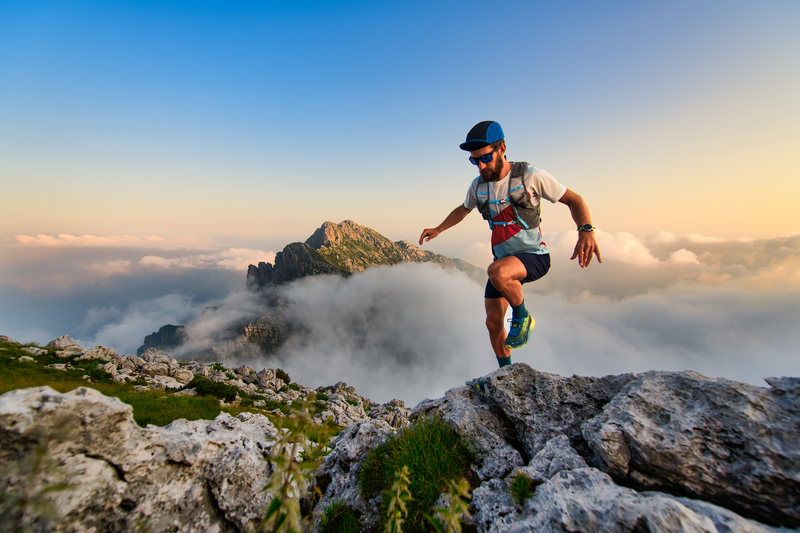
94% of researchers rate our articles as excellent or good
Learn more about the work of our research integrity team to safeguard the quality of each article we publish.
Find out more
ORIGINAL RESEARCH article
Front. Psychiatry , 21 July 2021
Sec. Mood Disorders
Volume 12 - 2021 | https://doi.org/10.3389/fpsyt.2021.674707
This article is part of the Research Topic Assessment and Diagnosis of Bipolar Disorder View all 9 articles
Background: Impulsive aggression represents a frequent characteristic of pediatric bipolar disorder (PBD). Cortical alterations associated with impulsive aggression and its multiple facets have not been investigated yet in youth with bipolar disorder.
Aim: To investigate the relationship between cortical thickness and facets of impulsive aggression in youth with PBD.
Materials and Methods: Twenty-three youth with PBD and 23 healthy controls (HC) were administered the aggression questionnaire (AQ) and underwent 3T magnetic resonance imaging scan. Cortical thickness was assessed with FreeSurfer. Canonical correlation analyses were used to investigate the relationship between AQ total and subscale scores and cortical thickness in youth with PBD.
Results: Youth with PBD had increased scores in the subscales of AQ-anger and AQ-hostility and cortical thinning in in areas belonging to the affective network (AN), frontoparietal network (FPN) and cingulo-opercular network (CON), i.e., right rostral anterior cingulate, right caudal anterior cingulate, right lateral orbitofrontal, right medial orbitofrontal, left and right inferior parietal, left posterior cingulate, left and right supramarginal left lingual cortices. Greater thickness in these networks positively correlated with the AQ-hostility subscale and negatively correlated with AQ-anger subscale.
Conclusions: The opposite patterns observed between areas belonging to AN, FPN, CON, and the two facets of IA, namely anger and hostility, corroborate clinical findings supporting the different nature of these two constructs.
Aggression is defined as behaviors in which physical force is used with the intent to harm or damage (1). Aggression can be purposeful, unemotional and controlled (i.e., predatory aggression), or manifest itself in reaction to a perceived threat in an emotionally charged situation [i.e., impulsive aggression (IA)] (2). IA manifests itself verbally or physically (3) and is the endpoint of a complex psychopathological cascade starting from hostility. Hostility is a cognitive construct defined as an attitude that involves the dislike and the negative evaluation of others and others' intentions (4). Such attitudinal trait predisposes individuals to respond to external cues with frequent and intense angry feelings and related behavior (4). Therefore, hostility preludes anger, i.e., a multifaceted construct consisting of physiological (general sympathetic arousal), cognitive (irrational beliefs, automatic thoughts, inflammatory imagery), phenomenological (subjective awareness and labeling of angry feelings) and behavioral (facial expressions and verbal/ behavioral anger expression strategies) facets (5–7) Anger in turn serves as a cue for developing physical or verbal aggression (8). IA is present across psychiatric diagnoses (9), but is especially present in bipolar disorder (BD) (10).
BD is a chronic and debilitating illness characterized by cyclic alternations of phases of elated mood, increased energy, reduced need for sleep, increased self-esteem, flight of ideas, distractibility and increased involvement in goal directed activity and, phases of depressed mood, feelings of guilt, motor retardation or restlessness, insomnia, and suicidal ideation (11). The onset of BD is usually in late adolescence/early adulthood, although it can also affect children (12, 13). Juvenile-onset BD, or pediatric bipolar disorder (PBD) has a more severe illness course than adult-onset BD, with higher rates of chronicity, cyclicity, and poorer prognosis (14). IA is noted to be a frequent characteristic in individuals with PBD. Indeed, Hernandez et al. (15), reported IA to be present in more than 50% of 7-year olds with PBD. In young adolescents, these rates (16) almost reached 40%. Rates of IA are higher in PBD compared with ADHD (17) or juvenile depression (16), and have been addressed as one of the most common “red flags” that should alert clinicians for the presence of BD in children and adolescents (13). Papolos et al. (18) included IA among a cluster of symptoms identifying a specific phenotype of PBD characterized by a poor prognosis. Furthermore, our group has shown that IA is associated with impairment in specific cognitive domains, such as affective processing and executive functions (19). Finally, IA is regarded as a common and major public health issue that increases the risk of substance abuse, suicidal behaviors, incarceration and violence in adulthood (20).
Despite the pivotal importance of IA in youth with PBD, its neural correlates have been poorly understood. Specifically, alterations in the cortical sheet, which changes with age (21, 22), in neurological (21, 23) and psychiatric disorders (24, 25), and represents a promising in-vivo biomarker proven useful for diagnosis (26), has not been investigated yet. The extant literature on this topic has mainly focused on healthy youth (27–32) with additional reports in youth with autism spectrum disorders (33) conduct disorder (CD) (30) or attention-deficit and hyperactivity disorder (ADHD) (34). These findings highlighted that, independently from the diagnosis, IA is related to thinning of areas of frontal and parietal lobes. Specifically, cortical thinning was reported in areas belonging to the affective network, i.e., an extensive network involved in mood experience and regulation; and to the control network, i.e., a hub of interrelated networks involved in monitoring performances and control impulses and behavior (35–39). Among all the areas involved, thinning in the anterior cingulate cortex (ACC), posterior cingulate cortex (PCC), orbitofrontal cortex (OFC), supramarginal gyrus (SMG) and precuneus were repeatedly reported. Furthermore, all the aforementioned studies limited the analyses of the neural correlates of IA to the whole construct, and to date, possible differences in cortical thickness and all the facets of IA need to be investigated.
The aim of this study is to evaluate the relationship between cortical thickness, IA, and its facets in youth with PBD. Given the transdiagnostic nature of IA, and its relationship with thinning in areas belonging to the affective network and to the control network, we expected youth with PBD to show inverse correlations between the several facets of IA and the cortical thickness of areas belonging to these networks. More specifically, given the most robust findings regarding thinning of ACC, PCC, OFC, SMG, and the precuneus in aggressive youth, we expected a negative relationship between levels of the different facets of IA and thickness of the aforementioned areas.
This study was approved by The Baylor College of Medicine Institutional Review Board. Study participants were recruited from the child and adolescent outpatient psychiatric clinic at Texas Children's Hospital in Houston between January 2016 and October 2019, whereas HCs were recruited through Craigslist. Parents/legal guardians of youth who met study criteria as determined by a phone screen were approached for participation. Research staff explained the study and study procedures to the parental/legal guardians and children/adolescents. Written informed consent from a parent/legal guardian and written assent from the youth were obtained before any study procedure was initiated.
Study participants included females and males, 7–17 years with a diagnosis of PBD and no history of psychiatric and neurologic disorder. Suitability for MRI scanning was also required for all the youths to be included. Exclusion criteria for youth with PBD were: (i) presence of an eating disorder, ADHD and anxiety disorders without comorbid PBD; (ii) comorbid substance use disorder; (iii) intellectual disability; (iv) comorbid autism spectrum disorder; (v) severe neurological conditions. Youth were diagnosed with BD type I and BD, type II per DSM-5 criteria; a diagnosis of BD, not otherwise specified (BDNOS) was made per the Course and Outcome of Bipolar Youth (COBY) research criteria (40).
Study participants were assessed using the 7.0.1 version of the Mini International Neuropsychiatric Interview-Kid-screen (MINI-KID) and the parent MINI-KID (41), and Wechsler Abbreviated Scale of Intelligence – II (WASI-II) (42). The MINI-KID and parent MINI-KID are structured interviews widely used to determine psychiatric diagnoses and have been updated to reflect DSM-5 diagnostic criteria. The WASI– II (WASI-II) (42) was used to determine age- and sex-corrected general intelligence (composite IQ score).
Severity of psychopathology was evaluated with the clinician rated 17-item Children's Depression Rating Scale (CDRS) for depressive symptoms (43) and clinician rated Young Mania Rating Scale (YMRS) for manic symptoms (44). YMRS scores were interpreted as follows: absence (0–12), minimal (13–20), mild (20–26), moderate (26–38),and severe (>38) manic symptoms, and CDRS scores are as follows: absence/minimal depressive symptoms (0 to 28), borderline (29–39) or frank depression (≥40) (43, 44). IA was assessed through the Aggression Questionnaire (AQ) (45), a 29-item scale composed by four subscales that measure four aspects of aggressive behaviors: hostility (AQ-hostility), anger (AQ-anger), verbal aggression (AQ-verbal aggression), physical aggression (AQ-physical aggression). The AQ provides also a total score (AQ-total).
All study participants underwent the same imaging protocol, which included a whole-brain T1-weighted scan acquired using a 3.0 T Siemens Trio scanner. Whole-brain T1-weighted images were obtained in the sagittal plane using the following sequence: TE/TR = 3.68/8.1 ms, matrix 256 × 256 × 180, voxel-size 1 × 1 × 1 mm3. Acquisition time lasted about 5 min. Cortical thickness was computed for 34 bilateral Desikan-Killiany (DK) atlas regions (46), using FreeSurfer 6.0 standard, automated cortical reconstruction pipeline (http://surfer.nmr.mgh.harvard.edu/). The processing steps were as follows: (i) Removal of non-brain tissue and transformation of the T1-weighted scans into the Talairach space (ii). Segmentation of subcortical white matter and gray matter anatomical volumes (47). (iii) Motion correction and non-uniform intensity normalization (48). (iv) Gray/white matter tessellation, topology correction (49) and intensity gradient-based surface deformation to generate gray/white and gray/cerebrospinal fluid surface models (49–51). The resulting surface models were then inflated and registered to a spherical surface atlas, allowing parcellation of cortical regions of interest (50, 52–54). Finally, regional cortical thicknesses were computed by taking the mean of the white-pial distance at all vertices within each parcellated region (26).
Shapiro Wilk's test was used to check for continuous dependent variables' distributions for each group separately. In case of normal distribution, multiple t-tests for continuous variables (i.e., age, IQ), were performed to assess differences in the sociodemographic and clinical characteristics between groups. In case of non-normal distribution, Mann-Whitney tests were performed. In regards to nominal variables (i.e., gender, race/ethnicity), chi-square tests were performed.
Shapiro Wilk's test was used to check for continuous dependent variables' distributions for each group separately. In case of normal distribution, t-tests were performed to investigate differences in psychopathology and cortical thickness between groups. Otherwise, Mannn-Whitney tests were performed. In each test, the two groups (i.e., PBD, HC) were used as independent variables and rating scales assessing severity of psychopathology, i.e., YMRS total score, CDRS total score, AQ-hostility, AQ-anger, AQ-verbal aggression, AQ-physical aggression, AQ-total scores, and cortical thickness of discrete cerebral areas were used as dependent variables. Age, gender and IQ were used as covariates of no interest. Bonferroni correction was applied for multiple comparisons. Specifically, regarding cortical thickness, significance of the p-value was set at p = 0.0007 (p = 0.05/68). For the rating scale scores, the significance of the p-value was set at p = 0.007(p = 0.05/7).
In order to investigate the bi-directional relationships between neurobiological, and psychopathological measures in youth with PBD, canonical correlation analyses (CCAs) were performed. CCAs represent an approach which identifies relationships between two canonical (latent) variates, one representing a set of independent variables (also called predictor variables), the other a set of dependent variables (also called criterion variables). The CCA is optimized such that the linear correlation between the two latent variates is maximized. In other words, CCA finds the eigenvalues and the corresponding eigenvectors of a certain function of covariances. The maximum eigenvalue of the function of covariances is the maximum canonical correlation of the first independent variate and the first dependent variate, and the second largest eigenvalue is the second largest canonical correlation, and so on. Separate sets of coefficients or weights are applied to the predictor and criterion variables to form the linear combinations.
These weights and related statistics, known as loadings, are used to interpret the results of canonical analysis. Therefore, interpretation of what the latent variates represent and how they are related to each other can be determined by the weighted loadings of individual measures on the latent structure, much like principal components analysis. A graphical representation of CCA is provided in Figure 1.
Figure 1. Graphical representation of canonical correlation analysis. LV, latent variate. W, variable's weight.
In this work, variables differentiating youth with PBD from HC entered the CCA, and then, a CCA was conducted for psychopathological measurement. Signs of loadings were used in order to interpret how scores on individual measures related to the latent variates. Therefore, loadings indicate what aspect of psychopathology is captured in each analysis, the neurobiological characteristics with which they are associated, and the nature of the relationship between them. In this view a positive value of a loading indicates higher scores on the individual measures whereas a negative value indicates lower scores on individual measures. Only moderate-strong loadings (beyond −0.2 or 0.2) were taken into account.
As comorbid conditions, medications and duration of illness might affect either IA and brain structure (55–57), additional analyses were conducted to assess the impact of such variables. In order to investigate the effects of comorbidity, the aforementioned t-tests were re-run after subtracting study participants with attention deficit hyperactivity disorder (ADHD; N = 7), obsessive-compulsive disorder (OCD; N = 1), panic disorder (N = 1), anxiety disorder (N = 4). To investigate the effect of medications, the PBD group was split in those with or without antidepressants (PBDAD, N = 12; PBDNAD, N = 11 respectively), antipsychotics (PBDAP, N = 10; PBDNAP, N = 13 respectively), mood stabilizers (PBDMS, N = 11; PBDNMS, N = 12 respectively) and mixed monoamine reuptake inhibitors (PBDMARI, N = 8; PBDNMARI, N = 15 respectively). Then multiple one-way analyses of variance (ANOVAs) were run. In each ANOVA, the aforementioned groups (PBDAD and PBDNAD; PBDAP and BBPNAP; PBDMS and PBDNMS; PBDMARI and PBDNMARI) were used as independent variables and rating scales assessing severity of psychopathology, i.e., YMRS total score, CDRS total score, AQ-hostility, AQ-anger, AQ-verbal aggression, AQ-physical aggression, AQ-total scores, and cortical thickness of discrete cerebral areas were used as dependent variables. Age, gender and IQ were used as covariates of no interest. Bonferroni correction was applied for multiple comparisons. As regards the effect of years of disease, such variable was included in the CCA in the first set of variates.
Sixty-two study participants entered the study. Eight subjects were excluded because they did not fulfill inclusion criteria. Additionally, other 6 subjects were excluded because of motion artifacts. Therefore, the final sample consisted of 46 study participants.
Shapiro-Wilks test showed that variables analyzed have a normal distribution, with the exception of IQ in PBD (W = 0.900; df = 23; p = 0.03). Therefore, Mann–Witney test was performed for investigating between-group differences in IQ. For the other continuous variables, t-tests were performed.
Study participants did not show differences in age, gender, race/ethnicity and IQ. More than a half of the study participants had at least one comorbidity. The most frequent comorbid condition was ADHD, followed by anxiety disorders. All study participants were on medications with most participants being on antidepressants and mood stabilizers (see Table 1).
Youth with PBD showed mild levels of both manic and depressive symptoms. Presence of both manic and depressive symptoms, even though with mild intensity, are indicative of the presence of a mixed state in the PBD group. As regards IA, youth with PBD showed higher scores on the AQ-anger and AQ-hostility subscales and higher scores on the AQ-total than HC (see Table 2).
T-tests revealed that in comparison to the HC, youth with PBD have reduced thickness in frontal (right rostral anterior cingulate, right caudal anterior cingulate, right lateral orbitofrontal, right medial orbitofrontal), parietal (left and right inferior parietal, left posterior cingulate, left and right supramarginal) and occipital (left lingual) areas (see Table 3 and Figure 2).
Figure 2. Differences in cortical thickness between subjects with pBD and HC. pBD, pediatric bipolar disorder; HC, healthy controls. IPC, inferior parietal cortex; SMG, supramarginal gyrus; LG, lingual gyrus; lOFC, lateral orbitofrontal cortex; mOFC, medial orbitofrontal cortex; pACC, posterior anterior cingulate cortex; PCC, posterior cingulate cortex; rACC, rostral anterior cingulate cortex.
Differences between youth with PBD and HC involve 5 psychopathological variables (i.e., YMRS, CDRS, AQ-anger; AQ-hostility. AQ-total) and the cortical thickness of 10 brain areas (i.e., right rostral anterior cingulate, right caudal anterior cingulate, right lateral orbitofrontal, right medial orbitofrontal, left and right inferior parietal, left posterior cingulate, left and right supramarginal, left lingual). Therefore, cortical thicknesses of the aforementioned brain areas were included in the first set, whereas psychopathological measures were included in the second set. Age, sex, and IQ entered the model in the second set in order to control the results for the effect of these variables. The CCA revealed that only the correlation between the first pair of variates was significant (see Table 4). In the CCA, thicknesses of the right rostral anterior cingulate, right caudal anterior cingulate, right lateral orbitofrontal, right medial orbitofrontal, left and right inferior parietal, left posterior cingulate, left and right supramarginal, left lingual negatively correlated with the latent variate. In regards to psychopathological measures, both CDRS and YMRS scores positively correlated with the latent variable, with the latter showing greater association than the former. In regards to IA, AQ-anger positively correlated with the latent variate, whereas AQ-hostility negatively correlated with it. Within the demographic characteristics, age showed a strong positive correlation with the latent variate. The other variables showed a weak relationship with the latent variate (see Figure 3).
Figure 3. Graphical representation of CCA in youth with PBD. Each variable's loading strength is represented with a color. As the loading approaches the value of −1, the loading color will approach the color deep blue. As the loading approaches the value of 1, the loading color will approach the color red. PBD, pediatric bipolar disorder. IPC, inferior parietal cortex; SMG, supramarginal gyrus; LG, lingual gyrus; lOFC, lateral orbitofrontal cortex; mOFC, medial orbitofrontal cortex; pACC, posterior anterior cingulate cortex; PCC, posterior cingulate cortex; rACC, rostral anterior cingulate cortex. LV, latent variate.
After removing youth with ADHD, OCD, panic disorder and anxiety disorder, t-tests regarding psychopathology and cortical thickness did not change. In regard to medications, one-way ANOVAs did not show any differences amongst groups with regard to psychopathology and cortical thickness. Regarding the impact of the duration of illness on the results found, the CAA revealed that only the correlation between the first pair of latent variates was significant (canonical correlation, 0.99; squared canonical correlation, 0.99; eigenvalue, 95.07; Wilk's lambda <0.001; p = 0.002). Duration of illness' loading on the latent variate reached a value of 0.158, meaning a weak correlation with the latent variate.
Results might be summarized as follows: (i) youth with PBD showed heightened levels of anger and hostility compared with HC. (ii) youth with PBD showed cortical thinning in prefrontal and parietal cortices. (iii) in youth with PBD, thinning of these cortices was associated with higher levels of anger and lower levels of hostility.
These findings are in line with those reporting heightened anger and hostility (58, 59) and low level of interpersonal violence despite the high levels of incarceration in youth with PBD (60, 61). Additionally, these findings replicate those reporting altered thickness in ACC and PCC (62), OFC (63, 64), inferior parietal cortex (IPC) (65), lingual cortex (LC) and supramarginal gyrus (SMG) (66) in youth with PBD even though cortical thinning has been found in other regions, such as the precentral and superior frontal (67) cortices, or temporal regions (62). Indeed, discrepancies with these findings might be due to the small sample size of our study, or the sample selection of the other studies, which mainly included young adults. Further studies, with larger sample sizes and narrower inclusion criteria are necessary to define alterations in cortical thickness in youth with PBD.
Thinner cortical areas in youth with PBD, i.e., ACC, PCC, OFC, IPC, SMG, LC, are part of two interconnected networks involved in emotion regulation and impulse control. The rostral part of the ACC and the medial and lateral parts of the OFC belong to the affective network (AN) (68–70). The OFC and ACC are densely interconnected with the amygdala and the other subcortical limbic and paralimbic regions through specific feedback and feedforward pathways that serve to convey attention to motivationally relevant stimuli and provide information about the emotional salience or significance of external stimuli in order to rapidly perceive reward contingency (35). Furthermore, both areas participate in the voluntary regulation of emotionally relevant states (36). The other regions belong to a broad network involved in the initiation, maintenance and control of goal directed behavior, and indirectly implicated in impulse control (37, 38). Such “control network” embeds several functions including attention, working memory, response selection, response inhibition, and task switching, that are activated over time in order to prevail against distraction and respond quickly to unpredictable demands that arise during performances (39). This network can be subdivided in two functionally distinct, and partially overlapping networks: the frontoparietal network (FPN) and the cingulo-opercular network (CON). The CON includes the dorsal1 ACC, the PCC, and the LG and contributes to the flexible control of human goal-directed behavior through the stable, across-trial implementation of task sets in downstream sensorimotor processors (37). On the other hand, the FPN includes the IPC, middle cingulate cortex, and supports control initiation and provides flexibility by adjusting control in response to feedback (38).
The CCA demonstrated that the indirect evidence of dysfunction in such networks, i.e., cortical thinning, is correlated with both CDRS and YMRS mean scores. Accordingly, alteration of the AN has been related to manic, depressive, and mixed states, in which depressive and manic phases co-occur (71, 72). Furthermore, the core clinical features of mixed states i.e., irritability, impulsivity and anger, have been related to alterations of both FPN and CON (73, 74). The CCA also show that cortical thinning of areas belonging to the AN, FPN and CON are associated with higher levels of anger, whereas, an opposite pattern was found with hostility. Anger and hostility are considered as two distinct constructs. Anger is seen as an emotional state consisting of physiological, phenomenological and expressive behavioral variables (6, 7) whereas hostility is regarded as more of a cognitive construct (75). Hostility involves cognitive variables of cynism, mistrust and denigration (76), which are mainly driven by a tendency toward viewing others' actions as hostile and purposeful when their intention is unclear, i.e., the hostile attributional bias (8). Activation of the AN, and more specifically, selective hyperactivation of the OFC, has been related to the development of preference for evaluating ambiguous social cues as hostile (77). On the other hand, activation of FPN and OCN has been related to detection of threat (78) and attention bias toward threat (79). Therefore, greater thickness of areas related to AN, FPN, and CON, possibly reflecting greater activity of these networks, might produce greater bias toward negative stimuli and greater alert toward the others, i.e., greater hostile attributional bias. This might turn in greater levels of hostility.
On the other hand, negative correlations between anger and thickness of cortical areas belonging to AN, FPN, and CON might reflect poor activity of these networks as anger outbursts increase. As mentioned before, altered activity of cortical areas belonging to AN are involved in emotion regulation. Poor emotion regulation does not allow for the inhibition of prepotent emotional impulses coming from subcortical areas, such as the amygdala (80). Poor activity in the FPN and CON might facilitate the transition of prepotent emotionally states into action through failure of response inhibition processes., i.e., the capability of withholding or canceling routinized, ongoing, or prepotent responses to enable goal-oriented behaviors (81, 82). Altered emotion regulation and response inhibition might allow emotional negativity to bypass deliberate processing and lead to anger outbursts.
This work has several limitations. First, the small sample size limits the generalizability of the results found. Therefore, larger sample sizes are needed to clearly investigate the relationship between cortical thickness and impulsive aggression in youth with PBD. Second, a narrower age range in youth is needed to investigate the interplay between brain regional developmental trajectories and psychopathology. The broad age range in the present study did not account for brain regional developmental maturation differences. In fact, regional gray matter thickness' plateau varies with age, spanning from 7 to 11 years old, and follows a maturational pattern that could be linear, quadratic or cubic (83). Additional studies with sample sizes including only children or adolescents are needed to investigate whether the present findings are applicable to either children and adolescents. Third, the effect of possible confounding variables might have interfered with the results found. Such variables have been proved to modify brain and behavior and include, but are not limited to specific medications (84–86), childhood trauma (87, 88) or predominant polarity (89). Specific work on the effect of these variables on the neural bases of impulsive aggression are warranted to clarify their impact.
This preliminary work highlights that altered thickness in areas belonging to the AN, PFN and CON are related to the different facets of impulsive aggression. Specifically, results suggest that hyperactivation of the AN, FPN, and CON might result in greater levels of hostile attributional bias, thus increasing levels of hostility. On the other hand, hypoactivation of the aforementioned structures are related to affective dysregulation and behavioral disinhibition, which can result in anger outbursts. Future research and larger samples, using different MRI techniques, are needed to clarify dysfunctional patterns underlying impulsive aggression and its multiple facets in youth with PBD. Novel treatments specifically restoring balance in the activity of such networks are warranted to prevent the development of hostile behaviors and anger outbursts in children and adolescents with PBD.
The raw data supporting the conclusions of this article will be made available by the authors upon request, without undue reservation.
The studies involving human participants were reviewed and approved by Baylor College of Medicine Institutional Review Board. Written informed consent to participate in this study was provided by the participants' legal guardian/next of kin.
KS, GS, and AS designed the study. AS, SK, and JS acquired data. CV and MDN analyzed the data, together with KS and SK. AS, AR, JCS, and GS drafted the manuscript. KS, AR, CV, MDN, and JCS revised the manuscript. All the authors approved the final version of the manuscript.
SK, JS, and KS were supported by the John S. Dunn Foundation (DUNN grant: 2544405602).
The authors declare that the research was conducted in the absence of any commercial or financial relationships that could be construed as a potential conflict of interest.
We express our gratitude to the study participants and their families who contributed their time and effort to participate in this study.
1. Stanford MS, Houston RJ, Mathias CW. Villemarette-pittman NR, Helfritz LE, Conklin SM. Characteriz Aggress Behav. (2003) 10:183–90. doi: 10.1177/1073191103010002009
2. McDermott BE, Quanbeck CD, Busse D, Yastro K, Scott CL. The accuracy of risk assessment instruments in the prediction of impulsive versus predatory aggression. Behav Sci Law. (2008) 26:759–77. doi: 10.1002/bsl.842
3. Kay SR, Wolkenfeld F, Murrill LM. Profiles of aggression among psychiatric patients: I. Nature and prevalence. J Nerv Ment Dis. (1988) 176:539–46. doi: 10.1097/00005053-198809000-00007
5. Berkowitz L. Aggression: its Causes, Consequences, and Control. New York, NY: McGraw-Hill (1993).
6. Eckhardt CI, Deffenbacher JL. Diagnosis of anger disorders. In: Kassinove H, editor. Anger Disorders: Definition, Diagnosis, and Treatment. Washington, DC: Taylor & Francis (1995).
7. Kassinove H, Sukhodolsky DG. Anger disorders: basic science and practice issues. In: Kassinove H, editor. Anger Disorders: Definition, Diagnosis, and Treatment. Washington, DC: Taylor & Francis (1995).
8. Eckhardt C, Norlander B, Deffenbacher J. The assessment of anger and hostility: a critical review. Aggress Violent Behav. (2004) 9:17–43. doi: 10.1016/S1359-1789(02)00116-7
9. Wong TY, Sid A, Wensing T, Eickhoff SB, Habel U, Gur RC, et al. Neural networks of aggression: ALE meta-analyses on trait and elicited aggression. Brain Struct Funct. (2019) 224:133–48. doi: 10.1007/s00429-018-1765-3
10. Latalova K, Kamaradova D, Prasko J. Suicide in bipolar disorder: a review. Psychiatr Danub. (2014) 26:108–14.
11. American Psychiatric Association. Diagnostic and StatisticalManual ofMental Disorders. 5th ed. (2013). Arlington, VA: American Psychiatric Association. doi: 10.1176/appi.books.9780890425596
12. Goodwin FK, Jamison KR. Manic-Depressive Illn Bipolar Disord Recurr Depress. 2nd ed. Oxford: Oxford University Press (2007).
13. Kowatch RA. Diagnosis, phenomenology, differential diagnosis, and comorbidity of pediatric bipolar disorder. J Clin Psychiatry. (2016) 77 (Suppl E1):e1. doi: 10.4088/JCP.15017su1c.01
14. Romero S, Birmaher B, Axelson D, Goldstein T, Goldstein BI, Gill MK, et al. Prevalence and correlates of physical and sexual abuse in children and adolescents with bipolar disorder. J Affect Disord. (2009) 112:144–50. doi: 10.1016/j.jad.2008.04.005
15. Hernandez M, Marangoni C, Grant M, Estrada J, Faedda G. Parental reports of prodromal psychopathology in pediatric bipolar disorder. Curr Neuropharmacol. (2016) 15:380–5. doi: 10.2174/1570159X14666160801162046
16. Connor DF, Ford JD, Pearson GS, Scranton VL, Dusad A. Early-onset bipolar disorder: characteristics and outcomes in the clinic. J Child Adolesc Psychopharmacol. (2017) 27:875–83. doi: 10.1089/cap.2017.0058
17. Doerfler LA, Connor DF, Toscano PF. Aggression, ADHD symptoms, and dysphoria in children and adolescents diagnosed with bipolar disorder and ADHD. J Affect Disord. (2011) 131:312–9. doi: 10.1016/j.jad.2010.11.029
18. Papolos D, Mattis S, Golshan S, Molay F. Fear of harm, a possible phenotype of pediatric bipolar disorder : a dimensional approach to diagnosis for genotyping psychiatric syndromes. J Affect Disord. (2009) 118:28–38. doi: 10.1016/j.jad.2009.06.016
19. Simonetti A, Kurian S, Saxena J, Verrico CD, Soares JC, Sani G, et al. Cognitive correlates of impulsive aggression in youth with pediatric bipolar disorder and bipolar offspring. J Affect Disord. (2021) 287:387–96. doi: 10.1016/j.jad.2021.03.044
20. Tremblay RE, Nagin DS, Séguin JR, Zoccolillo M, Zelazo PD, Boivin M, et al. Physical aggression during early childhood: trajectories and predictors. Pediatrics. (2004) 114:e43–50. doi: 10.1542/peds.114.1.e43
21. De Leo MJ, George AE, Golomb J, Tarshish C, Convit A, Kluger A, et al. Frequency of hippocampal formation atrophy in normal aging and Alzheimer's disease. Neurobiol Aging. (1997) 18:1–11. doi: 10.1016/S0197-4580(96)00213-8
22. Jack CR, Petersen RC, Xu YC, Waring SC, O'Brien PC, Tangalos EG, et al. Medial temporal atrophy on MRI in normal aging and very mild Alzheimer's disease. Neurology. (1997) 49:786–94. doi: 10.1212/WNL.49.3.786
23. Boeve BF, Maraganore DM, Parisi JE, Ahlskog JE, Graff-Radford N, Caselli RJ, et al. Pathologic heterogeneity in clinically diagnosed corticobasal degeneration. Neurology. (1999) 53:795–800. doi: 10.1212/WNL.53.4.795
24. Zipursky RB, Lim KO, Sullivan EV, Brown BW, Pfefferbaum A. Widespread cerebral gray matter volume deficits in schizophrenia. Arch Gen Psychiatry. (1992) 53:795–800. doi: 10.1001/archpsyc.1992.01820030027004
25. Zipursky RB, Lambe EK, Kapur S, Mikulis DJ. Cerebral gray matter volume deficits in first episode psychosis. Arch Gen Psychiatry. (1998) 55:540–6. doi: 10.1001/archpsyc.55.6.540
26. Fischl B, Dale AM. Measuring the thickness of the human cerebral cortex from magnetic resonance images. Proc Natl Acad Sci USA. (2000) 97:11050–5. doi: 10.1073/pnas.200033797
27. Tanzer M, Derome M, Morosan L, Salaminios G, Debbané M. Cortical thickness of the insula and prefrontal cortex relates to externalizing behavior: cross-sectional and prospective findings. Dev Psychopathol. (2020) 23:1–11. doi: 10.1017/S0954579420000619
28. Bos MGN, Wierenga LM, Blankenstein NE, Schreuders E, Tamnes CK, Crone EA. Longitudinal structural brain development and externalizing behavior in adolescence. J Child Psychol Psychiatry Allied Discip. (2018) 59:1061–72. doi: 10.1111/jcpp.12972
29. Yang Y, Joshi SH, Jahanshad N, Thompson PM, Baker LA. Neural correlates of proactive and reactive aggression in adolescent twins. Aggress Behav. (2017) 43:230–40. doi: 10.1002/ab.21683
30. Thijssen S, Ringoot AP, Wildeboer A, Bakermans-Kranenburg MJ, El Marroun H, Hofman A, et al. Brain morphology of childhood aggressive behavior: a multi-informant study in school-age children. Cogn Affect Behav Neurosci. (2015) 15:564–77. doi: 10.3758/s13415-015-0344-9
31. Ameis SH, Ducharme S, Albaugh MD, Hudziak JJ, Botteron KN, Lepage C, et al. Cortical thickness, cortico-amygdalar networks, and externalizing behaviors in healthy children. Biol Psychiatry. (2014) 75:65–72. doi: 10.1016/j.biopsych.2013.06.008
32. Nguyen TV, McCracken JT, Albaugh MD, Botteron KN, Hudziak JJ, Ducharme S, et al. testosterone-related structural brain phenotype predicts aggressive behavior from childhood to adulthood. Psychoneuroendocrinology. (2016) 63:109–18. doi: 10.1016/j.psyneuen.2015.09.021
33. Gouveia FV, Germann J, Devenyi GA, Morais RMCB, Santos APM, Fonoff ET, et al. Refractoriness of aggressive behaviour to pharmacological treatment: cortical thickness analysis in autism spectrum disorder. BJPsych Open. (2020) 6:e85. doi: 10.1192/bjo.2020.71
34. Cha J, Fekete T, Siciliano F, Biezonski D, Greenhill L, Pliszka SR, et al. Neural correlates of aggression in medication-naive children with ADHD: Multivariate analysis of morphometry and tractography. Neuropsychopharmacology. (2015) 40:1717–25. doi: 10.1038/npp.2015.18
35. Öngür D, Price JL. The organization of networks within the orbital and medial prefrontal cortex of rats, monkeys and humans. Cereb Cortex. (2000) 10:206–19. doi: 10.1093/cercor/10.3.206
36. Stein JL, Wiedholz LM, Bassett DS, Weinberger DR, Zink CF, Mattay VS, et al. validated network of effective amygdala connectivity. Neuroimage. (2007) 36:736–45. doi: 10.1016/j.neuroimage.2007.03.022
37. Dosenbach NUF, Fair DA, Miezin FM, Cohen AL, Wenger KK, Dosenbach RAT, et al. Distinct brain networks for adaptive and stable task control in humans. Proc Natl Acad Sci USA. (2007) 104:11073–8. doi: 10.1073/pnas.0704320104
38. Dosenbach NUF, Fair DA, Cohen AL, Schlaggar BL, Petersen SE. A dual-networks architecture of top-down control. Trends Cogn Sci. (2008) 12:99–105. doi: 10.1016/j.tics.2008.01.001
39. Li Q, Yang G, Li Z, Qi Y, Cole MW, Liu X. Conflict detection and resolution rely on a combination of common and distinct cognitive control networks. Neurosci Biobehav Rev. (2017) 83:123–31. doi: 10.1016/j.neubiorev.2017.09.032
40. Axelson D, Birmaher B, Strober M, Gill MK, Valeri S, Chiappetta L, et al. Phenomenology of children and adolescents with bipolar spectrum disorders. Arch Gen Psychiatry. (2006) 63:1139–48. doi: 10.1001/archpsyc.63.10.1139
41. Sheehan DV, Sheehan KH, Shytle RD, Janavs J, Bannon Y, Rogers JE, et al. Reliability and validity of the Mini International Neuropsychiatric Interview for Children and Adolescents (MINI-KID). J Clin Psychiatry. (2010) 71:313–26. doi: 10.4088/JCP.09m05305whi
42. Wechsler D. Wechsler Abbreviated Scale of Intelligence. San Antonio, TX: The Psychological Corporation (1999). doi: 10.1037/t15170-000
43. Mayes TL, Bernstein IH, Haley CL, Kennard BD, Emslie GJ. Psychometric properties of the Children's depression rating scale-revised in adolescents. J Child Adolesc Psychopharmacol. (2010) 20:513–6. doi: 10.1089/cap.2010.0063
44. Young RC, Biggs JT, Ziegler VE, Meyer DA. A rating scale for mania: reliability, validity and sensitivity. Br J Psychiatry. (1978) 133:429–35. doi: 10.1192/bjp.133.5.429
45. Buss AH, Perry M. The aggression questionnaire. J Pers Soc Psychol. (1992) 63:452–9. doi: 10.1037/0022-3514.63.3.452
46. Desikan RS, Ségonne F, Fischl B, Quinn BT, Dickerson BC, Blacker D, et al. An automated labeling system for subdividing the human cerebral cortex on MRI scans into gyral based regions of interest. Neuroimage. (2006) 31:968–80. doi: 10.1016/j.neuroimage.2006.01.021
47. Fischl B, Salat DH, Busa E, Albert M, Dieterich M, Haselgrove C, et al. Whole brain segmentation: Automated labeling of neuroanatomical structures in the human brain. Neuron. (2002) 33:341–55. doi: 10.1016/S0896-6273(02)00569-X
48. Sled JG, Zijdenbos AP, Evans AC. A nonparametric method for automatic correction of intensity nonuniformity in mri data. IEEE Trans Med Imaging. (1998) 17:87–97. doi: 10.1109/42.668698
49. Ségonne F, Pacheco J, Fischl B. Geometrically accurate topology-correction of cortical surfaces using nonseparating loops. IEEE Trans Med Imaging. (2007) 26:518–29. doi: 10.1109/TMI.2006.887364
50. Dale AM, Fischl B, Sereno MI. Cortical surface-based analysis: I. Segmentation and surface reconstruction. Neuroimage. (1999) 9:179–94. doi: 10.1006/nimg.1998.0395
51. Fischl B, Liu A, Dale AM. Automated manifold surgery: constructing geometrically accurate and topologically correct models of the human cerebral cortex. IEEE Trans Med Imaging. (2001) 20:70–80. doi: 10.1109/42.906426
52. Fischl B, Sereno MI, Dale AM. Cortical surface-based analysis: II. Inflation, flattening, and a surface-based coordinate system. Neuroimage. (1999) 9:195–207. doi: 10.1006/nimg.1998.0396
53. Fischl B, Sereno MI, Tootell RBH, Dale AM. High-resolution intersubject averaging and a coordinate system for the cortical surface. Hum Brain Mapp. (1999) 8:272–84.
54. Fischl B, Van Der Kouwe A, Destrieux C, Halgren E, Ségonne F, Salat DH, et al. Automatically parcellating the human cerebral cortex. Cereb Cortex. (2004) 14:11–22. doi: 10.1093/cercor/bhg087
55. Sani G, Kotzalidis GD, Vöhringer P, Pucci D, Simonetti A, Manfredi G, et al. Effectiveness of short-term olanzapine in patients with bipolar i disorder, with or without comorbidity with substance use disorder. J Clin Psychopharmacol. (2013) 33:231–5. doi: 10.1097/JCP.0b013e318287019c
56. Sani G, Simonetti A, Janiri D, Banaj N, Ambrosi E, De Rossi P, et al. Association between duration of lithium exposure and hippocampus/amygdala volumes in type I bipolar disorder. J Affect Disord. (2018) 232:341–8. doi: 10.1016/j.jad.2018.02.042
57. Jones RM, Arlidge J, Gillham R, Reagu S, Van Den Bree M, Taylor PJ. Efficacy of mood stabilisers in the treatment of impulsive or repetitive aggression: Systematic review and meta-analysis. Br J Psychiatry. (2011) 198:93–8. doi: 10.1192/bjp.bp.110.083030
58. Leibenluft E, Charney DS, Towbin KE, Bhangoo RK, Pine DS. Defining clinical phenotypes of juvenile mania. Am J Psychiatry. (2003) 160:430–7. doi: 10.1176/appi.ajp.160.3.430
59. Etain B, Lajnef M, Henry C, Aubin V, Azorin J-M, Bellivier F, et al. Childhood trauma, dimensions of psychopathology and the clinical expression of bipolar disorders: a pathway analysis. J Psychiatr Res. (2017) 95:37–45. doi: 10.1016/j.jpsychires.2017.07.013
60. Fovet T, Geoffroy PA, Vaiva G, Ph D, Adins C. Individuals with bipolar disorder and their relationship with the criminal justice system : a critical review. Psychiatr Serv. (2015) 66:348–53. doi: 10.1176/appi.ps.201400104
61. Ahonen L, Loeber R. Brent DA. The association between serious mental health problems and violence : some common assumptions and misconceptions. Trauma Violence Abuse. (2019) 20:613–25. doi: 10.1177/1524838017726423
62. Pina-Camacho L, Del Rey-Mejías Á, Janssen J, Bioque M, González-Pinto A, Arango C, et al. Age at first episode modulates diagnosis-related structural brain abnormalities in psychosis. Schizophr Bull. (2016) 42:344–57. doi: 10.1093/schbul/sbv128
63. Jalbrzikowski M, Freedman D, Hegarty CE, Mennigen E, Karlsgodt KH, Olde Loohuis LM, et al. Structural brain alterations in youth with psychosis and bipolar spectrum symptoms. bioRxiv. (2018) 58:1079–91. doi: 10.1016/j.jaac.2018.11.012
64. Huber RS, Subramaniam P, Kondo DG, Shi X, Renshaw PF, Yurgelun-Todd DA. Reduced lateral orbitofrontal cortex volume and suicide behavior in youth with bipolar disorder. Bipolar Disord. (2019) 21:321–9. doi: 10.1111/bdi.12729
65. Mitchell RHB, Metcalfe AWS, Islam AH, Toma S, Patel R, Fiksenbaum L, et al. Sex differences in brain structure among adolescents with bipolar disorder. Bipolar Disord. (2018) 20:448–58. doi: 10.1111/bdi.12663
66. Hibar DP, Westlye LT, Doan NT, Jahanshad N, Cheung JW, Ching CRK, et al. Cortical abnormalities in bipolar disorder: an MRI analysis of 6503 individuals from the ENIGMA Bipolar Disorder Working Group. Mol Psychiatry. (2018) 23:932–42. doi: 10.1038/mp.2017.73
67. Sultan AA, Kennedy KG, Fiksenbaum L, MacIntosh BJ, Goldstein BI. Neurostructural correlates of cannabis use in adolescent bipolar disorder. Int J Neuropsychopharmacol. (2020) 24:181–90. doi: 10.1093/ijnp/pyaa077
68. Li BJ, Friston K, Mody M, Wang HN, Lu HB, Hu DW, et al. brain network model for depression: from symptom understanding to disease intervention. CNS Neurosci Ther. (2018) 24:1004–19. doi: 10.1111/cns.12998
69. Del Casale A, Kotzalidis GD, Rapinesi C, Janiri D, Aragona M, Puzella A, et al. Neural functional correlates of empathic face processing. Neurosci Lett. (2017) 655:68–75. doi: 10.1016/j.neulet.2017.06.058
70. Kotzalidis GD, Rapinesi C, Savoja V, Cuomo I, Simonetti A, Ambrosi E, et al. Neurobiological evidence for the primacy of mania hypothesis. Curr Neuropharmacol. (2016) 15:339–52. doi: 10.2174/1570159X14666160708231216
71. Simonetti A, Lijffijt M, Swann AC. The neurobiology of mixed states. Psychiatr Clin North Am. (2020) 43:139–51. doi: 10.1016/j.psc.2019.10.013
72. Phillips ML, Swartz HA. A critical appraisal of neuroimaging studies of bipolar disorder: toward a new conceptualization of underlying neural circuitry and a road map for future research of neuroimaging studies of bipolar disorder: toward a new conceptualization of underlying ne. Am J Psychiatry. (2014) 171:829–43. doi: 10.1176/appi.ajp.2014.13081008
73. Kubera KM, Hirjak D, Wolf ND, Sambataro F, Thomann PA, Wolf RC. Intrinsic network connectivity patterns underlying specific dimensions of impulsiveness in healthy young adults. Brain Topogr. (2018) 31:477–87. doi: 10.1007/s10548-017-0604-9
74. Zhukovsky P, Morein-Zamir S, Meng C, Dalley JW, Ersche KD. Network failures: when incentives trigger impulsive responses. Hum Brain Mapp. (2020) 41:2216–28. doi: 10.1002/hbm.24941
75. Felsten G, Hill V. Aggression questionnaire hostility scale predicts anger in response to mistreatment. Behav Res Ther. (1999) 37:87–97. doi: 10.1016/S0005-7967(98)00104-1
76. Miller TQ, Smith TW, Turner CW, Guijarro ML, Hallet AJ. A meta-analytic review of research on hostility and physical health. Psychol Bull. (1996) 119:322–48. doi: 10.1037/0033-2909.119.2.322
77. Quan F, Zhu W, Dong Y, Qiu J, Gong X, Xiao M, et al. Brain structure links trait hostile attribution bias and attitudes toward violence. Neuropsychologia. (2019) 125:42–50. doi: 10.1016/j.neuropsychologia.2019.01.015
78. Parasuraman R, Galster S. Sensing, assessing, and augmenting threat detection: Behavioral, neuroimaging, and brain stimulation evidence for the critical role of attention. Front Hum Neurosci. (2013) 7:273. doi: 10.3389/fnhum.2013.00273
79. Hakamata Y, Sato E, Komi S, Moriguchi Y, Izawa S, Murayama N, et al. The functional activity and effective connectivity of pulvinar are modulated by individual differences in threat-related attentional bias. Sci Rep. (2016) 6:1–12. doi: 10.1038/srep34777
80. Simonetti A, Lijffijt M, Kahlon RS, Gandy K, Arvind RP, Amin P, et al. Early and late cortical reactivity to passively viewed emotional faces in pediatric bipolar disorder. J Affect Disord. (2019) 253:240–7. doi: 10.1016/j.jad.2019.04.076
81. Aron AR, Behrens TE, Smith S, Frank MJ, Poldrack RA. Triangulating a cognitive control network using diffusion-weighted Magnetic Resonance Imaging (MRI) and functional MRI. J Neurosci. (2007) 27:3743–52. doi: 10.1523/JNEUROSCI.0519-07.2007
82. Luna B, Marek S, Larsen B, Tervo-Clemmens B, Chahal R. An integrative model of the maturation of cognitive control. Annu Rev Neurosci. (2015) 38:151–70. doi: 10.1146/annurev-neuro-071714-034054
83. Shaw P, Kabani NJ, Lerch JP, Eckstrand K, Lenroot R, Gogtay N, et al. Neurodevelopmental trajectories of the human cerebral cortex. J Neurosci. (2008) 28:3586–94. doi: 10.1523/JNEUROSCI.5309-07.2008
84. Serata D, Kotzalidis GD, Rapinesi C, Janiri D, Di Pietro S, Callovini G, et al. Are 5-HT3 antagonists effective in obsessive-compulsive disorder? A systematic review of literature. Hum Psychopharmacol. (2015) 30:70–84. doi: 10.1002/hup.2461
85. De Filippis S, Cuomo I, Lionetto L, Janiri D, Simmaco M, Caloro M, et al. Intramuscular aripiprazole in the acute management of psychomotor agitation. Pharmacotherapy. (2013) 33:603–14. doi: 10.1002/phar.1260
86. Simonetti A, Sani G, Dacquino C, Piras F, De Rossi P, Caltagirone C, et al. Hippocampal subfield volumes in short- and long-term lithium-treated patients with bipolar I disorder. Bipolar Disord. (2016) 18:352–62. doi: 10.1111/bdi.12394
87. Janiri D, Sani G, De Rossi P, Piras F, Banaj N, Ciullo V, et al. Hippocampal subfield volumes and childhood trauma in bipolar disorders. J Affect Disord. (2019) 253:35–43. doi: 10.1016/j.jad.2019.04.071
88. Janiri D, Rossi P, De Kotzalidis GD, Girardi P, Koukopoulos AE, Reginaldi D, et al. Psychopathological characteristics and adverse childhood events are differentially associated with suicidal ideation and suicidal acts in mood disorders. Eur Psychiatry. (2018) 53:31–6. doi: 10.1016/j.eurpsy.2018.05.009
Keywords: pediatric bipolar disorder, impulsive aggression, cortical thickness, anger, hostility
Citation: Simonetti A, Kurian S, Saxena J, Verrico CD, Restaino A, Di Nicola M, Soares JC, Sani G and Saxena K (2021) Cortical Correlates of Impulsive Aggressive Behavior in Pediatric Bipolar Disorder. Front. Psychiatry 12:674707. doi: 10.3389/fpsyt.2021.674707
Received: 01 March 2021; Accepted: 15 June 2021;
Published: 21 July 2021.
Edited by:
Zhaoyu Gan, Third Affiliated Hospital of Sun Yat-sen University, ChinaReviewed by:
Alessandra Maria Passarotti, University of Illinois at Chicago, United StatesCopyright © 2021 Simonetti, Kurian, Saxena, Verrico, Restaino, Di Nicola, Soares, Sani and Saxena. This is an open-access article distributed under the terms of the Creative Commons Attribution License (CC BY). The use, distribution or reproduction in other forums is permitted, provided the original author(s) and the copyright owner(s) are credited and that the original publication in this journal is cited, in accordance with accepted academic practice. No use, distribution or reproduction is permitted which does not comply with these terms.
*Correspondence: Gabriele Sani, Z2FicmllbGUuc2FuaUB1bmljYXR0Lml0
†These authors have contributed equally to this work and share senior authorship
Disclaimer: All claims expressed in this article are solely those of the authors and do not necessarily represent those of their affiliated organizations, or those of the publisher, the editors and the reviewers. Any product that may be evaluated in this article or claim that may be made by its manufacturer is not guaranteed or endorsed by the publisher.
Research integrity at Frontiers
Learn more about the work of our research integrity team to safeguard the quality of each article we publish.