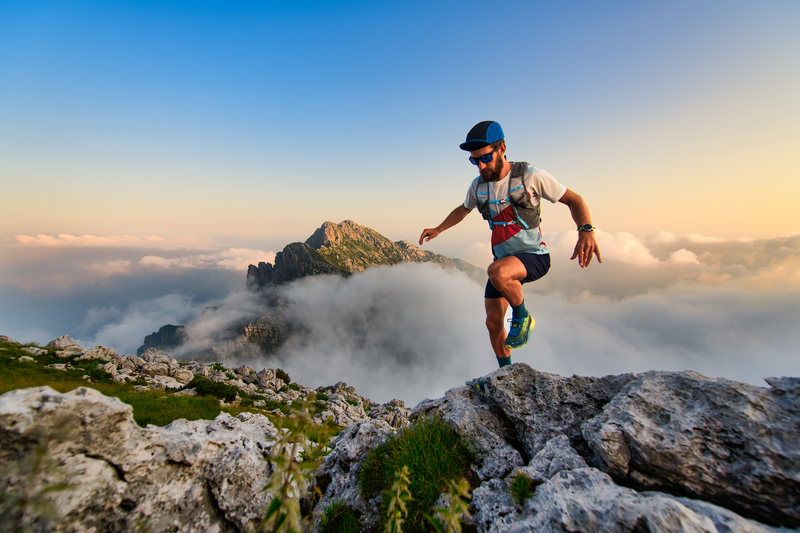
94% of researchers rate our articles as excellent or good
Learn more about the work of our research integrity team to safeguard the quality of each article we publish.
Find out more
SYSTEMATIC REVIEW article
Front. Psychiatry , 23 June 2021
Sec. Child and Adolescent Psychiatry
Volume 12 - 2021 | https://doi.org/10.3389/fpsyt.2021.665326
This article is part of the Research Topic Personalized Precision Medicine in Autism Spectrum Related Disorders View all 15 articles
In recent years, the application of virtual reality (VR) for therapeutic purposes has escalated dramatically. Favorable properties of VR for engaging patients with autism, in particular, have motivated an enormous body of investigations targeting autism-related disabilities with this technology. This study aims to provide a comprehensive meta-analysis for evaluating the effectiveness of VR on the rehabilitation and training of individuals diagnosed with an autism spectrum disorder. Accordingly, we conducted a systematic search of related databases and, after screening for inclusion criteria, reviewed 33 studies for more detailed analysis. Results revealed that individuals undergoing VR training have remarkable improvements with a relatively large effect size with Hedges g of 0.74. Furthermore, the results of the analysis of different skills indicated diverse effectiveness. The strongest effect was observed for daily living skills (g = 1.15). This effect was moderate for other skills: g = 0.45 for cognitive skills, g = 0.46 for emotion regulation and recognition skills, and g = 0.69 for social and communication skills. Moreover, five studies that had used augmented reality also showed promising efficacy (g = 0.92) that calls for more research on this tool. In conclusion, the application of VR-based settings in clinical practice is highly encouraged, although their standardization and customization need more research.
Autism spectrum disorder (ASD) is a complex neurodevelopmental disorder characterized by impairments in social communication and social interaction in conjunction with restricted, repetitive patterns of behavior, interests, or activities (1). Affecting 1 in 68, ASD is the most prevalent psychological childhood disorder with sustained long-term effects on the quality of life of these patients (2).
Although at present there is no particular accepted treatment for ASD, there is a growing consensus that appropriately targeted individualized behavioral and educational intervention programs [e.g., Treatment and Education of Autistic and Related Communication Handicapped Children (TEACCH) program, Early Intensive Behavioral program, Applied Behavior Analytic (ABA) program, Denver model, etc.] have the potential to positively impact the lives of individuals and their families (3–7). The increasing number of individuals with ASD together with the substantial achievements that have been made thus far by this behavioral rehabilitation programs has ignited a line of research aimed at developing several technologies with the focus on improving these programs (8). Some examples include robotics (9–11), interactive video modeling (12–14), mobile and touchpad devices (15–17), wearable training systems on Google Glass (18), and virtual reality (VR) (19, 20). Interestingly, individuals with ASD have shown special interest and adherence to computerized programs (21) and learning through it (22, 23). Moreover, the burden of many hours of training by a therapist can be alleviated by using technology-based training at home.
Among these technologies, VR has become one of the most promising tools to address the psychological needs of people with ASD in various settings. Since two decades ago, VR was introduced as an effective tool in the neurocognitive rehabilitation of patients with ASD (24). This effectiveness has been approved by a decade of research afterward practicing different types of VR configurations on patients with different levels of disorder (25, 26). Besides, some efforts could have possibly improved the application of VR technology in recent works by proposing consideration of psychological theories in task design (27) and highlighting particular features of VR configurations and human–VR interactions (28). VR reduces the social pressure on the patient and provides a realistic environment for more effective training and possibly reduces the needed training hours. Current studies cover a great range of training interventions, including training of social adaptation and communication skills (29–31); emotional skills (32–34); daily living skills such as shopping (35, 36), driving (37–39), and street crossing (40, 41); and cognitive functions (42–44).
VR is a human–computer interface, which by using computer graphics generates a multidimensional environment with multiple sensory channels that allow individuals to explore the virtual environment (VE) through visual, auditory, tactile, and sometimes even olfactory perception, creating an interactive and immersive experience for the user (45, 46). VR can be implemented in head-mounted visual display (HMD) systems, head and body tracking, CAVE (Cave Automatic Virtual Environment) automatic VEs or room-like displays, and other technologies. They can be used to create a realistic sense of “presence” within a computer-generated environment (47). Augmented reality (AR), which can be considered as another type of VR, is a real-time view of an existing world that is superimposed by some virtual data. Unlike VR technology that fully submerges people in an artificial environment avoiding the existing world, AR technology enhances the feeling by overlaying the computer-generated things over the real world (48).
VR training offers several advantages; perhaps the most important one is to provide a safe access to realistic environments that would be considered dangerous in the real world along with active participation in the virtual world. Furthermore, by providing flexibility in controlling the task complexity, reinforcement through repetition and real-time visual and auditory feedback, VR enhances enjoyment and thus improves learning quality through it. These favorable properties of VR have made it a viable tool to be used in training and rehabilitation (49, 50).
In the past decade, VR has served as an effective new treatment tool in different areas such as rehabilitation in post-stroke patients (51, 52), pain management (53), phobias, posttraumatic stress disorders, obsessive–compulsive disorders, anxiety and stress disorders (54), depression (55), attention-deficit/hyperactivity disorder (ADHD) (56), cerebral palsy (57), and of course, ASD.
Although during recent years, several systematic reviews have been conducted to evaluate the efficacy of technology application on training and teaching different skills such as communication and social skills (58, 59), academic skills (60), or information processing (61), only the contribution of Mesa-Gresa et al. (62) was focused on VR and autism as an evidence-based systematic review on the effectiveness of VR-based intervention in ASD. However, their study did not provide a statistical analysis of outcomes for different clinical targets; besides, the included population in their study was limited to children and adolescents.
To date of this study, there is only one meta-analysis on technology-based intervention such as computer games, interactive DVDs, shared active surfaces, and VR in patients with autism (63). Their study presented a comprehensive meta-analysis on the technology-based intervention used in ASD people; however, the type of technology used in their included studies was mostly based on computer gaming software. Since the time of that study, the number of studies applying VR technology for training patients with autism has witnessed a dramatic surge.
Hence, we have tried to conduct a comprehensive meta-analysis focused on the effectiveness of VR technology per se in the training and rehabilitation of patients with autism. To achieve this goal, we performed a systematic search for studies assessing this type of intervention on the ASD population and evaluated the effectiveness of VR training on different skills including social and communication, emotion regulation, daily living, and cognitive skills (CS). We evaluated and compared the effect sizes (ESs) of different skills' improvement to appraise the most influenced clinical targets.
We systematically searched clinical and technical databases including PubMed, ERIC, Web of Science, PsycINFO, and IEEE following a comprehensive search strategy with the main search terms of virtual reality, augmented reality, artificial reality, computer-simulated reality, virtual environment, virtual world, computer-simulated environment, mediated reality, and mixed reality for intervention and the search terms of autism spectrum disorder, pervasive children developmental disorder, and Asperger for disorder, considering adjusted queries for each database. The detailed search strategy and search queries for each database can be seen in Supplementary Material. The initial search yielded a total of 1,204 articles. There was no limit on the publication date, and the search is updated until October 19, 2019.
After removing duplicate records, 915 articles remained for the preliminarily screening of titles and abstracts. Those studies presenting original work and discussed virtual or artificial realities for rehabilitation and training of the ASD population that were published in a peer-reviewed journal or peer-edited conference proceeding books were selected. Case reports, review articles, records that contained only an abstract, and records in non-English languages have been discarded. The articles left were 52 randomized clinical trials, cohort studies, and case series. The full texts of these articles were retrieved for more detailed consideration.
A full-text detailed review was done using the PICO (patient, intervention, comparison, and outcome) process (Figure 1B). The criteria for including studies in our meta-analysis were (1) participants of any age were diagnosed with ASD with a formal diagnostic tool; (2) intervention was conducted on an interactive VR-based setting; (3) the designed intervention aimed at improving skills related to the core symptoms or deficits of ASD; and (4) the same measured data were available on a control group as for the intervention group that measurements performed on the intervention group before undertaking the goal intervention or on a control group that did not receive the goal intervention; and (5) intervention outcomes were assessed by a quantitative measure that was similar for the intervention and control conditions. The studies that did not comply with these criteria were excluded. Along with those, nine records that contained incomplete and/or inaccurate outcome reports in its text or figures were contacted for further information (34, 35, 64–69). One of them responded (41) and thus included in the study. Besides that, five other articles were excluded for a very small sample size (<3) (34, 70–73) and three others for an unfavorable design of the experiment (e.g., single case reports) (32, 74, 75). In the end, 33 studies were proven to be eligible for entering the meta-analysis. The flow diagram of the study selection process is presented in Figure 1A.
Figure 1. (A) Flow diagram of study selection and identification process. (B) Schematic presentation of PICO for this study.
All the studies were coded for the following items: definite diagnosis of disease, diagnostic tool, mean and standard deviation of age of participants, number of participants assigned and completed the course of intervention in the target and control groups, contributing factors and modalities that experimenters controlled for inclusion of study population, concomitant comorbidities with ASD in participants, type of intervention technology (VR or AR), technical details of intervention, experiment properties and intensity of intervention, design of experiment (uncontrolled or case–control), purpose of experiment, and outcome measures and their descriptions.
ASD is a very heterogeneous disorder. Different patients may vary hugely in levels of deficit in different aspects of cognitive functionalities. Thus, most of the studies had attempted measuring multiple outcomes for assessment of therapeutic effectiveness. Dealing with this variability in study outcomes, we categorized them into four major categories: social and communication skills (SCS; e.g., social adaptation and interaction, communication, social reciprocity, social responsiveness, negotiation skills, theory of mind), emotion recognition and regulation skills (ERS; e.g., emotion expression, affect recognition, stress, and anxiety management), daily living skills (DLS; e.g., driving, shopping, street crossing, and job interview skills), and CS (e.g., attention and concentration, reasoning and problem solving, executive function, language, and metacognition). By this means, we were able not only to determine the general effectiveness of VR training but also to distinguish different aspects of ASD-related disabilities in terms of benefit they receive from intervention.
There was a considerable number of trials in which outcomes were assessed by a measure that was mostly intuitive and specifically designed for that experiment (e.g., number of greeting with a friend in VE or subject performance in a face detection task, driving, cross walking, shopping task, or construction play task) rather than measures that are widely used in the field [e.g., Social Responsiveness Scale (SRS) score of social awareness, PEP-3 score affective expressions, Adaptive Behavior Assessment System score of leisure, etc.]. We classified these two types of measures as non-formal and formal, respectively, and considered it as a possible moderator of measured training effectiveness for each trial. Another presumed moderator was the type of technology used for intervention, namely, VR or AR, which are characteristically different in terms of design and application. To explore the effectiveness of the intervention at any age, we assumed four age categories of 4–8, 8–12, 12–16, and older than 16 years. Each trial fell into one of these categories based on their participants' mean age. In a considerable number of trials, patients had some concomitant comorbidity along with their main disorder, ASD. To see how much this comorbidity affected the results of the intervention, we considered the presence or absence of comorbidity as another moderator and compared the results of interventions when having or not having concomitant comorbidity. These four categorical moderators were defined for further subgroup meta-analysis. The trials in which full information regarding any of these moderators was not available were excluded from analysis for that moderator.
Subgroup meta-regression was also applied to three continuous moderator variables: number of intervention sessions, sex, and publication date. These variables were defined as the number of separate sessions or visits in which intervention was applied, male ratio (number male subjects divided by the total number of subjects), and the year of publication of the study, respectively.
Similar to the majority of studies in the literature of training effectiveness, the pool of studies in our meta-analysis included a mixture of two major types of experiment designs, namely, controlled and uncontrolled designs. In controlled or independent-group design, one group received the training, and the other group served as a control. The difference between the groups on the outcome measure was used as an estimate of the treatment effect. On the other hand, in the uncontrolled or single-group pretest posttest design, each individual was measured before and after treatment, and the difference between the individuals' scores before and after it was used as an estimate of the treatment effect.
As the characteristic distinction between these two types of designs can lead to a significant difference in estimated ES and its precision, we opted for design-specific estimations proposed by Morris and DeShon (76) for each study. For uncontrolled studies, the repeated measure ES was calculated as the mean of change from pretest to post-test scores divided by its standard deviation, which is equivalent to the t statistic of paired t-test between two pre-test and post-test data. Then, the Standardized Mean Difference (SMD) for these trials was calculated as follows:
where t represents t statistic, and n represents the number of participants. We used the t statistic values provided in the contents of articles whenever possible or calculated them from the exact pretest and posttest scores.
For controlled studies, the SMD at the posttest was calculated as follows:
where μc represents mean of the control group, μi represents mean of the intervention group, and SDpool is calculated as follows:
where Ni is the size of the intervention group, Nc is the size of the control group, SDi is the standard deviation of the intervention group, and SDc is the standard deviation of the control group at posttest.
For two controlled studies in which pretest data were available for both intervention and control groups, SMD was calculated as follows:
where μposti represents the mean of intervention group scores at posttest; μprei, the mean of intervention group scores at pretest; μpostc, the mean of control group scores at posttest; μprec, the mean of control group scores at pretest; and SDpre is again calculated with the following equation:
where SDprei and SDprec represent the standard deviation of the intervention and control groups' scores at the pretest, respectively (77). All of the aforementioned calculations of SMDs were done in a way that ensures the highest precision in the estimation of each experiment's ES by the available information.
The final ES indicator, Hedges g, then defined as the product of the output SMD and small sample correction factor where df is degrees of freedom. ESs were calculated and reported so that a positive sign represents an improvement in the target skill.
After the computation of ESs for each of the trials, we found that most of them reported more than one estimated value, which is called dependent nested ESs in the literature of meta-analysis. Assuming independence between estimated values for multiple outcomes in each study is usually trivial and thus obtaining a study-level ES by averaging the values within studies might lead to some useful information loss. Handling the dependency among ES estimates, three main methods have been proposed to date: multivariate meta-analysis, three-level meta-analysis, and robust variance estimation (RVE) (78). Multivariate meta-analysis is applied when one or multiple outcomes measured in each study are from a set of known and fixed outcomes across studies. The measured outcomes in our meta-analysis were highly variable from study to study, so we could not apply multivariate analysis. Because of the small sample size of the controlled trials, some of the estimated results of the three-level analysis were underpowered and unreliable, which would question drawn conclusions based on them. So, we opted for the third introduced method, RVE. It was shown that this method accommodates well the dependence arising from multiple sources simultaneously, including multiple measures and multiple treatment groups (78) and thus can be a felicitous choice for our study. Further details on the application of the RVE method on our data are described in Results.
According to the guidelines of Cohen (79), an absolute ES of 0.2–0.3 is regarded as a small effect, ~0.5 as a medium effect, and from 0.8 on as a large effect.
Heterogeneity was assessed by Cochran Q, I2, and τ2 statistics. I2 describes the percentage of variation in studies. The smaller the I2, the lower the level of heterogeneity among estimated values. τ2 statistic is also a measure of between-study variance of ESs. When Q statistic is very small, the estimated I2 is not accurate in capturing the real heterogeneity (80). In these cases, τ2 is more informative specifically when comparing among subgroups with low heterogeneity.
Publication bias was investigated by visual inspection of funnel plots looking for any clue of asymmetry plus Egger intercept test (81) to validate the conclusions.
All the analyses in the main text were done using customized scripts in MathWorks' MATLAB. Three-level meta-analysis was performed in R using an available R package (82).
Thirty-three studies complied with the inclusion and exclusion criteria (see Methods) and entered into the meta-analysis.
The interventions were applied by a controlled experiment design in seven studies and by an uncontrolled design in 24 studies. There were two studies that recruited both types of controlled and uncontrolled designs (mixed-design) (83, 84). As these mixed-design studies included different participants in each design group, we treated them as separate uncorrelated trials. Doing so, we based our analysis on 35 independent trials obtained from 33 studies (The term trial refers to an independent design group consistently thereafter in this article). All in all, 540 ASD participants were included in this study, of which 360 belong to uncontrolled and 180 to controlled trials. There were also 156 ASD patients in the control arm of controlled trials who received neither VR-based nor conventional intervention.
In four controlled trials, the same outcomes were measured before (baseline) and after training in both the control and intervention arms. In the remaining five controlled trials, these measurements were done only after training, and there were no baseline data provided in any control or intervention group. Of 26 uncontrolled trials, three of them applied ABC measurement strategy in a way that outcomes were measured in three temporal phases: after the first session (pre), after the last session (post), and a while after completion of intervention (follow-up) (29, 44, 85). In the other trials, the measurements were performed before (pre) and after (post) interventions. In two trials (one from control and the other one from uncontrolled trials), measurement once was done after a non-VR conventional training, and it was repeated after VR-based target training (43, 86). The data in the first condition were labeled pre-intervention, and in the latter labeled post-intervention. A prior exposure to any type of training was neither recognized nor mentioned in the other studies. The identified pre-intervention and post-intervention data for each trial were used in computing ES statistics (see Methods for more detail).
The diagnostic tools used to integrate patients into the study were different across trials. For instance, in two trials, diagnosis was confirmed by the Diagnostic and Statistical Manual of Mental Disorders, Fourth Edition (DSM-IV) (83, 85), in four other trials by Autism Diagnostic Observation Schedule (ADOS) (39, 42, 87, 88), in one of them by Social Communication Questionnaire (SCQ) (89), in the other two trials by SRS-II (90, 91), in the other 6 by DSM-V (41, 92–94), and in another one by Childhood Autism Rating Scale (CARS) (40). The diagnostic tool in the remaining 20 trials was not mentioned. Regarding stage/level of disease, three trials included patients with high-functioning ASD (HFASD) (90, 94, 95), one trial had patients with low-functioning ASD (LFASD) (92), two trials included Asperger or pervasive developmental disorder—not otherwise specified patients (31, 87), two other trials had patients with either HFASD or Asperger (96, 97), and one trial had patients with Asperger who received the intervention (98). The level of disease in the other 26 trials was not specified. Several trials considered controlling some contributing factors in the population of their study that could potentially impact the outcome of the intervention. For example, eight trials controlled the participants for IQ score (30, 41, 42, 85, 88, 94, 95, 99), two trials for SCQ score (44, 83), one trial for SRS-II score (39), one other trial for PEP-3 score of language and motor skills (92), and one trial for ASI score (86). In six uncontrolled and three controlled trials, patients had another concomitant comorbidity or disorder alongside their main disorder, ASD. Examples include some trials that had patients with a diagnosis of ADHD (42, 44), some other trials that included patients with phobia (83, 89), and finally other trials in which some patients had an intellectual disability or language disorder (40, 41, 86, 92, 93).
AR and VR were integrated into training paradigms through various tools and platforms in the 35 identified trials. From these trials, five opted for AR and 30 for VR to deliver their intervention. They implemented most of these AR-based programs through smartphone, tablet, or desktop applications and platforms to augment three-dimensional (3D) visual features to the more simplistic features conventionally used in the training paradigms, making them more appealing and engaging for children with ASD (29, 43, 85, 98). There was one trial that used Google smart glasses equipped with blink sensors, gyroscope, camera, and display screen (44). In this trial, positive feedback was displayed on the screen whenever the participant could successfully gaze at the instructor's face and detect his/her emotion. Alternatively, most of the VR-based interventions were designed on immersive 3D VE settings in which audiovisual scenes were presented on the walls and ceiling of a room where the participant could fit in different characters in realistic social scenarios. There were also trials that training was based on a particular virtual agent that the participant could play and interact with it. Some VR interventions were also planned on desktop computers using commercial VR software and some of them on HMD devices, which are recently being more and more available and gaining popularity.
More details of included studies for uncontrolled and controlled trials are provided in Tables 1, 2, respectively.
In the 35 trials entailed in our meta-analysis, ES Hedges g were computed for 167 total number of outcome measures, in which 45 pertained to controlled and 122 pertained to uncontrolled trials. As ESs in two groups were significantly different from each other (p = 0.0003, unpaired t-test), we were not allowed to combine them into one group, and so we have done all further analyses separately for each of them.
To compute the study-level ESs, its variance, and also between-study variance, we followed the procedure described by Hedges et al. (102). Based on this procedure, an estimate of within-study correlation (ρ) is needed to compute other statistics. As this estimate could not be extracted from most of our included studies, we ran a sensitivity analysis by choosing various values for ρ ranging from 0 to 1 and then computed other statistics based on the chosen value. The results of sensitivity analysis are given in Supplementary Table 1. By this analysis, we inferred that the value study-level computed ES estimates are sensitive to the choice of ρ, but it was an ascending function of ρ (the larger the ρ, the larger the estimated study-level ESs). Therefore, to avoid any overestimation in computing summary ESs, we fixed the value of ρ at 0 and performed the analysis with this value. It is noteworthy to say that the procedure that we applied in this study is the most parsimonious one avoiding any overstatement of results, but in a realistic situation, the observed effectiveness might be larger as assuming the existence of some level of correlation between study outcomes seems to be a rational assumption. For each trial, we first computed study-level ESs for all of the outcomes irrespective of their category applying RVE procedure, which gave us overall ES of each study. Then, we repeated this procedure on estimated ESs of outcomes in each category of each study to obtain category-based ESs of that study.
In the first step, we computed the overall ES for each trial. For nine controlled trials, summary effect size was at medium range (g = 0.45, SEg = 0.25) with moderate heterogeneity (I2 = 49.5% and τ2 = 0.055). Excluding one of the potential outliers with much larger ES (g = 1.8) (97) led to a bit smaller summary ES, but it was still at the medium range of effectiveness (g = 0.38, SEg = 0.2) (Figure 2). For the 26 uncontrolled trials, a large positive ES was found (g = 0.74, SEg = 0.17) with low heterogeneity (I2 = 2.5% and τ2 = 0.11). Excluding one of the potential outliers with extremely large ES (g = 4.8) (85) led to similar results (g = 0.736, SEg = 0.17) (Figure 3).
Figure 2. Forest plot of overall effectiveness of VR training for controlled trials with 95% confidence interval. Solid vertical lines represent strong effect size boundary (g = −0.8 and 0.8), and dashed vertical lines represent weak effect size boundary (g = −0.3 and 0.3).
Figure 3. Forest plot of overall effectiveness of VR training for uncontrolled trials with 95% confidence interval. Solid vertical lines represent strong effect size boundary (g = −0.8 and 0.8), and dashed vertical lines represent weak effect size boundary (g = −0.3 and 0.3).
We have interpreted the results for overall effectiveness of studies with random-effects model of meta-analysis relying more on controlled trials because of their more robust experimental design.
Further, ESs were computed for each skill category (defined in Methods). In controlled trials, SCS was addressed in five trials, ERS in three trials, DLS in two trials, and CS in one trial. The effectiveness of VR training was weak for SCS (g = 0.2, SEg = 0.23, τ2 = 0.03), weak to moderate for ERS (g = 0.34, SEg = 0.06, τ2 = 0.02), and again very strong in DLS (g = 1.37, SEg = 0.18, τ2 = 1.12). The single trial that addressed CS revealed weak to moderate effectiveness (g = 0.37, SEg = 0.002). Regarding heterogeneity in these estimated summary ESs, a considerably large amount of between-study variance (τ2) was observed in DLS category in both design groups, but it was relatively small for SCS, ERS, and CS, and it was small for ERS (compare the τ2 values presented above) (Figure 4).
Figure 4. Funnel plot for VR training effectiveness of both uncontrolled and controlled trials with pseudo−95% confidence interval. Red area represents SCS; green area represents ERS; blue area represents DLS; and yellow area represents CS. Solid vertical lines represent strong effect size boundary (g = −0.8 and 0.8), and dashed vertical lines represent weak effect size boundary (g = −0.3 and 0.3). Filled and empty circles represent Hedges g value of uncontrolled and controlled trials, respectively. Solid lines represent g with 95% confidence interval of uncontrolled trials and dashed lines represent g with 95% confidence interval of controlled trials.
In uncontrolled trials, SCS had been addressed in 11 trials, ERS in 10 trials, DLS in nine trials and CS in seven trials. VR training led to medium to strong effectiveness in SCS (g = 0.68, SEg = 0.08, τ2 = 0.13), medium effectiveness in ERS (g = 0.46, SEg = 0.05, τ2 = 0.07), strong effectiveness in DLS (g = 1.16, SEg = 0.09, τ2 = 0.48), and medium effectiveness in CS (g = 0.45, SEg = 0.02, τ2 = 0.03) (Figure 5). Thus, while in other skills we observe promising effectiveness, the DLS is proven to be the most affected area as its strong effectiveness was consistent among both controlled and uncontrolled trials.
Figure 5. Forest plot of skill-based training effectiveness for uncontrolled trials with 95% confidence interval. Red area represents SCS; green area represents ERS; blue area represents DLS; and yellow area represents CS. Solid vertical lines represent strong effect size boundary (g = −0.8 and 0.8), and dashed vertical lines represent weak effect size boundary (g = −0.3 and 0.3).
As a sizable number of trials had not used any legitimate criteria for screening the participants undergoing intervention (e.g., IQ score, social responsiveness score, disease severity, etc.), we recomputed overall ESs for those trials that screened their population applying this kind of criteria to see how much our results would be biased by this potential confounding factor. In the population screened trials, the results for controlled trials were g = 0.25, SEg = 0.1, k = 7, and τ2 = 0.01 and for uncontrolled trials were g = 0.72, SEg = 0.05, k = 12, and τ2 = 0.04 and. These results in unscreened trials were g = 0.8, SEg = 0.05, k = 2, and τ2 = 0.35 for controlled and g = 0.73, SEg = 0.2, k = 14, and τ2 = 0.18 for uncontrolled trials.
Comparing these ESs among two screen groups, the results were not meaningfully different from each other in uncontrolled trials unlike substantial decline from unscreened to screened trials in controlled interventions. Because of very small sample size of unscreened controlled trials, the results derived from them seem less reliable, although more cautions should be devoted to screening population before intervention.
The results of the subgroup analysis for controlled trials would be underpowered and misleading because of its small sample size, so the conclusions of subgroup meta-analysis and meta-regression are limited to the data of uncontrolled trials. By the way, the results of these analyses for controlled trials are available in Supplementary Tables 4, 5.
We performed a subgroup meta-analysis for the categorical moderators described in Methods. Results showed that the overall ESs that had been computed based on the data obtained from non-formal measures were somehow larger than those obtained from formal measures (g = 0.93 k = 11, and τ2 = 0.2 for non-formal and g = 0.66, k = 15, and τ2 = 0.09 for formal trials). This can be due to the customized measurements that suit the intervention design, less validity of measures, and susceptibility to the rater bias. Regarding the frequency of each measure's application for categories, most of them had applied formal measures except DLS, which application of non-formal measures was more frequent. In DLS, the summary ES was a bit larger for formal measures, although it was derived from only three trials (g = 1.24, k = 4, and τ2 = 0.55 for formal and g = 1.06, k = 5, and τ2 = 0.28 for non-formal measures). For the type of technology (VR or AR), AR interventions led to a larger overall summary ES (g = 0.91, k = 5, and τ2 = 0.3 for AR and g = 0.71, k = 21, and τ2 = 0.1 for VR). The most of AR interventions were applied for CS that showed more effective training in this skill than VR (g = 0.72, k = 3, and τ2 = 0.15 for AR and g = 0.33, k = 4, and τ2 = 0.01 for VR). Regarding intervention effectiveness for age categories, results showed that skill acquiring, in general, got better as the participants got older (g = 0.8, k = 4, and τ2 = 0.13 for ages 4–8 years; g = 0.57, k = 7, and τ2 = 0.04 for ages 8–12 years; g = 0.84, k = 7, and τ2 = 0.09 for ages 12–16 years; and g = 0.85, k = 6, and τ2 = 0.36 for ages >16 years). Skill categories followed the same trend as the strongest effectiveness observed in the age older than 16 years for all of them (g = 0.98, k = 2, and τ2 = 0.3 for SCS; g = 0.46, k = 4, and τ2 = 0.03 for ERS; and g = 1.33, k = 3, and τ2 = 0.82 for DLS for other age groups; Table 3). It is also noteworthy to say that effectiveness was relatively strong for CS in participants aged 4–8 years, which was the major outcome addressed by our included trials in this age group (g = 0.77, k = 3, τ2 = 0.15). These results point to a more favorable effect of VR interventions for older patients. Subgroup analysis for comorbidity revealed considerable decline in training effectiveness on ASD patients with concomitant comorbidity as g = 0.77 in 20 trials with τ2 = 0.13 in which patients did not have any specified comorbidity dropped to g = 0.6 in six trials with τ2 = 0.03 in which patients had some type of comorbidity alongside their main disease. This effect was even more sophisticated in controlled trials so that g = 0.57 in six trials with τ2 = 0.08 without specified comorbidity reduced to g = 0.11 in three trials with τ2 = 0.03 in which a comorbidity was diagnosed. The full Results can be seen in Table 3.
Table 3. Subgroup meta-analysis results for type of measure, type of technology, and age moderators.
To see if there is any significant interaction between continuous moderators (number of sessions, sex, and publication date) and effectiveness of the intervention, we opted for univariate linear regression on weighted ESs as a function of each of these moderators for all designs and skill categories (Table 4). Significant relationship was found in publication date for overall (N = 122, beta1 = 0.4, p = 0.02) and DLS (N = 30, beta1 = 0.95, p = 0.006), which show that over time, intervention qualities have been likely to be improved as the technology has been advancing; there was no significant association between the number of sessions or gender and computed ESs in any of outcome categories.
Visual inspection of the funnel plot (Figure 6) for both controlled and uncontrolled overall ESs pointed to a symmetrical funnel for both trials. To validate this conclusion statistically, we applied Egger regression intercept test. The test results corroborated the visual inspection by revealing no significant bias for uncontrolled and controlled trials [intercept= 0.27 (p = 0.24) for uncontrolled and intercept = 0.1 (p = 0.88) for controlled trials]. This implies that drawn conclusions are robust and reliable.
Figure 6. Funnel plot for VR training effectiveness of both uncontrolled and controlled trials with pseudo 95% confidence interval. Filled and empty circles represent Hedges g value of uncontrolled and controlled trials respectively. Solid lines represent g with 95% confidence interval of uncontrolled trials and dashed lines represent g with 95% confidence interval of controlled trials.
Comparing effectiveness of VR training with some of conventional behavioral programs that were addressed by three meta-analysis studies, we observed a comparable moderate effectiveness of our study with the most of clinical targets appraised by them. One exception was effectiveness of early intensive behavioral intervention on full-scale IQ of patients with ASD, which was proven to be strong (g = 1.1 of nine controlled studies). The other interesting finding was that TEACCH and ABA programs were not effective in improving daily living skills (g = 0.34 from 6 and g = 0.14 from 29 studies, respectively), while the effectiveness of VR training on this clinical target was very strong as it was observed in both controlled and uncontrolled trials. For full results on this part, see Table 5.
We performed a systematic review and meta-analysis on the effectiveness of applying VR-based therapeutic interventions on the alleviation of deficits in ASD patients. Based on the results of 26 uncontrolled and nine controlled trials, we concluded that VR technology can be a viable tool for designing interventions aimed at enhancing and improving different skills in people suffering from ASD at any age. To our knowledge, this is the first meta-analysis focusing exclusively on the effectiveness of VR-based interventions for training ASD patients. Although there are some meta-analysis studies available on the same topic with various types of new technologies that may (46, 63) or may not (103–105) include VR, the number of trials that methodologically focused on VR was not large enough to draw rigorous conclusions around their efficacy. The increase in the number of VR interventions has been conducted recently; besides, its public availability has justified the need for this study. Overall moderate effectiveness of VR interventions that we observed in this study is in line with the results of previously mentioned studies. Our study shows moderate effectiveness (g = 0.44) of VR interventions based on controlled trials and strong effectiveness (g = 0.73) based on uncontrolled trials. Although the number of uncontrolled trials was conspicuously larger than controlled ones (26–9), a more credible design of controlled trials leads us to the point to claim moderate effectiveness of VR-based training in individuals with ASD.
Low heterogeneity in uncontrolled trials would provide further support for the conclusion drawn from these trials. Moderate heterogeneity in controlled trials cast doubts on interpretation of their results that could be explained by their relative small sample size (only nine trials comparing to 26 uncontrolled trials) and also the heterogeneity of control groups.
Further analysis of entailed categories of skills revealed relatively the same moderate effectiveness of intervention for SCS, ERS, and CS except daily living skills that outperformed other categories with promising large effectiveness in both design groups. This effect was proven to be consistent across different trials with different designs as heterogeneity was low for both of them. This finding can be specifically of interest because of the more reflective nature of DL skills, which means that they are gained and generalized in later stages of cognitive development, and ASD subjects are required to be trained for it similarly to their neurotypical counterparts. Unlike reflective skills, communication or emotional skills are more intuitive in the sense that they are generally gained in the early stages of development without any specific effort. It is also possible that the observed large effect originated from the larger mean age of participants in this outcome category comparing to other categories, which have given them superiority and dexterity in learning and practicing skills. Nevertheless, significantly larger effectiveness of VR training for DLS compared to others is persuasive enough for us to put forward the hypothesis that reflective skills hold more potential toward improvement by training than intuitive skills. Here we may have corroborated this hypothesis for VR-based training. On the other hand, medium effectiveness for communication, emotion, and CS may be due to the complex mental nature of these skills. As it is reflected in the recent systematic review (62), although these skills have been the center of attention in most studies, just partial improvements have been made. Knowing this, in future efforts, a more elaborated intervention design seems necessary to ascertain the effectiveness of VR training on these types of skills. Taken together, we encourage psychiatrists and educators of people with ASD to practice this type of technology with more focus on daily living skills.
The results of our subgroup analysis are merely discussed on overall outcomes of uncontrolled trials. The small sample size of other subgroups precluded us from drawing a strong conclusion for them. The effectiveness of an intervention based on formal and non-formal measures was roughly similar and around moderate range, which indicates that our results might not be affected with the existence of non-formal assessments. Apart from that, cautious interpretation of informal measures should be considered, and it is possible that defined informal measures could be biased. The effectiveness of AR was similar and even a little larger than VR interventions. Although the sample size of the AR subgroup was relatively small, considering its low heterogeneity, the resultant conclusion on this subgroup can be reliable. This is particularly important because AR interventions can be conducted by means of AR-enabled mobile phones, which is ubiquitous nowadays providing more controlled interventions for larger populations of patients with ASD. The superiority of AR can be assigned to its simplicity in design and convenience of use compared to VR in which tasks are designed and applied in more complex environments with more parameters to understand and deal with. This simplicity can lead to a sooner and better engagement of participants in the task specifically for younger children.
The results of the subgroup analysis for age categories revealed that performance improves as the age gets larger. Particularly, it is important to note that this improvement is happening not only in daily living skills, which are reflective skills and later in development, but also in other intuitive areas, such as social skills and emotion recognition skills. This phenomenon may be induced by two factors. First, patients with autism presumably develop a kind of mechanism to overcome the deficits primarily caused by ASD, and so they assimilate to their milieu as they age. Second, older patients may have the advantage that they understand the task and VR environment better, and so they interact with it more efficiently, resulting in improved performance. In the first age category (4–8 years old), a notable relatively strong effectiveness was observed in CS, which was the only addressed area in this age group. Despite the small sample size, the relatively large ES was persuasive enough for us to consider it. This large effect may also be seen in other areas of SCS, ERS, and DLS; therefore, we encourage the scientific community to target their interventions on these areas too.
We observed a substantial decline in the effectiveness of training on patients who suffered from some sort of concomitant comorbidity along with ASD. This phenomenon was particularly interesting in controlled trials as observed moderate effectiveness of training on ASD patients without other comorbidity was completely vanished when concomitant comorbidity was taken into account. This alarms the future practitioners who are trying to improve skills in patients with ASD by means of VR interventions to carefully screen their target patients for having other concomitant comorbidity.
The effectiveness of training for HFASD patients was moderate to large, which was equivalent to its overall value regardless of the level of the disorder. In most of the studies, whether composed of a combination of LFASD and HFASD participants or the level of disease was not specified, direct association between level of disease and effectiveness of intervention could not be derived. For this reason, we call for papers with more focus on defining the level and functionality of disease in included participants for better characterizing the target population of intervention.
The results of meta-regression revealed a significant correlation between publication date and VR training effectiveness, which can be interpreted under improvement in the design and conduction of VR interventions over time. Surprisingly, effectiveness was not influenced by the total number of intervention sessions. It is important to mind that the session's duration and its distribution over the course of intervention were unreported or highly heterogeneous among the trials, and therefore, the net number of sessions might not be a good representative for intensity and quality of intervention. For this reason, hesitant interpretations warrant caution, and more controlled interventions in terms of design, duration, and longevity are needed for more conclusive interpretations on this matter. The sex of participants was not a significant moderator of the results in our study as it is not seen in other studies of this kind.
Comparing the results of the current meta-analysis with those of more conventional training programs (Table 5), it is evident that VR-based training is at least as effective in most study endpoints as traditional programs. In addition, the more flexible and favorable nature of VR leads to more elaborate task designs, more enthusiasm in participants to do those tasks, and ultimately more accurate assessments of improvement. These factors together might result in more ecological validity of VR-based experiments and more reliability of their results.
The strong effectiveness of daily living skills (reflected in both controlled and uncontrolled trials) was achieved only through VR-based training, not conventional training. It is therefore sensible to use VR to design rehabilitation programs aimed at daily living skills in clinical practice. In the other clinical targets, a further improvement in the design and application of VR technology is still required.
Most of our included studies were uncontrolled pretest–posttest trials. It has been argued that these types of trials should be avoided in meta-analysis as the pretest and posttest scores are not independent of each other, and thus, accurate calculation of SMDs requires knowledge of correlation value between these two scores, which is not provided in most of the studies (106). Aside from that, perhaps due to differing epistemological bases of research being carried out in this broad domain, most of the studies have adopted this type of design for their intervention, which makes considering this massive body of data for analysis inevitable. Here, we have done all the calculations with the premise of independent pretest and posttest scores (zero correlation), which is subsequently leading to the largest pooled variance and thus the smallest possible value of computed ES. For this reason, we claim that our applied method is the most parsimonious one avoiding any overestimation in computing the ESs.
Although the number of participants in most studies was rather low, and so their estimations would not be adequately powered, its effect might be compensated by a considerably large number of included trials. Many trials had not screened participants for critical contributing factors that could affect the outcome. This issue seemed to be a challenge for our results. However, later analysis relieved this by showing that the summary ES of those trials that screened included population did not deviate drastically from those who did not perform this screening.
The type of VR technology applied by studies was diverse enough to prevent us from establishing a systematic relationship between the technology type and its effectiveness, so further studies are required to investigate such a connection. To our surprise, restricted and repetitive behavior, which is one of the core symptoms of ASD, was not addressed by any of studies, so more experiments are encouraged to be targeted in this area in the future works. Follow-up assessment of participants was performed in an only limited number of trials; therefore, the maintenance of treatment effects, although important, could not be assessed in our study.
The current findings support the effectiveness of VR training to improve ASD-related disabilities. The strong observed effectiveness for daily living skills could justify the application of VR interventions in clinical practice. For future research, the designed experiments need to be more controlled in terms of selection of participants, type and duration of intervention, and choice of a measurement tool, and finally, more efforts should be devoted to follow-up assessments carried out weeks or months after the end of the intervention to ensure that the effects of training are consolidated and maintained.
The original contributions presented in the study are included in the article/Supplementary Material, further inquiries can be directed to the corresponding author/s.
A-HV and FA designed the study and wrote the protocol. BK, RK, and MR conducted literature searches and provided summaries of previous research studies. BK analyzed data and produced figures. BK and RK wrote the first draft of the manuscript. A-HV supervised the study. All authors contributed to and have approved the final manuscript.
The authors declare that the research was conducted in the absence of any commercial or financial relationships that could be construed as a potential conflict of interest.
The Supplementary Material for this article can be found online at: https://www.frontiersin.org/articles/10.3389/fpsyt.2021.665326/full#supplementary-material
1. American Psychiatric Association. Diagnostic and Statistical Manual of Mental Disorders (DSM-5®). Washington DC: American Psychiatric Pub. (2013). doi: 10.1176/appi.books.9780890425596
2. Baio J. Prevalence of autism spectrum disorder among children aged 8 years - autism and developmental disabilities monitoring network, 11 sites, United States, 2010. Autism and Developmental Disabilities Monitoring Network. Surveillance Year 2010 Principal Investigators. In: Centers for Disease Control and Prevention (U.S.), National Center on Birth Defects and Developmental Disabilities (Centers for Disease Control and Prevention), editors, MMWR Surveillance Summaries: Morbidity and Mortality Weekly Report Surveillance Summaries. (2014). p. 63. Available online at: https://stacks.cdc.gov/view/cdc/22182 (accessed May 31, 2021).
3. Virues-Ortega J, Julio FM, Pastor-Barriuso R. The TEACCH program for children and adults with autism: a meta-analysis of intervention studies. Clin Psychol Rev. (2013) 33:940–53. doi: 10.1016/j.cpr.2013.07.005
4. Eldevik S, Hastings RP, Hughes JC, Jahr E, Eikeseth S, Cross S. Meta-analysis of early intensive behavioral intervention for children with autism. J Clin Child Adolesc Psychol. (2009) 38:439–50. doi: 10.1080/15374410902851739
5. Makrygianni MK, Gena A, Katoudi S, Galanis P. The effectiveness of applied behavior analytic interventions for children with Autism Spectrum Disorder: a meta-analytic study. Res Autism Spectr Disord. (2018) 51:18–31. doi: 10.1016/j.rasd.2018.03.006
6. Dawson G, Rogers S, Munson J, Smith M, Winter J, Greenson J, et al. Randomized, controlled trial of an intervention for toddlers with autism: the Early Start Denver Model. Pediatrics. (2010) 125:e17–23. doi: 10.1542/peds.2009-0958
7. Weitlauf AS, McPheeters ML, Peters B, Sathe N, Travis R, Aiello R, et al. Therapies for Children With Autism Spectrum Disorder: Behavioral Interventions Update. Rockville, MD: Agency for Healthcare Research and Quality (2014). Available online at: http://www.ncbi.nlm.nih.gov/books/NBK241444/ (accessed April 22, 2021).
8. Miller HL, Bugnariu NL. Level of immersion in virtual environments impacts the ability to assess and teach social skills in autism spectrum disorder. Cyberpsychol Behav Soc Netw. (2016) 19:246–56. doi: 10.1089/cyber.2014.0682
9. Billard A, Robins B, Nadel J, Dautenhahn K. Building Robota, a mini-humanoid robot for the rehabilitation of children with autism. Assist Technol. (2007) 19:37–49. doi: 10.1080/10400435.2007.10131864
10. Dautenhahn K. Roles and functions of robots in human society: implications from research in autism therapy. Robotica. (2003) 21:443–52. doi: 10.1017/S0263574703004922
11. Moghadas M, Moradi H. Analyzing human-robot interaction using machine vision for autism screening. In: 2018 6th RSI International Conference on Robotics and Mechatronics (IcRoM). Tehran (2018). p. 572–6. doi: 10.1109/ICRoM.2018.8657569
12. Aldi C, Crigler A, Kates-McElrath K, Long B, Smith H, Rehak K, et al. Examining the effects of video modeling and prompts to teach activities of daily living skills. Behav Anal Practice. (2016) 9:384–8. doi: 10.1007/s40617-016-0127-y
13. Golan O, Ashwin E, Granader Y, McClintock S, Day K, Leggett V, et al. Enhancing emotion recognition in children with autism spectrum conditions: an intervention using animated vehicles with real emotional faces. J Autism Dev Disord. (2010) 40:269–79. doi: 10.1007/s10803-009-0862-9
14. Macpherson K, Charlop MH, Miltenberger CA. Using portable video modeling technology to increase the compliment behaviors of children with autism during athletic group play. J Autism Dev Disord. (2015) 45:3836–45. doi: 10.1007/s10803-014-2072-3
15. Burckley E, Tincani M, Guld Fisher A. An iPad™-based picture and video activity schedule increases community shopping skills of a young adult with autism spectrum disorder and intellectual disability. Dev Neurorehabil. (2015) 18:131–6. doi: 10.3109/17518423.2014.945045
16. Cihak DF, Wright R, Ayres KM. Use of self-modeling static-picture prompts via a handheld computer to facilitate self-monitoring in the general education classroom. Educ Train Autism Dev Disabil. (2010)45:136−49.
17. El Zein F, Gevarter C, Bryant B, Son S-H, Bryant D, Kim M, et al. A comparison between iPad-Assisted and teacher-directed reading instruction for students with Autism Spectrum Disorder (ASD). J Dev Phys Disabil. (2016) 28:195–215. doi: 10.1007/s10882-015-9458-9
18. Kinsella BG, Chow S, Kushki A. Evaluating the usability of a wearable social skills training technology for children with autism spectrum disorder. Front Robot AI. (2017) 4:31. doi: 10.3389/frobt.2017.00031
19. Parsons S, Cobb S. State-of-the-art of virtual reality technologies for children on the autism spectrum. Eur J Special Needs Educ. (2011) 26:355–66. doi: 10.1080/08856257.2011.593831
20. Parsons S, Mitchell P. The potential of virtual reality in social skills training for people with autistic spectrum disorders. J Intellect Disabil Res. (2002) 46:430–43. doi: 10.1046/j.1365-2788.2002.00425.x
21. Moore M, Calvert S. Brief report: vocabulary acquisition for children with autism: teacher or computer instruction. J Autism Dev Disord. (2000) 30:359–62. doi: 10.1023/A:1005535602064
22. Bernard-Opitz V, Sriram N, Nakhoda-Sapuan S. Enhancing social problem solving in children with autism and normal children through computer-assisted instruction. J Autism Dev Disord. (2001) 31:377–84. doi: 10.1023/A:1010660502130
23. Williams C, Wright B, Callaghan G, Coughlan B. Do children with autism learn to read more readily by computer assisted instruction or traditional book methods? a pilot study. Autism. (2002) 6:71–91. doi: 10.1177/1362361302006001006
24. Cheryl G. Trepagnier. Virtual environments for the investigation and rehabilitation of cognitive and perceptual impairments. NeuroRehabilitation. (1999) 12:63–72. doi: 10.3233/NRE-1999-12107
25. Bellani M, Fornasari L, Chittaro L, Brambilla P. Virtual reality in autism: state of the art. Epidemiol Psychiatr Sci. (2011) 20:235–8. doi: 10.1017/S2045796011000448
26. Georgescu AL, Kuzmanovic B, Roth D, Bente G, Vogeley K. The use of virtual characters to assess and train non-verbal communication in high-functioning autism. Front Hum Neurosci. (2014) 8:807. doi: 10.3389/fnhum.2014.00807
27. Rajendran G. Virtual environments and autism: a developmental psychopathological approach. J Comput Assist Learn. (2013) 29:334–47. doi: 10.1111/jcal.12006
28. Parsons S. Authenticity in virtual reality for assessment and intervention in autism: a conceptual review. Educ Res Rev. (2016) 19:138–57. doi: 10.1016/j.edurev.2016.08.001
29. Kurniawan I. The improvement of autism spectrum disorders on children communication ability with PECS method Multimedia Augmented Reality-Based. J Phys. (2018) 2018:12009. doi: 10.1088/1742-6596/947/1/012009
30. Manju T, Padmavathi S, Tamilselvi D. A rehabilitation therapy for autism spectrum disorder using virtual reality. In: Venkataramani GP, Sankaranarayanan K, Mukherjee S, Arputharaj K, Sankara Narayanan S, editors, Smart Secure Systems – IoT and Analytics Perspective. Singapore: Springer Singapore. (2018). p. 328–36. doi: 10.1007/978-981-10-7635-0_26
31. Zhao H, Swanson AR, Weitlauf AS, Warren ZE, Sarkar N. Hand-in-hand: a communication-enhancement collaborative virtual reality system for promoting social interaction in children with autism spectrum disorders. IEEE Trans Human-Machine Syst. (2018) 48:136–48. doi: 10.1109/THMS.2018.2791562
32. Bekele E, Crittendon J, Zheng Z, Swanson A, Weitlauf A, Warren Z, et al. Assessing the utility of a virtual environment for enhancing facial affect recognition in adolescents with autism. J Autism Dev Disord. (2014) 44:1641–50. doi: 10.1007/s10803-014-2035-8
33. Ip HHS, Wong SWL, Chan DFY, Byrne J, Li C, Yuan VSN, et al. Enhance emotional and social adaptation skills for children with autism spectrum disorder: a virtual reality enabled approach. Comput Educ. (2018) 117:1–15. doi: 10.1016/j.compedu.2017.09.010
34. Lorenzo G, Lledo A, Pomares J, Roig R. Design and application of an immersive virtual reality system to enhance emotional skills for children with autism spectrum disorders. Comput Educ. (2016) 98:192–205. doi: 10.1016/j.compedu.2016.03.018
35. Adjorlu A, Høeg ER, Mangano L, Serafin S. Daily living skills training in virtual reality to help children with autism spectrum disorder in a real shopping scenario. In: 2017 IEEE International Symposium on Mixed and Augmented Reality (ISMAR-Adjunct). Nantes (2017). p. 294–302. doi: 10.1109/ISMAR-Adjunct.2017.93
36. Lamash L, Klinger E, Josman N. Using a virtual supermarket to promote independent functioning among adolescents with Autism Spectrum Disorder. In: 2017 International Conference on Virtual Rehabilitation (ICVR). Montreal (2017). p. 1–7. doi: 10.1109/ICVR.2017.8007467
37. Ross V, Cox DJ, Reeve R, Brown T, Moncrief M, Schmitt R, et al. Measuring the attitudes of novice drivers with autism spectrum disorder as an indication of apprehensive driving: going beyond basic abilities. Autism Int J Res Practice. (2018) 22:62–9. doi: 10.1177/1362361317735959
38. Wade J, Bian D, Fan J, Zhang L, Swanson A, Sarkar M, et al. A virtual reality driving environment for training safe gaze patterns: application in individuals with ASD. In: Antona M, Stephanidis C, editors, Universal Access in Human-Computer Interaction: Access to Learning, Health and Well-Being, UAHCI 2015, PT III. (2015). p. 689–97. (Lecture Notes in Computer Science; vol. 9177). doi: 10.1007/978-3-319-20684-4_66
39. Wade J, Zhang L, Bian D, Fan J, Swanson A, Weitlauf A, et al. A gaze-contingent adaptive virtual reality driving environment for intervention in individuals with autism spectrum disorders. ACM Trans Interactive Intelligent Systems. (2016) 6:3. doi: 10.1145/2892636
40. Josman N, Ben-Chaim HM, Friedrich S, Weiss PL. Effectiveness of virtual reality for teaching street-crossing skills to children and adolescents with autism. Int J Disabil Hum Dev. (2008) 7:49–56. doi: 10.1515/IJDHD.2008.7.1.49
41. Saiano M, Pellegrino L, Casadio M, Summa S, Garbarino E, Rossi V, et al. Natural interfaces and virtual environments for the acquisition of street crossing and path following skills in adults with Autism Spectrum Disorders: a feasibility study. J Neuroeng Rehabil. (2015) 12:17. doi: 10.1186/s12984-015-0010-z
42. Didehbani N, Allen T, Kandalaft M, Krawczyk D, Chapman S. Virtual reality social cognition training for children with high functioning autism. Comput Hum Behav. (2016) 62:703–11. doi: 10.1016/j.chb.2016.04.033
43. Nubia RM, Fabián GR, Wilson RA, Wilmer PB. Development of a mobile application in augmented reality to improve the communication field of autistic children at a Neurorehabilitar Clinic. In: 2015 Workshop on Engineering Applications - International Congress on Engineering (WEA). Bogota (2015). p. 1–6. doi: 10.1109/WEA.2015.7370154
44. Vahabzadeh A, Keshav NU, Salisbury JP, Sahin NT. Improvement of attention-deficit/hyperactivity disorder symptoms in school-aged children, adolescents, and young adults with autism via a digital smartglasses-based socioemotional coaching aid: short-term, uncontrolled pilot study. JMIR Mental Health. (2018) 5:9631. doi: 10.2196/mental.9631
45. Burdea GC, Coiffet P. Virtual Reality Technology. Cambridge, MA: John Wiley & Sons (2003). doi: 10.1162/105474603322955950
46. Self T, Scudder RR, Weheba G, Crumrine D. A virtual approach to teaching safety skills to children with autism spectrum disorder. Top Lang Disord. (2007) 27:242. doi: 10.1097/01.TLD.0000285358.33545.79
47. Mishkind MC, Norr AM, Katz AC, Reger GM. Review of virtual reality treatment in psychiatry: evidence vs. current diffusion and use. Curr Psychiatry Rep. (2017) 19:80. doi: 10.1007/s11920-017-0836-0
48. Raaja NR, Shiva GS, Mithun P, Vijayabhas PVM. A review on: augmented reality technologies, systems and applications. J Appl Sci. (2014) 14:1485–95. doi: 10.3923/jas.2014.1485.1495
49. Holden MK. Virtual environments for motor rehabilitation: review. CyberPsychol Behav. (2005) 8:187–211. doi: 10.1089/cpb.2005.8.187
50. Weiss PL, Kizony R, Feintuch U, Katz N. Virtual reality in neurorehabilitation. Textbook Neural Repair Rehabil. (2006) 51:182–97. doi: 10.1017/CBO9780511545078.015
51. Iruthayarajah J, McIntyre A, Cotoi A, Macaluso S, Teasell R. The use of virtual reality for balance among individuals with chronic stroke: a systematic review and meta-analysis. Top Stroke Rehabil. (2017) 24:68–79. doi: 10.1080/10749357.2016.1192361
52. Li Z, Han X-G, Sheng J, Ma S-J. Virtual reality for improving balance in patients after stroke: a systematic review and meta-analysis. Clin Rehabil. (2016) 30:432–40. doi: 10.1177/0269215515593611
53. Luo H, Cao C, Zhong J, Chen J, Cen Y. Adjunctive virtual reality for procedural pain management of burn patients during dressing change or physical therapy: a systematic review and meta-analysis of randomized controlled trials. Wound Repair Regenerat. (2019) 27:90–101. doi: 10.1111/wrr.1
54. Kampmann IL, Emmelkamp PM, Morina N. Meta-analysis of technology-assisted interventions for social anxiety disorder. J Anxiety Disord. (2016) 42:71–84. doi: 10.1016/j.janxdis.2016.06.007
55. Fodor LA, Cotet CD, Cuijpers P, Szamoskozi S, David D, Cristea IA. The effectiveness of virtual reality based interventions for symptoms of anxiety and depression: a meta-analysis. Sci Rep. (2018) 8:1–13. doi: 10.1038/s41598-018-28113-6
56. Bashiri A, Ghazisaeedi M, Shahmoradi L. The opportunities of virtual reality in the rehabilitation of children with attention deficit hyperactivity disorder: a literature review. Korean J Pediatr. (2017) 60:337–43. doi: 10.3345/kjp.2017.60.11.337
57. Chen Y-P, Lee S-Y, Howard AM. Effect of virtual reality on upper extremity function in children with cerebral palsy: a meta-analysis. Pediatric Phys Therapy. (2014) 26:289–300. doi: 10.1097/PEP.0000000000000046
58. DiGennaro Reed FD, Hyman SR, Hirst JM. Applications of technology to teach social skills to children with autism. Res Autism Spectr Disord. (2011) 5:1003–10. doi: 10.1016/j.rasd.2011.01.022
59. Wainer AL, Ingersoll BR. The use of innovative computer technology for teaching social communication to individuals with autism spectrum disorders. Res Autism Spectr Disord. (2011) 5:96–107. doi: 10.1016/j.rasd.2010.08.002
60. Pennington RC. Computer-assisted instruction for teaching academic skills to students with autism spectrum disorders: a review of literature. Focus Autism Other Dev Disabil. (2010) 25:239–48. doi: 10.1177/1088357610378291
61. Den WB, Sterkenburg PS. Self-controlled technologies to support skill attainment in persons with an autism spectrum disorder and/or an intellectual disability: a systematic literature review. Disabil Rehabil Assist Technol. (2015) 10:1–10. doi: 10.3109/17483107.2014.921248
62. Mesa-Gresa P, Gil-Gómez H, Lozano-Quilis J-A, Gil-Gómez J-A. Effectiveness of virtual reality for children and adolescents with autism spectrum disorder: an evidence-based systematic review. Sensors. (2018) 18:2486. doi: 10.3390/s18082486
63. Grynszpan O, Weiss PL, Perez-Diaz F, Gal E. Innovative technology-based interventions for autism spectrum disorders: a meta-analysis. Autism. (2014) 18:346–61. doi: 10.1177/1362361313476767
64. Bekele E, Wade J, Bian D, Fan J, Swanson A, Warren Z, et al. Multimodal adaptive social interaction in virtual environment (MASI-VR) for children with Autism spectrum disorders (ASD). In: 2016 IEEE Virtual Reality (VR). Greenville, SA: IEEE (2016). p. 121–30. doi: 10.1109/VR.2016.7504695
65. Bozgeyikli L, Bozgeyikli E, Raij A, Alqasemi R, Katkoori S, Dubey R. Vocational rehabilitation of individuals with autism spectrum disorder with virtual reality. ACM Trans Accessible Comput. (2017) 10:3046786. doi: 10.1145/3046786
66. Cox DJ, Brown T, Ross V, Moncrief M, Schmitt R, Gaffney G, et al. Can youth with autism spectrum disorder use virtual reality driving simulation training to evaluate and improve driving performance? an exploratory study. J Autism Dev Disord. (2017) 47:2544–55. doi: 10.1007/s10803-017-3164-7
67. Dickinson K, Place M. The impact of a computer-based activity program on the social functioning of children with autistic spectrum disorder. Games Health J. (2016) 5:209–15. doi: 10.1089/g4h.2015.0063
68. Escobedo L, Tentori M, Quintana E, Favela J, Garcia-Rosas D. Using augmented reality to help children with autism stay focused. IEEE Pervasive Comput. (2014) 13:38–46. doi: 10.1109/MPRV.2014.19
69. Lorenzo G, Pomares J, Lledo A. Inclusion of immersive virtual learning environments and visual control systems to support the learning of students with asperger syndrome. Comput Educ. (2013) 62:88–101. doi: 10.1016/j.compedu.2012.10.028
70. Chen C-H, Lee I-J, Lin L-Y. Augmented reality-based self-facial modeling to promote the emotional expression and social skills of adolescents with autism spectrum disorders. Res Dev Disabil. (2015) 36:396–403. doi: 10.1016/j.ridd.2014.10.015
71. Cheng Y, Huang C-L, Yang C-S. Using a 3D immersive virtual environment system to enhance social understanding and social skills for children with autism spectrum disorders. Focus Autism Other Dev Disabil. (2015) 30:222–36. doi: 10.1177/1088357615583473
72. Ke F, Im T. Virtual-reality-based social interaction training for children with high-functioning autism. J Educ Res. (2013) 106:441–61. doi: 10.1080/00220671.2013.832999
73. Wang M, Reid D. Using the virtual reality-cognitive rehabilitation approach to improve contextual processing in children with autism. Sci World J. (2013) 2013:716890. doi: 10.1155/2013/716890
74. Kim K, Rosenthal MZ, Gwaltney M, Jarrold W, Hatt N, McIntyre N, et al. A virtual joy-stick study of emotional responses and social motivation in children with autism spectrum disorder. J Autism Dev Disord. (2015) 45:3891–9. doi: 10.1007/s10803-014-2036-7
75. Parsons S. Learning to work together: designing a multi-user virtual reality game for social collaboration and perspective-taking for children with autism. Int J Child-Computer Interact. (2015) 6:28–38. doi: 10.1016/j.ijcci.2015.12.002
76. Morris SB, DeShon RP. Combining effect size estimates in meta-analysis with repeated measures and independent-groups designs. Psychol Methods. (2002) 7:105. doi: 10.1037/1082-989X.7.1.105
77. Morris SB. Estimating effect sizes from pretest-posttest-control group designs. Org Res Methods. (2008) 11:364–86. doi: 10.1177/1094428106291059
78. Moeyaert M, Ugille M, Beretvas SN, Ferron J, Bunuan R, Noortgate WV den. Methods for dealing with multiple outcomes in meta-analysis: a comparison between averaging effect sizes, robust variance estimation and multilevel meta-analysis. Int J Soc Res Methodol. (2017) 20:559–72. doi: 10.1080/13645579.2016.1252189
79. Cohen J. Statistical Power Analysis for the Social Sciences. Cambridge, MA: Academic press (1988).
80. Borenstein M, Higgins JPT, Hedges LV, Rothstein HR. Basics of meta-analysis: I2 is not an absolute measure of heterogeneity. Res Synthesis Methods. (2017) 8:5–18. doi: 10.1002/jrsm.1230
81. Egger M, Smith GD, Schneider M, Minder C. Bias in meta-analysis detected by a simple, graphical test. BMJ. (1997) 315:629–34. doi: 10.1136/bmj.315.7109.629
82. Cheung MW-L. metaSEM: an R package for meta-analysis using structural equation modeling. Front Psychol. (2015) 5:1521. doi: 10.3389/fpsyg.2014.01521
83. Maskey M, Rodgers J, Ingham B, Freeston M, Evans G, Labus M, et al. Using virtual reality environments to augment cognitive behavioral therapy for fears and phobias in autistic adults. Autism Adulthood Challenges Manag. (2019) 1:134–45. doi: 10.1089/aut.2018.0019
84. Yuan SNV, Ip HHS. Using virtual reality to train emotional and social skills in children with autism spectrum disorder. London J Primary Care. (2018) 10:110–2. doi: 10.1080/17571472.2018.1483000
85. Chen C-H, Lee I-J, Lin L-Y. Augmented reality-based video-modeling storybook of non-verbal facial cues for children with autism spectrum disorder to improve their perceptions and judgments of facial expressions and emotions. Comput Hum Behav. (2016) 55:477–85. doi: 10.1016/j.chb.2015.09.033
86. Zhang L, Warren Z, Swanson A, Weitlauf A, Sarkar N. Understanding performance and verbal-communication of children with ASD in a collaborative virtual environment. J Autism Dev Disord. (2018) 48:2779–89. doi: 10.1007/s10803-018-3544-7
87. Kandalaft MR, Didehbani N, Krawczyk DC, Allen TT, Chapman SB. Virtual reality social cognition training for young adults with high-functioning autism. J Autism Dev Disord. (2013) 43:34–44. doi: 10.1007/s10803-012-1544-6
88. Stichter JP, Laffey J, Galyen K, Herzog M. iSocial: delivering the social competence intervention for adolescents (SCI-A) in a 3D virtual learning environment for youth with high functioning autism. J Autism Dev Disord. (2014) 44:417–30. doi: 10.1007/s10803-013-1881-0
89. Maskey M, Lowry J, Rodgers J, McConachie H, Parr JR. Reducing specific phobia/fear in young people with autism spectrum disorders (ASDs) through a virtual reality environment intervention. PLoS ONE. (2014) 9:e100374. doi: 10.1371/journal.pone.0100374
90. Smith MJ, Fleming MF, Wright MA, Losh M, Humm LB, Olsen D, et al. Brief report: vocational outcomes for young adults with autism spectrum disorders at 6 months after virtual reality job interview training. J Autism Dev Disord. (2015) 45:3364–9. doi: 10.1007/s10803-015-2470-1
91. Smith MJ, Ginger EJ, Wright K, Wright MA, Taylor JL, Humm LB, et al. Virtual reality job interview training in adults with autism spectrum disorder. J Autism Dev Disord. (2014) 44:2450–63. doi: 10.1007/s10803-014-2113-y
92. Chen F, Wang L, Peng G, Yan N, Pan X. Development and evaluation of a 3-D virtual pronunciation tutor for children with autism spectrum disorders. PLoS ONE. (2019) 14:e0210858. doi: 10.1371/journal.pone.0210858
93. Simoes M, Bernardes M, Barros F, Castelo-Branco M. Virtual travel training for autism spectrum disorder: proof-of-concept interventional study. JMIR Serious Games. (2018) 6:8428. doi: 10.2196/games.8428
94. Yang YJD, Allen T, Abdullahi SM, Pelphrey KA, Volkmar FR, Chapman SB. Brain responses to biological motion predict treatment outcome in young adults with autism receiving Virtual Reality Soc Cogn Train. (2017) 93:55–66. doi: 10.1016/j.brat.2017.03.014
95. Ke F, Moon J. Virtual collaborative gaming as social skills training for high-functioning autistic children. Br J Educ Technol. (2018) 49:728–41. doi: 10.1111/bjet.12626
96. Milne M, Luerssen MH, Lewis TW, Leibbrandt RE, Powers DMW. Development of a virtual agent based social tutor for children with autism spectrum disorders. In: The 2010 International Joint Conference on Neural Networks (IJCNN). Barcelona (2010). p. 1–9. doi: 10.1109/IJCNN.2010.5596584
97. Strickland DC, Coles CD, Southern LB. JobTIPS: a transition to employment program for individuals with autism spectrum disorders. J Autism Dev Disord. (2013) 43:2472–83. doi: 10.1007/s10803-013-1800-4
98. Bai Z, Blackwell AF, Coulouris G. Using augmented reality to elicit pretend play for children with autism. IEEE Trans Visual Comput Graph. (2015) 21:598–610. doi: 10.1109/TVCG.2014.2385092
99. Ip HHS, Wong SWL, Chan DFY, Byrne J, Li C, Yuan VSN, et al. Virtual reality enabled training for social adaptation in inclusive education settings for school-aged children with autism spectrum disorder (ASD). In: Cheung SKS, Kwok LF, Shang J, Wang A, Kwan R, editors. Blended Learning: Aligning Theory With Practices, ICBL 2016. City Univ Hong Kong; Caritas Inst Higher Educ; Hong Kong Soc Multimedia & Image Comp; Hong Kong Pei Hua Educ Fdn. Beijing: Lecture Notes in Computer Science (2016). vol. 9757, p. 94–102. doi: 10.1007/978-3-319-41165-1_9
100. Bernardini S, Porayska-Pomsta K, Sampath H. Designing an intelligent virtual agent for social communication in autism. In: Proceedings of the Ninth AAAI Conference on Artificial Intelligence and Interactive Digital Entertainment. Boston, MA: AAAI Press (2014). p. 9–15. Available online at: http://dl.acm.org/citation.cfm?id=3014712.3014715 (accessed October 27, 2018).
101. Miller IT, Wiederhold BK, Miller CS, Wiederhold MD. Virtual reality air travel training with children on the autism spectrum: a preliminary report. Cyberpsychol Behav Soc Netw. 23:10–5. doi: 10.1089/cyber.2019.0093
102. Hedges LV, Tipton E, Johnson MC. Robust variance estimation in meta-regression with dependent effect size estimates. Research Synthesis Methods. (2010) 1:39–65. doi: 10.1002/jrsm.5
103. Hong ER, Ganz JB, Mason R, Morin K, Davis JL, Ninci J, et al. The effects of video modeling in teaching functional living skills to persons with ASD: a meta-analysis of single-case studies. Res Dev Disabil. (2016) 57:158–69. doi: 10.1016/j.ridd.2016.07.001
104. Hong ER, Gong L, Ninci J, Morin K, Davis JL, Kawaminami S, et al. A meta-analysis of single-case research on the use of tablet-mediated interventions for persons with ASD. Res Dev Disabil. (2017) 70:198–214. doi: 10.1016/j.ridd.2017.09.013
105. Wilkinson KM, Hennig S. The state of research and practice in augmentative and alternative communication for children with developmental/intellectual disabilities. Mental Retard Dev Disabil Res Rev. (2007) 13:58–69. doi: 10.1002/mrdd.20133
Keywords: autism spectrum disorder, virtual reality, rehabilitation, technology, augmented reality
Citation: Karami B, Koushki R, Arabgol F, Rahmani M and Vahabie A-H (2021) Effectiveness of Virtual/Augmented Reality–Based Therapeutic Interventions on Individuals With Autism Spectrum Disorder: A Comprehensive Meta-Analysis. Front. Psychiatry 12:665326. doi: 10.3389/fpsyt.2021.665326
Received: 07 February 2021; Accepted: 12 May 2021;
Published: 23 June 2021.
Edited by:
Raz Gross, Sheba Medical Center, IsraelReviewed by:
Yoon Phaik Ooi, University of Basel, SwitzerlandCopyright © 2021 Karami, Koushki, Arabgol, Rahmani and Vahabie. This is an open-access article distributed under the terms of the Creative Commons Attribution License (CC BY). The use, distribution or reproduction in other forums is permitted, provided the original author(s) and the copyright owner(s) are credited and that the original publication in this journal is cited, in accordance with accepted academic practice. No use, distribution or reproduction is permitted which does not comply with these terms.
*Correspondence: Abdol-Hossein Vahabie, aC52YWhhYmllQHV0LmFjLmly
Disclaimer: All claims expressed in this article are solely those of the authors and do not necessarily represent those of their affiliated organizations, or those of the publisher, the editors and the reviewers. Any product that may be evaluated in this article or claim that may be made by its manufacturer is not guaranteed or endorsed by the publisher.
Research integrity at Frontiers
Learn more about the work of our research integrity team to safeguard the quality of each article we publish.