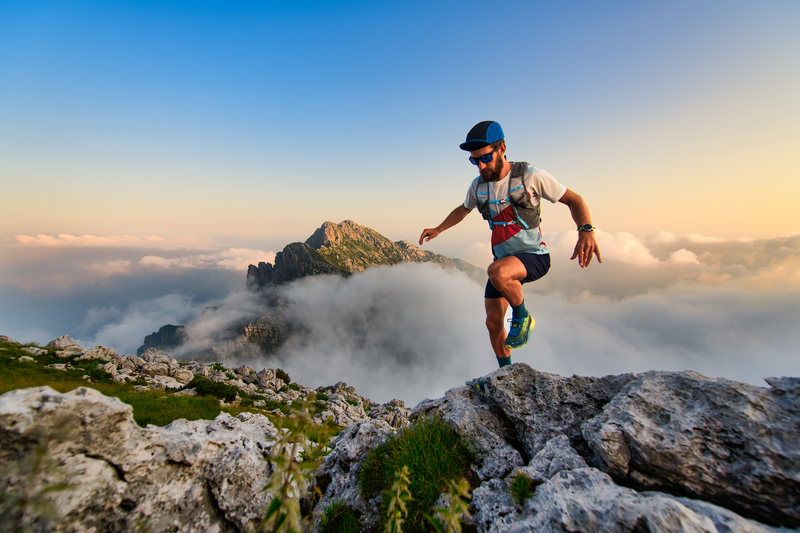
94% of researchers rate our articles as excellent or good
Learn more about the work of our research integrity team to safeguard the quality of each article we publish.
Find out more
ORIGINAL RESEARCH article
Front. Psychiatry , 17 June 2021
Sec. Child and Adolescent Psychiatry
Volume 12 - 2021 | https://doi.org/10.3389/fpsyt.2021.647243
This article is part of the Research Topic Spatial Navigation and Neurodevelopmental Disorders View all 8 articles
Background: Topographic memory is the ability to reach various places by recognizing spatial layouts and getting oriented in familiar environments. It involves several different cognitive abilities, in particular executive functions (EF), such as attention, working memory, and planning. Children with attention deficit hyperactivity disorder (ADHD) show impairments in inhibitory control, regulation of attention, planning, and working memory.
Aim: The aim of this study was to evaluate the topographic memory in children with ADHD-combined subtype (ADHD-C).
Method: Fifteen children (8–10 years) with a diagnosis of ADHD-C (DSM-5) (ADHD-C group) were compared to 15 children with typical development (TD group) of the same age. All children performed Raven's colored progressive matrices (CPM) test to obtain a measure related with cognitive functioning. The walking Corsi test (WalCT), a large-scale version of the Corsi block-tapping test, was used to assess topographic memory in experimental environment.
Results: A higher impairment was observed in ADHD-C than TD with significant differences in the WalCT, in particular on the topographic short-term memory (TSTM) task, on the topographic learning (TL) task, and on the repetition number (RN) task during the TL task. Perseverative errors were reported in performing the square-sequence in the WalCT. Zero-order correlations showed a positive correlation between TSTM and auditory attention, and memory of design of NEPSY-II and digit span of WISC-IV. No statistically significant differences were found between the ADHD-C group and TD group in the TL task in the WalCT condition.
Conclusion: In ADHD-C, initial topographic learning was compromised whereas the long-term retention of learned topographical material seemed to not be impaired. In particular, these impairments seem to be linked with difficulties in sustained attention, in spatial memory for novel visual materials, in a poor working memory, and in perseverative behaviors.
Human spatial cognition is a fundamental ability in humans. The visual and tactile world consists of objects situated in space, so it is essential from the first months of life to understand the characteristics of the surrounding area and object positions in it. This knowledge allows children to mentally and physically organize objects in their world. Indeed spatial awareness and spatial relations allow children to locate objects and navigate successfully in their environment, while spatial language allows children to express specific needs and describe the world (1).
Topographic memory is one component of spatial cognition that corresponds to the ability to reach various places in the environment, recognize spatial layouts, orient in familiar environments (2, 3), and to encode and maintain online sequences of environmental cues that are central during navigation (4, 5).
Topographic memory involves several cognitive processes and skills such as the ability to retain the spatial layout of an environment, to memorize and recognize complex visuo-spatial configurations, to find a shortcut between two locations, or to create an interconnected network among different paths (6–8). For a successful topographic memory, individuals have to access at least two types of spatial representations: the online representation of their position in the environment, and the offline representation of the environment (9). Therefore, it is essential that the individual create a stable mental representation of the environment, representing a “cognitive map” (7, 10, 11).
Indeed, human navigation develops gradually at distinct time points (12, 13), in parallel with the development of executive functions (EF) and language (5). By the age of 6–9 months, children are just able to use path integration, that is the ability to memorize their movements in the environment, and to get oriented on the basis of geometric features of the environment. They become able to deal with a spatial array from a novel viewpoint using landmarks only at 5 years old (5). Cognitive mapping starts to develop very late, at 7–8 years of age, and is fully functional by the age of 10 (12). However, the accuracy and the efficiency of navigational abilities continues to improve into adolescence, depending, at least in part, on the maturation of sensory and motor systems, combined with environmental feedback. In order to navigate in a successful way, humans must be able to process, integrate, and manipulate information derived from internal and external factors pertaining to time and space. The body's sensations and perceptions, environmental signals, and landmarks or reference points, are equally important for reaching a place and memorizing a path (13).
Successful navigation is known to be strictly connected with the activity of several brain areas such as parieto-medial temporal networks (14), the posterior cingulate cortex (PCC), the retrosplenial cortex (RSC) (15), and the medial temporal lobe structures. Several neuroimaging studies have also revealed an increased activity in prefrontal areas during spatial navigation tasks (16–20). As described above, human navigation is constituted by multicomponent abilities, which need several cognitive processes, such as attention, memory, perception, and decision-making, for correct development and functioning. Indeed, even a single impairment in these processes may negatively affect navigation (21–24). Recently, in the adult population, several variable levels of navigational skills were reported and a specific developmental disorder, developmental topographical disorientation (DTD), was described. This disorder is characterized by a life-long inability in orientation despite otherwise well-preserved cognitive functions, and without other neurological conditions (25–31). This suggests the importance of understanding if and how the development of navigational skills is affected by the development of the other cognitive domains, particularly executive functions with specific attention toward working memory that is known to be important in maintaining online navigational information.
In this regard, the investigation of the spatial navigation in children, presenting this selective impairment, may shed light on those factors causative of the development of navigational skills. For this purpose, the study of topographic memory in children with attention deficit hyperactivity disorder (ADHD) could be crucial as a disorder of EFs, which are proposed to be a core deficit of this pathology (32, 33). Willcutt et al. (34) conducted a meta-analysis to evaluate the performance in executive function tasks in children and adolescents with ADHD showing weaknesses in several key EF domains, but the strongest and most consistent effects were obtained on measures of response inhibition, vigilance, spatial working memory, and some measures of planning. One of the most prominent cognitive weaknesses in patients with ADHD appears to be visuospatial working memory (VSWM), including short-term memory (STM) and central executive (CE) function (35). ADHD-combined subtype (ADHD-C), described as the more pervasive and impairing form of the disorder (36), shows significantly higher impairing rates in the functioning of vigilance, sustained attention, visual attention task, especially inhibition and shifting, visuo-spatial short-term memory, working memory, short-term memory compared to TD, and the other ADHD subtypes (37–41). Pasini et al. (42) conducted a study on attention and executive functions profile in drug-naive ADHD subtypes, founding that ADHD patients, inattentive and combined subtypes, differ from controls on response inhibition, divided attention, phonological, and visual object working memory and on variability of reaction times (43).
Attention and executive functions (EF), in particular inhibition and VSWM, play a critical role in the development of topographic memory, so it is important to investigate the development of topographic memory in children with ADHD that tend to fail in tasks both requiring an attentional load and involving EF (44–46). Furthermore, deficits in executive functions could also affect learning processes. In particular, several studies and clinical evidence (47–50) showed that children with ADHD present an impairment in sustained attention, flexibility, problem solving, and lower levels of task persistence, which has an impact on learning processes.
To investigate the development of navigational skills in ADHD children, it is important to consider that the age period of 8–10 years represents a crucial developmental phase for both executive functions and navigational skills [e.g., (4, 51–54)]. Previous studies found that the abilities to bind geometric environmental features, landmark identity, and directional estimations get developed at the age of 6–8 years, in accordance with the development of visuospatial processing and language (5, 7). Egocentric information, which includes spatial information about the location of the subject in the environment, is gradually supplemented with allocentric coding, which involves spatial information about the reciprocal object's positions (55). Furthermore, the relation-place strategies required for cognitive mapping start to develop around 7–8 years of age and are fully functioning by the age of 10 (56–60). It is important to note that several previous studies showed a cortical maturation delay in ADHD children, in particular in the prefrontal regions involved in attention, planning, and navigational abilities [e.g., (61–64)].
The aim of this study was to evaluate the topographic memory in children with ADHD-C compared with a group of typical developmental (TD) children. To this purpose, we used the WalCT (4, 51, 65, 66), a test widely used both in clinical and experimental settings to study topographic memory. This study was novel in its attempt to compare the performance of children with ADHD-C with a control group without any ADHD symptoms on topographical memory. The aim was to address the following questions:
1) Does the ADHD-C group perform poorly on the topographic short-term memory task compared to TD children?
Based on previous studies that confirmed a strong relationship between working memory and navigation (4, 67–74) and based on previous results showing low working-memory performances in children with ADHD [e.g., (75)], poor performance on the topographic short-term memory (TSTM) task in children with ADHD-C compared to TD children was expected. To support of this hypothesis, Kofler et al. (76) found that impaired working memory in children with ADHD determined consistent difficulties in anticipating, planning, enacting, and maintaining goal-directed actions, all fundamental abilities for successful navigation.
2) Does the ADHD-C group perform poorly on topographic learning task compared to TD children?
To our knowledge, this study is the first that assesses, in children with ADHD-C, not only some aspects of topographic short-term memory, but also the ability to learn new paths. It is known that sustained attention and flexibility in problem solving play an important role in learning and that these abilities seem to be compromised in children with ADHD (47, 49, 50). For such a reason, we also expected to find deficits in learning paths in children with ADHD-C but not in children with TD.
3) Does the ADHD-C group perform poorly on the topographic delayed recall (TDR) task compared to TD children?
Few studies have assessed long-term memory (both learning and delayed recall) in children with ADHD. A recent meta-analysis (77) found that adults with ADHD performed significantly worse than controls on verbal but not on visual long-term memory and memory acquisition subtests. A long-term memory deficit was strongly statistically related to memory acquisition deficits. In contrast, no retrieval problems were observable suggesting that memory deficits in adults with ADHD reflect a learning deficit induced at the stage of encoding. Furthermore, Skowronek (78) showed equal or enhanced performance on long-term episodic tasks in children with ADHD compared to TD children. Based on this evidence, we hypothesize that we will not find any differences in long-term memory between the two groups.
4) Are there any correlations between topographic memory and executive function performances in the ADHD-C group?
Topographic memory involves several different cognitive abilities, in particular executive functions (EFs), such as attention and working memory (21, 22, 24). To date no studies have evaluated the correlations between topographic memory and executive function performances in ADHD-C children, we expected a positive correlation between topographic short-term memory (TSTM) and the “attention and executive function” domain of NEPSY-II and topographic short-term memory (TSTM) and the working memory index of WISC-IV.
It is important to note that some navigational tasks might be impaired in both groups (children with ADHD and TD) because of age/stage of development. In particular, we considered the ability to use cognitive maps. Indeed, Lehnung et al. (56) showed that 10-year-old children tended to use cognitive-map strategies at greater rates and generally made fewer errors than younger children in a spatial orientation task, furthermore children who suffered from a traumatic brain injury before 10 years of age showed greater long-term impairments in the ability to orient themselves by means of cognitive maps. Newcombe (13) reported that by around 12 years, we can observe adult-level performance and adult patterns of individual differences on cognitive mapping tasks and these abilities continue to improve, along with the adaptive combination of various kinds of input, at least until adolescence. Each of these lines of development depends at least in part on the maturation of sensory and motor systems, combined with environmental feedback. Taken together, these findings suggest that several complex spatial cognition abilities develop rapidly between 7 and 12 years of age (79). Furthermore, different complex navigational skills depend on the development and maturation of several networks and brain areas such as the hippocampus, retrosplenial cortex, and prefrontal cortex (PFC) whose development is prolonged, continuing into adolescence [e.g., (15, 51, 80)].
In addition, the relation-place strategies required for cognitive mapping start to develop around 7 or 8 years of age and are fully functional by the age of 10 (56–60). Therefore, both groups, with ADHD-C and TD children, might have difficulties in transforming egocentric information into an allocentric representation of the experimental setting.
For this study, we assessed a sample of 100 children and adolescents (aged between 5.1 and 15.4 years), that had been referred to the Department of Human Neuroscience with a diagnostic suspicion of ADHD by parents, teachers, or a pediatrician. All children and parents underwent an initial interview with a child neuropsychiatrist who recorded the child's medical history, followed by an evaluation performed by a multidisciplinary team of experts constituted by a neuropsychiatrist and four psychologists. For the diagnosis, several neuropsychological and emotional tests were administered (see Supplementary Material).
After this assessment, for the ADHD-C group, patients needed to meet the following criteria for hyperkinetic disorder (HKD) for ICD-10 (81) and ADHD-C (48): six (or more) symptoms of hyperactivity and impulsivity and six (or more) symptoms of inattention persisting for at least 6 months to a degree that is inconsistent with developmental level and that negatively impacts social and academic/occupational activities. We chose to focus on the ADHD-C subtype because it defines a more pervasive and generally more impairing form of the disorder (36). In particular, some studies highlighted an association between specific subtypes of ADHD and TD and specific executive function impairments (38–40). In particular, children and adolescents with the ADHD-C subtype seemed to show significantly more impaired functioning on perseverative errors, visual attention tasks, especially inhibition and shifting, visuo-spatial short-term memory, working memory, and shortterm memory compared to TD and the other ADHD subtypes (38–41, 82). Furthermore, besides having a diagnosis of ADHD-C, the individuals included in the study had to meet the following criteria of inclusion: age 8–10, I.Q. ≥85, an average score on Raven's colored progressive matrices (percentile rank ≥ 37.5 = category average) (83), no previous treatment, no other diagnosis of a comorbidity, no primary visual or hearing impairments, and no other neurological or organic disease. After they were initially screened to determine their eligibility based on the aforementioned criteria, a sample of 15 children (age range: 8–10 y, M= 8.73, s.d.: 0.70; M:12; F:3) with a diagnosis of ADHD-C (experimental group: EG), based on DSM-5 (48) were recruited from the Department of Human Neuroscience.
We conducted an informal interview with parents of children that took part in the study as the control group (TD group) before the enrolment screening. None of these children had ever been previously reported for emotional, behavioral, or learning difficulties. No primary visual and hearing impairments, neurological and organic disease, or other neurodevelopmental issues were described. The individuals included in the study as TD had to meet the following criteria of inclusion: age 8–10, an average score on Raven's colored progressive matrices (percentile rank ≥ 37.5 = category average) (83), no emotional, behavioral, or learning difficulties, no primary visual or hearing impairments, no other neurodevelopmental, neurological, or organic disease. A typical developmental (TD) sample of 15 children (age range: 8–10 y, M = 8.87, s.d.: 0.83; M:10 F:5), with an average score on Raven's colored progressive matrices (percentile rank ≥ 37.5 = category average) [see Table 1; (84, 85)] without ADHD took part in the study as the comparison group (TD group).
We administered Raven's colored progressive matrices to both groups to obtain a measure of non-verbal intelligence and to exclude the presence of a visuospatial deficit. All subjects were of Caucasian origin and came from families with middle-class socio economic status (class 2: household income = €28,000–55,000; current Italian economic parameters), confirmed during the interview with parents. TD children were recruited from primary schools, describing the study protocol individually to their parents. Table 1 reports the descriptive statistics for the sample.
An informed written consent form, requiring active consent from caregivers, was signed. Each child, before taking part in the study, give an informed verbal assent. This research has been revised and approved by the Local Ethics Committees (Protocol number:416/16) in accordance with the tenets of the latest Declaration of Helsinki.
All children in both the groups performed Raven's CPM to obtain a cognitive functioning measurement. The CPM is internationally recognized as a culture-fair test of non-verbal intelligence (84, 85) designed for the use of children between the ages of 5½ and 11½. The test consists of 36 items in three sets (A, Ab, B), with 12 items per set (86). As reported in Table 1, the ADHD-C group and TD group fell in the average range (ADHD-C group, raw score = 24.67, TD group, raw score = 27.67).
The WalCT (4, 5, 51, 65, 66) (Figure 1), a large-scale version of the Corsi block-tapping test [CBT: (87)] was used to assess short- and long-term topographic memory. Specifically, the WalCT measures the memory of short paths in the navigational vista space, which is, according to Wolbers and Wiener (88), the portion of the navigational space that can be seen from a single location or with little exploratory movements.
Figure 1. (A) Walking Corsi test (WalCT). The WalCT was the apparatus used for measuring topographical memory. Nine black squares (30 × 30 cm) were placed on a 2.50 × 3.00 m white carpet. The scaled position and the relative spatial layout of the squares were the same as in the Corsi block-tapping test (CBT). (B) The outline map of the WalCT on which children had to draw the previously learned path. Written informed consent was obtained from the parents of the child represented in the figure for publication of this experiment. A copy of the written consent is available for review by the Editor-in-Chief of this journal.
It was set up in a wide room of the Department of Human Neuroscience. It was composed of nine green squares (30 × 30 cm) that were placed on a carpet on the floor, reproducing the same standard positions as used in the CBT (see Figure 1A). In this test, the subject had to walk and reach different locations. The experimenter illustrated the sequence by walking on the carpet and stopping on each square for 2 s. Then, the subject had to repeat the same sequence as the experimenter by walking and stopping on the squares included in the sequence.
Following standard procedures (51, 65, 66), participants performed three different tasks, assessing:
1) Topographic short-term memory (TSTM), in which a square span was obtained, that is, the number of squares in the longest sequence of squares that a child was able to repeat in the correct order immediately after the presentation.
During the task, the number of squares to be reproduced gradually increased in length (from a 2-square sequences to a 9-square sequences). Children were required to reproduce the square sequences shown by the examiner by actually stepping on the green squares included in the sequence previously shown by the examiner.
2) Topographic learning (TL), in which participants were asked to learn a fixed supra-span sequence (a path), which was calculated by considering the square span of the child + 2 squares according to standard procedures (5, 89). In each trial, after the examiner presented the sequence, the child was invited to walk on the carpet to reproduce it, stepping out of the carpet when he/she had finished. In each trial, the number of correct black squares reproduced in the sequence was calculated as the final score. During the task no feedback about the correctness of performance was provided. The learning criterion (indicating that learning was achieved) corresponded to three consecutive correct reproductions in a row of the sequence; in case the child did not reach the learning criterion the sequence was repeated for a maximum of 18 trials (5, 89).
3) Topographic delayed recall (TDR) of the supra-span sequence, in which 5 min later (the child and examiner spent this time out of the room where the WalCT was placed), participants were required to show the learned sequence. The examiner did not remind the child of the sequence and the child had to reproduce what he/she remembered of the long previously learnt sequence.
At the end of the TDR task, the children had to use a felt-tip marker to retrace the pathway they had learned on the outline map of the WalCT (Figure 1B).
The whole protocol was administered in a single session on ~30 min at the Department of Human Neuroscience.
For the aim of this study, descriptions and scores of the “attention and executive function” and “memory and learning” domains of NEPSY II (90) and the working memory indexes of WISC IV (91, 92) are reported.
In NEPSY II (90), the subtests administered to the children with ADHD in the domain “attention and executive function” were as follows:
Animal sorting (AS): assessing the ability to formulate basic concepts, to sort those concepts into categories, and to shift from one concept to another.
Auditory attention (AA): assessing selective auditory attention and the ability to sustain it (vigilance).
Visual attention (VA): assessing the ability to sustain selective visual attention.
Response set (RS): the first task assesses the ability to shift and maintain a new and complex set involving both the inhibition of previously learned responses and correctly responding to matching or contrasting stimuli. In the second task, the child listens to a series of words and touches the appropriate circle when he/she hears a target word.
Design fluency (DS): assessing the child's ability to generate unique designs by connecting up to five dots, presented in two arrays: structured and random.
Inhibition (IN): assessing the ability to inhibit automatic responses in favor of novel responses and the ability to switch between response types (90).
The scores of the children with ADHD on the “attention and executive function” subtests of NEPSY II (90) are reported in Table A1.
In NEPSY II (90) the subtests administered to children with ADHD in the “memory and learning” domain are as follows:
List memory (LM): assessing verbal learning and memory, rate of learning, and the role of interference in recall for verbal material.
Memory for designs (MFD): assessing the spatial memory for novel visual material.
Memory for faces (MFF): assessing the ability to encode facial features, as well as face discrimination and recognition.
Memory for names (MFN): assessing the ability to learn the names of children over three trials.
Narrative memory (NM): assessing the memory for organized verbal material under free recall, cued recall, and recognition conditions.
Sentence repetition (SR): assessing the ability to repeat sentences of increasing complexity and length.
Word list interference (WLI): assessing the verbal working memory, repetition, and word recall following interference (90).
The scores of the children with ADHD on the “memory and learning” subtests of NEPSY II (90) are reported in Table A2.
Working memory indexes This index is a measure of an individual's ability to hold verbal information in short-term memory and to manipulate that information. It consists of two subtests:
Digit span (DS): The examiner reads a series of numbers and the child has to repeat them. Then, the examiner again reads a series of numbers but this time the child is required to say them back in reverse order.
Letter number sequencing (LNS): The examiner reads a series of letters and numbers and the child is required to repeat them back with the letters in alphabetical order and the numbers in numerical order (The WISC IV and WAIS IV Subtests, Weiss, data, 2003). Table A3 reports the working memory indexes (WMI) of WISC IV (91, 92) of children with ADHD.
To compare the performances of the two groups (ADHD-C vs. TD group) in the WalCT, we used the g formula of Hedges and Olkin (93), which can be interpreted with the same rules as Cohen's d (94). Indeed, Hedges' g and Cohen's d are extremely similar except when the sample size is small, in this case, Hedges' g outperforms Cohen's d (95). Values for Hedges' g that range from 0.20 to 0.49 are reported as small effect sizes. Values that range from 0.50 to 0.79 are reported as moderate effect sizes, and values of 0.80 or more are reported as large effect sizes. Rosenthal (96) added a classification of very large, defined as being equivalent to or >1.30. In addition to the effect size, we computed the 95% confidence interval (CI) for the effect size, which is usually interpreted as the range of values that encompass the population, or the true value, estimated by a certain statistic with a given probability (97–99). According to Nakagawa and Cuthill (100), when we have a mean difference of 28 with 95% CI = −1 to 59, the result is not statistically significant (at an α level of 0.05) because the CI includes zero, while another mean difference of 28 with 95% CI = 9–49 is statistically significant because the CI does not include zero. Differences in the number of perseverative errors, committed by the two groups during WalCT, were evaluated by the Chi-square (Chi2) test. Zero-order correlation was conducted to measure the strength and direction of association that exists between the ADHD-C group's performances in the WalCT and the performances in NEPSY II (90) (attention, executive function, and memory and learning domains) and in the WISC-IV (working memory index) (91, 92).
To our current knowledge, this is the first study evaluating topographic memory in children with ADHD-C of a school age (ranging from 8 to 10 years) thus we conducted a post-hoc power analysis (i.e., OBSERVED power) based on the effect size, sample size, and parameter estimate from the data set, which found the following observed power for the variables considered: TSTM (0.93), TL (0.67), RN (0.76), OM (0.26), WalCT perseverative errors (0.88).
Regarding the control variables of age and Raven score, the two groups were balanced for age but not for Raven score with a moderate Hedges' g (ADHD-C group: M = 24.67, SD = 5.67; TD group: M = 27.67, SD = 2.44; g: −0.67; CI = −1.40 to 0.07).
However, in examining the 95% CI for the effect size, for the Raven score, a mean difference of −3 with a 95% CI = −1.40 to 0.07 was not statistically significant because the CI included zero. Furthermore, the two groups were balanced for gender; the ADHD group was composed of three girls and 12 boys and the TD group consisted of five girls and 10 boys [ = 0.682; p > 0.05]. All children of both groups were of Caucasian origin and came from families with middle-class socio economic status.
In our sample, the majority of children with ADHD showed below-average performances in the attention and executive function domain of NEPSY II (90), in particular 67% of children performed below average on the animal sorting (AS) subtest, 80% on the auditory attention (AA) subtest, 40% on the visual attention (VA) subtest, 80% on the responses et (RS) subtest, 60% on the design fluency (DF) subtest, and 74% on the inhibition (IN) subtest (Table A1).
In the memory and learning domain of NEPSY II (90), 60% of children performed below average on the list memory (LM) subtest, 47% on the design fluency (DF) subtest, 40% on the memory for designs (MFD) subtest, 33% on the memory for faces (MFF) subtest, 20% on the memory for names (MFN) subtest, 47% on the narrative memory (NM) subtest, 47% on the sentence repetition (SR) subtest, and 47% on the word list interference (WLI) subtest (Table A2).
Below average performances on the working memory index of the WISC-IV (91, 92) were reported by 40% of children with ADHD, 27% on the digit span (DS) subtest and 33% on letter number sequencing (LNS) (Table A3).
By comparing the ADHD group with the TD group, it emerged that the ADHD group had significantly lower performance in several tasks. In particular, the Hedges' g ranged from 0.50 to 1.67 (i.e., from medium effect to very large effect) on the topographic short-term memory (TSTM) task, topographic learning (TL) task, repetition number task during the TL task, and the WalCT outline map task (Table 2); however, in examining the 95% CI for the effect size, for the WalCT outline map task, a mean difference of 23.34 with a 95% CI = −1.22 to 0.23 was not statistically significant because the CI included zero (Table 2). All children of both groups correctly performed the topographic delayed recall (TDR) task without errors, so this score was not inserted into the table. The skewness and kurtosis statistics appeared to be very dependent on the sample size. Smaller sample sizes can give results that are very misleading. The statistics for skewness and kurtosis simply did not provide any useful information beyond that already given by the measures of location and dispersion (101).
For the perseverative errors in the WalCT, the Chi2 test showed significant differences between the two groups with respect to the distribution of the levels of the variables [ = 8.88, p = 0.003]. In particular, the ADHD group committed a significantly higher number of perseverative errors than the TD group in the WalCT (Table 3).
Zero-order correlations showed a positive correlation between the topographic short-term memory (TSTM) task and auditory attention (AA) task (r = 0.55, p = 0.03), and the memory of design task (r = 0.67, p = 0.01) of NEPSY II, and the digit span task (r = 0.54, p = 0.04) of WISC-IV (Table 4). Furthermore, a correlation between delayed recall and response set (RS) (r = −0.66, p = 0.01) of NEPSY II was found (Table 4).
Table 4. Significant zero-order correlations between ADHD-C's performances on WalCT and NEPSY II and on WalCT and WISC-IV.
To our knowledge, the current study is the first to assess the topographic memory in children with a diagnosis of ADHD-C using the WalCT (4, 51, 65, 66), testing the subject's ability to get oriented while really walking and moving through the environment. We found that the presence of an ADHD-C diagnosis negatively affected topographic memory performances in the WalCT. In regard to the first hypothesis of this work, we found an impairment in the topographic short-term memory in children with ADHD-C. This finding is in line with previous studies on working memory and visuospatial short-term memory in children with ADHD (e.g., (35, 102)). Kofler et al. (76) found that impaired working memory in children with ADHD determined consistent difficulties in anticipating, planning, enacting, and maintaining goal-directed actions, all fundamental abilities for a successful navigation. Zero-order correlations were conducted to measure the association between the ADHD-C group's performances in the WalCT and the performances in NEPSY II (90) and in WISC-IV (91, 92), confirmed this finding. Indeed, we found a positive correlation between the ADHD-C group's performances on TSTM and on digit span of WISC-IV (working memory index). During the digit span task, the child had to repeat a dictated series of digits forwards and another series backwards. The forward condition assessed span capacity, and the backward condition evaluated the ability to manipulate information in working memory (103). Rosenthal et al. (96) compared the performances on forward digit recall and on backward digit recall in children with ADHD, finding that the ADHD-predominantly inattentive group was able to recall significantly more digits backward than the ADHD-combined type group, showing a specific deficit in working memory. They also found an association between working memory and cortical thickness of the left medial temporal lobe in a sample of children with ADHD during the backward digit span subtest of WISC-IV (104). A recent study (105) found that human spatial navigation training is associated with changes in cortical thickness. Participants exhibited large improvements in navigation performance after 4 months of training, and these improvements were partly maintained 4 months after the end of training. In according to neural plasticity, younger adults showed higher performance than older adults. Considering this evidence, it would be interesting to assess if spatial navigational training in children with ADHD-C might increase cortical thickness, and in turn improve working memory ability. Taking into account that for pre-school children with TD a navigational training program fostered the acquisition of survey representation that is typically developed much later (106).
Furthermore, our finding showed that TSTM appears to be correlated with auditory attention (AA). It assessed the selective auditory attention and the ability to maintain concentrated attention over prolonged periods of time (vigilance or sustained attention). Children with ADHD-C could show a lower TSTM than TD because they could not sustain their concentration during the presentation of the sequences by the examiner. So, they were able to repeat only the first squares presented. To support of this hypothesis, Tucha et al. (107) assessed attention functioning in children with ADHD—predominantly hyperactive-impulsive type and children with ADHD—combined type, and showed that in comparison to healthy participants, they were impaired in measures of vigilance, divided attention, selective attention, and flexibility. Also, Pasini et al. (42), found that an ADHD-C and ADHD-I group showed an impairment in divided attention, response inhibition (prepotent response and interference control), and phonological and visual-object working memory.
Eventually, TSTM appears to be correlated with the memory for designs (MFD) task of NEPSY II that assessed spatial memory for novel visual material. In memory for designs, the child is shown a grid with four to ten designs on a page, which is then removed impeding the child from seeing the material to remember. The child then selects the designs from a set of cards and places the cards on a grid in the same location as previously shown (108).
A low score on MFD may suggest difficulty with route memorization for details, with location of visual stimuli details in the two-dimensional space and with learning for visuospatial information. Although there are few studies using tests to assess visuo-spatial working memory (VSWM) in children with ADHD-C, our findings are consistent with Westerberg et al. (35), who showed that in a specific sample of boys with ADHD-C and with no comorbidity (like our sample expect for the gender), VSWM is an important component of ADHD symptomatology. Also (37) investigated visuo-spatial and verbal WM performance in children with combined-type ADHD and their matched controls, finding that children with ADHD-C had deficits, relative to controls, on tasks designed to assess visuo-spatial WM. It is important to highlight that a delay in cortical maturation in children with ADHD has been reported by several authors [e.g., (62–64)] which showed a marked delay in children with ADHD in attaining peak thickness in particular in the prefrontal regions important for attention, planning, and navigational abilities: the median age of the attained peak thickness of cortical points for the ADHD group was 10.5 years, which was significantly later than the median age of 7.5 years for TD children.
Furthermore, children with ADHD-C performed significantly worse than controls in topographic learning (TL), they made more mistakes in executing the square-sequence path and needed a significantly higher number of repetitions to learn the path than children with typical development.
It is known that the maintenance of attention and cognitive flexibility play an important role in learning (47, 49, 50). Cognitive flexibility (CF) allows us to rapidly adapt our thoughts and behaviors in response to changing environmental demands and goals (109, 110), and CF is related specifically to working memory and inhibitory control (111). Cognitive flexibility plays a relevant role in learning and in complex problem solving. It allows us to select the strategy we need to adapt to different situations, capturing information from the environment, and responding in flexible ways by adjusting our behavior to the changes and demands of the situation. Perseverative errors committed by participants on executive function tasks such as the Wisconsin card sort test [WCST, (112)] are said to reflect cognitive inflexibility. An interesting finding of this work, that could have important consequences for clinical practice, is the fact that children with ADHD-C committed more perseverative errors during the topographic learning (TL) tasks than the TD children, showing more cognitive inflexibility. It is recognized that children with ADHD have difficulty in changing their responses even when the feedback suggests that their response is ineffective or maladaptive (75, 82, 113–115). Ahmadi et al. (82) investigated the differences between ADHD subtypes in terms of executive function profile, finding that children with the ADHD combined type showed more perseverative responses and perseverative errors than children with the ADHD predominantly inattentive type and TD children. In the study conducted by Lawrence et al. (113), children's performance on both neuropsychological and real-life measures of executive function and processing speed were compared, finding that problems in goal-directed behavior during real-life measures (number of deviations from designated route) were related to problems in set-shifting on the WCST (perseverative responding). Vallesi et al. (115) demonstrated that drug-naïve ADHD children were less able than TD children to switch from quick to accurate decision making, when required by the task demands, showing a deficit in the flexible regulation of strategic behavior. According to Fuster (116) and Barkley (33), perseverative errors in children with ADHD could be due to an interaction between behavioral inhibition and working memory; they fail to hold in mind information on the success of their response immediately preceding trials (retrospection), which then feeds forward to influence or even stop immediate future responses. On this topic, a growing body of research has focused on neural correlates of error detection reflected in the event-related potential (ERP). In particular, two ERP components seem to be involved in error processing: error-related negativity (ERN) reflecting a “monitoring system” to detect errors, and error positivity (Pe) reflecting a “remedial action system” to compensate for errors (117). Children with ADHD seem to have no compromises in early error monitoring processes related to error detection, whereas they show abnormal response strategy adjustments (117). In accordance with these findings, recent research demonstrated that it is very difficult to learn from our mistakes. Monfardini et al. (118) administered the same task in rhesus macaques and humans in which they could see that two items concealed a reward (a coin for humans, a candy for macaques). The authors found that choice-induced preference strongly affected individual learning. The monkeys and humans performed much more poorly after an initial negative choice than after an initial positive choice, indeed, poor learning from errors due to over-valuation of personal choices is among the decision-making biases shared by humans and animals.
Furthermore, Robaey et al. (119) based on their finding suggested a different strategy to help children with ADHD symptoms better orientate in space. They found a significant interaction between the presence of ADHD symptoms and learning strategy in virtual navigation tasks suggesting that children with ADHD symptoms primarily rely on caudate nucleus-dependent response learning strategies at the expense of hippocampus-dependent spatial strategies. They suggest that promoting a response strategy (counting, using anon-spatial systematic pattern of open and closed pathways, etc.) could help them to be better oriented when introducing them to a new spatial environment.
Unlike previous studies (120, 121), we did not find differences in the delayed recall between the two groups. From our results, it would seem that once the children have learned the path, they have no difficulty in keeping it in their memory and repeating it after 5 min. Although several long-term memory (LTM) tests used this interval of time or even shorter (e.g., Rey Figure Memory Recall) and in accordance with Miller's and Atkinson and Shiffrin's definition of short-term memory (STM), 5 min are a short time of delay and it is possible that some differences may emerge with longer time intervals. Future studies should also take into account different long-term intervals of time. Based on our findings, ADHD-C seemed to be associated with impaired initial topographic learning, probably due in particular to selective auditory attention and to the ability to maintain attention over prolonged periods of time (vigilance or sustained attention), to poor working memory and spatial memory for novel visual material, and perseverative behavior (cognitive inflexibility), whereas long-term retention of the learned topographical material was not compromised. This finding is consistent with previous studies that found that children with ADHD tend to display intact LTM if the material is encoded successfully (122, 123), suggesting that if children with ADHD have encoded information into long-term memory, they have no difficulties in retaining and retrieving that information (124).
We did not find significant differences between the two groups on the WalCT outline map task. Generally, all children of both groups showed difficulties in reproducing the learned path on the map. This finding is consistent with previous studies that showed how some aspects of human navigation are acquired later mainly because the neural bases underlying different processes have different maturational rates. Indeed, the neural structures that allow path integration and reorientation are already fully developed in toddlers, whereas more complex navigational skills require the activity of neural areas (such as the prefrontal cortex, PFC) which present a prolonged development, continuing into adolescence (51, 54).
Based on our findings it would seem that children with ADHD-C have a poor topographic span and a specific impairment in learning a new path, but when it is acquired, they have no difficulty in keeping it in their memory and repeating it after 5 min. In fact, children with ADHD-C presented significantly worse performances than controls in the topographic short-term memory (TSTM) and topographic learning (TL) tasks but not in topographic delayed recall (TDR). These impairments are probably due to the difficulty of maintaining their attention over prolonged periods of time (sustained attention), a spatial memory for novel visual material, poor working memory and visuo-spatial working memory, and perseverative behaviors. In regard to perseverative behaviors, children with ADHD-C tend to repeat the committed errors even if feedback suggests that their response is ineffective or maladaptive. Based on these results and on the conducted studies by Monfardini et al. (118), it would be interesting to design topographic training for children with ADHD-C based on the observation of errors of other subjects, in order to assess if they are able to overcome the bias linked to the choice-induced preference and commit fewer perseverative errors, learning the new path faster.
This study presents some limitations: the sample size does not allow for generalizations about findings and for such a reason we could not investigate the presence of individual differences (such as gender) on topographic memory in our sample, although previous studies found no gender differences in children aged 4–11 years on WalCT performance (4, 51). We followed strict criteria of inclusion in the sample recruitment: diagnosis of ADHD-C (DSM-5), age 8–10, IQ ≥85, an average score on Raven's colored progressive matrices (84, 85), no previous treatment, no other diagnosis of comorbidity, no primary visual or hearing impairments, and no other neurological or organic disease. These criteria led to the recruitment of a small sample of children with ADHD-C. In particular, it is very difficult to find a child with pure ADHD, because the presence of a comorbid disorder in neurodevelopmental disorders, and in particular, in ADHD children is more the rule than the exception (125). Also, the average age of diagnosis for ADHD is usually 6–8 years, so it is difficult to recruit children with pure ADHD-C who received their first diagnosis after the age of 8. As for the control group, we conducted a formal interview with parents and teachers to obtain information about the children but the influence of emotional variables (i.e., anxiety) was not considered in the neurocognitive performance.
Further studies should compare different subtypes of ADHD [predominantly inattentive ADHD (ADHD/I) vs. hyperactive-impulsive subtype (ADHD/HI) vs. ADHD-C] to evaluate if there are differences between these groups in topographic memory and to better understand the role of attention, hyperactivity, and impulsivity in navigational performances.
Eventually, several previous studies showed cortical maturation delay in ADHD children, particularly in prefrontal regions, relevant for the attention, planning, and navigational abilities that often disappear during adolescence [e.g., (61–64, 126–129)]. It would be interesting to assess further studies on topographic memory in older ADHD children compared with younger ADHD children in order to investigate whether part of the difficulties displayed by young ADHD-C children could be explained by a developmental delay that improves over time.
The original contributions presented in the study are included in the article/Supplementary Material, further inquiries can be directed to the corresponding author/s.
The studies involving human participants were reviewed and approved by Ethics Committee Of Policlinico Umberto I, Rome, Italy (Protocol number:416/16). Written informed consent to participate in this study was provided by the participants' legal guardian/next of kin. Written informed consent was obtained from the individual(s), and minor(s)' legal guardian/next of kin, for the publication of any potentially identifiable images or data included in this article.
MV recorded clinical data. NF conducted the research and wrote the manuscript with LP. SR and DA analyzed clinical data and conducted the statistical analysis. VG, GN MR, and CG coordinated and supervised the work and critically reviewed the manuscript. VB revised the English language editing. All authors have read and approved the final manuscript.
This project has received funding from the European Innovation Council, under the European Union's Horizon 2020 Research and Innovation Programme, Grant Agreement No 952095, project IM-TWIN—from Intrinsic Motivations to Transitional Wearable INtelligent companions for autism spectrum disorder.
The authors declare that the research was conducted in the absence of any commercial or financial relationships that could be construed as a potential conflict of interest.
The Supplementary Material for this article can be found online at: https://www.frontiersin.org/articles/10.3389/fpsyt.2021.647243/full#supplementary-material
1. Platas LM. Objects and Our Place Among Them. (2017). Available online at: http://prek-math-te.stanford.edu/spatial-relations/objects-and-our-place-among-them#1 (accessed October, 2020).
2. Palmiero M, Piccardi L. The role of emotional landmarks on topographical memory. Front Psychol. (2017) 10:763. doi: 10.3389/fpsyg.2017.00763
3. Bartonek Å, Piccardi L, Guariglia C. Topographical working memory in children with cerebral palsy. J Mot Behav. (2021) 53:200–8. doi: 10.1080/00222895.2020.1748861
4. Piccardi L, Palermo L, Leonzi M, Risetti M, Zompanti L, D'Amico S, et al. The Walking Corsi Test (WalCT): a normative study of topographical working memory in a sample of 4- to 11-year-olds. Clin Neuropsychol. (2014) 28:84–96. doi: 10.1080/13854046.2013.863976
5. Piccardi L, Palermo L, Bocchi A, Guariglia C, D'Amico S. Does spatial locative comprehension predict landmark-based navigation. PLoS ONE. (2015) 10:e0115432. doi: 10.1371/journal.pone.0115432
6. Boccia M, Nemmi F, Guariglia C. Neuropsychology of environmental navigation in humans: review and meta-analysis of FMRI studies in healthy participants. Neuropsychol Rev. (2014) 24:236–51. doi: 10.1007/s11065-014-9247-8
7. Guariglia C, Piccardi L. Les Arts De La Mémoire Et Les Images Mentales, Berthoz A. John Scheid Ariadne's Thread and the Unravelling of Navigational Skills Development. (2018). p. 209–20. Available online at: https://books.openedition.org/cdf/5577?format=embed (accessed October, 2020).
8. Hegarty M, Burte H, Boone AP. Individual differences in large-scale spatial abilities and strategies. In: Montello DR, editor. Handbook of Behavioral and Cognitive Geography . Northampton, MA: Edward Elgar Publishing (2018). p. 231–46. doi: 10.4337/9781784717544.00022
9. Wolbers T, Hegarty M. What determines our navigational abilities? Trends Cogn Sci. (2010) 14:138–46. doi: 10.1016/j.tics.2010.01.001
10. O'Keefe J, Nadel L. The hippocampus as a cognitive map. Behav Brain Sci. (1979) 2:487–94. doi: 10.1017/S0140525X00063949
11. O'Keefe J, Dostrovsky J. The hippocampus as a spatial map. preliminary evidence from unit activity in the freely-moving rat. Brain Res. (1971) 34:171–5. doi: 10.1016/0006-8993(71)90358-1
12. Acredolo LP. Development of spatial orientation in infancy. Dev Psychol. (1978) 14:224–34. doi: 10.1037/0012-1649.14.3.224
13. Newcombe NS. Navigation and the developing brain. J Exp Biol. (2019) 222(Pt Suppl. 1):jeb186460. doi: 10.1242/jeb.186460
14. Kravitz DJ, Saleem KS, Baker CI, Mishkin M. A new neural framework for visuospatial processing. Nat Rev. (2011) 12:217–30. doi: 10.1038/nrn3008
15. Mao D, Kandler S, McNaughton BL, Bonin V. Sparse orthogonal population representation of spatial context in the retrosplenial cortex. Nat Commun. (2017) 8:243. doi: 10.1038/s41467-017-00180-9
16. Grön G, Wunderlich AP, Spitzer M, Tomczak R, Riepe MW. Brain activation during human navigation: gender-different neural networks as substrate of performance. Nat Neurosci. (2000) 3:404–8. doi: 10.1038/73980
17. Hartley T, Maguire EA, Spiers HJ, Burgess N. The well-worn route and the path less traveled: distinct neural bases of route following and wayfinding in humans. Neuron. (2003) 37:877–88. doi: 10.1016/S0896-6273(03)00095-3
18. Maguire EA, Burgess N, Donnett JG, Frackowiak RS, Frith CD, O'Keefe J. Knowing where and getting there: a human navigation network. Science. (1998) 280:921–4.
19. Yoshida W, Ishii S. Resolution of uncertainty in prefrontal cortex. Neuron. (2006) 50:781–9. doi: 10.1016/j.neuron.2006.05.006
20. Carrieri M, Lancia S, Bocchi A, Ferrari M, Piccardi L, Quaresima V. Does ventrolateral prefrontal cortex help in searching for the lost key? Evidence from an fNIRS study. Brain Imaging Behav. (2018) 12:785–97. doi: 10.1007/s11682-017-9734-7
21. Berthoz A, Viaud-Delmon I. Multisensory integration in spatial orientation. Curr Opin Neurobiol. (1999) 9:708–12. doi: 10.1016/S0959-4388(99)00041-0
22. Ciaramelli E. The role of ventromedial prefrontal cortex in navigation: a case of impaired wayfinding and rehabilitation. Neuropsychologia. (2008) 46:2099–105. doi: 10.1016/j.neuropsychologia.2007.11.029
23. Guariglia C, Piccardi L. Environmental orientation and navigation in different types of unilateral neglect. Exp Brain Res. (2010) 206:163–9. doi: 10.1007/s00221-010-2310-7
24. Brown TI, Chrastil ER. Editorial: spatial navigation: memory, mechanisms, and executive function interactions. Front Hum Neurosci. (2019) 13:202. doi: 10.3389/fnhum.2019.00202
25. Iaria G, Bogod N, Fox CJ, Barton JJ. Developmental topographical disorientation: case one. Neuropsychologia. (2009) 47:30–40. doi: 10.1016/j.neuropsychologia.2008.08.021
26. Iaria G, Barton JJS. Developmental topographical disorientation: a newly discovered cognitive disorder. Exp Brain Res. (2010) 206:189–96. doi: 10.1007/s00221-010-2256-9
27. Iaria G, Burles F. Developmental topographical disorientation. Trends Cogn Sci. (2016) 20:720–2. doi: 10.1016/j.tics.2016.07.004
28. Bianchini F, Incoccia C, Palermo L, Piccardi L, Zompanti L, Sabatini U, et al. Developmental topographical disorientation in a healthy subject. Neuropsychologia. (2010) 48:1563–73. doi: 10.1016/j.neuropsychologia.2010.01.025
29. Bianchini F, Palermo L, Piccardi L, Incoccia C, Nemmi F, Sabatini U, et al. Where Am I? A new case of developmental topographical disorientation. J Neuropsychol. (2014) 8:107–24. doi: 10.1111/jnp.12007
30. Nemmi F, Bianchini F, Piras F, Péran P, Palermo L, Piccardi L, et al. Finding my own way: an fMRI single case study of a subject with developmental topographical disorientation. Neurocase. (2015) 21:573–83. doi: 10.1080/13554794.2014.960424
31. Piccardi L, De Luca M, Di Vita A, Palermo L, Tanzilli A, Dacquino C, et al. Evidence of taxonomy for developmental topographical disorientation: developmental landmark agnosia case 1. Appl Neuropsychol Child. (2019) 8:187–98. doi: 10.1080/21622965.2017.1401477
32. Schreiber JE, Possin KL, Girard JM, Rey-Casserly C. Executive function in children with attention deficit/hyperactivity disorder: the nih examiner battery. J Int Neuropsychol Soc. (2014) 20:41–51. doi: 10.1017/S1355617713001100
33. Barkley RA. Attention-Deficit Hyperactivity Disorder. A Handbook for Diagnosis and Treatment. 3rd Edn. New York, NY: Guilford Press (2006).
34. Willcutt EG, Doyle AE, Nigg JT, Faraone SV, Pennington BF. Validity of the executive function theory of attention-deficit/hyperactivity disorder: a meta-analytic review. Biol Psychiatry. (2005) 57:1336–46. doi: 10.1016/j.biopsych.2005.02.006
35. Westerberg H, Hirvikoski T, Forssberg H, Klingberg T. Visuo-spatial working memory span: a sensitive measure of cognitive deficits in children with ADHD. Child Neuropsychol. (2004) 10:155–61. doi: 10.1080/09297040409609806
36. NICE. Attention Deficit Hyperactivity Disorder. (2018). Available online at: https://www.nice.org.uk/guidance/ng87/evidence/full-guideline-pdf-4783651311 (accessed October, 2020).
37. Sowerby P, Seal S, Tripp G. Working memory deficits in ADHD: the contribution of age, learning/language difficulties, and task parameters. J Atten Disord. (2011) 15:461–72. doi: 10.1177/1087054710370674
38. Skogli EW, Egeland J, Andersen PN, Hovik KT, Øie M. Few differences in hot and cold executive functions in children and adolescents with combined and inattentive subtypes of ADHD. Child Neuropsychol. (2013) 20:162–81. doi: 10.1080/09297049.2012.753998
39. Dovis S, Van der Oord S, Wiers RW, Prins PJM. ADHD subtype differences in reinforcement sensitivity and visuospatial working memory. J Clin Child Adoles Psychol. (2015) 44:859–74. doi: 10.1080/15374416.2014.895940
40. Fernández-Andrés MI, Tejero P, Vélez-Calvo X. Visual attention orthographic word recognition and executive functioning in children with ADHD. Dyslexia, or ADHD + Dyslexia. J Atten Disord. (2021) 25:942–53. doi: 10.1177/1087054719864637
41. Rosenthal EN, Riccio CA, Gsanger KM, Jarratt KP. Digit Span components as predictors of attention problems and executive functioning in children. Arch Clin Neuropsychol. (2006) 21:131–9. doi: 10.1016/j.acn.2005.08.004
42. Pasini A, Paloscia C, Alessandrelli R, Porfirio MC, Curatolo P. Attention and executive functions profile in drug naive ADHD subtypes. Brain Dev. (2007) 29:400–8 doi: 10.1016/j.braindev.2006.11.010
43. Paloscia C, Aceti F, Baglioni V, Pasini A. Executive function deficits in Attention-Deficit/Hyperactivity, Disorder, and Asperger Syndrome. Riv Psichiatr. (2013) 48:441–7. doi: 10.1708/1379.15338
44. Willcutt EG. The prevalence of DSM-IV Attention-deficit/hyperactivity disorder: a meta-analytic review. Neurotherapeutics. (2012) 9:490–9. doi: 10.1007/s13311-012-0135-8
45. Kasper LJ, Alderson RM, Hudec KL. Moderators of working memory deficits in children with attention-deficit/hyperactivity disorder (ADHD): a meta-analytic review. Clin Psychol Rev. (2012) 32:605–17. doi: 10.1016/j.cpr.2012.07.001
46. Maccari L, Casagrande M, Martella D, Anolfo M, Rosa C, Fuentes LJ, et al. Change blindness in children with ADHD: a selective impairment in visual search? J Atten Disord. (2013) 17:620–7. doi: 10.1177/1087054711433294
47. Colomer C, Berenguer C, Roselló B, Baixauli I, Miranda A. The impact of inattention, hyperactivity/impulsivity symptoms, and executive functions on learning behaviors of children with ADHD. Front Psychol. (2017) 8:540. doi: 10.3389/fpsyg.2017.00540
48. American Psychiatric Association (APA). Diagnostic and Statistical Manual of Mental Disorders, 5th edition, Washington, DC: American Psychiatric Association (APA) (2013). doi: 10.1176/appi.books.9780890425596
49. Hoza B, Pelham WE, Waschbusch DA, Kipp H, Owens JSJ. Academic task persistence of normally achieving ADHD and control boys: performance, self-evaluations, and attributions. Consult Clin Psychol. (2001) 69:271–83. doi: 10.1037/0022-006X.69.2.271
50. Carlson CL, Booth JE, Shin M, Canu WH. Parent-, teacher-, and self-rated motivational styles in ADHD subtypes. J Learn Disabil. (2002) 35:104–13. doi: 10.1177/002221940203500202
51. Piccardi L, Leonzi M, D'Amico S, Marano A, Guariglia C. Development of navigational working memory: evidence from 6- to 10-year-old children. Br J Dev Psychol. (2014) 32:205–17. doi: 10.1111/bjdp.12036
52. Carriedo N, Corral A, Montoro PR, Herrero L, Rucián M. Development of the updating executive function: from 7-year-olds to young adults. Dev Psychol. (2016) 52:666–78. doi: 10.1037/dev0000091
53. Teffer K, Semendeferi K. Human prefrontal cortex. Prog Brain Res. (2012) 195:191–218. doi: 10.1016/B978-0-444-53860-4.00009-X
54. Casey BJ, Galvan A, Hare TA. Changes in cerebral functional organization during cognitive development. Curr Opin Neurobiol. (2005) 15:239–44. doi: 10.1016/j.conb.2005.03.012
55. Colombo D, Serino S, Tuena C, Pedroli E, Dakanalis A, Cipresso P, et al. Egocentric and allocentric spatial reference frames in aging: a systematic review. Neurosci Biobehav Rev. (2017) 80:605–21. doi: 10.1016/j.neubiorev.2017.07.012
56. Lehnung M, Leplow B, Friege L, Ferstl R, Mehdorn M. Development of spatial memory and spatial orientation in preschoolers and primary school children. Br J Psychol. (1998) 89:463–80. doi: 10.1111/j.2044-8295.1998.tb02697.x
57. Lehnung M, Leplow B, Ekroll V, Benz B, Ritz A, Mehdorn M, et al. Recovery of spatial memory and persistence of spatial orientation deficits after traumatic brain injury during childhood. Brain Injury. (2003) 17:855–69. doi: 10.1080/0269905031000089369
58. Overman WH, Pate BJ, Moore K, Peuster A. Ontogeny of place learning in children as measured in the radial arm maze, Morris search task, and open field task. Behav Neurosci. (1996) 110:1205–28. doi: 10.1037/0735-7044.110.6.1205
59. Laurance H, Learmonth A, Nadel L, Jacobs J. Maturation of spatial navigation strategies: convergent findings from computerized spatial environments and self-report. J Cogn Dev. (2003) 4:211–38. doi: 10.1207/S15327647JCD0402_04
60. Leplow B, Lehnung M, Pohl J, Herzog A, Ferstl R, Mehdorn M. Navigational place learning in children and young adults as assessed with a standardized locomotor search task. Br J Psychol. (2003) 94:299–317. doi: 10.1348/000712603767876244
61. Faraone SV, Biederman J, Mick E. Decline of attention deficit hyperactivity disorder: a meta-analysis of follow-up studies. Psychol Med. (2006) 36:159–65. doi: 10.1017/S003329170500471X
62. Shaw P, Eckstrand K, Sharp W, Blumenthal J, Lerch JP, Greenstein D, et al. Attention-deficit/hyperactivity disorder is characterized by a delay in cortical maturation. Proc Natl Acad Sci USA. (2007) 104:19649–54. doi: 10.1073/pnas.0707741104
63. Shaw P, Malek M, Watson B, Sharp W, Evans A, Greenstein D. Development of cortical surface area and gyrification in attention-deficit/hyperactivity disorder. Biol Psychiatry. (2012) 72:191–7. doi: 10.1016/j.biopsych.2012.01.031
64. Myatchin I, Lemiere J, Danckaerts M, Lagae L. Within-subject variability during spatial working memory in children with ADHD: an event-related potentials study. Eur Child Adolesc Psychiatry. (2012) 21:199–210. doi: 10.1007/s00787-012-0253-1
65. Piccardi L, Iaria G, Ricci M, Bianchini F, Zompanti L, Guariglia C. Walking in the Corsi test: which type of memory do you need? Neurosci Lett. (2008) 432:127–31. doi: 10.1016/j.neulet.2007.12.044
66. Piccardi L, Bianchini F, Argento O, De Nigris A, Maialetti A, Palermo L, et al. The Walking Corsi Test (WalCT): standardization of the topographical memory test in an Italian population. Neurol Sci. (2013) 34:971–8. doi: 10.1007/s10072-012-1175-x
67. Garden S, Cornoldi C, Logie RH. Visuo-spatial working memory in navigation. Appl Cogn Psychol. (2002) 16:35. doi: 10.1002/acp.746
68. Smith AD, Gilchrist ID, Hood BM. Children's search behavior in large-scale space: developmental components of exploration. Perception. (2005) 34:1221–9. doi: 10.1068/p5270
69. Coluccia E, Bosco A, Brandimonte MA. The role of visuo-spatial working memory in map learning: new findings from a map drawing paradigm. Psychol Res. (2007) 71:359–72. doi: 10.1007/s00426-006-0090-2
70. Nori R, Grandicelli S, Giusberti F. Individual differences in visuo-spatial working memory and real- world wayfinding. Swiss J Psychol. (2009) 68:7–16. doi: 10.1024/1421-0185.68.1.7
71. Baumann O, Skilleter AJ, Mattingley JB. Short-term memory maintenance of object locations during active: which working memory subsystem is essential? Psychol Res. (2007) 71:359–72. doi: 10.1371/journal.pone.0019707
72. Piccardi L, Nori R. Effects of visuo-spatial working memory on wayfinding ability. In: Levin ES, editor. Working Memory: Capacity, Developments and Improvement Techniques. New York, NY: Nova Science Publishers (2011). p. 81–108.
73. Nys M, Hickmann M, Gyselinck V. The role of verbal and visuo-spatial working memory in the encoding of virtual routes by children and adults. J Cogn Psychol. (2018) 30:710–27. doi: 10.1080/20445911.2018.1523175
74. Blacker KJ, Weisberg SM, Newcombe NS, Courtney SM. Keeping track of where we are: spatial working memory in navigation. Visual Cogn. (2017) 25:691–702. doi: 10.1080/13506285.2017.1322652
75. Barkley RA. Behavioral inhibition, sustained attention, and executive functions: constructing a unifying theory of ADHD. Psychol Bull. (1997) 121:65–94. doi: 10.1037/0033-2909.121.1.65
76. Kofler MJ, Sarver DE, Harmon SL, Moltisanti A, Aduen PA, Soto EF, et al. Working memory and organizational skills problems in ADHD. J Child Psychol Psychiatry. (2018) 59:57–67. doi: 10.1111/jcpp.12773
77. Skodzik T, Holling H, Pedersen A. Long-term memory performance in adult ADHD. J Atten Disord. (2017) 21:267–83. doi: 10.1177/1087054713510561
78. Skowronek JS. (2005) Long -Term Episodic Memory in Children With Attention -Deficit /Hyperactivity Disorder. doctoral dissertation. Available online at: https://scholars.unh.edu/cgi/viewcontent.cgi?article=1272&context=dissertation (accessed October, 2020).
79. Nazareth A, Weisberg SM, Margulis K, Newcombe NS. Charting the development of cognitive mapping. J Exp Child Psychol. (2018) 170:86–106. doi: 10.1016/j.jecp.2018.01.009
81. World Health Organization (WHO). The ICD-10 Classification of Mental and Behavioural Disorders. Geneva: World Health Organization (1993).
82. Ahmadi N, Mohammadi MR, Araghi SM, Zarafshan H. Neurocognitive profile of children with Attention Deficit Hyperactivity Disorders (ADHD): a comparison between subtypes. Iran J Psychiatry. (2014) 9:197–202.
83. Raven J, Raven JC, Court JH. Raven Manual, Section 2 (Coloured Progressive Matrices). Oxford: Oxford Psychologist Press (1998).
85. Belacchi C, Scalisi TG, Cannoni E, Cornoldi C. Manuale CPM. Coloured Progressive Matrices. Standardizzazione Italiana CPM Handbook. Colored Progressive Matrices. Italian Standardization. Florence: Giunti O. S (2008).
86. Bass N. The Raven's Coloured Progressive Matrices Test: A Pilot Study for the Establishment of Normative Data for Xhosaspeaking Primary School Pupils in the Grahamstown Region. Grahamstown: Rhodes University, (2000). p. 156.
87. Corsi PM. Human memory and the medial temporal region of the brai. Dissert Abstracts Int. (1972) 34:891B.
88. Wolbers T, Wiener JM. Challenges for identifying the neural mechanisms that support spatial navigation: the impact of spatial scale. Front Hum Neurosci. (2014) 4:571. doi: 10.3389/fnhum.2014.00571
89. Bocchi A, Giancola M, Piccardi L, Palmiero M, Nori R, D'Amico S. How would you describe a familiar route or put in order the landmarks along it? It depends on your cognitive style! Exp Brain Res. (2018) 2018:3121–9 . doi: 10.1007/s00221-018-5367-3
90. Korkman M, Kirk U, Kemp S. NEPSY-II. Second Edition. A Developmental Neuropsychology Assessment. it. a cura di. In: Urgesi C, Fabbro F, editors. Firenze: Giunti O.S. Organizzazioni Speciali (2011).
91. Wechsler D. Wechsler Intelligence Scale for Children-Forth Edition (WISC-IV). San Antonio, TX: Psychological Corporation (2003). doi: 10.1037/t15174-000
92. Orsini A, Pezzuti L, Picone L. WISC-IV and contributo alla taratura italiana. Firenze: Giunti Organizzazioni Speciali (2012).
93. Hedges LV, Olkin I. Statistical Methods for Meta-Analysis. San Diego, CA: Academic Press (1985).
94. Cohen J. Statistical Power Analysis for the Behavioral Sciences. New York, NY: Routledge Academic (1988).
95. Hedges L. Distribution theory for glass's estimator of effect size and related estimators. J Educ Stat. (1981) 6:107–28. doi: 10.3102/10769986006002107
96. Rosenthal JA. Qualitative descriptors of strength of association and effect size. J Soc Serv Res. (1996) 21:37–59. doi: 10.1300/J079v21n04_02
97. Cohen J. Things i have learned (so far). Am Psychol. (1990) 45:1304–12. doi: 10.1037/0003-066X.45.12.1304
99. Quinn GP, Keough MJ. Experimental Design and Data Analysis for Biologists. Cambridge: Cambridge University Press (2002). doi: 10.1017/CBO9780511806384
100. Nakagawa S, Cuthill IC. Effect size, confidence interval and statistical significance: a practical guide for biologists. Biol Rev. (2007) 82:591–605. doi: 10.1111/j.1469-185X.2007.00027.x
102. Yang BR, Chan RC, Gracia N, Cao XY, Zou XB, Jing J, et al. Cool and hot executive functions in medication-naive attention deficit hyperactivity disorder children. Psychol Med. (2011) 41:2593–602. doi: 10.1017/S0033291711000869
103. Holdnack JA. The development, expansion, and future of the WAIS-IV as a cornerstone in comprehensive cognitive assessments. In: Goldstein G, Allen ND, DeLuca J, editors. Handbook of Psychological Assessment IV (Fourth Edition). Amsterdam: Elsevier Ltd (2019). p. 103–39. doi: 10.1016/B978-0-12-802203-0.00004-3
104. Pastura G, Kubo TT, Regalla MA, Mesquita CM, Coutinho G, Gasparetto EL, et al. Working memory and left medial temporal cortical thickness. Arq Neuropsiquiatr. (2016) 74:785–90. doi: 10.1590/0004-282X20160123
105. Wenger E, Schaefer S, Noack H, Kühn S, Mårtensson J, Heinze J, et al. Cortical thickness changes following spatial navigation training in adulthood and aging. Neuroimage. (2012) 59:3389–97. doi: 10.1016/j.neuroimage.2011.11.015
106. Boccia M, Rosella M, Vecchione F, Tanzilli A, Palermo L, D'Amico S, et al. Enhancing allocentric spatial recall in pre-schoolers through navigational training programme. Front Neurosci. (2017) 11:574. doi: 10.3389/fnins.2017.00574
107. Tucha O, Walitza S, Mecklinger L, Sontag TA, Kübber S, Linder M, et al. Attentional functioning in children with ADHD - predominantly hyperactive-impulsive type and children with ADHD - combined type. J Neural Transm. (2006) 113:1943–53. doi: 10.1007/s00702-006-0496-4
108. Korkman. NEPSY-II (2007) Chapter 2. (2007). Available online at: https://images.pearsonclinical.com/images/Products/NEPSY-II/Clin_Chp_2.pdf (accessed October, 2020).
109. Carroll DJ, Blakey E, FitzGibbon L. Cognitive flexibility in young children: beyond perseveration. Child Dev Perspect. (2016) 10:211–5. doi: 10.1111/cdep.12192
110. Chevalier N, Sheffield TD, Nelson JM, Clark CA, Wiebe SA, Espy KA. Underpinnings of the costs of flexibility in preschool children: the roles of inhibition and working memory. Dev Neuropsychol. (2012) 37:99–118. doi: 10.1080/87565641.2011.632458
111. Garon N, Bryson SE, Smith IM. Executive function in preschoolers: a review using an integrative framework. Psychol Bull. (2008) 134:31–60. doi: 10.1037/0033-2909.134.1.31
112. Grant DA, Berg EA. A behavioral analysis of degree of reinforcement and ease of shifting to new responses in a Weigl-type card-sorting problem. J Exp Psychol. (1948) 38:404–11. doi: 10.1037/h0059831
113. Lawrence V, Houghton S, Douglas G, Durkin K, Whiting K, Tannock R. Executive function and ADHD: a comparison of children's performance during neuropsychological testing and real-world activities. J Atten Disord. (2004) 7:137–49. doi: 10.1177/108705470400700302
114. Bahçivan Saydam R, Ayvaşik HB, Alyanak B. Executive functioning in subtypes of attention deficit hyperactivity disorder. Noro Psikiyatr Ars. (2015) 52:386–92. doi: 10.5152/npa.2015.8712
115. Vallesi A, D'Agati E, Pasini A, Pitzianti M, Curatolo P. Impairment in flexible regulation of speed and accuracy in children with ADHDJ. Int Neuropsychol Soc. (2013) 19:1016–20. doi: 10.1017/S1355617713000969
117. Wiersema JR, van der Meere JJ, Roeyers H. ERP correlates of impaired error monitoring in children with ADHD. J Neural Transm. (2005) 112:1417–30. doi: 10.1007/s00702-005-0276-6
118. Monfardini E, Gaveau V, Boussaoud D, Hadj-Bouziane F, Meunier M. Social learning as a way to overcome choice-induced preferences? Insights from humans and rhesus macaques. Front Neurosci. (2012) 6:127. doi: 10.3389/fnins.2012.00127
119. Robaey P, McKenzie S, Schachar R, Boivin M, Bohbot VD. Stop and look! Evidence for a bias towards virtual navigation response strategies in children with ADHD symptoms. Behav Brain Res. (2016) 298:48–54. doi: 10.1016/j.bbr.2015.08.019
120. Rhodes SM, Park J, Seth S, Coghill DR. A comprehensive investigation of memory impairment in attention deficit hyperactivity disorder and oppositional defiant disorder. J Child Psychol Psychiatry. (2012) 53:128–37. doi: 10.1111/j.1469-7610.2011.02436.x
121. Yáñez-Téllez G, Romero-Romero H, Rivera-García L, Prieto-Corona B, Bernal-Hernández J, Marosi Holczberger E, et al. Cognitive and executive functions in ADHD. Actas Esp Psiquiatr. (2012) 40:293–8.
122. Kibby MY, Cohen MJ. Memory functioning in children with reading disabilities and/or attention deficit/hyperactivity disorder: a clinical investigation of their working memory and long-term memory functioning. Child Neuropsychol. (2008) 14:525–46. doi: 10.1080/09297040701821752
123. Muir-Broaddus JE, Rosenstein LD, Medina DE, Soderberg C. Neuropsychological test performance of children with ADHD relative to test norms and parent behavioral ratings. Arch Clin Neuropsychol. (2002) 17:671–89. doi: 10.1093/arclin/17.7.671
124. Kaplan BJ, Dewey D, Crawford SG, Fisher GC. Deficits in long-term memory are not characteristic of ADHD. attention deficit hyperactivity disorder. J Clin Exp Neuropsychol. (1998) 20:518–28. doi: 10.1076/jcen.20.4.518.1477
125. Reale L, Bonati M. ADHD prevalence estimates in Italian children and adolescents: a methodological issue. Ital J Pediatr. (2018) 44:108. doi: 10.1186/s13052-018-0545-2
126. Kalff AC, de Sonneville LMJ, Hurks PPM, Hendriksen JG, Kroes M, Feron FJ, et al. Low- and high-level controlled processing in executive motor control tasks in 5- to 6-year-old children at risk of ADHD. J Child Psychol Psychiatry. (2003) 44:1049–57. doi: 10.1111/1469-7610.00189
127. Krain AL, Castellanos FX. Brain development and ADHD. Clin Psychol Rev. (2006) 26:433–44. doi: 10.1016/j.cpr.2006.01.005
128. Smith AB, Taylor E, Brammer M, Toone B, Rubia K. Task-specific hypoactivation in prefrontal and temporoparietal brain regions during motor inhibition and task switching in medication-naive children and adolescents with attention deficit hyperactivity disorder. Am J Psychiatry. (2006) 163:1044–51. doi: 10.1176/ajp.2006.163.6.1044
Keywords: navigational memory, executive function, attention deficit hyperactivity disorder, navigational skills, topographic memory
Citation: Faedda N, Guariglia C, Piccardi L, Natalucci G, Rossetti S, Baglioni V, Alunni Fegatelli D, Romani M, Vigliante M and Guidetti V (2021) Link Between Topographic Memory and the Combined Presentation of ADHD (ADHD-C): A Pilot Study. Front. Psychiatry 12:647243. doi: 10.3389/fpsyt.2021.647243
Received: 29 December 2020; Accepted: 26 April 2021;
Published: 17 June 2021.
Edited by:
Veit Roessner, University Hospital Carl Gustav Carus, GermanyReviewed by:
Joanne Cotton, University of Cambridge, United KingdomCopyright © 2021 Faedda, Guariglia, Piccardi, Natalucci, Rossetti, Baglioni, Alunni Fegatelli, Romani, Vigliante and Guidetti. This is an open-access article distributed under the terms of the Creative Commons Attribution License (CC BY). The use, distribution or reproduction in other forums is permitted, provided the original author(s) and the copyright owner(s) are credited and that the original publication in this journal is cited, in accordance with accepted academic practice. No use, distribution or reproduction is permitted which does not comply with these terms.
*Correspondence: Vincenzo Guidetti, dmluY2Vuem8uZ3VpZGV0dGlAdW5pcm9tYTEuaXQ=
Disclaimer: All claims expressed in this article are solely those of the authors and do not necessarily represent those of their affiliated organizations, or those of the publisher, the editors and the reviewers. Any product that may be evaluated in this article or claim that may be made by its manufacturer is not guaranteed or endorsed by the publisher.
Research integrity at Frontiers
Learn more about the work of our research integrity team to safeguard the quality of each article we publish.