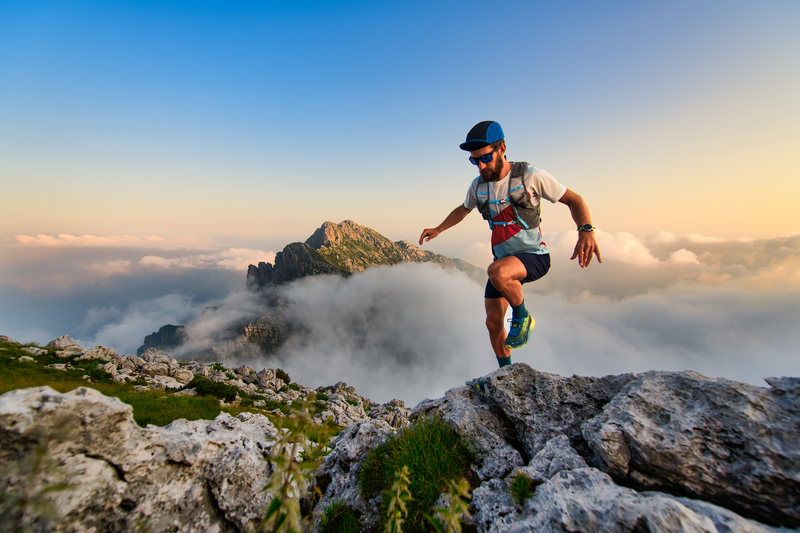
95% of researchers rate our articles as excellent or good
Learn more about the work of our research integrity team to safeguard the quality of each article we publish.
Find out more
ORIGINAL RESEARCH article
Front. Psychiatry , 23 September 2021
Sec. Child and Adolescent Psychiatry
Volume 12 - 2021 | https://doi.org/10.3389/fpsyt.2021.714301
This article is part of the Research Topic Internet Addiction & Gaming Disorders in Children and Adolescents View all 9 articles
Introduction: Globally, more people are spending time on the Internet and gaming since the outbreak of the Coronavirus Disease 2019 (COVID-19). Consequently, concerns about developing behavioral addiction of adolescents have been raised. Such risk could be greater for adolescents in South Korea where the majority of adolescents have access to the Internet and own a smartphone. In fact, statistics indicate that Korean youths are spending significantly more time on the Internet and gaming during the COVID-19 pandemic. Previous studies on the patterns of time spent on the Internet and Internet gaming show inconsistent results. The aim of this study is to investigate the latent profiles of the Internet and Internet game usage among adolescents in South Korea.
Method: Data from a national survey on elementary and middle school students across South Korea were used. The sample consists of 3,149 respondents, and 2,984 responses were analyzed after removing missing responses. Latent profile analysis was performed to investigate the number of latent profiles for the Internet and Internet game usage time. To validate the profiles, differences in problematic gaming behavior, sex, and neuroticism were examined.
Results: Seven profiles were found: Casual User, Moderate User, Smartphone User, Internet User, PC Internet Gamer, Heavy User, and Excessive User. Validation of the profiles indicated differences in problematic gaming behavior, sex, and neuroticism among selected profiles.
Conclusion: This study presented different profiles of the Internet and Internet game usage among adolescents in South Korea. Profiles with higher game usage time scored higher in problematic game use compared to other profiles. Males were more likely to be in the profiles with high gaming time, and females were more likely to be in Internet and Smartphone User profiles. The results indicate that Internet and Internet gaming usage patterns could be classified by the type of device used and the content of the Internet.
During the Coronavirus Disease 2019 (COVID-19) pandemic, social distancing, school closures, and quarantine became the new norm worldwide. These phenomena resulted in people spending more time at home and on the Internet (1). For example, Steam, the biggest digital personal computer (PC) gaming platform and market, announced that it has reached the highest number of concurrent users in its 16-year service history at 20,313,451 (2). As for mobile gaming, Google reported that the duration and frequency of gaming increased in two-thirds of mobile gamers across 10 countries (3). Online gaming increased by 75% in the USA (4).
With increased Internet and game usage due to the COVID-19 pandemic, there have been concerns regarding an elevated risk of developing behavioral addictions, especially Internet and gaming addiction (5–9). Increased levels of depression, anxiety, and stress due to the pandemic (10, 11) could make individuals more susceptible to addictive behaviors such as Internet surfing and gaming (6). In particular, children and adolescents could be more vulnerable to such risks (5, 12). Recent studies found a link between adolescents' mental health and their use of the Internet and gaming during the COVID-19 pandemic (13–15).
The risk of developing Internet addiction and Internet gaming addiction could be particularly high for the adolescents in South Korea. Among Korean adolescents, 99.1% use the Internet regularly, 58.8% play a PC game, and 82.4% play a mobile game (16). A significant percentage of these adolescents reported that their time spent on PC game (45.5%) and mobile game (56.6%) increased after the COVID-19 outbreak. Thus, an in-depth investigation into the trend of increased time spent on Internet and Internet gaming of South Korean adolescents is warranted.
Different diagnostic criteria for Internet addiction have been proposed under the terms such as “Internet addiction disorder,” “problematic Internet use,” “pathological Internet use,” and “Internet dependency” (17). Griffiths (18) suggested that Internet behaviors could be addictive much like substance addiction. This perspective brought Internet gaming disorder (IGD) in Section III of the 5th edition of Diagnostic and Statistical Manual of Mental Disorders (DSM-5) (19). In 2019, the 11th edition of the International Classification of Disease (ICD-11) also added a classification of GD. Despite the inclusion of IGD in DSM-5 and GD in ICD-11, the debate regarding the definition, diagnosis, and treatment of Internet and gaming addiction is still ongoing (20–26).
One issue is the distinction between Internet addiction disorder and IGD. Generally, Internet gaming-related addictive behaviors have been considered as a subtype of Internet addiction. Young (27) introduced the concept of Internet addiction with five subtypes—one of which was computer addiction with obsessive gaming. Young (28) later redefined Internet addiction as an impulse control disorder and specified excessive gaming as one of three subtypes of Internet addiction. In practice, however, the terms Internet addiction and IGD have been used interchangeably (29) and research studies have presented contradictory results. Tiego et al. (30) reported that the pattern of problematic Internet usage was represented by the level of overall severity rather than severity of a specific Internet activity. On the other hand, Király et al. (31) argued that problematic Internet use and problematic Internet gaming are different. Their results showed that problematic Internet use showed associations with all kinds of Internet activities while problematic Internet gaming was correlated with Internet gaming only. More recently, a systematic review by Burleigh et al. (32) suggested that Internet addiction and GD could be different constructs as they showed differently altered brain activities.
Another debate is about the difference between Internet gaming by PC and smartphone. In the past, IGD studies focused heavily on computer games (33). However, with the introduction of a smartphone and its global popularity, users of smartphone Internet games or mobile games increased dramatically. The total revenue for the mobile game industry was $86.3 billion in 2020, which increased seven times since 2012 (34). In 2020, 70.5% of South Koreans played at least one type of games, and 91.1% of gamers played mobile games (16). Compared to PC Internet games, mobile games have distinct characteristics such as accessibility and availability (35), as well as the purpose and the context of playing (36). While some scholars argue that mobile games have potential risks of social isolation and losing control similar to traditional videogames (36), results from related studies are inconsistent. In a study by Lopez-Fernandez et al. (37), one-third of participants used mobile games and using mobile games did not predict problematic usage. Also, Paik et al. (38) compared the prevalence of IGD among gamers by PC, smartphone, and both. The results indicated that gamers that used both media showed higher IGD prevalence and psychological symptoms while smartphone-only gamers had the lowest IGD prevalence. However, Liu et al. (39) presented that mobile gaming and the frequency of smartphone usage were significantly associated with smartphone addiction.
Whether time spent on the Internet and Internet gaming is a meaningful indicator of addictive behavior is also an important discussion. Although more time spent on the Internet and Internet gaming does not directly lead to addictive behaviors, the association between time spent and addictive behaviors using the Internet has been frequently reported. In the pathology model, scholars [(Chou and Hsiao, 2000; Chou et al., 1998; Hur, 2006; Morahan-Martin, 1999, (27) as cited in Tokunaga and Rains (40)] suggest that problematic Internet use is considered as a clinical pathology that psychological problem increases the time spent on the Internet which ultimately leads to problematic Internet usage (40). On the other hand, the deficient self-regulation perspective (41) suggests that amount of time spent is rather an outcome of problematic Internet usage (40). As these two models explained how problematic Internet usage is related to time spent on the Internet, the results from Tokunaga and Rains (40) showed that problematic Internet use significantly predicted time spent using the Internet. Similarly, in the study of Rho et al. (42), weekday game time was a risk factor for IGD, and in Laconi et al. (43), time spent on the Internet was a significant predictor of IGD score. Also, there was a strong correlation between time spent playing online games and IGD compared to offline games (44) and time spent on online gaming was associated with negative consequences (45). However, the results of more recent studies were inconclusive, with one study in China (46) finding a substantial link between IGD and daily gaming time, but another in South Korea finding only a limited relationship (47).
At the face of an increased risk for developing addictive Internet and Internet gaming behavior worldwide, the present study investigated into the latent profiles of South Korean adolescents in terms of their time spent on the Internet by PC and smartphone and Internet gaming by PC and smartphone. The purpose of the present study is to explore if unique patterns of Internet media usage exist, and if those patterns differ depending on the type of media (Internet vs. game) and device used (PC vs. smartphone). The present study consists of two parts. First, a latent profile analysis of usage patterns will be performed based on the time spent on the Internet by PC and smartphone as well as the time spent on Internet gaming by PC and smartphone. Second, to validate the uniqueness of the identified latent profiles, differences in problematic game usage, sex, and neuroticism will be examined as predictors of profile membership, as differences among those factors have previously been reported (48–52).
The data used in this study were secondary data obtained from a national survey conducted by the National Youth Policy Institute. For the survey, elementary (grades 4–6) and middle school students (grades 7–9) from all seven provinces of South Korea were recruited using quota sampling based on the 2020 Statistical Yearbook of Education published by the Ministry of Education. The data were collected in person by a survey agency from November 2020 to December 2020. In total, 1,500 responses from elementary students and 1,649 responses from middle-school students were collected. There was no evidence of systematic missing. Also, because the number of missing responses was not significant, listwise deletion was used. After the deletion, a total of 2,984 responses were analyzed. The mean age of the participants was 13.6 years, and 48.1% of them were female.
The time spent on four Internet-related activities was measured by self-reported open-ended questions: time spent on the Internet using PC, time spent on the Internet gaming using PC, time spent on smartphone except gaming, and time spent on Internet gaming using smartphone. The participants were asked to write down the average amount of time (in hours and minutes) spent on four activities during a weekday within the last 3 months. All the responses were converted into minutes. Although the participants were asked to answer using any possible natural numbers, the vast majority of responses were answered by an increment of 30 min. For the analysis, responses of 0 min were coded as 0, ≤ 30 min as 0.5, over 30 min and ≤ 60 min as 1, and so on.
Problematic game usage was measured by the Maladaptive Game Use Scale developed by the Korea Creative Content Agency (53). The scale measures the negative consequences of game usage within the past 1 year. The scale consists of 21 items measured on a four-point Likert scale, ranging from 1 (strongly disagree) to 4 (strongly agree). The scale has seven factors: withdrawal, tolerance, excessive usage, control impairment, compulsive usage, neglecting daily activity, and gaming despite negative consequences. Having a score of 9 or higher on three or more factors would be classified as “problematic usage.” The scale showed high internal consistency (Cronbach's α = 0.92) as well as acceptable construct validity, convergent validity, and concurrent validity (53). For the present study, the internal consistency was 0.94 for the total scale and 0.65 to 0.90 for the subscales.
Neuroticism was measured using two items on a four-point Likert scale ranging from 1 (strongly disagree) to 4 (strongly agree). Two items inquired about the frequency of mood fluctuations and depression symptoms. Higher scores indicate higher neuroticism.
Because there are no strong hypotheses about how people spend their time on the Internet, the aim of the present study was to identify unique latent groups (54). Thus, latent profile analysis (LPA) was performed to investigate the number of unique patterns on the Internet and Internet game usage time by PC and smartphone among adolescents in South Korea. To determine the optimum number of latent profiles, fit indices such as Akaike's information criterion (AIC), Bayesian information criterion, sample-size adjusted BIC (SABIC), entropy, and likelihood ratio tests such as Lo–Mendell–Rubin adjusted likelihood ratio test (LMR-LRT) and bootstrap likelihood ratio test (BLRT) were considered (55, 56). Also, parsimony, simplicity, clarity, and interpretability (56) were considered in identifying the number of profiles.
To validate the profiles, multinomial regression analysis and one-way analysis of covariance were performed. Multinomial analysis was conducted on sex and “problematic usage” to predict class membership using the R3STEP procedure (57). One-way analysis of covariance (ANCOVA) was performed after controlling for sex and school level to compare problematic game usage among profiles. Bonferroni correction was applied to adjust for comparing multiple profiles.
LPA and multinomial regression were conducted using Mplus 8.2 software, and one-way ANCOVA was conducted using SPSS 22.0 software.
Mean, standard deviation, and correlation analyses were performed, and the results are presented in Tables 1, 2. Adolescents spent an average of 2.16 h per day on their smartphones and 1.08 h per day on PC Internet games. Being a male correlated positively with the total MGUS score and time spent playing Internet games on a PC and negatively with neuroticism and smartphone usage time. Neuroticism showed a significant correlation with the total MGUS score, time spent on the Internet by PC, mobile gaming by smartphone, and smartphone use.
LPA was performed to determine if heterogeneous profiles of Internet and Internet game usage time by different devices exist among adolescents in South Korea. Based on previous studies about the possible subtypes of Internet and Internet game addiction, six to seven classes were expected to emerge. To determine the number of profiles, fit indices such as AIC, BIC, SABIC, entropy, and likelihood ratio tests such as LMR-LRT and BLRT were compared from unitary solution until the likelihood ratio tests were not significant. The LMR-LRT was not significant after nine profiles but AIC, BIC, SABIC, and BLRT reported improved model fit after nine profiles (see Table 3). The entropy for all nine profiles was above 0.8, ranging from 0.87 to 0.90.
After the analysis of fit indices, theoretical and conceptual considerations were taken into account when deciding on the number of profiles. Specifically, six, seven, and eight solution models were compared for parsimony and interpretability. In the eight-solution model, despite the best statistical fit, no meaningful distinction could be made between class 2 and class 6. These two were merged into a single class in the seven-solution model. In the seven-solution model, four classes represented different levels of general usage time while three classes represented high usage time in a specific content. In the six-solution model, class 5 and class 7 of the seven-solution model which showed meaningfully different usage patterns were merged into a single class. Thus, the model with seven profiles was selected and further analyzed. Seven profiles consisted of 147, 1,736, 295, 153, 113, 473, and 67 adolescents each, and its descriptive statistics are presented in Table 4.
Similar to previous research, four profiles showed generalized usage patterns with different intensity while the other three profiles showed very high usage time in a single or specific context. The first profile (4.93%) displayed the longest time spent on mobile games and using a smartphone, but spent the lowest time on games and the Internet using PC. This profile was named “Smartphone User.” The second profile (58.18%) was named as “Casual User” with short usage time on all four measures. The third profile (9.89%) showed a high usage time on the Internet using both PC and smartphone, very little usage of the PC Internet game, and average usage of mobile game. This profile was named “Internet User.” The fourth profile (5.13%) was named “PC Internet Gamer” with a very high usage time on the PC Internet game and average usage time on other three measures. The fifth profile (3.79%), named “Heavy User,” displayed a high usage time on all four measures. The sixth profile (15.85%) displayed an average usage time on all four measures and was named “Moderate User.” The last profile (2.25%) showed the highest usage time on the Internet and Internet game using PC, and very high usage in both mobile game and smartphone. This profile was named “Excessive User.”
After the identification of seven meaningful profiles of the Internet and Internet game usage time, the association between sex and the profiles was examined. In Table 5, the odd ratio and statistical significance of being classified into other profiles against the “Casual User” profile are shown. The results indicated that being a male means a higher chance of being classified into the “PC Internet Gamer” [OR = 6.54, p < 0.001], “Heavy User” [OR = 2.72, p = 0.006], and “Moderate User” [OR = 4.94, p < 0.001] profiles compared to the “Casual User” profile. Also, males were more likely to be classified as “Excessive User” [OR = 2.21, p = 0.051] than “Causal User” with a marginal significance.
The differences in problematic game usage among seven profiles were tested using one-way ANCOVA after controlling for the effect of sex and school level. The results are presented in Tables 6, 7. The main effect of profile membership was significant (F = 44.861, p < 0.001). The post-hoc test indicated that the scores of “Casual User” and “Internet User” were the smallest and that those of “Smartphone user” and “Moderate User” were lower than those of “PC Internet Gamer,” “Heavy User,” and “Excessive user” profiles. The scores of “Excessive User” were higher than those of “Heavy User” and “PC Internet Gamer” (p < 0.05).
In neuroticism, the main effect was significant (F = 8.169, p < 0.001). The post-hoc test indicated that the neuroticism scores of “Excessive User,” “Internet User,” and “Smartphone User” were significantly higher compared to the scores of “Casual User” and “Moderate User” (p < 0.05). The results are presented in Tables 8, 9.
With the heightened risk of addictive behaviors related to the Internet and Internet gaming among adolescents due to the COVID-19 pandemic, the present study investigated the latent profiles based on the Internet and Internet game usage time. Using LPA, seven profiles were identified and their associations with problematic game usage, sex, and neuroticism were examined. The majority (74.03%) of the participants had “Casual User” and “Moderate User” profiles, with below average or average time spent on all four areas (Internet on PC; Internet game on PC; Internet on smartphone; mobile game on smartphone). “Heavy User” (3.79%) and “Excessive User” (2.25%) profiles exhibited high or very high usage time in all four areas. “PC Internet Gamer” (5.13%), “Smartphone User” (4.93%), and “Internet User” (9.89%) profiles had a very high usage time for only a particular content. The proportion of the profiles with the potential risk of developing IGD was 6.04%. The proportion is comparable to the global IGD prevalence of 1.96% (58) but lower than the IGD prevalence of adolescents reported in Germany [14.3%; (59)] and China [17%; (46)].
Among the seven identified profiles, only the “Internet User” profile reported high Internet usage time. Compared to the “Internet User” profile, all other profiles except “Casual User” profile showed equal or greater usage time in PC Internet game, mobile game, or both. In fact, when comparing profiles with higher gaming usage to profiles with lower gaming usage, profiles with higher gaming usage scored higher on problematic game usage. In addition, profiles with higher Internet usage scored higher in neuroticism compared to profiles with lower Internet usage. This result partly supports the argument of Griffiths and Pontes (29) that the Internet addiction disorder and GD should be considered as different concepts. The study from Király et al. (31) showed similar results where problematic online gaming was associated with a preference for online gaming only and not for any other online activities, and the association was much stronger for males. Similarly, our results indicated that four out of five profiles related to heavy gaming had a higher proportion of males. In brief, our study adds to a trend of studies that support that the Internet addiction and Internet game addiction are different phenomena and reprove the lingering tendency to use these two terms interchangeably. In South Korea, many preventive and intervention programs targeting Internet addiction and Internet gaming addiction for adolescents still do not distinguish the two phenomena and rather define Internet gaming addiction as a subtype of Internet addiction (60–64).
Problematic gaming or gaming addiction have been largely associated with being male (65, 66), and even a recent IGD prevalence study reported a higher ratio of IGD prevalence in male adolescents (52). However, the results of the present study suggest that more attention may be warranted for female adolescent gamers. As expected, the “PC Internet Gamer” profile comprised a very high male ratio, and three other gaming profiles (“Moderate user,” “Heavy User,” “Excessive User”) also had a high male ratio.
However, the “Smartphone User” profile had a much higher ratio of females. People included in this profile spent a great amount of time on a smartphone (8.63 h), and more than half of their smartphone usage was for gaming (4.93 h). Moreover, problematic game usage scores of the “Smartphone User” profile were significantly higher than those of “Causal User” and “Internet User” and were not significantly different from that of “Moderate user,” “Heavy User,” and “PC Internet Gamer” profiles, and lower compared to “Excessive User” profiles.
The females in the “Smartphone User” profile may not be classified as smartphone addicts on the outside, and interventions may target smartphone addiction only. However, these female smartphone users are likely to engage in problematic gaming. Studies have suggested that the negative consequences of gaming may be different for adolescent gamers by sex. For instance, the reward and lose processing within IGD activates in the opposite direction in males and females (66). In addition, recent brain studies revealed that there are differences in brain activity and features regarding Internet gaming (67, 68) in females that they could be more vulnerable to IGD (65). In the present study, the “Smartphone User” profile was one of three profiles (“Internet User” and “Excessive User”) with higher neuroticism scores. In accordance with the result of the present study and recent studies about female gamers, King and Potenza (69) argued that more attention about female gamers is required in both clinical and research fields as only limited numbers of studies have been conducted (69). However, because this study's findings were based on cross-sectional data, the directionality of relationships cannot be assumed, and confounding is a possibility.
The game industry and gaming culture have gone through drastic changes, especially in the realm of Internet gaming. Unlike in the past when the Internet was more common with few Internet gamers (31), today almost everyone plays games and increasingly more people are playing Internet games on smartphones than on PCs (34). In 2020, 58.5% of South Korean adolescent gamers played on PCs while 82.4% of them played on smartphones (16). In the present study, the average time spent on playing mobile games (1.76 h) was higher than the average time spent on gaming (1.08 h) and Internet surfing (1.51 h) using PCs.
Although the Internet gaming trend has moved from PCs to smartphones in South Korea, the Korean government policies, research studies, and intervention programs still place more focus on PC Internet games. Moreover, many gaming addiction studies in South Korea still apply the theoretical base and measures from the study of Young et al. (1999), which was groundbreaking back then but is now more than 20 years old (70).
More recently, the interaction of the person–affect–cognition–execution model (71) describes the addiction process as the interaction of context, reaction, and consequence of behaviors. Considering the difference between a mobile game and a PC Internet game, the context, purpose, and consequences of playing a mobile game and a PC Internet game may take different paths and necessitate different interventions. The bigger problem is that most Internet game studies and intervention programs in South Korea utilize Internet addiction measures rather than Internet game addiction scales (70), and they are not based on recent behavioral addiction models. Internet gaming research in South Korea must be updated with the changing industry and cultural trends.
There are three major limitations in the present study. First, there are measurement issues within the study. All measures were self-report measures, and the participants were asked to recall their time spent on the Internet and Internet gaming retrospectively. Compared to measuring the usage time directly by using the time-keeping application or software, self-reported and retrospective information may not be as reliable. Also, social desirability might have influenced the reported time spent on gaming. In South Korea, despite its prevalence, gaming is stigmatized within the society and often blamed as the cause of criminal acts as serious as murder incidents (70). Lastly, because the sampling procedure was not probability sampling, the representativeness of the sample may be undermined. However, the sample was based on the Statistical Yearbook of Education of the Ministry of Education in South Korea, which has sampling guidelines that aim for acceptable representativeness.
Second, the survey sought to measure problematic game usage but did not include Internet addiction-related scales. The results from the present study suggest that Internet addiction might be different from Internet gaming addiction in terms of problematic game usage and the sex ratio. However, a direct comparison using an Internet addiction scale and a problematic game usage scale may prove to be useful. In addition, ANCOVA results of the present study do not convey any directionality and causality between problematic game usage, sex, and neuroticism.
Lastly, the present study sought to investigate the Internet and gaming profiles of adolescents in South Korea during the COVID-19 pandemic. As different literatures suggest, many scholars warned about a heightened risk for developing behavior addiction such as Internet addiction and IGD. However, the current study did not provide any information or analysis that compares the Internet and gaming behaviors before the pandemic and during the pandemic. Thus, no implications can be made regarding the influence of the pandemic on these behaviors.
This study provides an analysis of Internet usage and Internet gaming behavior of a nationally representative sample in South Korea during the COVID-19 pandemic. The results from the present study partly support the idea that the IGD is a distinct disorder from Internet addiction. In addition, the findings support the notion that mobile gamers are different from PC Internet gamers. Lastly, this study provides a substantial support for creating a greater attention on female gamers in practice and research.
The data analyzed in this study is subject to the following licenses/restrictions: The datasets analyzed for this study cannot be made publicly available. The dataset is from the national survey by the National Youth Policy Institute in South Korea in 2020, and the authors do not have the right to release the dataset. Requests to access these datasets should be directed to Dongil Kim, ZGlraW1lZHVAc251LmFjLmty.
Ethical approval was not provided for this study on human participants because the present study used anonymized secondary data from the survey from the National Youth Policy Institute in South Korea. Written informed consent for participation was not required for this study in accordance with the national legislation and the institutional requirements.
DK: writing of the first draft and final draft of the manuscript, acquisition of data, interpretation of analysis, concept and design of the research, and final approval for publication. JL: writing of the first draft and final draft of the manuscript, analysis and interpretation of data, concept and design of the research, and final approval for publication. JN: writing of the final draft of the manuscript, interpretation of data, and final approval for publication.
The authors declare that the research was conducted in the absence of any commercial or financial relationships that could be construed as a potential conflict of interest.
All claims expressed in this article are solely those of the authors and do not necessarily represent those of their affiliated organizations, or those of the publisher, the editors and the reviewers. Any product that may be evaluated in this article, or claim that may be made by its manufacturer, is not guaranteed or endorsed by the publisher.
This work was supported by the Ministry of Education of Republic of Korea and the National Research Foundation of Korea (NRF-2020S1A3A2A02103411).
1. Sun Y, Li Y, Bao Y, Meng S, Sun Y, Schumann G, et al. Increased addictive internet and substance use behavior during the COVID-19 pandemic in China. Am J Addict. (2020) 29:268–70. doi: 10.1111/ajad.13066
2. Perez M. Video Games Are Being Played at Record Levels as the Coronavirus Keeps People Indoors. (2020). Available online at: https://www.forbes.com/sites/mattperez/2020/03/16/video-games-arebeing-played-at-record-levels-as-the-coronavirus-keepspeople-indoors/#70eb644e57ba
3. Narusk K. The Impact of Lockdown on Mobile Gamers Behaviors. (2020). Available online at: https://medium.com/googleplaydev/the-impact-of-lockdown-on-mobile-gamers-behaviors-dffb05f07c4e
4. Javed J. eSports and Gaming Industry Thriving as Video Games Provide Escape From Reality During Coronavirus Pandemic. (2020). Available online at: https://www.wfaa.com/article/sports/esports-gaming-industry-thriving-as-video-gamesprovide-escape-from-reality-during-coronavirus-pandemic/287-5953d982-d240-4e2b-a2ba-94dd60a8a383
5. Kar SK, Arafat SY, Sharma P, Dixit A, Marthoenis M, Kabir R. COVID-19 pandemic and addiction: current problems and future concerns. Asian J Psychiatry. (2020) 51:102064. doi: 10.1016/j.ajp.2020.102064
6. Király O, Potenza MN, Stein DJ, King DL, Hodgins DC, Saunders JB, et al. Preventing problematic internet use during the COVID-19 pandemic: consensus guidance. Compre Psychiatry. (2020) 100:152180. doi: 10.1016/j.comppsych.2020.152180
7. Pfefferbaum B, North CS. Mental health and the Covid-19 pandemic. N Engl J Med. (2020) 383:510–2. doi: 10.1056/NEJMp2008017
8. Reger MA, Stanley IH, Joiner TE. Suicide mortality and coronavirus disease 2019—a perfect storm?. JAMA Psychiatry. (2020) 77:1093–4. doi: 10.1001/jamapsychiatry.2020.1060
9. Volkow ND. Collision of the COVID-19 and addiction epidemics. Ann Intern Med. (2020) 173:61–2. doi: 10.7326/M20-1212
10. Gong S, Li LZ, Wang S. Youth mental health before and after the control of the coronavirus disease 2019: a nationally representative cohort study of Chinese college students. J Affect Disord Rep. (2021) 3:100066. doi: 10.1016/j.jadr.2020.100066
11. Hu Y, Qian Y. COVID-19 and adolescent mental health in the United Kingdom. J Adolesc Health. (2021) 69:26–32. doi: 10.1016/j.jadohealth.2021.04.005
12. King DL, Delfabbro PH, Billieux J, Potenza MN. Problematic online gaming and the COVID-19 pandemic. J Behav Addict. (2020) 9:184–6. doi: 10.1556/2006.2020.00016
13. Chen IH, Chen CY, Pakpour AH, Griffiths MD, Lin CY. Internet-related behaviors and psychological distress among schoolchildren during COVID-19 school suspension. J Am Acad Child Adolesc Psychiatry. (2020) 59:1099–02.e1. doi: 10.1016/j.jaac.2020.06.007
14. Giardina A, Di Blasi M, Schimmenti A, King DL, Starcevic V, Billieux J. Online gaming and prolonged self-isolation: evidence from Italian gamers during the COVID-19 outbreak. Clin Neuropsychiatry. (2021) 18:65–74. doi: 10.36131/cnfioritieditore210106
15. Zhu S, Zhuang Y, Lee P, Li JCM, Wong PW. Leisure and problem gaming behaviors among children and adolescents during school closures caused by COVID-19 in Hong Kong: quantitative cross-sectional survey study. JMIR Seri Games. (2021) 9:e26808. doi: 10.2196/26808
16. Korea Creative Content Agency. 2020 Gamer Report. (2020). Available online at: https://www.kocca.kr/cop/bbs/view/B0000147/1842858.do?searchCnd=&searchWrd=&cateTp1=&cateTp2=&useAt=&menuNo=201825&categorys=0&subcate=0&cateCode=&type=&instNo=0&questionTp=&uf_Setting=&recovery=&option1=&option2=&year=&categoryCOM062=&categoryCOM063=&categoryCOM208=&categoryInst=&morePage=&delCode=0&qtp=&pageIndex=1#
17. Kuss DJ, Griffiths MD, Karila L, Billieux J. Internet addiction: A systematic review of epidemiological research for the last decade. Curr Pharm Des. (2014) 20:4026–52. doi: 10.2174/13816128113199990617
18. Griffiths M. A ‘components' model of addiction within a biopsychosocial framework. J Subst Use. (2005) 10:191–7. doi: 10.1080/14659890500114359
19. Cerniglia L, Griffiths MD, Cimino S, De Palo V, Monacis L, Sinatra M, et al. A latent profile approach for the study of internet gaming disorder, social media addiction, and psychopathology in a normative sample of adolescents. Psychol Res Behav Manage. (2019) 12:651–9. doi: 10.2147/PRBM.S211873
20. King DL, Delfabbro PH, Potenza MN, Demetrovics Z, Billieux J, Brand M. Logic, evidence and consensus: towards a more constructive debate on gaming disorder. Austra N Z J Psychiatry. (2019) 53:1047–9. doi: 10.1177/0004867419864435
21. Griffiths MD, Van Rooij AJ, Kardefelt-Winther D, Starcevic V, Király O, Pallesen S, et al. Working towards an international consensus on criteria for assessing Internet gaming disorder: A critical commentary on Petry et al. (2014). Addiction. (2016) 111:167–75. doi: 10.1111/add.13057
22. Kuss DJ, Griffiths MD, Pontes HM. Chaos and confusion in DSM-5 diagnosis of internet gaming disorder: issues, concerns, and recommendations for clarity in the field. J Behav Addict. (2017) 6:103–9. doi: 10.1556/2006.5.2016.062
23. Aarseth E, Bean AM, Boonen H, Colder Carras M, Coulson M, Das D, et al. Scholars' open debate paper on the world health organization ICD-11 gaming disorder proposal. J Behav Addict. (2017) 6:267–70. doi: 10.1556/2006.5.2016.088
24. Dullur P, Starcevic V. Internet gaming disorder does not qualify as a mental disorder. Austra N Z J Psychiatry. (2018) 52:110–1. doi: 10.1177/0004867417741554
25. Schimmenti A, Starcevic V. Logical fallacies in justifying problematic gaming as a mental disorder. Austra N Z J Psychiatry. (2019) 53:604–5. doi: 10.1177/0004867418821431
26. Pontes HM, Griffiths MD. A new era for gaming disorder research: Time to shift from consensus to consistency. Addict Behav. (2019) 103:106059. doi: 10.1016/j.addbeh.2019.106059
27. Young KS. Internet addiction: the emergence of a new clinical disorder. Cyberpsychol Behav. (1998) 1:237–44. doi: 10.1089/cpb.1998.1.237
28. Young K. Internet addiction: diagnosis and treatment considerations. J Contemp Psychother. (2009) 39:241–6. doi: 10.1007/s10879-009-9120-x
29. Griffiths MD, Pontes HM. Internet addiction disorder and internet gaming disorder are not the same. J Addict Res Ther. (2014) 5:e124. doi: 10.4172/2155-6105.1000e124
30. Tiego J, Lochner C, Ioannidis K, Brand M, Stein DJ, Yücel M, et al. Problematic use of the internet is a unidimensional quasi-trait with impulsive and compulsive subtypes. BMC Psychiatry. (2019) 19:348. doi: 10.1186/s12888-019-2352-8
31. Király O, Griffiths MD, Urbán R, Farkas J, Kökönyei G, Elekes Z, et al. Problematic internet use and problematic online gaming are not the same: findings from a large nationally representative adolescent sample. Cyberpsychol Behav Soc Netw. (2014) 17:749–54. doi: 10.1089/cyber.2014.0475
32. Burleigh TL, Griffiths MD, Sumich A, Wang GY, Kuss DJ. Gaming disorder and internet addiction: a systematic review of resting-state EEG studies. Addict Behav. (2020) 107:106429. doi: 10.1016/j.addbeh.2020.106429
33. Kuss DJ, Griffiths MD. Internet gaming addiction: a systematic review of empirical research. Int J Ment Health Addict. (2012) 10:278–96. doi: 10.1007/s11469-011-9318-5
34. Chiovato L. Hypercasual Games Introduced Millions of Consumers to the World of Gaming: What's Next. (2021). Available online at: https://newzoo.com/
35. Engl S, Nacke LE. Contextual influences on mobile player experience–A game user experience model. Entertain Comput. (2013) 4:83–91 doi: 10.1016/j.entcom.2012.06.001
36. Chen C, Leung L. Are you addicted to candy crush saga? An exploratory study linking psychological factors to mobile social game addiction. Telemat Inform. (2016) 33:1155–66. doi: 10.1016/j.tele.2015.11.005
37. Lopez-Fernandez O, Männikkö N, Kääriäinen M, Griffiths MD, Kuss DJ. Mobile gaming does not predict smartphone dependence: a cross-cultural study between Belgium and Finland. J Behav Addict. (2018) 7:88–99. doi: 10.1556/2006.6.2017.080
38. Paik SH, Cho H, Chun JW, Jeong JE, Kim DJ. Gaming device usage patterns predict internet gaming disorder: comparison across different gaming device usage patterns. Int J Environ Res Public Health. (2017) 14:1512. doi: 10.3390/ijerph14121512
39. Liu CH, Lin SH, Pan YC, Lin YH. Smartphone gaming and frequent use pattern associated with smartphone addiction. Medicine. (2016) 95:e4068. doi: 10.1097/MD.0000000000004068
40. Tokunaga RS, Rains SA. An evaluation of two characterizations of the relationships between problematic internet use, time spent using the internet, psychosocial problems. Hum Commun Res. (2010) 36:512–45. doi: 10.1111/j.1468-2958.2010.01386.x
41. LaRose R, Lin CA, Eastin MS. Unregulated internet usage: addiction, habit, or deficient self-regulation? Media Psychol. (2003) 5:225–53. doi: 10.1207/S1532785XMEP0503_01
42. Rho MJ, Lee H, Lee TH, Cho H, Jung DJ, Kim DJ, et al. Risk factors for internet gaming disorder: psychological factors and internet gaming characteristics. Int J Environ Res Public Health. (2018) 15:40. doi: 10.3390/ijerph15010040
43. Laconi S, Pirès S, Chabrol H. Internet gaming disorder, motives, game genres and psychopathology. Comput Hum Behav. (2017) 75:652–9. doi: 10.1016/j.chb.2017.06.012
44. Lemmens JS, Hendriks SJ. Addictive online games: examining the relationship between game genres and internet gaming disorder. Cyberpsychol Behav Soc Netw. (2016) 19:270–6. doi: 10.1089/cyber.2015.0415
45. Hellström C, Nilsson KW, Leppert J, Åslund C. Influences of motives to play and time spent gaming on the negative consequences of adolescent online computer gaming. Comput Hum Behav. (2012) 28:1379–87. doi: 10.1016/j.chb.2012.02.023
46. Liao Z, Huang Q, Huang S, Tan L, Shao T, Fang T, et al. Prevalence of internet gaming disorder and its association with personality traits and gaming characteristics among Chinese adolescent gamers. Front Psychiatry. (2020) 11:1266. doi: 10.3389/fpsyt.2020.598585
47. Kim D, Lee J. addictive internet gaming usage among korean adolescents before and after the outbreak of the COVID-19 pandemic: a comparison of the latent profiles in 2018 and 2020. Int J Environ Res Public Health. (2021) 18:7275. doi: 10.3390/ijerph18147275
48. Mehroof M, Griffiths MD. Online gaming addiction: the role of sensation seeking, self-control, neuroticism, aggression, state anxiety, trait anxiety. Cyberpsychol Behav Soc Netw. (2010) 13:313–6. doi: 10.1089/cyber.2009.0229
49. Li H, Zou Y, Wang J, Yang X. Role of stressful life events, avoidant coping styles, and neuroticism in online game addiction among college students: a moderated mediation model. Front Psychol. (2016) 7:1794. doi: 10.3389/fpsyg.2016.01794
50. Buono FD, Paul E, Sprong ME, Smith EC, Garakani A, Griffiths MD. Gaming and gaming disorder: a mediation model gender, salience, age of gaming onset, and time spent gaming. Cyberpsychol Behav Soc Netw. (2020) 23:647–51. doi: 10.1089/cyber.2019.0445
51. Marciano L, Camerini AL, Schulz PJ. Neuroticism in the digital age: a meta-analysis. Comput Hum Behav Rep. (2020) 2:100026. doi: 10.1016/j.chbr.2020.100026
52. Yu Y, Mo PK, Zhang J, Li J, Lau JT. Why is internet gaming disorder more prevalent among Chinese male than female adolescents? The role of cognitive mediators. Addict Behav. (2021) 112:106637. doi: 10.1016/j.addbeh.2020.106637
53. Korea Creative Content Agency. Comprehensive Scale For Assessing Game Behavior Manual. (2010). Available online at: https://www.kocca.kr/knowledge/research/__icsFiles/afieldfile/2010/08/18/uXS53sbDSI3a.pdf
54. Finch WH, Bronk KC. Conducting confirmatory latent class analysis using M plus. Struct Equat Model. (2011) 18:132–51. doi: 10.1080/10705511.2011.532732
55. Tein JY, Coxe S, Cham H. Statistical power to detect the correct number of classes in latent profile analysis. Struct Equat Model Multidiscipl J. (2013) 20:640–57. doi: 10.1080/10705511.2013.824781
56. Spurk D, Hirschi A, Wang M, Valero D, Kauffeld S. Latent profile analysis: a review and “how to” guide of its application within vocational behavior research. J Vocat Behav. (2020) 120:103445. doi: 10.1016/j.jvb.2020.103445
57. Asparouhov T, Muthén B. Auxiliary variables in mixture modeling: Three-step approaches using M plus. Struct Equat Model Multidiscipl J. (2014) 21:329–41. doi: 10.1080/10705511.2014.915181
58. Stevens MW, Dorstyn D, Delfabbro PH, King DL. Global prevalence of gaming disorder: a systematic review and meta-analysis. Austra N Z J Psychiatry. (2020) 55:553–68. doi: 10.1177/0004867420962851
59. Wartberg L, Kriston L, Thomasius R. Internet gaming disorder and problematic social media use in a representative sample of German adolescents: prevalence estimates, comorbid depressive symptoms and related psychosocial aspects. Comput Hum Behav. (2020) 103:31–6. doi: 10.1016/j.chb.2019.09.014
60. Kim H, Cho M. Meta-analysis of internet addiction treatment programs for adolescents. Ment Health Soc Work. (2015) 43:89–118.
61. Shin S, Ryu S, Kim B, Lee D, Chung Y. The development and effectiveness of MI group counseling program for adolescents internet addiction. Korean J Counsel. (2015) 16:89–109. doi: 10.15703/kjc.16.4.201508.89
62. Ha J, Shin S. The effectiveness of youth internet addiction group counseling programs: a meta-analysis. Korean J Psychol Gen. (2016) 35:191–216. doi: 10.22257/kjp.2016.03.35.1.191
63. Kim D. A systematic review on intervention program of smartphone addiction. J Korea Acad Indust Cooperat Soc. (2020) 21:276–88. doi: 10.5762/KAIS.2020.21.3.276
64. Kim Y. Meaning and challenge of WHO ICD011 code 6C51(gaming disorder). Inha Law Reivew. (2020) 23:161–87. doi: 10.22789/IHLR.2020.03.23.1.161
65. Wang Z, Hu Y, Zheng H, Yuan K, Du X, Dong G. Females are more vulnerable to internet gaming disorder than males: evidence from cortical thickness abnormalities. Psychiatry Res Neuroimag. (2019) 283:145–53. doi: 10.1016/j.pscychresns.2018.11.001
66. Zhang J, Hu Y, Wang Z, Wang M, Dong GH. Males are more sensitive to reward and less sensitive to loss than females among people with internet gaming disorder: fMRI evidence from a card-guessing task. BMC Psychiatry. (2020) 20:357. doi: 10.1186/s12888-020-02771-1
67. Dong G, Wang L, Du X, Potenza MN. Gender-related differences in neural responses to gaming cues before and after gaming: implications for gender-specific vulnerabilities to internet gaming disorder. Soc Cogn Affect Neurosci. (2018) 13:1203–14. doi: 10.1093/scan/nsy084
68. Dong G, Wang Z, Wang Y, Du X, Potenza MN. Gender-related functional connectivity and craving during gaming and immediate abstinence during a mandatory break: implications for development and progression of internet gaming disorder. Prog Neuro Psychopharmacol Biol Psychiatry. (2019) 88:1–10. doi: 10.1016/j.pnpbp.2018.04.009
69. King DL, Potenza MN. Gaming disorder among female adolescents: a hidden problem?. J Adolesc Health. (2020) 66:650–2. doi: 10.1016/j.jadohealth.2020.03.011
70. Song D. Critical analysis of gaming disorder/addiction researches on neuroimages, measurement tools, research process. Korean Game Soc. (2019) 19:135–45. doi: 10.7583/JKGS.2019.19.1.135
71. Brand M, Wegmann E, Stark R, Müller A, Wölfling K, Robbins TW, et al. The interaction of person-affect-cognition-execution (I-PACE) model for addictive behaviors: update, generalization to addictive behaviors beyond internet-use disorders, and specification of the process character of addictive behaviors. Neurosci Biobehav Rev. (2019) 104:1–10. doi: 10.1016/j.neubiorev.2019.06.032
Keywords: Internet gaming, adolescents, Internet addiction, South Korea, sex, mental health
Citation: Kim D, Lee J and Nam JK (2021) Latent Profile of Internet and Internet Game Usage Among South Korean Adolescents During the COVID-19 Pandemic. Front. Psychiatry 12:714301. doi: 10.3389/fpsyt.2021.714301
Received: 25 May 2021; Accepted: 23 August 2021;
Published: 23 September 2021.
Edited by:
Cecilia Cheng, The University of Hong Kong, Hong Kong, Hong Kong, SAR ChinaReviewed by:
Lu Yu, Hong Kong Polytechnic University, Hong Kong, Hong Kong, SAR ChinaCopyright © 2021 Kim, Lee and Nam. This is an open-access article distributed under the terms of the Creative Commons Attribution License (CC BY). The use, distribution or reproduction in other forums is permitted, provided the original author(s) and the copyright owner(s) are credited and that the original publication in this journal is cited, in accordance with accepted academic practice. No use, distribution or reproduction is permitted which does not comply with these terms.
*Correspondence: Junwon Lee, c3luY21hc3Rlcjd4QHNudS5hYy5rcg==
Disclaimer: All claims expressed in this article are solely those of the authors and do not necessarily represent those of their affiliated organizations, or those of the publisher, the editors and the reviewers. Any product that may be evaluated in this article or claim that may be made by its manufacturer is not guaranteed or endorsed by the publisher.
Research integrity at Frontiers
Learn more about the work of our research integrity team to safeguard the quality of each article we publish.