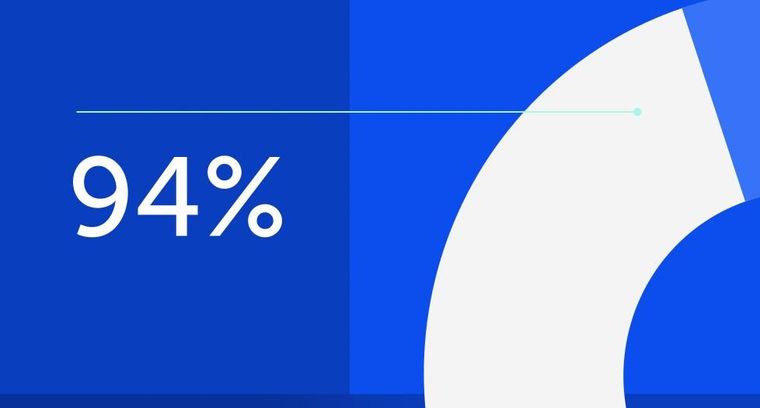
94% of researchers rate our articles as excellent or good
Learn more about the work of our research integrity team to safeguard the quality of each article we publish.
Find out more
SYSTEMATIC REVIEW article
Front. Psychiatry, 06 September 2021
Sec. Social Psychiatry and Psychiatric Rehabilitation
Volume 12 - 2021 | https://doi.org/10.3389/fpsyt.2021.691251
This article is part of the Research TopicShared Decision Making in Mental Health: International Perspectives on ImplementationView all 13 articles
Background: Shared decision-making (SDM) in mental healthcare has received increased attention as a process to reinforce person-centered care. With the rapid development of digital health technology, researchers investigate how digital interventions may be utilized to support SDM. Despite the promise of digital interventions to support SDM, the effect of these in mental healthcare has not been evaluated before. Thus, this paper aims to assess the effect of SDM interventions complimented by digital technology in mental healthcare.
Objective: The objective of this review was to systematically examine the effectiveness of digital SDM interventions on patient outcomes as investigated in randomized trials.
Methods: We performed a systematic review and meta-analysis of randomized controlled trials on digital SDM interventions for people with a mental health condition. We searched for relevant studies in MEDLINE, PsycINFO, EMBASE, CINAHL, and the Cochrane Central Register of Controlled Trials. The search strategy included terms relating to SDM, digital systems, mental health conditions, and study type. The primary outcome was patient activation or indices of the same (e.g., empowerment and self-efficacy), adherence to treatment, hospital admissions, severity of symptoms, and level of functioning. Secondary outcomes were satisfaction, decisional conflict, working alliance, usage, and adherence of medicine; and adverse events were defined as harms or side effects.
Results: Sixteen studies met the inclusion criteria with outcome data from 2,400 participants. Digital SDM interventions had a moderate positive effect as compared with a control condition on patient activation [standardized mean difference (SMD) = 0.56, CI: 0.10, 1.01, p = 0.02], a small effect on general symptoms (SMD = −0.17, CI: −0.31, −0.03, p = 0.02), and working alliance (SMD = 0.21, CI: 0.02, 0.41, p = 0.03) and for improving decisional conflict (SMD = −0.37, CI: −0.70, −0.05, p = 0.02). No effect was found on self-efficacy, other types of mental health symptoms, adverse events, or patient satisfaction. A total of 39 outcomes were narratively synthesized with results either favoring the intervention group or showing no significant differences between groups. Studies were generally assessed to have unclear or high risk of bias, and outcomes had a Grading of Recommendations Assessment, Development and Evaluation (GRADE) rating of low- or very low-quality evidence.
Conclusions: Digital interventions to support SDM may be a promising tool in mental healthcare; but with the limited quality of research, we have little confidence in the estimates of effect. More quality research is needed to further assess the effectiveness of digital means to support SDM but also to determine which digital intervention features are most effective to support SDM.
Systematic Review Registration: PROSPERO, identifier CRD42020148132.
Digital health technology has become an integrated part of the global healthcare system and is continuously developing and growing. Within mental healthcare, traditional means of care are being complemented by health technology such as smartphone decision aids, web-based self-management systems, or online support groups. As technology develops, new possibilities arise; and the World Health Organization advocated through the global strategy on digital health for 2020–2024 to use digital technology for more person-centered healthcare (1). Person-centered care focuses on placing people at the center of their healthcare, and technology may complement this in various ways such as supporting people to become more aware of their health and needs.
Researchers within mental healthcare have increasingly turned their attention to shared decision-making (SDM) as a process to reinforce person-centered care (2). SDM can be defined as a process involving at least two people (e.g., patient and provider) who share information, discuss options, and collaborate to reach a mutual decision (3). SDM aims to ensure that both patient and provider are actively involved in decision-making processes and that their unique competences are utilized. Providers have an expertise in information on symptoms management, treatment options, and potential benefits or side effects; while patients are experts on their needs, preferences, goals, and values. SDM may be affected by mechanics surrounding the patient and provider such as their individual engagement, working alliance, and mutual understanding of one another but also the risk associated with the decision. If done successfully, SDM may increase autonomy, self-management, working alliance, satisfaction, and quality of care (4). For SDM to be successful, both the patient and provider must be engaged in the patients' care. Patients have indicated that being an active partner and embracing the same qualities as one would expect from their provider is necessary for the success of SDM (e.g., honesty, responsibility, and trust) (3). Patients in mental healthcare have also indicated that they want to be active participants when making health decisions (5). Still, SDM has not been widely implemented in clinical practice with barriers such as time constraints at consultations, providers believing they can guess how the patients wish to be involved, or uncertainty of how to fit SDM into the workflow (2, 6). In addition, recent research notes that SDM may be easier to incorporate when making a decision has a low personal risk and may be more difficult to incorporate when decisions have a higher risk such as adjusting one's medication (7). A systematic review—covering 33 studies on including patients in decision-making—reported that a minority of healthcare providers consistently attempted to facilitate patient involvement, and even fewer adjust care to patient preferences (6). The review highlights SDM interventions as a means to promote patient-involving behaviors but that the responsibility of facilitating SDM cannot lie solely with the provider—decision aids and communication tools may serve as part of the solution (6). Therefore, to facilitate the process of SDM, research has begun to investigate how digital interventions may be utilized to support SDM and potentially address some of its barriers. Using technology as the tool and SDM as the process, randomized controlled trials (RCTs) are investigating whether digital SDM interventions are effective at promoting person-centered care.
Previous systematic reviews and meta-analyses have found that SDM interventions have a small effect on empowerment for people with psychosis and that SDM may increase provider facilitation of patient involvement (8, 9), while meta-analyses on the effect of digital interventions for mental health have found an effect at improving symptoms (10). However, a systematic assessment of the effect of digital interventions to support SDM in mental healthcare has not been conducted before. Thus, this paper aims to assess the effect of SDM interventions complimented by digital technology in mental healthcare for promoting person-centered care. Using subgroup analyses, we explored whether the effect was dependent on the type of digital intervention, age, or mental health condition. The results of these meta-analyses may guide future research and stakeholders in how digital technology may complement SDM in mental healthcare.
This systematic review and meta-analysis followed the PRISMA statement and adhered to the registered online protocol at PROSPERO (CRD42020148132) (11).
We defined SDM in this review as a process with three main components: (1) sharing information; (2) discussing treatment options; and (3) reaching a mutual decision that both parties can agree upon. Around these three components lie several surrounding mechanisms affecting this process such as learning about the patient, supporting the patient to initiate discussions with the provider, or evaluating the decision. The process of SDM and its surrounding mechanisms is illustrated in Supplementary Material 1. This definition is based on previous research investigating patients' understanding of SDM and also a systematic review on the most common components of SDM models (3, 12). In this review, an SDM intervention is an intervention that supports at least one of the three main components of the SDM process.
Digital interventions were defined as information and/or communication technology delivered via phones, computers, personal digital assistants, or other similar devices. Interventions did not have to be internet-based.
Mental health conditions were defined in concordance to the Diagnostic and Statistical Manual of Mental Disorders (DSM) and International Classification of Diseases (ICD).
We conducted a systematic literature search of the following databases up to March 2021: MEDLINE, CINAHL, EMBASE, PsycINFO, and the Cochrane Central Register of Controlled Trials. The PICO framework was used to develop the search strategy. Our search terms focused on SDM, digital health technology, mental health, and RCTs. The complete search strategy is listed in the Supplementary Materials. Our search terms on SDM were developed based on existing Cochrane reviews on SDM (9, 13). Due to the complexity of SDM, our search terms focused on person-centered terms (e.g., patient involvement), technique style (e.g., decision aids), and relationship components (e.g., working alliance). Search terms on digital health technology focused on components such as e-Health, m-Health, and information technology. Search terms on mental health were broad and attempted to reach all mental health conditions. The reference lists of retrieved studies were checked to identify further eligible studies.
Only RCTs presenting original data were included in the review. For a study to be included in the review, 50% of the participants needed to have a mental health condition as defined by the DSM and ICD. Besides having a mental health condition, there were no restrictions regarding clinical or demographic characteristics of the participants. Exclusion criteria were studies focusing on relatives rather than the patient or provider. For an intervention to be included, it had to cover one of the three main components of SDM and use a digital tool for people with a mental health condition (as defined above).
The search strategy was developed by the author TV and approved by CH and LK (see Supplementary Material 2 for the search string). All identified studies were extracted and exported into Zotero reference manager software by TV. All identified studies were title screened by TV against inclusion/exclusion criteria to determine eligibility for selection. The abstract screen and full-text assessments were independently performed by CH and TV with a 74% agreement. In case of disagreements, a third reviewer (LK) was included in the discussion.
For included studies, the following data were extracted by TV into predefined tables: year of publication, sample size, mental health condition, type of intervention, duration of intervention, type of outcome, results (number of events, means, and SD), control condition, type of setting, and baseline demographics (age, gender, and the highest educational level). The authors of the retrieved papers were contacted if clarification was needed or if data were not accessible from the article.
All analyses were conducted by Review Manager 5.3.5, using random-effects model to account for heterogeneity. The total difference in changes on measurements for patient activation or indices of the same between digital interventions and controls were pooled to compute the overall effect size of the digital interventions with 95% confidence intervals. TV and CH assessed the risk of bias in the included studies using Cochrane Collaboration's Risk of Bias tool. This tool assesses studies six areas, ranking each area as high, low, or unknown for risk of bias. The areas are sequence generation, allocation sequence concealment, blinding of participants and personnel, blinding of outcome assessment, incomplete outcome data, and selective outcome reporting. In addition, TV and CH used the Grading of Recommendations Assessment, Development and Evaluation (GRADE) system to assess the quality of evidence of each outcome by downgrading from high by one level for each serious issue identified in the domains: risk of bias, inconsistency, indirectness, imprecision, and publication bias (14). In case of a disagreement on the assessment of the studies' risk of bias and the outcomes' GRADE score, a third reviewer was included in the discussion. As stated in our protocol, subgroup analysis was performed for type of intervention (web-based, PC software, or smartphone/tablet application) and diagnosis (11). However, due to discrepancies in the length of the interventions, subgroup analysis on the duration of intervention was also included and was divided into short-term (<3 months) and long-term interventions (>3 months). The cutoff at 3 months was chosen to ensure that trials would be divided somewhat equally and that subgroup analysis on duration would be feasible. For outcomes only occurring in one trial or outcome data not appropriate for a meta-analysis (and where the corresponding author was unable to assist), we did a narrative synthesis. The synthesis was done by counting the numbers of trials reporting a significant positive effect, no effect, and a negative effect for each outcome.
Decisional conflict was not originally planned as an outcome for our meta-analysis according to our protocol; however, after discovering several of the studies measuring this area and considering its relation to SDM, it was included (15–18).
The search resulted in 1,911 references, 1,098 after duplicates were removed and 277 after title screening. After abstract screen and full-text assessment, 12 articles were assessed as eligible. Four additional articles were included after reading protocols from the initial search where authors of unpublished results were contacted. Thus, 16 RCTs investigating digital SDM interventions within mental health were included in the meta-analysis. The progress of including and excluding articles is shown in the PRISMA flow diagram Figure 1.
Figure 1. PRISMA flow diagram (19).
Characteristics of the 16 study populations, aim, duration, comparison treatment, and outcome measures are presented in Table 1.
Overall, 2,400 participated in the 16 trials with a mean sample size of 150 ranging from 50 to 507 participants. Mean age for the participants was 40 years (SD = 8.5) ranging from 15 to 51 years. The proportion of females was 49% across all studies ranging from 14 to 100%. The mean duration of the interventions was approximately 3.4 months ranging from 1 day to 12 months. Because of this variation, studies were divided into either short-term intervention (3 months or less) or long-term intervention (more than 3 months): 11 trials were categorized as short-term interventions (15–18, 20–22, 24, 25, 27, 29) and five trials as long-term interventions (23, 26, 28, 30, 31). Seven studies investigated a system accessible through a website (15–18, 20–22), six studies investigated a computer software program (23–26, 29, 31), one study investigated a smartphone application (27), and two studies investigated a tablet application (28, 30). Half of the 16 studies reported using some form of clinician training and that the intervention was used in conjunction with the provider or a peer worker (16, 22–24, 26, 28, 30, 31). Studies that did not actively involve the clinicians mentioned, however, that patients were encouraged to talk with their provider about the intervention. Eleven studies mentioned an inclusion criterion of mental health condition, four studies mentioned recruiting from mental health services (15, 18, 24, 26), and one study mentioned recruiting from primary care; and a majority of their participants were assessed as depressed by the research team (29). Ten studies recruited exclusively or primarily participants with psychosis, schizophrenia, or related disorder (20–26, 28, 30, 31). Three studies recruited primarily participants with depression or depressive symptoms (17, 18, 29), one study recruited participants with a personality disorder (16), one study focused entirely on bipolar disorder (15), and one study only mentioned that participants were recruited from a mental health setting without specifying the type of mental health condition (27).
All included studies were assessed for risk of bias using the Cochrane Collaboration's Risk of Bias tool on the following domains: random sequence generation, allocation concealment, selective reporting, blinding of participants and personnel, blinding of outcome assessments (objective and subjective), attrition bias, and other bias. The risk of bias for each study is presented in Figure 2 showing that the most frequent risk factor for bias was blinding of participants and blinding of subjective outcome assessment. Furthermore, more than half of the studies were at high risk of bias in terms of attrition bias and other bias.
Results of the pooled effect size from digital SDM interventions on patient activation, self-efficacy, empowerment, and patient involvement with the individual effect of each intervention are presented in Figure 3. The random-effects meta-analysis revealed a moderate significant effect of digital SDM interventions to promote patient activation in comparison with a control group (two studies, N = 77, standardized mean difference (SMD) = 0.56, CI: 0.10, 1.01, p = 0.02) (27, 31). Variation across trials due to heterogeneity was not present (Chi2 = 0.68, p = 0.41, I2 = 0%). There was no significant effect on self-efficacy (three studies, N = 787, SMD = −0.02 CI: −0.16, 0.12, p = 0.74) (22, 29, 30). Variation across trials due to heterogeneity was not present (Chi2 = 0.55, p = 0.76, I2 = 0%). Meta-analysis on empowerment and patient involvement was not applicable due to only one study respectively reported data on these areas. Still, the results of these single studies are included in Figure 3, indicating no significant effect of digital SDM interventions on empowerment (SMD = 0.81, CI: −0.03, 1.65, p = 0.06) (27) or patient involvement (SMD = 0.01, CI: −0.29, 0.31, p = 0.95) (16). Due to differences between these aspects and the fact that one study measured both activation and empowerment, the total score in Figure 3 was removed to avoid study participants being counted twice.
Results of the pooled effect size from digital SDM interventions on symptoms together with the individual effect of each intervention are presented in Figure 4. The random-effects meta-analysis revealed a small significant effect of digital SDM interventions to improve general symptoms (three studies, N = 769, −0.17, CI: −0.31, −0.03, p = 0.02) (16, 23, 30) but revealed no effect on positive (two studies (same research group), N = 593, SMD = −0.15, CI: −0.31, 0.01, p = 0.07) (23, 30), negative symptoms [two studies (same research group), N = 594, SMD = −0.08, CI: −0.24, 0.08, p = 0.35] (23, 30), overall psychiatric symptoms (two studies, N = 103, SMD = −0.10, CI: −0.49, 0.29, p = 0.62) (22, 31), depressive symptoms (two studies, N = 403, SMD = 0.10, CI: −0.10, 0.30, p = 0.32) (18, 29), or anxiety (two studies, N = 166, SMD = −0.27, CI: −0.58, 0.04, p = 0.09) (15, 18). Due to differences between the types of symptoms and that studies appeared more than once, the total score in Figure 4 was removed to avoid study participants being counted twice.
Variation across trials due to heterogeneity was not present for positive symptoms (Chi2 = 0.23, p = 0.63, I2 = 0%), negative symptoms (Chi2 = 0.03, p = 0.87, I2 = 0%), overall psychiatric symptoms (Chi2 = 0.24, p = 0.63, I2 = 0%), or anxiety (Chi2 = 0.41, p = 0.52, I2 = 0%) but was present for general symptoms (Chi2 = 6.21, p = 0.04, I2 = 68%) and depressive symptoms (Chi2 = 3.32, p = 0.07, I2 = 70%). Egger's test was not performed due to the small sample of studies.
Results of the pooled effect size from digital SDM interventions on adverse events defined as harms or side effects together with the individual effect of each intervention are presented in Figure 5. Only measurements of side effects of medication were identified from the studies. The random-effects meta-analysis revealed no significant effect of digital SDM interventions to improve side effects induced by medication (two studies, N = 102, SMD = 0.08, CI: −0.31, 0.48, p = 0.67) (22, 31). Variation across trials due to heterogeneity was not present (Chi2 = 0.01, p = 0.93, I2 = 0%). Subgroup analysis for the primary outcomes showed no significant differences, which are included in the Supplementary Materials 3–8).
Results of the pooled effect size from digital SDM interventions on the secondary outcomes are presented in Figures 6–12. The random-effects meta-analysis revealed a small positive effect of digital SDM interventions for improving working alliance (four studies, N = 423, SMD = 0.21, CI: 0.02, 0.41, p = 0.03) (16, 28, 30, 31), a small-to-moderate positive effect for improving decisional conflict (four studies, N = 550, SMD = −0.37, CI: −0.70, −0.05, p = 0.02) (15–18), and no significant effect on patient satisfaction (seven studies, N = 465, SMD = 0.12, CI: −0.07, 0.30, p = 0.21) (21, 22, 24, 27, 28, 30, 31). Variation across trials due to heterogeneity was present for decisional conflict (Chi2 = 10.69, p = 0.01, I2 = 72%) but not for satisfaction (Chi2 = 2.48, p = 0.87, I2 = 0%) and working alliance (Chi2 = 2.96, p = 0.04, I2 = 0%). Assessment of publication bias was only performed for patient satisfaction due to the limited amount of studies for each pooled outcome. Via visual inspection of funnel plots, we did not assess any publication bias for patient satisfaction. Subgroup analysis on satisfaction by duration, type of intervention, and diagnosis showed no statistical significant differences between groups (Figures 6–8). Subgroup analysis on working alliance by duration, type of intervention, and diagnosis showed no statistical significant differences between groups (Figures 9–11). Subgroup analysis on decisional conflict by diagnosis showed a tendency for a greater effect for populations with symptoms of depression than other types of symptoms (two studies, N = 232, SMD = −0.61 CI: −0.94, −0.28, p = 0.0003; Figure 12). Subgroup analysis on decisional conflict by duration and type of intervention was not performed due to all studies being in the same subgroup.
The outcomes had a GRADE rating of very low quality for patient activation and low quality for self-efficacy, adverse events, symptoms, working alliance, decisional conflict, and patient satisfaction. This implies that we have little confidence in the estimates of effect for these outcomes.
Table 2 presents the results of the narrative synthesis of 39 outcomes. The results on these outcomes were mixed between showing no difference between the groups and favoring the intervention group. None of the studies found effects favoring the control group.
Our review on the effectiveness of digital SDM interventions in mental healthcare included 2,400 participants across 16 RCTs examining digital interventions to support SDM with the majority conducted on psychosis, schizophrenia, or similar disorders.
The main analysis found that digital SDM interventions led to a moderate significant effect on improving patient activation in mental healthcare but not on self-efficacy, empowerment, or subjective level of patient involvement. Such result could be that digital SDM tools are more effective at addressing the concepts of patient activation. However, only two small sampled studies investigated the effectiveness on patient activation, three studies on self-efficacy, and one study each on empowerment and patient-involvement. Therefore, more quality research on the effectiveness of SDM interventions on patient activation or indices of the same are greatly needed to investigate this further.
As for symptoms, the use of a digital SDM intervention had a significant effect on general symptoms but not on positive, negative, anxiety, depressive, or overall psychiatric symptoms. Still, subgroup analysis showed no differences between types of symptoms, and with the GRADE level of the symptom outcomes, more research is needed to assess the effects of digital SDM tools on severity of symptoms.
We identified two trials that included an outcome of adverse events showing no significant differences between groups. In addition, none of the studies investigated potential negative effects of using the digital intervention. The current evidence on the effectiveness of digital interventions on adverse events is scarce, which also has been highlighted by other systematic reviews (32). While there are many positive possibilities with digital health technology, it is essential to also examine the potential negative effects of these tools especially with the continuous and rapid development of IT.
For the secondary outcomes, a small significant effect was found for working alliance and decisional conflict. The most frequent outcome measured by the 16 trials, patient satisfaction, revealed no significant effect; but authors of the included trials indicates that “instruments focusing on satisfaction might suffer from ceiling effects” (21). As previous reviews have reported mixed results on patient satisfaction (9, 33) with it being advocated as an argument for implementing SDM (4), future trials may wish to consider their choice of measurement for patient satisfaction and pilot test to identify a potential ceiling effect. Lastly, the narrative synthesis indicates a broad range of outcomes that digital SDM interventions may have an effect on, such as knowledge, unmet needs, and the level of SDM.
The significant effect on patient activation, working alliance, and decisional conflict may indicate that SDM benefits the collaboration between patient and provider. Future research may wish to investigate whether SDM is directly associated with an effect on health outcomes or if the collaboration serves as a mediator for health outcomes (e.g., severity of symptoms).
The fact that the most frequent outcome measures in this review were assessed by half of the trials and that only two trials measured our planned primary outcome, patient activation, highlights the vast differences in how trials evaluate their SDM interventions. Furthermore, although all included interventions support SDM, only three studies directly measured the level of SDM (16, 27, 31). According to our protocol, we had also planned to conduct meta-analyses on other outcomes (i.e., adherence to treatment, hospital admissions, level of functioning, and adherence/usage of medicine) (11). These analyses were, however, not possible due to either lack of studies assessing the outcome or outcomes not being compatible for meta-analysis. These outcomes were instead included in the narrative synthesis. The vast differences in how studies evaluate their SDM intervention create a limitation for reviews and meta-analysis since the combined data for each specific outcome are scarce.
Although all included studies investigated an intervention to support SDM, none of the included studies addressed all aspects of SDM, and only three studies included a measurement to assess the overall level of SDM. This may highlight a challenge in how to measure and evaluate the effect on SDM. The included trials in this review could be divided into two groups of systems: (1) a system developed to be used independently of the involvement of a provider or (2) a system developed to be incorporated into the collaboration with the provider. A system to support the patient could be a tool aimed at improving the patient's knowledge on needs, values, options, and the feeling of being prepared for the consultation. A system to support the consultation could be a tool aimed to ensure that a range of life domains and treatment aspects were consistently and structurally addressed and that patients' perspectives were always elicited at the consultation. For interventions not actively including the provider, patients were still encouraged to use the tool in collaboration with their provider (e.g., sharing one's self-assessments or showing one's notes). However, a challenge occurs when either the patient or provider is not actively included: the responsibility of incorporating and using the tool in the consultation is no longer shared and is instead solely placed on either the patient or provider—a responsibility that may be overwhelming for some. Also, as reported by one of the studies “A one-off intervention […] may be insufficient to improve patient involvement in decision-making” (22), indicating that tools to support patients may be helpful but insufficient on their own. Similarly, SDM on its own has shown to be difficult to incorporate into clinical practice due to its complexity and vagueness on how to translate its theoretical model into practice. Therefore, research has called for diverse ways in which SDM principles can be translated into practice such as decision aids (33).
With the vast differences in how researchers are developing tools to support SDM, quality guidance to develop and to assess these tools are needed. Such assessment is possible for, e.g., patient decision aids where International Patient Decision Aid Standards (IPDAS) may be used to assess the quality of the tool (18). In this review, five studies defined their intervention as a decision aid, but only one study mentioned having developed their intervention based on IPDAS guidelines. Since decision aids are not the only mean to support SDM, similar assessment tools could assist in providing clarity on the similarities between tools to support SDM and their level of quality. In addition, future trials investigating the effect of a digital SDM intervention are encouraged to consider including a measurement of adherence/usage of the tool and have their participants evaluate the tool. Reporting an observed effect of a tool in combination with data on how the tool was used will assist future trials and stakeholders to determine whether an effect is dependent on a certain level of usage or acceptance or if the participants found the tool meaningful. While digital interventions may be able to address some of the barriers associated with SDM, it is also important to consider what barriers are introduced with a digital intervention. Traditional barriers for digital interventions may be privacy and data security concerns, but there is also a need for more evidence on how digital interventions may be influenced by variables such as user engagement, data-driven feedback, or individual expectations and characteristics (34).
A majority of the included trials investigated either a web-based intervention or a computer software, while only three studies investigated a smartphone or tablet application. This limited the possibilities to assess the effectiveness of a digital intervention depending on its features or system category (e.g., web-based, computer software, and smartphone app). To determine what digital features are the most effective at supporting SDM, more research investigating different types of features is needed.
Our review has several strengths. Firstly, it provides evidence regarding the effectiveness of digital SDM interventions, which has not been conducted before. Secondly, this meta-analysis strictly follows the registered protocol describing our search strategy, types of studies to be included, data extraction, and targeted outcome measures (11). The only change was the inclusion of one extra secondary outcome, which was done due to several studies assessing this outcome (decisional conflict). A limitation to the study was our inclusion criteria on SDM. The complexness of SDM creates several ways to support SDM, which may cause high heterogeneity between studies. Because of the broad definition of SDM, many of the included trials share similarities while also differing from one another. Subgroup analysis on what components of SDM were used could be highly relevant to identify what aspect of SDM is providing the largest effectiveness. However, such subgroup analysis may be difficult without a clear definition of the SDM process and necessitates that studies clearly describe how their intervention supports SDM. Our study was also limited by not acquiring unpublished literature and assessing publication bias for only one outcome due to the limited amount of studies reporting the same outcomes.
Digital interventions to support SDM may be a promising tool in mental healthcare. The complexness of SDM and possibilities with digital tools create many possibilities for researchers as showcased in this review. It is still unclear which features of digital tools are most effective at supporting the SDM process. More quality research is needed to further assess the effectiveness of digital means to support SDM but also to determine which intervention features are most effective in supporting SDM.
The data analyzed in this study is subject to the following licenses/restrictions: Most data from the included trials can be found online. For some trials, we had to contact the corresponding author to receive the data for analysis. Requests to access these datasets should be directed to dG9iaWFzLnZpdGdlckByZWdpb25oLmRr (I am happy to put you in contact with those authors who delivered raw data).
TV, CH, and LK designed the search strategy and the study protocol. TV and CH screened the identified studies against inclusion/exclusion criteria to determine eligibility for selection. TV performed the data analysis under supervision of CH and wrote the first draft of the manuscript. CH, LK, MN, SA, and LP all critically revised the manuscript. All authors approved the final version of the manuscript.
The authors declare that the research was conducted in the absence of any commercial or financial relationships that could be construed as a potential conflict of interest.
All claims expressed in this article are solely those of the authors and do not necessarily represent those of their affiliated organizations, or those of the publisher, the editors and the reviewers. Any product that may be evaluated in this article, or claim that may be made by its manufacturer, is not guaranteed or endorsed by the publisher.
We would like to thank TrygFonden for making the study possible (Grant number: 115441). TrygFonden is a non-profit foundation, and its core areas include safety, health, and well-being.
The Supplementary Material for this article can be found online at: https://www.frontiersin.org/articles/10.3389/fpsyt.2021.691251/full#supplementary-material
1. World Health Organization. Draft Global Strategy on Digital Health 2020–2024. WHO (2020). Available online: https://www.who.int/docs/default-source/documents/gs4dhdaa2a9f352b0445bafbc79ca799dce4d.pdf?sfvrsn=f112ede5_42 (accessed May 9, 2020.
2. Salyers MP, Zisman-Ilani Y. Shared decision-making self-directed care. In: Goldman HH, Frank R, Morrissey J, editors. The Palgrave Handbook of American Mental Health Policy. Philadelphia: Palgrave MacMillan (2020). doi: 10.1007/978-3-030-11908-9_8
3. Eliacin J, Salyers MP, Kukla M, Matthias MS. Patients' understanding of shared decision making in a mental health setting. Qual Health Res. (2015) 25:668–78. doi: 10.1177/1049732314551060
4. Drake RE, Deegan PE, Rapp C. The promise of shared decision making in mental health. Psychiatr Rehabil J. (2010) 34:7–13. doi: 10.2975/34.1.2010.7.13
5. Hamann J, Cohen R, Leucht S, Busch R, Kissling W. Do patients with schizophrenia wish to be involved in decisions about their medical treatment? AJP. (2005) 162:2382–4. doi: 10.1176/appi.ajp.162.12.2382
6. Couët N, Desroches S, Robitaille H, Vaillancourt H, Leblanc A, Turcotte S, et al. Assessments of the extent to which health-care providers involve patients in decision making: a systematic review of studies using the OPTION instrument. Health Expect. (2015) 18:542–61. doi: 10.1111/hex.12054
7. Zisman-Ilani Y, Lysaker PH, Hasson-Ohayon I. Shared risk taking: shared decision making in serious mental illness. Psychiatr Serv. (2021) 72:461–3. doi: 10.1176/appi.ps.202000156
8. Stovell D, Morrison AP, Panayiotou M, Hutton P. Shared treatment decision-making and empowerment related outcomes in psychosis: systematic review and meta-analysis. Br J Psychiatry. (2016) 209:23–8. doi: 10.1192/bjp.bp.114.158931
9. Duncan E, Best C, Hagen S. Shared decision making interventions for people with mental health conditions. Cochrane Database Syst Rev. (2010) 2010:CD007297. doi: 10.1002/14651858.CD007297.pub2
10. Harrer M, Adam SH, Baumeister H, Cuijpers P, Karyotaki E, Auerbach RP, et al. Internet interventions for mental health in university students: a systematic review and meta-analysis. Int J Methods Psychiatr Res. (2019) 28:e1759. doi: 10.1002/mpr.1759
11. Vitger T, Korsbek L, Stephen A, Petersen L, Nordentoft M, Hjorthøj C. Digital Shared Decision-Making Interventions in Mental Healthcare: Protocol for a Systematic Review and Meta-Analysis. PROSPERO (2020). Available online at: https://www.crd.york.ac.uk/PROSPERO/display_record.php?RecordID=148132 (accessed May 9, 2020).
12. Bomhof-Roordink H, Gärtner FR, Stiggelbout AM, Pieterse AH. Key components of shared decision making models: a systematic review. BMJ Open. (2019) 9:e031763. doi: 10.1136/bmjopen-2019-031763
13. Légaré F, Adekpedjou R, Stacey D, Turcotte S, Kryworuchko J, Graham ID, et al. Interventions for increasing the use of shared decision making by healthcare professionals. Cochrane Database Syst Rev. (2018) 7:CD006732. doi: 10.1002/14651858.CD006732.pub4
14. GRADEpro GDT. GRADEpro Guideline Development Tool [Software]. McMaster University, Evidence Prime, Inc. (2020). Available online at: gradepro.org
15. Fisher A, Keast R, Costa D, Sharpe L, Manicavasagar V, Anderson J, et al. Improving treatment decision-making in bipolar II disorder: a phase II randomised controlled trial of an online patient decision-aid. BMC Psychiatry. (2020) 20:447. doi: 10.1186/s12888-020-02845-0
16. Metz M, Elfeddali I, Veerbeek M, de Beurs E, Beekman A, van der Feltz-Cornelis C. Effectiveness of a multi-facetted blended eHealth intervention during intake supporting patients and clinicians in shared decision making: a cluster randomised controlled trial in a specialist mental health outpatient setting. PLoS ONE. (2018) 13:e0199795. doi: 10.1371/journal.pone.0199795
17. Perestelo-Perez L, Rivero-Santana A, Sanchez-Afonso JA, Perez-Ramos J, Castellano-Fuentes CL, Sepucha K, et al. Effectiveness of a decision aid for patients with depression: a randomized controlled trial. Health Expect. (2017) 20:1096–105. doi: 10.1111/hex.12553
18. Vigod SN, Hussain-Shamsy N, Stewart DE, Grigoriadis S, Metcalfe K, Oberlander TF, et al. A patient decision aid for antidepressant use in pregnancy: pilot randomized controlled trial. J Affect Disord. (2019) 251:91–9. doi: 10.1016/j.jad.2019.01.051
19. Page MJ, McKenzie JE, Bossuyt PM, Boutron I, Hoffmann TC, Mulrow CD, et al. The PRISMA 2020 statement: an updated guideline for reporting systematic reviews. BMJ. (2021) 372:n71. doi: 10.1136/bmj.n71
20. Steinwachs DM, Roter DL, Skinner EA, Lehman AF, Fahey M, Cullen B, et al. A web-based program to empower patients who have schizophrenia to discuss quality of care with mental health providers. Psychiatr Serv. (2011) 62:1296–302. doi: 10.1176/ps.62.11.pss6211_1296
21. van der Krieke L, Emerencia AC, Boonstra N, Wunderink L, de Jonge P, Sytema S. A web-based tool to support shared decision making for people with a psychotic disorder: randomized controlled trial and process evaluation. J Med Internet Res. (2013) 15:e216. doi: 10.2196/jmir.2851
22. Moncrieff J, Azam K, Johnson S, Marston L, Morant N, Darton K, et al. Results of a pilot cluster randomised trial of the use of a medication review tool for people taking antipsychotic medication. BMC Psychiatry. (2016) 16. Available online at: https://search.ebscohost.com/login.aspx?direct=true&db=psyh&AN=2016-33373-001&site=ehost-live
23. Priebe S, McCabe R, Bullenkamp J, Hansson L, Lauber C, Martinez-Leal R, et al. Structured patient-clinician communication and 1-year outcome in community mental healthcare: cluster randomised controlled trial. Br J Psychiatry. (2007) 191:420–6. doi: 10.1192/bjp.bp.107.036939
24. Woltmann EM, Wilkniss SM, Teachout A, McHugo GJ, Drake RE. Trial of an electronic decision support system to facilitate shared decision making in community mental health. Psychiatric Services. (2011) 62:54–60. doi: 10.1176/ps.62.1.pss6201_0054
25. Manthey TJ. A pilot study of my voice: strengths-based and self-directed recovery planning. ProQuest Information & Learning. (2014). Available online at: https://search.ebscohost.com/login.aspx?direct=true&db=psyh&AN=2014-99111-078&site=ehost-live
26. Campbell SusanR, Holter MarkC, Manthey TrevorJ, Rapp CharlesA. The effect of commonground software and decision support center. Am J Psychiatr Rehabil. (2014) 17:166–80. doi: 10.1080/15487768.2014.916126
27. Edbrooke-Childs J, Edridge C, Averill P, Delane L, Hollis C, Craven MP, et al. A feasibility trial of power up: smartphone app to support patient activation and shared decision making for mental health in young people. JMIR Mhealth Uhealth. (2019) 7:e11677. doi: 10.2196/11677
28. MacInnes D, Kinane C, Parrott J, Mansfield J, Craig T, Eldridge S, et al. A pilot cluster randomised trial to assess the effect of a structured communication approach on quality of life in secure mental health settings: the comquol study. BMC Psychiatry. (2016) 16:335. doi: 10.1186/s12888-016-1046-8
29. Kravitz RL. Patient engagement programs for recognition and initial treatment of depression in primary care: a randomized trial. JAMA. (2013) 310:1818–28. doi: 10.1001/jama.2013.280038
30. Priebe S, Kelley L, Golden E, McCrone P, Kingdon D, Rutterford C, et al. Effectiveness of structured patient-clinician communication with a solution focused approach (DIALOG+) in community treatment of patients with psychosis–a cluster randomised controlled trial. BMC Psychiatry. (2013) 13:173. doi: 10.1186/1471-244X-13-173
31. Yamaguchi S, Taneda A, Matsunaga A, Sasaki N, Mizuno M, Sawada Y, et al. Efficacy of a peer-led, recovery-oriented shared decision-making system: a pilot randomized controlled trial. Psychiatr Serv. (2017) 68:1307–11. doi: 10.1176/appi.ps.201600544
32. Firth J, Torous J. Smartphone apps for schizophrenia: a systematic review. JMIR Mhealth Uhealth. (2015) 3:e102. doi: 10.2196/mhealth.4930
33. Zisman-Ilani Y, Barnett E, Harik J, Pavlo A, O'Connell M. Expanding the concept of shared decision making for mental health: systematic search and scoping review of interventions. MHRJ. (2017) 22:191–213. doi: 10.1108/MHRJ-01-2017-0002
Keywords: shared decision-making, systematic review and meta-analysis, mental health, digital health (eHealth), patient activation
Citation: Vitger T, Korsbek L, Austin SF, Petersen L, Nordentoft M and Hjorthøj C (2021) Digital Shared Decision-Making Interventions in Mental Healthcare: A Systematic Review and Meta-Analysis. Front. Psychiatry 12:691251. doi: 10.3389/fpsyt.2021.691251
Received: 05 April 2021; Accepted: 27 July 2021;
Published: 06 September 2021.
Edited by:
Yaara Zisman-Ilani, Temple University, United StatesReviewed by:
Shaun Liverpool, Edge Hill University, United KingdomCopyright © 2021 Vitger, Korsbek, Austin, Petersen, Nordentoft and Hjorthøj. This is an open-access article distributed under the terms of the Creative Commons Attribution License (CC BY). The use, distribution or reproduction in other forums is permitted, provided the original author(s) and the copyright owner(s) are credited and that the original publication in this journal is cited, in accordance with accepted academic practice. No use, distribution or reproduction is permitted which does not comply with these terms.
*Correspondence: Tobias Vitger, dG9iaWFzLnZpdGdlckByZWdpb25oLmRr
Disclaimer: All claims expressed in this article are solely those of the authors and do not necessarily represent those of their affiliated organizations, or those of the publisher, the editors and the reviewers. Any product that may be evaluated in this article or claim that may be made by its manufacturer is not guaranteed or endorsed by the publisher.
Research integrity at Frontiers
Learn more about the work of our research integrity team to safeguard the quality of each article we publish.