- 1Institute of Global Health and Human Ecology, School of Sciences and Engineering, The American University in Cairo, Cairo, Egypt
- 2Faculty of Medicine, Mansoura University, Mansoura, Egypt
- 3Global Brain Health Institute, Trinity College Dublin, Dublin, Ireland
Modern research has proven that the “typical patient” requiring standardized treatments does not exist, reflecting the need for more personalized approaches for managing individual clinical profiles rather than broad diagnoses. In this regard, precision psychiatry has emerged focusing on enhancing prevention, diagnosis, and treatment of psychiatric disorders through identifying clinical subgroups, suggesting personalized evidence-based interventions, assessing the effectiveness of different interventions, and identifying risk and protective factors for remission, relapse, and vulnerability. Literature shows that recent advances in the field of precision psychiatry are rapidly becoming more data-driven reflecting both the significance and the continuous need for translational research in mental health. Different etiologies underlying depression have been theorized and some factors have been identified including neural circuitry, biotypes, biopsychosocial markers, genetics, and metabolomics which have shown to explain individual differences in pathology and response to treatment. Although the precision approach may prove to enhance diagnosis and treatment decisions, major challenges are hindering its clinical translation. These include the clinical diversity of psychiatric disorders, the technical complexity and costs of multiomics data, and the need for specialized training in precision health for healthcare staff, besides ethical concerns such as protecting the privacy and security of patients' data and maintaining health equity. The aim of this review is to provide an overview of recent findings in the conceptualization and treatment of depression from a precision mental health perspective and to discuss potential challenges and future directions in the application of precision psychiatry for the treatment of depression.
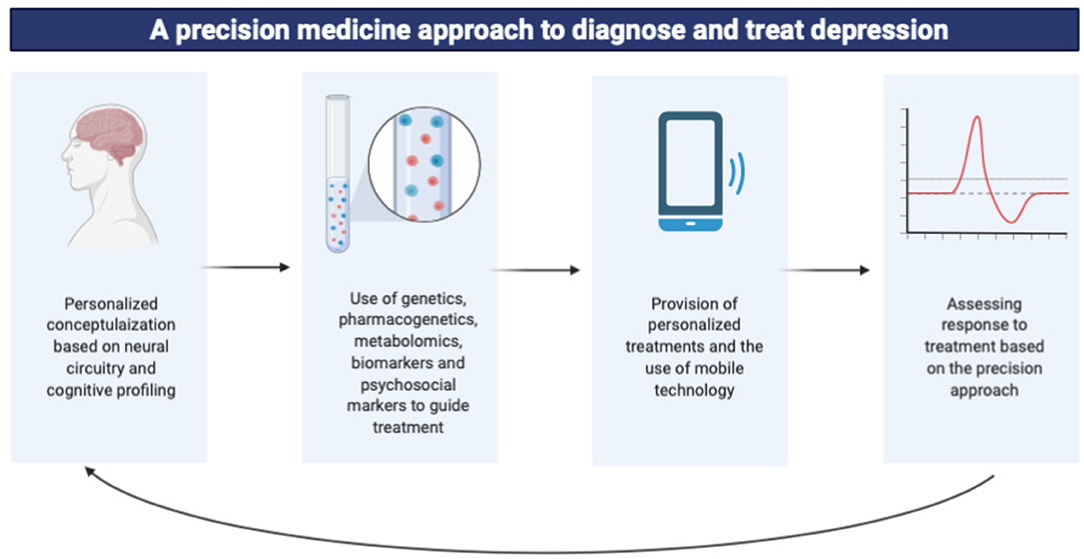
Graphical Abstract. A summary of the precision medicine approach in the diagnosis and treatment of major depressive disorder using multi-omics data.
Key Concepts
This paper aimed to provide an overview of the implementation of precision psychiatry in the treatment of major depressive disorder using multiomics data. Key concepts are summarized in Figure 1.
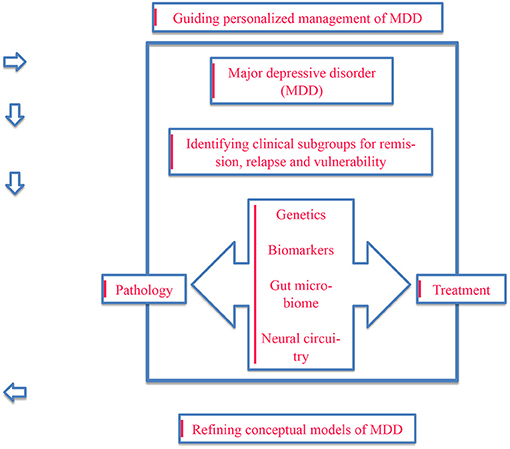
Figure 1. An illustration of the key concepts focusing on the need for conceptualizing and treating major depressive disorder from a precision psychiatry perspective using multiomics data.
Brief Historical Perspective
Research has substantially examined various risk and protective factors in relation to psychiatric disorders besides evidence-based treatments. With more advances and insights into the pathology of different disorders, a new concept of precision medicine has emerged. According to Fernandes et al. (1), precision psychiatry is a branch of precision medicine focused on enhancing prevention, diagnosis, and treatment of psychiatric disorders through identifying clinical subgroups in relation to specific disorders, identifying subgroups that may benefit from specific disorders, assessing the effectiveness of different interventions with different subgroups, and identifying risk and protective factors for remission, relapse, and vulnerability (1).
This approach is in line with the recommendations of Insel, former director of the National Institute of Mental Health (2) who highlighted the need for precision medicine for mental disorders and, for that goal, the integration of data covering different biopsychosocial aspects when considering risk and vulnerability of an individual. However, in order to achieve the ultimate goal of personalizing mental health, there is a need for comprehensive translational research that include data from clinical trials, routine care, and cohort studies.
For example, the newly established Center for Precision Mental Health and Wellness at Stanford University aims to bridge the knowledge gap between brain sciences and mental health based in an attempt to come up with effective, evidence-based translational approaches in assessment, diagnosis, and treatment of mental disorders, more specifically depression. This is based on the examination and classification of malfunctioning brain circuitry using advanced imaging technologies. Other mental health initiatives have taken a similar path toward the enhancement of the effectiveness of mental health services through the translation of neuroscience into clinical practice, especially with increasing prevalence of mental disorders, and therefore the demands for effective services, worldwide.
Depression, diagnostically recognized as major depressive disorder, is a major health concern affecting the lives of more than 264 million people worldwide and contributing to the disability and global burden of disease. With increasing and alarming global prevalence, it has become one of the priorities covered by WHO's mental health Gap Action Programme (mhGAP) calling for “a comprehensive, coordinated response to mental disorders at the country level” as per the 2013 World Health Assembly resolution (3). The aim of this review is to provide an overview of reported findings in the understanding of the pathology and treatment of depression from a precision mental health perspective and to report on potential challenges of the implementation of precision psychiatry in the treatment of depression.
Summary of the Established Principles
Earlier studies have attempted to show neural circuits underlying the pathogenesis of different manifestations of negative affective states in animal models (4) and have therefore inspired more recent neuroimagining research focusing on the neural circuitry of the depressed human brain. This is in line with the National Institute of Mental Health (NIMH) calling for mapping “the connectomes for mental illness” (NIMH's Strategic Plan, Objective 1, Strategy 1.3). Such endeavors have led to the conceptualization of depression as diseased brain networks rather than diseased brain regions reflecting the involvement of different neurotransmitter systems and different mechanisms in its pathology (5, 6).
Traditionally, depressive disorders are hypothesized to be directly linked with the processing of reward and punishment within different neural circuits (7). Disruptions in the topological organization of reward circuits have shown to predict severity of depressive symptoms and cognitive deficits associated with depression (8). More recently, studies have established more specific neural circuits in relation to depression, including treatment resistant depression (9).
Another ground-breaking approach toward conceptualizing depression is through identifying specific disease-related neural circuits building up on current knowledge of the large-scale circuits responsible for various cognitive and emotional processes. This is based on the assumption that functional and structural connectivity dysfunctions underlie specific “biotypes” of mental disorders. In this regard, it is argued that depression cannot be explained in terms of one pathological mechanism. For example, six neural circuit dysfunctions that have proven to be influential in both depression and anxiety have been identified in terms of their role in moderating salience, negative affect, positive affect, attention, and cognitive control in addition to the default mode (10).
Theoretical symptomatic biotypes based on six neural circuits are also proposed; these include rumination, anxious avoidance, negative bias, threat dysregulation, anhedonia, context insensitivity, inattention, and cognitive dyscontrol. In relation to precision medicine, such neural circuit biotypes can ideally guide personalized treatments for higher effectiveness. For instance, based on neural circuitry, it is suggested that patients with the negative affect biotype might mostly benefit from cognitive behavioral therapies compared to patients with the salience biotype who might mostly benefit from selective serotonin reuptake inhibitors and/or deep brain stimulation. In order to develop such an approach, use of quantitative metrics is recommended in order to categorize subgroups of patients fitting in each biotype and to identify symptoms in relation to neural circuit dysfunctions (10).
Current State of the Art
Genetics
Early researchers have attempted to demonstrate a link between genetics and mental disorders and in 1973, Gottesman and Shields introduced the concept of “endophenotype” in an attempt to categorize psychiatric symptoms into specific phenotypes hypothesized to be influenced by genetics. Neuropattern, a new concept used in diagnosis based on the idea of endophenotypes, was then proposed. One exploratory study showed its potential in showing a greater reduction in symptomatic severity and faster response to treatment in the Neuropattern group compared to standard care (11). Such approaches are based on the genetic variations underlying differences in brain circuitry which can explain the differences in affective, cognitive, and behavioral symptoms. With more advances in methodologies, it is estimated that genetics account for up to 42% of variations in response to antidepressants (12). Current knowledge about the role of some genes in the depression and response to treatment is growing quickly; however, most established findings are related to the pharmacokinetic genes, CYP2D6 and CYP2C19 (13) on which selective serotonin-reuptake inhibitors dosing guidelines are based (14). A recent review provided a summary of genetic studies examining specific single nucleotide polymorphisms (SNPs) that are relevant to response to antidepressants. These include COMT, HTR2A, HTR1A, CNR1, SLC6A4, NPY, MAOA, IL1B, GRIK4, BDNF, GNB3, FKBP5, CYP2D6, CYP2C19, and ABCB1 (15). It has also been demonstrated that multiple gene modules could represent cortical dysfunction in different disorders including major depressive disorder (16). In terms of its mechanism of action, such genes are involved in the up regulation of the hypothalamic-pituitary-adrenal (HPA) axis, therefore, contributing to the development of depressive symptoms. Looking into geneX environment interactions, preliminary research also suggests the potential use of epigenetics as biomarkers for predicting response to electroconvulsive therapy (ECT) which is commonly used in treatment-resistant and severe cases of depression (17).
Biomarkers
From an inflammatory perspective, depression can be regarded as aa pro-inflammatory condition marked by the different inflammatory biomarkers such as interleukin IL-6, TNFα, and C reactive protein (18). An alternative formulation of depression has been proposed building on the neurotrophic hypothesis which conceptualizes depression in terms of pathological alternations of growth factor proteins. One study aimed to examine the role of growth factor proteins in inpatients with treatment-resistant depression specifically (19). Blood analysis was done comparatively between patients and healthy controls to examine serum biomarkers. Findings suggest that, compared to healthy controls, patients had lower serum levels of brain-derived neurotrophic factors and vascular endothelial growth factor-C on one hand, and higher angioporetin-1 receptor biomarkers on the other hand. Additionally, and compared to patients who showed improvements in symptomatology, higher levels of vascular endothelial growth factor-D, and lower levels of vascular endothelial growth factor-C and F predicted treatment resistance in non-responders.
Psychosocial Markers
From a cognitive perspective, research has identified specific cognitive biases involved in the processing of affective information which can be used as indicators for specific symptomatology in mood disorders, including depression. This is in reference to “hot” cognitive processes, which are emotionally-loaded, as opposed to “cold” emotion-independent cognitions. For example, patients with major depressive disorder tend to have significant cognitive biases that contribute to their symptoms in addition to slow responses, poor response to positive stimuli, heightened sensitivity to negative stimuli, and a tendency to make negative interpretations of ambiguous stimuli (20). As discussed earlier, these biases can be linked with biological models of mood disorders. Research also suggests that such hot cognitive affective abnormalities can predict response to psychopharmacological treatment and that therefore they can be used as predictors of treatment efficacy in themselves (21, 22). According to Cotter and Barnett (23), such models can be used in translational advances such as identifying, managing at-risk individuals, and guiding treatment. For example, patients who do not have such cognitive biases are less likely to benefit from cognitive behavioral therapies that aims for cognitive restructuring for the alleviation of symptoms. They may still benefit from other interventions, either psychopharmacological or psychotherapeutic, that may target the symptomatology is this specific subgroup in order to optimize treatment.
Another systematic review generated a concept map for identifying patients requiring highly specialized treatment using non-metric multidimensional scaling and agglomerative hierarchical cluster analysis. The concept map was generated suggested 10 clusters of factors that predicted need for highly specialized interventions. These include severity, onset and treatment course, other psychiatric comorbidities including personality disorders and substance use disorders, somatic comorbidity, unhealthy coping styles, exposure to childhood trauma, social risk factors, and overall psychosocial dysfunction. Such findings highlight the need for comprehensive clinical assessment of patients using both clinical and non-clinical factors (24).
Treatment and Precision Psychiatry
Despite current knowledge of the heterogeneity of depression, treatments are almost still the same for patients from different clinical profiles. This may explain the finding that roughly 30% of patients with depression recover when using the first prescribed drug (25) and that an estimate of 55% will experience at least one side effect (26). The precision approach may enhance treatment choice as shown in a model involving the specification of a depressive subtype based on symptoms (i.e.: melancholic, psychotic, atypical, and anxious) and making drug choices accordingly. Within each subtype, the model proposes that treatment choice should take into consideration patient-related variables such as personality, personal preferences, comorbidity, and reactions to side-effects. In this regard, treatment is based on a bottom-up approach rather than a generic top-down approach that typically ignores differences in clinical profiles assuming homogeneity (27). Along the same lines, Saltiel and Silvershein (28) called for an adoption of a mechanism-based approach to pharmacotherapy based on the current knowledge of the effects of some drugs on specific clusters of symptoms of depression (anxiety, cognitive impairment, insomnia, hypersomnia, pain, fatigue, psychomotor problems, poor appetite, etc.) that are associated with specific neural circuits. Research also suggests that several factors may explain individual differences in responses to treatment. For example, several findings suggest a link between major depressive disorder and specific microbiome profiles and, similarly, between the use of antidepressants and the gut microbiota being mediated by different forms of antimicrobial activity (29). For example, as one of the most commonly prescribed antidepressants, citalopram is correlated with an increase in the Enterobacteriaceae family (30). Similarly, the use of antidepressants has been correlated with increased bacterial taxa, namely the Helicobacter, Asteroleplasma, Marinilactibacillus genera, Bacillus, and Succinivibrionaceae (31). Additionally, antidepressant pharmacokinetics have proven to differ based on age (32) and sex (33).
The effectiveness of pharmacogenetic-guided treatments in improving clinical outcomes in patients with major depressive disorder and in reducing potential side effects also supports the adoption of a personalized approach. For instance, one randomized controlled trial showed sustained improvement over a 12-week follow-up period and increased side effect tolerability when using pharmacogenetic testing for guiding drug choice (34). In the absence sound empirical evidence supporting the application of such techniques clinically, randomized controlled trials should be encouraged in order to research more genomic information that can foster precision medicine. One study is a prospective randomized controlled trial aiming to assess the effectiveness of pharmacogenetics-guided treatment in cases of depression compared to standard treatments. Using the NeuroIDgenetix® test to identify gene–drug and drug–drug interactions for different medications, results showed significantly higher response and remission rates among patients who received pharmacogenetics-guided treatment (35). Personalized psychiatry has also expanded to include the field of psychotherapy and more research have addressed the application of person-centered therapies in the treatment of depression [see, (36)]. However, more research is warranted in the area of personality psychotherapy, and combined psychotherapy, and pharmacotherapy.
A summary of all studies covered in section Current state of the art in provided in Table 1.
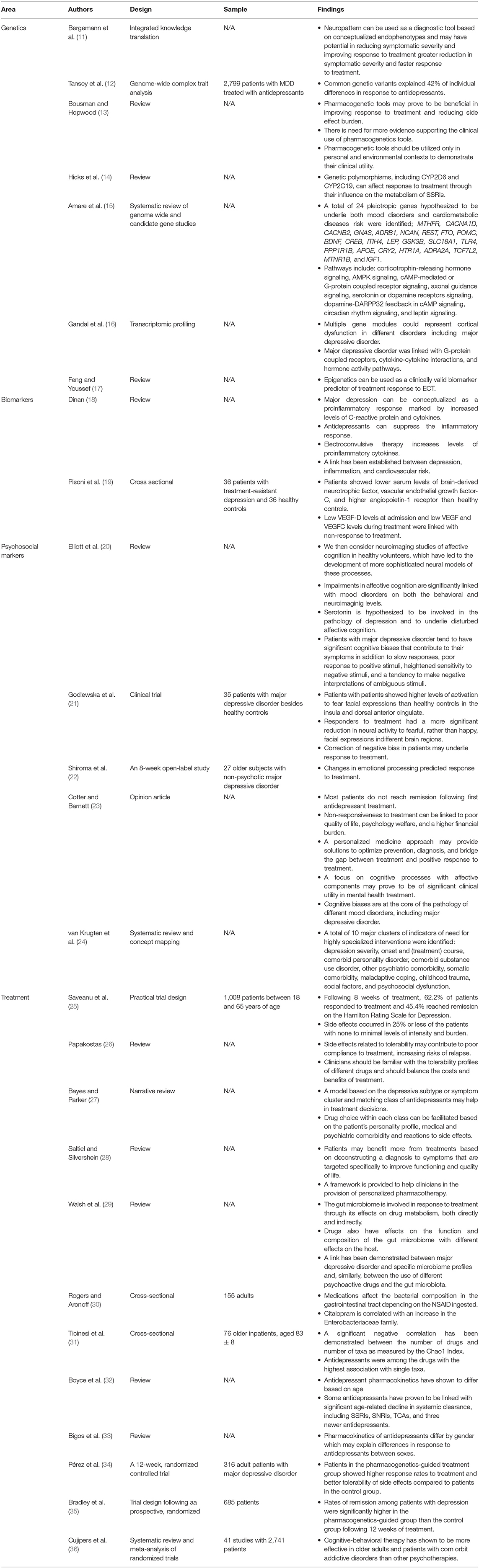
Table 1. A summary of studies on genetics, biomarkers, psychosocial markers, and the treatment of major depressive disorder from a personalized perspective.
Challenges in Application
Computer and information technologies have developed over the past years making it easier for research to utilize clinical datasets with high levels of accuracy and relevance, and possibly benefit from artificial intelligence-based modeling systems to predict disease and treatment outcomes. Well-established softwares have been introduced to the precision medicine software market including 2bPrecise, PierianDx, and others. Despite all advances toward adopting a personalized approach in mental health services, and more specifically in the treatment of depressive disorders, several challenges may still limit its clinical utility. One limitation hindering the generalization of such findings to real-life practice is the fact that the majority of clinical trials recruit patients with mild and moderate severities excluding those with more severe symptoms, making them not representative of the actual patient population. Additionally, there is wide disagreement about the validity of categorizing depression into specific subtypes based on symptomatology and the presence of specific endophenotypes (27).
Besides the clinical complexity of depressive disorders, one challenge in precision psychiatry is the technical complexity required to process and analyze multiomics data generated from multidimensional datasets and/or using artificial intelligence modeling. This, despite the availability of health records, may limit the ability to integrate patient information in a clinically relevant manner. Additionally, the variations in the technical means of data collection may be another factor limiting theutility of multiomics data (37). Additionally, the cost-effectiveness of some testing techniques is still not well-established in the first place (38).
Data-driven categorization in clinical practice may help facilitate further screenings and referrals of patients in a cost-effective manner; however, different costs associated with the application of precision psychiatry should also be considered. For example, using new reference paradigms require the appropriate training of healthcare staff in different aspects of precision medicine, including genomics, pharmacogenomics, biomarker in order to allow them to use and interpret generated data. Healthcare and medical research institutions may need to make use of advanced computational systems with additional costs required for the management of data security and privacy, data analysis and interpretation besides the risk of algorithmic failure (37). The costs required for the attainment and analysis of biomarkers and other precision tools may hinder their use in clinical practice, especially in low-income countries (39). This may also mean the presence of health disparities and inequities between patients who may or may not afford precision health services (40).
Various ethical concerns are of utmost importance in relation to precision psychiatry. First of all, protecting the security and privacy of data is challenging and is made more challenging while using electronic health records. Second, the concept of patient stratification, which is at the core of precision medicine, is another substantial concern given the risk of injustice or social discrimination against patients in less privileged groups (i.e., patients with expected poor prognosis) (41), or potentially less profitable patients (i.e., patients of specific socioeconomic backgrounds). Finally, and specific to the field of psychiatry, the use of artificial intelligence in clinical decision making [see (42)] may pose a special concern given the significance of human contact in mental health services.
Highlight of Future Directions
With more advances in telepsychiatry, research has explored the use of artificial intelligence in moving toward personalized management of mental disorders. For example, technology-based behavioral sensing may prove to be effective in measuring subjective functioning, making inferences about symptoms, and guiding treatment management. For example, self-help tool can be provided through downloadable mobile applications (43). Research does not only support the effectiveness of internet-based interventions in the self-management of depression, but also the symptom-specific effects of such interventions. For example, the well-established Deprexis program that demonstrated effectiveness in terms of symptomatic improvements and low dropout rates has also proven to be specifically effective in alleviating seven specific symptoms of depression in mild and moderate cases (44). Such findings support the need for high specificity in designing automated self-help programs (45).
Functional neuroimaging is another promising tool in guiding treatment decision by providing individual treatment response predictions. Through revealing the pretreatment activation level in some specific neural circuitries, it can be used to predict response to both pharmacological and psychotherapeutic treatments. Patients with higher pretreatment activation level of the ventral and pregenual anterior cingulate cortex (ACC) show better response to antidepressants than to psychotherapy. In light of such findings, functional neuroimaging may ideally guide treatment choice in different subtypes of depression (46). Similarly, a recent systematic review on the use of neuroimagining and behavioral predictors of treatment efficacy identified low baseline responsively in limbic regions of the brain and increased medial and dorsal prefrontal responses to emotional stimuli as the most consistently significant predictors of response to pharmacological treatment for depression (47).
Author Contributions
RD conceptualized and drafted the work. MS reviewed the work. All authors contributed to the article and approved the submitted version.
Conflict of Interest
The authors declare that the research was conducted in the absence of any commercial or financial relationships that could be construed as a potential conflict of interest.
References
1. Fernandes BS, Williams LM, Steiner J, Leboyer M, Carvalho AF, Berk M. The new field of “precision psychiatry”. BMC Med. (2017) 15:80. doi: 10.1186/s12916-017-0849-x
2. Insel T. Director's Blog: Precision Medicine for Mental Disorders. (2015). Available online at: https://www.nimh.nih.gov/about/directors/thomas-insel/blog/2015/precision-medicine-for-mental-disorders.shtml
3. World Health Organization. Depression: Fact Sheet. World Health Organization. (2020). Available online at: https://www.who.int/news-room/fact-sheets/detail/depression
4. Panksepp J. Affective Neuroscience: The Foundations of Human and Animal Emotions. New York, NY: Oxford University Press (1998).
5. Chaudhury D, Liu H, Han MH. Neuronal correlates of depression. Cell Mol Life Sci. (2015) 72:4825–48. doi: 10.1007/s00018-015-2044-6
6. Mayberg HS. Defining the neural circuitry of depression: toward a new nosology with therapeutic implications. Biol Psychiatry. (2007) 61:729–30. doi: 10.1016/j.biopsych.2007.01.013
7. Eshel N, Roiser JP. Reward and punishment processing in depression. Biol Psychiatry. (2010). 68:118–24. doi: 10.1016/j.biopsych.2010.01.027
8. Gong L, Yin Y, He C, Ye Q, Bai F, Yuan Y, et al. Disrupted reward circuits is associated with cognitive deficits and depression severity in major depressive disorder. J Psychiatr Res. (2017) 84:9–17. doi: 10.1016/j.jpsychires.2016.09.016
9. Barreiros AR, Chakouch C, Breukelaar I, Chen W, Rai S, Mayur P, et al. Neural circuits underlying treatment-resistant depression. Biol Psychiatry. (2020) 87:S311. doi: 10.1016/j.biopsych.2020.02.801
10. Williams LM. Precision psychiatry: a neural circuit taxonomy for depression and anxiety. Lancet Psychiatry. (2016) 3:472–80. doi: 10.1016/S2215-0366(15)00579-9
11. Bergemann N, Bruhn K, Loscheider K, Vogt D, Böhnke JR, Gerhards F. how to determine whether conceptual endophenotypes can improve clinical outcomes in patients suffering from major depression: an exploratory approach. Psychoneuroendocrinology. (2019) 105:195–204. doi: 10.1016/j.psyneuen.2019.03.010
12. Tansey KE, Guipponi M, Hu X, Domenici E, Lewis G, Malafosse A, et al. Contribution of common genetic variants to antidepressant response. Biol Psychiatry. (2013) 73:679–82. doi: 10.1016/j.biopsych.2012.10.030
13. Bousman CA, Hopwood M. Commercial pharmacogenetic-based decision-support tools in psychiatry. Lancet Psychiatry. (2016) 3:585–90. doi: 10.1016/S2215-0366(16)00017-1
14. Hicks JK, Bishop JR, Sangkuhl K, Muller DJ, Ji Y, Leckband SG, et al. Clinical Pharmacogenetics Implementation Consortium (CPIC) Guideline for CYP2D6 and CYP2C19 genotypes and dosing of selective serotonin reuptake inhibitors. Clin Pharmacol Ther. (2015) 98:127–34. doi: 10.1002/cpt.147
15. Amare AT, Schubert KO, Baune BT. Pharmacogenomics in the treatment of mood disorders: strategies and opportunities for personalized psychiatry. EPMA J. (2017) 8:211–27. doi: 10.1007/s13167-017-0112-8
16. Gandal MJ, Haney JR, Parikshak NN, Leppa V, Ramaswami G, Hartl C, et al. (2018). Shared molecular neuropathology across major psychiatric disorders parallels polygenic overlap. Science. 359:693–7. doi: 10.1126/science.aad6469
17. Feng T, Youssef NA. Can epigenetic biomarkers lead us to precision medicine in predicting treatment response and remission for patients being considered for ECT? Psychiatry Res. (2020) 284:112659. doi: 10.1016/j.psychres.2019.112659
18. Dinan TG. Inflammatory markers in depression. Curr Opin Psychiatry. (2009) 22:32–36. doi: 10.1097/YCO.0b013e328315a561
19. Pisoni A, Strawbridge R, Hodsoll J, Powell TR, Breen G, Hatch S, et al. Growth factor proteins and treatment-resistant depression: a place on the path to precision. Front Psychiatry. (2018) 9:386. doi: 10.3389/fpsyt.2018.00386
20. Elliott R, Zahn R, Deakin JF, Anderson IM. (2011). Affective cognition and its disruption in mood disorders. Neuropsychopharmacology. 36:153–82. doi: 10.1038/npp.2010.77
21. Godlewska BR, Browning M, Norbury R, Cowen PJ, Harmer CJ. Early changes in emotional processing as a marker of clinical response to SSRI treatment in depression. Transl Psychiatry. (2016) 6:e957. doi: 10.1038/tp.2016.130
22. Shiroma PR, Thuras P, Johns B, Lim KO. Emotion recognition processing as early predictor of response to 8-week citalopram treatment in late-life depression. Int J Geriatr Psychiatry. (2014) 29:1132–9. doi: 10.1002/gps.4104
23. Cotter J, Barnett JH. Using affective cognition to enhance precision psychiatry. Front Psychiatry. (2018) 9:288. doi: 10.3389/fpsyt.2018.00288
24. van Krugten FCW, Goorden M, van Balkom AJLM, Spijker J, Brouwer WBF, Hakkaart-van Roijen L. Indicators to facilitate the early identification of patients with major depressive disorder in need of highly specialized care: a concept mapping study. Depress Anxiety. (2018) 35:346–52. doi: 10.1002/da.22741
25. Saveanu R, Etkin A, Duchemin AM, Goldstein-Piekarski A, Gyurak A, Debattista C, et al. The international study to predict optimized treatment in depression (iSPOT-D): outcomes from the acute phase of antidepressant treatment. J Psychiatr Res. (2015) 61:1–12. doi: 10.1016/j.jpsychires.2014.12.018
27. Bayes A, Parker G. How to choose an antidepressant medication. Acta Psychiatr Scand. (2018) 139:280–91. doi: 10.1111/acps.13001
28. Saltiel PF, Silvershein DI. Major depressive disorder: mechanism-based prescribing for personalized medicine. Neuropsychiatr Dis Treat. (2015) 11:875–88. doi: 10.2147/NDT.S73261
29. Walsh J, Griffin BR, Clarke G, Hyland NP. Drug-gut microbiota interactions: implications for neuropharmacology. Br J Pharmacol. (2018) 175:4415–29. doi: 10.1111/bph.14366
30. Rogers MAM, Aronoff DM. The influence of non-steroidalanti-inflammatory drugs on the gut microbiome. lin Microbiol Infect. (2016) 22:178.e1–9. doi: 10.1016/j.cmi.2015.10.003
31. Ticinesi A, Milani C, Lauretani F, Nouvenne A, Mancabelli L, Lugli GA, et al. Gut microbiota composition is associated withpolypharmacy in elderly hospitalized patients. Sci Rep. (2017) 7:11102. doi: 10.1038/s41598-017-10734-y
32. Boyce RD, Handler SM, Karp JF, Hanlon JT. Age-related changes in antidepressant pharmacokinetics and potential drug-drug onteractions: a comparison of evidence-based literature and package insert information. Am J Geriatr Pharmacother. (2012) 10:139–50. doi: 10.1016/j.amjopharm.2012.01.001
33. Bigos KL, Pollock BG, Stankevich BA, Bies RR. Sex differences in the pharmacokinetics and pharmacodynamics of antidepressants: an updated review. Gender Med. (2009) 6:522–43. doi: 10.1016/j.genm.2009.12.004
34. Pérez V, Salavert A, Espadaler J, Tuson M, Saiz-Ruiz J, Sáez-Navarro C, et al. Efficacy of prospective pharmacogenetic testing in the treatment of major depressive disorder: results of a randomized, double-blind clinical trial. BMC Psychiatry. (2017) 17:250. doi: 10.1186/s12888-017-1412-1
35. Bradley P., Shiekh M, Mehra V, Vrbicky K, Layle S, Olson MC, et al. Improved efficacy with targeted pharmacogenetic-guided treatment of patients with depression and anxiety: a randomized clinical trial demonstrating clinical utility. J Psychiatr Res. (2018) 96:100–7. doi: 10.1016/j.jpsychires.2017.09.024
36. Cujipers P, Ebert DD, Acarturk C, Andersson G, Cristea IA. Personalized psychotherapy for adult depression: a meta-analytic review. Behav Ther. (2016) 47:966–80. doi: 10.1016/j.beth.2016.04.007
37. Uddin M, Wang Y, Woodbury-Smith M. Artificial intelligence for precision medicine in neurodevelopmental disorders. NPJ Digit. Med. (2019) 2:112. doi: 10.1038/s41746-019-0191-0
38. Berm EJ, Looff M, Wilffert B, Boersma C, Annemans L, Vegter S, et al. Economic evaluations of pharmacogenetic and pharmacogenomic screening tests: a systematic review. Second update of the literature. PLoS ONE. (2016) 11:e0146262. doi: 10.1371/journal.pone.0146262
39. Vieta E. Personalized medicine applied to mental health: precision psychiatry. Rev Psiquiatr Sal Ment Engl Edn. (2015) 8:117–8. doi: 10.1016/j.rpsmen.2015.03.007
40. Hekler E, Tiro JA, Hunter CM, Nebeker C. Precision health: the role of the social and behavioral sciences in advancing the vision, Ann Behav Med. (2020) 54:805–26. doi: 10.1093/abm/kaaa018
41. Batten JN. How stratification unites ethical issues in precision health. AMA J Ethics. (2018) 20:E798–803. doi: 10.1001/amajethics.2018.798
42. Lamanna C, Byrne L. Should artificial intelligence augment medical decision making? The case for an autonomy algorithm. AMA J Ethics. (2018) 20:E902–10. doi: 10.1001/amajethics.2018.902
43. Aung MH, Matthews M, Choudhury T. Sensing behavioral symptoms of mental health and delivering personalized interventions using mobile technologies. Depress Anxiety. (2017) 34:603–9. doi: 10.1002/da.22646
44. Twomey C, O'Reilly G, Meyer B. Effectiveness of an individually-tailored computerized CBT programme (deprexis) for depression: a meta-analysis. Psychiatry Res. (2017) 256:371–7. doi: 10.1016/j.psychres.2017.06.081
45. Boschloo L, Cujipers P, Karyotaki E, Berger T, Moritz S, Meyer B, et al. Symptom-specific effectiveness of an internet-based intervention in the treatment of mild to moderate depressive symptomatology: the potential of network estimation techniques. Behav Res Ther. (2019) 122:103440. doi: 10.1016/j.brat.2019.103440
46. Ball TM, Stein MB, Paulus MP. Toward the application of functional neuroimagining to individualized treatment for anxiety and depression. Depress Anxiety. (2014) 31:920–33. doi: 10.1002/da.22299
Keywords: depression, neural circuitry, psychosocial markers, biomarkers, precision mental health
Citation: Deif R and Salama M (2021) Depression From a Precision Mental Health Perspective: Utilizing Personalized Conceptualizations to Guide Personalized Treatments. Front. Psychiatry 12:650318. doi: 10.3389/fpsyt.2021.650318
Received: 06 January 2021; Accepted: 13 April 2021;
Published: 11 May 2021.
Edited by:
Tetsufumi Kanazawa, Osaka Medical College, JapanReviewed by:
Michaela Filiou, University of Ioannina, GreeceCarlos M. Opazo, University of Melbourne, Australia
Copyright © 2021 Deif and Salama. This is an open-access article distributed under the terms of the Creative Commons Attribution License (CC BY). The use, distribution or reproduction in other forums is permitted, provided the original author(s) and the copyright owner(s) are credited and that the original publication in this journal is cited, in accordance with accepted academic practice. No use, distribution or reproduction is permitted which does not comply with these terms.
*Correspondence: Reem Deif, reem.deif@aucegypt.edu