurements for Speaker Recognition. Eighth Annual Conference of the International Speech Communication Association. (2007) p. 778–781.
43. Taguchi T, Tachikawa H, Nemoto K, Suzuki M, Nagano T, Tachibana R, et al. Major depressive disorder discrimination using vocal acoustic features. J Affect Disord. (2018) 225:214–20. doi: 10.1016/j.jad.2017.08.038
44. France DJ, Shiavi RG, Wilkes DM. Acoustical properties of speech as indicators of depression and suicidal risk. IEEE Transactions On Biomedical Engineering. (2000) 47:9. doi: 10.1109/10.846676
45. Cummins N, Epps J, Breakspear M, Goecke R. An Investigation of Depressed Speech Detection: Features and Normalization. 12th Annual Conference of the International Speech Communication Association. (2011) p. 2997–3000.
46. Patro H, Senthil Raja G, Dandapat S. Statistical feature evaluation for classification of stressed speech. Int J Speech Technol. (2007) 10:143–52. doi: 10.1007/s10772-009-9021-0
47. Newell EE, McCoy SK, Newman ML, Wellman JD, Gardner SK. You sound so down: capturing depressed affect through depressed language. J Lang Soc Psychol. (2018) 37:451–74. doi: 10.1177/0261927X17731123
48. Tausczik YR, Pennebaker JW. The psychological meaning of words: LIWC and computerized text analysis methods. J Lang Soc Psychol. (2010) 29:24–54. doi: 10.1177/0261927X09351676
49. Sonnenschein AR, Hofmann SG, Ziegelmayer T, Lutz W. Linguistic analysis of patients with mood and anxiety disorders during cognitive behavioral therapy. Cogn Behav Ther. (2018) 47:315–27. doi: 10.1080/16506073.2017.1419505
50. Arevian AC, Bone D, Malandrakis N, Martinez VR, Wells KB, Miklowitz DJ, et al. Clinical state tracking in serious mental illness through computational analysis of speech. PLoS ONE. (2020) 15:e0225695. doi: 10.1371/journal.pone.0225695
51. Murray LL. Distinguishing clinical depression from early Alzheimer's disease in elderly people: Can narrative analysis help? Aphasiology. (2010) 24:928–39. doi: 10.1080/02687030903422460
52. Rabbi M, Ali S, Choudhury T, Berke E. Passive and In-Situ assessment of mental and physical well-being using mobile sensors. In :Proceedings of the 13th international conference on Ubiquitous computing - UbiComp'11. Beijing, China: ACM Press. (2011) p. 385. doi: 10.1145/2030112.2030164
53. Andresen EM, Malmgren JA, Carter WB, Patrick DL. Screening for depression in well older adults: evaluation of a short form of the CES-D (Center for Epidemiologic Studies Depression Scale). Am J Prev Med. (1994) 10:77–84.
54. Smith M, Dietrich BJ, Bai E, Bockholt HJ. Vocal pattern detection of depression among older adults. (2019) 29:440–9. doi: 10.1111/inm.12678
55. Little B, Alshabrawy O, Stow D, Ferrier IN, McNaney R, Jackson DG, et al. Deep learning-based automated speech detection as a marker of social functioning in late-life depression. Psychol Med. (2020) 1–10. doi: 10.1017/S0033291719003994
56. Lee S, Suh SW, Kim T, Kim K, Lee KH, Lee JR, et al. Screening major depressive disorder using vocal acoustic features in the elderly by sex. J Affect Disord. (2021) 291:15–23. doi: 10.1016/j.jad.2021.04.098
57. Albuquerque L, Valente ARS, Teixeira A, Figueiredo D, Sa-Couto P, Oliveira C. Association between acoustic speech features and non-severe levels of anxiety and depression symptoms across lifespan. PLoS ONE. (2021) 16:e0248842. doi: 10.1371/journal.pone.0248842
58. Titze IR. Physiologic and acoustic differences between male and female voices. J Acoust Soc Am. (1989) 85:1699–707. doi: 10.1121/1.397959
59. Murton OM, Hillman RE, Mehta DD, Semigran M, Daher M, Cunningham T, et al. Acoustic speech analysis of patients with decompensated heart failure: A pilot study. J Acoust Soc Am. (2017) 142:EL401. doi: 10.1121/1.5007092
60. Amir O, Anker SD, Gork I, Abraham WT, Pinney SP, Burkhoff D, et al. Feasibility of remote speech analysis in evaluation of dynamic fluid overload in heart failure patients undergoing haemodialysis treatment. ESC Heart Failure. (2021) 8:2467–72. doi: 10.1002/ehf2.13367
61. Livingston G, Huntley J, Sommerlad A, Ames D, Ballard C, Banerjee S, et al. Dementia prevention, intervention, and care: 2020 report of the Lancet Commission. Lancet. (2020) 396:413–46. doi: 10.1016/S0140-6736(20)30367-6
62. Leyhe T, Reynolds CF. 3rd, Melcher T, Linnemann C, Klöppel S, Blennow K, et al. A common challenge in older adults: Classification, overlap, and therapy of depression and dementia. Alzheimer's Dement. (2017) 13:59–71. doi: 10.1016/j.jalz.2016.08.007
63. Charlesworth CJ, Smit E, Lee DSH, Alramadhan F, Odden MC. Polypharmacy among adults aged 65 years and older in the United States: 1988-2010. J Gerontol A Biol Sci Med Sci. (2015) 70:989–95. doi: 10.1093/gerona/glv013
64. Ferrand CT. Harmonics-to-noise ratio: an index of vocal aging. J Voice. (2002) 16:8. doi: 10.1016/s0892-1997(02)00123-6
65. Gorham-Rowan MM, Laures-Gore J. Acoustic-perceptual correlates of voice quality in elderly men and women. J Commun Disord. (2006) 39:171–84. doi: 10.1016/j.jcomdis.2005.11.005
66. Fraser KC, Rudzicz F, Hirst G. Detecting late-life depression in Alzheimer's disease through analysis of speech and language. In: Proceedings of the Third Workshop on Computational Lingusitics and Clinical Psychology. San Diego, CA, USA: Association for Computational Linguistics. (2016) p. 1–11. doi: 10.18653/v1/W16-0301
67. Ahmed S, de Jager CA, Haigh A-M, Garrard P. Semantic processing in connected speech at a uniformly early stage of autopsy-confirmed Alzheimer's disease. Neuropsychology. (2013) 27:79–85. doi: 10.1037/a0031288
68. Szatloczki G, Hoffmann I, Vincze V, Kalman J, Pakaski M. Speaking in Alzheimer's disease, is that an early sign? Importance of changes in language abilities in Alzheimer's Disease. Front Aging Neurosci. (2015) 7:195. doi: 10.3389/fnagi.2015.00195
69. Mueller KD, Koscik RL, Hermann BP, Johnson SC, Turkstra LS. Declines in connected language are associated with very early mild cognitive impairment: results from the Wisconsin registry for Alzheimer's prevention. Front Aging Neurosci. (2018) 9:437. doi: 10.3389/fnagi.2017.00437
70. Hoffmann I, Nemeth D, Dye CD, Pákáski M, Irinyi T, Kálmán J. Temporal parameters of spontaneous speech in Alzheimer's disease. Int J Speech Lang Pathol. (2010) 12:29–34. doi: 10.3109/17549500903137256
71. Sumali B, Mitsukura Y, Liang K, Yoshimura M, Kitazawa M, Takamiya A, et al. Speech quality feature analysis for classification of depression and dementia patients. Sensors. (2020) 20:3599. doi: 10.3390/s20123599
72. Flint AJ, Black SE, Campbell-Taylor I, Gailey GF, Levinton C. Acoustic analysis in the differentiation of Parkinson's disease and major depression. J Psycholinguist Res. (1992) 21:383–99. doi: 10.1007/BF01067922
73. König A, Linz N, Zeghari R, Klinge X, Tröger J, Alexandersson J, et al. Detecting apathy in older adults with cognitive disorders using automatic speech analysis. J Alzheimer's Disease. (2019) 69:1183–93. doi: 10.3233/JAD-181033
74. Kichloo A, Albosta M, Dettloff K, Wani F, El-Amir Z, Singh J, et al. Telemedicine, the current COVID-19 pandemic and the future: a narrative review and perspectives moving forward in the USA. Fam Med Com Health. (2020) 8:e000530. doi: 10.1136/fmch-2020-000530
75. Gosse PJ, Kassardjian CD, Masellis M, Mitchell SB. Virtual care for patients with Alzheimer disease and related dementias during the COVID-19 era and beyond. CMAJ. (2021) 193:E371–7. doi: 10.1503/cmaj.201938
76. Barr PJ, Ryan J, Jacobson NC. Precision assessment of COVID-19 phenotypes using large-scale clinic visit audio recordings: harnessing the power of patient voice. J Med Internet Res. (2021) 23:e20545. doi: 10.2196/20545
77. Leung YW, Wouterloot E, Adikari A, Hirst G, de Silva D, Wong J, et al. Natural language processing–based virtual cofacilitator for online cancer support groups: protocol for an algorithm development and validation study. JMIR Res Protoc. (2021) 10:e21453. doi: 10.2196/21453
78. Frid EJ, Safra H, Hazan LL, Lokey D, Hilu L, Manevitz LO, et al. Computational diagnosis of Parkinson's disease directly from natural speech using machine learning techniques. In: 2014 IEEE International Conference on Software Science, Technology and Engineering. (2014) p. 50–53. doi: 10.1109/SWSTE.2014.17
79. Singh S, Xu W. Robust detection of Parkinson's disease using harvested smartphone voice data: a telemedicine approach. Telemedicine and e-Health. (2019) 26:327–34. doi: 10.1089/tmj.2018.0271
80. Yamada Y, Shinkawa K, Kosugi A, Kobayashi M, Takagi H, Nemoto M, et al. Predicting future accident risks of older drivers by speech data from a voice-based dialogue system: a preliminary result. In: Spohrer J, Leitner C, editors. Advances in the Human Side of Service Engineering. Cham: Springer International Publishing. (2021) p. 131–137.
81. Funk B, Sadeh-Sharvit S, Fitzsimmons-Craft EE, Trockel MT, Monterubio GE, Goel NJ, et al. A Framework for applying natural language processing in digital health interventions. J Med Internet Res. (2020) 22:e13855. doi: 10.2196/13855
82. Kallipolitis A, Galliakis M, Menychtas A, Maglogiannis I. Affective analysis of patients in homecare video-assisted telemedicine using computational intelligence. Neural Comput & Applic. (2020) 32:17125–36. doi: 10.1007/s00521-020-05203-z
83. Abbas A, Schultebraucks K, Galatzer-Levy IR. Digital measurement of mental health: challenges, promises, and future directions. Psychiatr Ann. (2021) 51:14–20. doi: 10.3928/00485713-20201207-01
84. Pradhan A, Lazar A. Voice technologies to support aging in place: opportunities and challenges. Innovation in Aging. (2020) 4:317–8. doi: 10.1093/geroni/igaa057.1016
85. Choi YK, Thompson HJ, Demiris G. Use of an internet-of-things smart home system for healthy aging in older adults in residential settings: pilot feasibility study. JMIR Aging. (2020) 3:e21964. doi: 10.2196/21964
86. Rantz MJ, Scott SD, Miller SJ, Skubic M, Phillips L, Alexander G, et al. Evaluation of health alerts from an early illness warning system in independent living. Comput Inform Nurs. (2013) 31:274–80. doi: 10.1097/NXN.0b013e318296298f
87. Ho A. Are we ready for artificial intelligence health monitoring in elder care? BMC Geriatr. (2020) 20:358. doi: 10.1186/s12877-020-01764-9
88. Chung J, Bleich M, Wheeler DC, Winship JM, McDowell B, Baker D, et al. Attitudes and perceptions toward voice-operated smart speakers among low-income senior housing residents: comparison of pre- and post-installation surveys. Gerontol Geriatr Med. (2021) 7:233372142110058. doi: 10.1177/23337214211005869
89. Stanberry B. Telemedicine: barriers and opportunities in the 21st century. J Intern Med. (2000) 247:615–28. doi: 10.1046/j.1365-2796.2000.00699.x
90. Chung J, Thompson HJ, Joe J, Hall A, Demiris G. Examining Korean and Korean American older adults' perceived acceptability of home-based monitoring technologies in the context of culture. Inform Health Soc Care. (2017) 42:61–76. doi: 10.3109/17538157.2016.1160244
91. Chattaraman V, Kwon W-S, Gilbert JE, Ross K. Should AI-Based, conversational digital assistants employ social- or task-oriented interaction style? A task-competency and reciprocity perspective for older adults. Computers in Human Behavior. (2019) 90:315–30. doi: 10.1016/j.chb.2018.08.048
92. Pou-Prom C, Raimondo S, Rudzicz F. A conversational robot for older adults withAlzheimer's disease. J Hum-Robot Interact. (2020) 9:1–25. doi: 10.1145/3380785
Keywords: geriatric mental health, depression, speech, natural language processing, artificial intelligence, digital health, late-life depression
Citation: DeSouza DD, Robin J, Gumus M and Yeung A (2021) Natural Language Processing as an Emerging Tool to Detect Late-Life Depression. Front. Psychiatry 12:719125. doi: 10.3389/fpsyt.2021.719125
Received: 01 June 2021; Accepted: 11 August 2021;
Published: 06 September 2021.
Edited by:
Ipsit Vahia, McLean Hospital, United StatesReviewed by:
Sheng-Min Wang, Catholic University of Korea, South KoreaCopyright © 2021 DeSouza, Robin, Gumus and Yeung. This is an open-access article distributed under the terms of the Creative Commons Attribution License (CC BY). The use, distribution or reproduction in other forums is permitted, provided the original author(s) and the copyright owner(s) are credited and that the original publication in this journal is cited, in accordance with accepted academic practice. No use, distribution or reproduction is permitted which does not comply with these terms.
*Correspondence: Danielle D. DeSouza, ZGFuaWVsbGVAd2ludGVybGlnaHRsYWJzLmNvbQ==
Disclaimer: All claims expressed in this article are solely those of the authors and do not necessarily represent those of their affiliated organizations, or those of the publisher, the editors and the reviewers. Any product that may be evaluated in this article or claim that may be made by its manufacturer is not guaranteed or endorsed by the publisher.
Research integrity at Frontiers
Learn more about the work of our research integrity team to safeguard the quality of each article we publish.