- Department of Pediatrics, Fujian Medical University Union Hospital, Fuzhou, China
Background: Previous studies have identified differentially expressed microRNAs in autism spectrum disorder (ASD), however, results are discrepant. We aimed to systematically review this topic and perform bioinformatic analysis to identify genes and pathways associated with ASD miRNAs.
Methods: Following the Preferred Reporting Items for Systematic reviews and Meta-Analyses, we searched the Web of Science, PubMed, Embase, Scopus, and OVID databases to identify all studies comparing microRNA expressions between ASD persons and non-ASD controls on May 11, 2020. We obtained ASD miRNA targets validated by experimental assays from miRTarBase and performed pathway enrichment analysis using Metascape and DIANA-miRPath v3. 0.
Results: Thirty-four studies were included in the systematic review. Among 285 altered miRNAs reported in these studies, 15 were consistently upregulated, 14 were consistently downregulated, and 39 were inconsistently dysregulated. The most frequently altered miRNAs including miR-23a-3p, miR-106b-5p, miR-146a-5p, miR-7-5p, miR-27a-3p, miR-181b-5p, miR-486-3p, and miR-451a. Subgroup analysis of tissues showed that miR-146a-5p, miR-155-5p, miR-1277-3p, miR-21-3p, miR-106b-5p, and miR-451a were consistently upregulated in brain tissues, while miR-4742-3p was consistently downregulated; miR-23b-3p, miR-483-5p, and miR-23a-3p were consistently upregulated in blood samples, while miR-15a-5p, miR-193a-5p, miR-20a-5p, miR-574-3p, miR-92a-3p, miR-3135a, and miR-103a-3p were consistently downregulated; miR-7-5p was consistently upregulated in saliva, miR-23a-3p and miR-32-5p were consistently downregulated. The altered ASD miRNAs identified in at least two independent studies were validated to target many autism risk genes. TNRC6B, PTEN, AGO1, SKI, and SMAD4 were the most frequent targets, and miR-92a-3p had the most target autism risk genes. Pathway enrichment analysis showed that ASD miRNAs are significantly involved in pathways associated with cancer, metabolism (notably Steroid biosynthesis, Fatty acid metabolism, Fatty acid biosynthesis, Lysine degradation, Biotin metabolism), cell cycle, cell signaling (especially Hippo, FoxO, TGF-beta, p53, Thyroid hormone, and Estrogen signaling pathway), adherens junction, extracellular matrix-receptor interaction, and Prion diseases.
Conclusions: Altered miRNAs in ASD target autism risk genes and are involved in various ASD-related pathways, some of which are understudied and require further investigation.
Introduction
Autism spectrum disorder (ASD) is a category of clinically and genetically heterogeneous neurodevelopmental disorder characterized by impaired social function and repetitive, restricted behaviors (1). Genetics plays a significant role in the cause of ASD. Hundreds of different genetic loci, including non-coding mutations (2), single-nucleotide variants, chromosome abnormalities, and copy number variations have been associated with ASD (3, 4). However, many different variants share common biological pathways (5). Thus, identifying converging biological pathways and molecular mechanisms responsible for this disorder has much translational and clinical value (5).
MicroRNAs (miRNAs) are a class of small, non-coding RNAs with the main functions of regulating mRNA destabilization and modifying protein levels (6). One miRNA can target up to hundreds of different mRNAs, and a single mRNA may be regulated by many miRNAs. Thus, miRNAs–mRNAs form complicated gene regulatory networks and participate in various biological functions (7), including brain development and function (8). By regulating local gene expression, miRNAs can control cell fate determination, neurogenesis, cell migration, neuronal polarization, synapse development, and synaptic plasticity (9). In neurodevelopmental disorders, miRNAs are often dysregulated, indicating that miRNAs play an important part in the etiology and/or maintenance of neurological disorders (9).
Since the first study performed by Abu-Elneel and his colleagues in 2008 (10), miRNA expression profiling in ASD has been performed in brain tissue samples (10–18), serum (19–23), blood (24–30), peripheral blood monocytes (31–33), lymphoblast cell lines (34–37), saliva (38–40), reprogrammed induced pluripotent stem cell-derived neurons (41), olfactory mucosal stem cells, and skin fibroblasts (42) in studies comparing ASD persons and controls. There were a significant number of miRNAs in ASD samples and control with different expressions; however, many discrepancies among studies exist. The discrepancies may be due to differences in tissue types, genetic and environmental origin of the tissue sources, RNA extraction methods, miRNA detection, and validation methods (such as microarray, miRNA-seq, and RT-qPCR), data normalization methods, identification of new miRNAs, and miRNAs' annotation changes in the miRbase database, which stores information about individual microRNAs since 2002 (43). The biological function of these microRNAs in ASD pathogenesis remains little known, and there remains a question as to which miRNAs may be significant specific signatures as personalized biomarkers or therapeutic targets.
Until now, the literature reviews of ASD miRNAs were primarily narrative, and conclusive results were not available for comprehensive functional analysis (44). Therefore, an updated systematic review is needed. The objective of this research was to systematically review the literature to assess which microRNAs were altered in children and adults with ASD when compared to non-ASD controls from case-control studies and standardize them to miRBase version 22.1 (43). Furthermore, validated gene targets of these miRNAs were obtained and pathway enrichment analysis was used to assess the physiological impact of miRNA dysregulation in ASD pathology. Our study may clarify the ambiguities and contradictions in this research field and promote future further studies to better understand microRNAs' function in ASD. This may also contribute to the use of microRNAs as potential personalized biomarkers and therapeutic targets.
Materials and Methods
Criteria for Considering Studies for This Review
Types of Participants
We included children and adults with ASD diagnosed by an established classification system or clinical assessment, including individuals with autistic disorder, Asperger's disorder, and pervasive developmental disorder–not otherwise specified (PDD-NOS). Participants with comorbidities were not excluded.
Types of Exposures
The exposure was ASD diagnosis.
Types of Control
The control participants were non-ASD individuals.
Types of Outcome Measures
The outcome measure was microRNA expression level.
Types of Studies
Original research case-control studies written in English were eligible for this review. Literature reviews, non-human studies, comments, opinion articles, expert opinions, letters, news reports, hypotheses, conference summaries, book sections, patent descriptions, same study reports, non-ASD or non-microRNA expression studies, and studies that are not case-control were excluded.
Database Search Strategies for Identification of Studies
We conducted a systematic literature search in Web of Science, PubMed, Embase, Scopus, and OVID databases without initial date restriction up to and including May 11, 2020. The search criteria in five databases are provided in Supplementary Material 1. No language restriction was applied. The search yielded 2,718 references.
Selection of Studies
All references were managed in the EndNote X9 software (Thomson Reuters, New York, NY, USA). Initially, duplicate references were removed. Two reviewers (ZXH and GFC) independently screened titles and abstracts based on the inclusion and exclusion criteria. Lists were compared, and a consensus was reached through discussion or with a third reviewer in case of disagreement. This systematic review followed the Preferred Reporting Items for Systematic reviews and Meta-Analyses (PRISMA) statements (Figure 1; Supplementary Material 2), with some modifications (45).
Data Collection
Two reviewers (ZXH and HRG) independently collected the following data items from the full text and supplementary data of each included study: first author, year of publication, country of study, tissue types, diagnostic measure, sample sizes, the age of cases and controls, the gender of cases and controls, miRNA expression assay type, lists of upregulated and downregulated miRNAs with statistically significant expression changes, and differential expression criteria. Lists were compared, and a consensus was reached through discussion or with a third reviewer (GFC) in case of disagreement. All miRNA names from different studies were standardized to miRBase version 22.1 (43). Pre-miRNAs, viral miRNAs, and non-miRNAs were not used in the bioinformatic analysis.
Enrichment for ASD Risk Genes Among Validated Targets for Each Overlapping miRNAs in ASD
To explore the experimentally validated target genes of each microRNAs that were dysregulated in at least two independent studies (ASD-miRNAs), we obtained ASD-miRNAs targets that had been experimentally verified using reporter assays, western blots, microarrays, or next-generation sequencing studies, among other methods, from the miRTarBase database (Supplementary Material 3) (46). We did not perform further filtering based on strength of evidence. Next, we systematically evaluated and compared whether targets of the differentially expressed miRNAs are enriched for ASD risk genes (Supplementary Material 4) from the Simons Foundation Autism Research Initiative (SFARI, https://gene.sfari.org/database/human-gene/) (47) and AutDB database (http://autism.mindspec.org/autdb/HG_Home.do) (48), which are based on candidate gene studies, common variant association, genetic syndromes, and copy number variation.
Subgroup Analysis of Tissues and Pathway Enrichment Analysis of Validated Targets for Each ASD-miRNAs
To further explore the associated biological pathways of ASD-miRNAs in specific tissues, firstly the web-based software portal Metascape was used to conduct Kyoto Encyclopedia of Genes and Genomes (KEGG) and Reactome pathways analysis of validated targets from miRTarbase for each of ASD-miRNAs (49), with a background set of genes that are expressed in the brain, salivary glands, blood, and immune cells (Supplementary Material 5, data available from v20.1.proteinatlas.org) (50) for miRNA detected in these tissues. There are 16,227 genes in the brain (data available from https://www.proteinatlas.org/humanproteome/brain/human+brain), 15,218 in the salivary glands (data available from https://www.proteinatlas.org/humanproteome/tissue/salivary+gland), and 14,812 in “blood and immune cells” (data available from https://www.proteinatlas.org/humanproteome/celltype/blood+26+immune+cells). Studies using brain tissue, reprogrammed induced pluripotent stem cell-derived neurons, or olfactory mucosal stem cells were classified as “brain” tissue. Studies using serum, blood, lymphoblast cell lines, or peripheral blood mononuclear cells were classified as “blood or immune cell” tissue. Studies using saliva were classified as “salivary gland.” Terms with p-value < 0.01, a minimum count of 3, and enrichment factor of >1.5 were considered as significant (using hypergeometric test and Benjamini–Hochberg p-value correction).
Explore KEGG Pathways That Are Commonly Targeted by Multiple miRNAs
Moreover, to investigate KEGG pathways that are simultaneously controlled by multiple ASD-miRNAs, dysregulated ASD-miRNAs in the brain, or consistently upregulated and downregulated ASD-miRNAs in blood or saliva, were inputted into the online software DIANA-miRPath v3.0 (51), respectively. The KEGG analysis was used, and the human species was selected. In the optional gene filter menu, genes that are expressed in the brain, salivary glands, blood, and immune cells (data available from v20.1.proteinatlas.org, http://www.proteinatlas.org) (50) were uploaded respectively. The interactions dataset selected for all microRNAs was TarBase v7.0, based on previous positive and negative experiments. In the advanced statistics options, the Fisher's Exact Test (Hypergeometric Distribution), Benjamini–Hochberg's False Discovery Rate (FDR) correction, and more conservative statistics (DAVID's EASE score) with a p-value threshold of 0.05 were selected. After inputting miRNAs into the software, “Pathways union” was selected to identify pathways containing more than one associated miRNA, which can give meta-analysis statistics for the assessment of combined miRNA action.
Quality Assessment of Selected Studies
We assessed the methodological quality of the included studies using the Newcastle-Ottawa Scale (NOS) for case-control studies (52). The scale includes three categories: selection, comparability, and exposure. A higher total quality score indicates better study quality, and the highest quality score is 10 (Supplementary Material 6).
Results
Selection and Characteristics of Included Independent Studies
Using Web of Science, PubMed, Embase, Scopus, and OVID databases, a total of 2,718 studies were identified; another three articles were added from the review citation. Among them, 1,385 were duplicates; a total of 1,336 articles were included in the title and abstract screening after which 48 articles remained. After evaluating the full text, 14 studies were excluded. Figure 1 displays the PRISMA flowchart for the study selection process.
Details of the characteristics of the selected studies are shown in Supplementary Material 6. The year of publication was from 2008 to 2020. In total, this review pooled results from over 1,000 subjects with ASD and almost 1,000 controls. Five studies included only male participants (10, 18, 29, 35, 41). One study included only female participants (53). In all, there are more males than females with ASD (773:192). Participants' ages ranged from 2 to 81 years. In all 34 included studies, the participants had ASD diagnoses. The Autism Diagnostic Interview-Revised (ADI-R) was the most used diagnostic instrument among the studies, followed by the Diagnostic and Statistical Manual of Mental Disorders, 5th Edition (DSM-5), Autism Diagnostic Interview-Revised (ADOS), Diagnostic and Statistical Manual of Mental Disorders, 4th Edition, Text Revision (DSM-IV-TR), and Diagnostic and Statistical Manual of Mental Disorders, 4th Edition (DSM-IV). Six studies did not provide the ASD diagnostic criteria but did report that the participants had ASD, including autistic disorder, Asperger's disorder, and pervasive developmental disorder–not otherwise specified (PDD-NOS) (10, 14, 18, 33, 35, 53); thus, they are included in this study. Ten of these studies involved brain tissue (10–18, 53), 15 involved peripheral blood (19–33), four employed lymphoblastoid cell lines (34–37), three examined saliva (38–40), 1 examined reprogrammed induced pluripotent stem cell-derived neurons (41), and 1 examined olfactory mucosal stem cells and skin fibroblasts (42). Variation in these studies is due to differences in the patients investigated, the tissue examined, microRNA profiling and analysis methods, the number of samples, and the statistical analysis approach used.
Differentially Expressed miRNAs
In the 34 selected miRNA expression profiling studies, 285 differentially expressed mature miRNAs were reported that compared over 1,000 subjects with ASD and almost 1,000 controls (Supplementary Material 6). Of the 68 differentially expressed miRNAs identified in at least two studies (ASD-miRNAs), 29 miRNAs had a consistent direction, 15 upregulated and 14 downregulated (Table 1), and 39 inconsistently dysregulated (Table 2). Among them, miR-23a-3p, miR-106b-5p, miR-146a-5p, miR-7-5p, miR-27a-3p, miR-451a, miR-181b-5p, and miR-486-3p are the most frequently reported (Tables 1, 2). Certain microRNAs changed in different ways depending on gender; for example, miR-148b-3p was shown to be downregulated solely in male ASD participants (Tables 1, 2; Supplementary Material 6).
Subgroup analysis of tissues showed that in brain samples, six miRNAs (miR-146a-5p, miR-155-5p, miR-1277-3p, miR-21-3p, miR-106b-5p, and miR-451a) were consistently upregulated, 1 miRNA (miR-4742-3p) was consistently downregulated, and seven miRNAs were inconsistently dysregulated (Supplementary Material 6). In blood and immune cell samples, four miRNAs (miR-146a-5p, miR-23b-3p, miR-483-5p, and miR-23a-3p) were consistently upregulated, seven miRNAs (miR-15a-5p, miR-193a-5p, miR-20a-5p, miR-574-3p, miR-92a-3p, miR-3135a, and miR-103a-3p) were consistently downregulated, and 19 miRNAs were inconsistently dysregulated (Supplementary Material 6). In saliva samples, one miRNA (miR-7-5p) was consistently upregulated, two miRNAs (miR-23a-3p and miR-32-5p) were consistently downregulated, and two miRNAs were inconsistently dysregulated (Supplementary Material 6).
Validated Gene Targets of Each Dysregulated microRNAs Overlap With ASD Risk Genes
Compared to experimentally validated microRNA-targets based on miRTarBase, each ASD-miRNA targets many genes. miR-92a-3p, miR-15b-5p, miR-93-5p, and miR-155-5p are among microRNAs that have the greatest number of validated ASD risk gene targets (Table 1, Supplementary Materials 7–9). The most frequently targeted ASD candidate genes were TNRC6B, PTEN, AGO1, AGO2, SKI, and SMAD4 (Supplementary Materials 7–9).
Subgroup Analysis of Tissues and ASD-miRNAs Associated KEGG and Reactome Pathways
Using the Metascape, a diverse range of KEGG and Reactome pathways were significantly enriched for validated gene targets of each dysregulated microRNAs (Supplementary Materials 7–9). Furthermore, using the software DIANA-mirPath v.3, bioinformatics analysis revealed that among the 7, 11, and 3 consistently dysregulated ASD-miRNAs in the brain, blood, and saliva respectively, there were various commonly targeted pathways (Figures 2A–C). Enriched KEGG pathways were most significantly associated with cancer, metabolism (notably steroid biosynthesis, fatty acid metabolism, fatty acid biosynthesis, lysine degradation, biotin metabolism), cell cycle, cell signaling (especially Hippo, FoxO, TGF (transforming growth factor)-beta, p53, thyroid hormone, and estrogen signaling pathway), adherens junction, extracellular matrix–receptor interaction, prion diseases, etc., (Figures 2A–C).
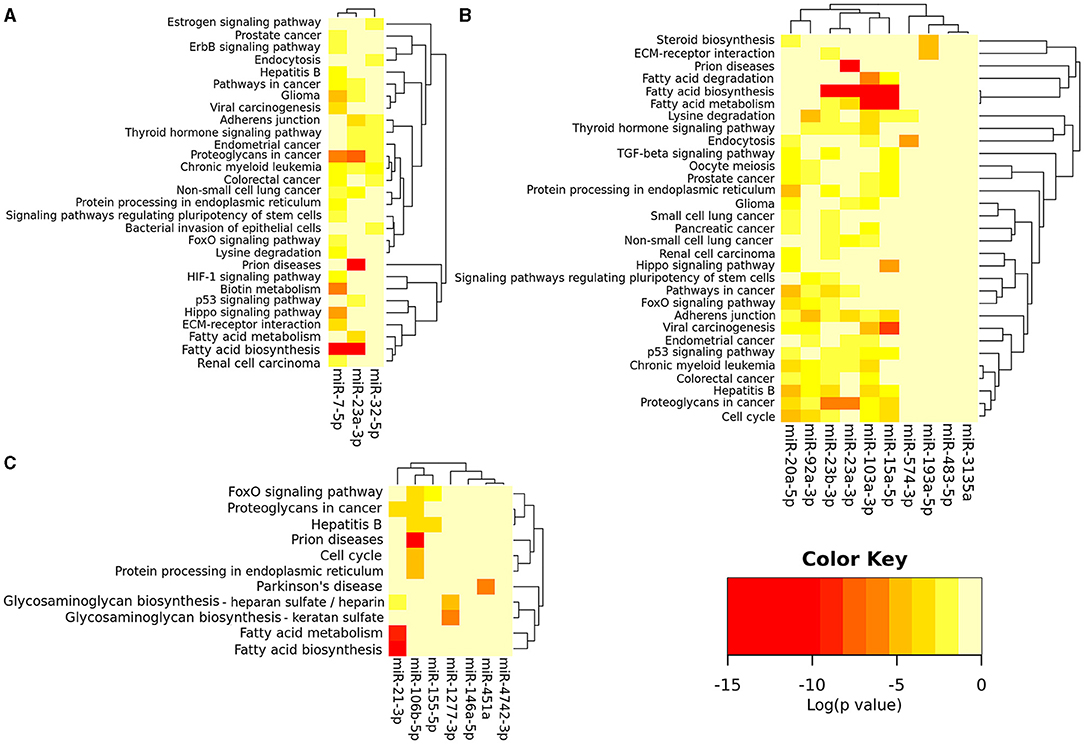
Figure 2. miRNAs vs. pathways heatmap (Clustering based on significance levels) of the consistently dysregulated ASD-miRNAs in blood and immune cells (A), saliva (B), and brain tissue (C). The heatmap was created from the DIANA mirPath v 3.0. Darker shades represent more significant. Dendrograms depicts hierarchical clustering results for miRNAs and pathways, respectively. The miRNA axis represents the clustered miRNAs with similar pathway targeting patterns. A similar clustering is shown on the pathway axis. Interactions dataset: TarBase v7.0; Analysis: pathways union; p-value threshold: 0.05; FDR Correction, Conservative Statistics.
Discussion
In this report, we systematically reviewed the ASD-related miRNAs from 34 independent profiling studies. Up until now, more than 285 mature microRNAs have been identified (Supplementary Material 6), of which 68 had altered levels in at least two independent studies comparing autistic persons with controls (Tables 1, 2). The most frequently altered miRNAs in all studies include miR-23a-3p, miR-106b-5p, miR-146a-5p, miR-7-5p, and miR-27a-3p. MiRNAs that were dysregulated in at least two studies were validated to target a number of ASD risk genes. Among them, TNRC6B, PTEN, AGO1, AGO2, SKI, and SMAD4 were the most frequent (Supplementary Materials 7–9), and miR-92a-3p has the most target ASD risk genes (Table 1, Supplementary Material 8). Bioinformatic analysis showed that validated targets of each microRNA were enriched in various KEGG and Reactome pathways. Moreover, tissue subgroup enrichment analysis by using mirPath v.3 showed that various commonly targeted pathways were identified for the 7, 11, and 3 consistently dysregulated ASD-miRNAs in brain, blood, and saliva, respectively. Our study clarified miRNAs involved in ASD and explored their target genes and significantly associated pathways, which are the focus of our discussion next.
miR-23a-3p and miR-27a-3p belong to the same cluster and participate in several ASD-related pathways, such as lysine degradation and fatty acid metabolism (Supplementary Materials 7–9; Figures 2A,B). Our results showed that miR-23a-3p targets many ASD risk genes, including PTEN, TSC1, and KDM3B (Supplementary Material 7). miR-23a plays a crucial role in neurogenesis (9). For example, miR-23a-3p controls oligodendrocyte differentiation and myelin formation by targeting PTEN and manipulating PTEN/PI3K/Akt/mTOR pathway (55). It also directly targets TSC1, which is crucial in the mTOR signaling pathway associated with synaptic protein defects in autistic persons (54). miR-27a-3p targets many ASD risk genes, including IGF1, KMT2A, KMT2C, KMT5B, and IL6 (Supplementary Material 8). For example, miR-27a-3p targets insulin-like growth factor-1 (IGF-1), which binds to the IGF-1 receptor and activates PI3K and MAPK pathways and various downstream signaling pathways, including mTOR, p53, and FoxO signaling pathway. Insulin-like growth factor-1 plays an important role in brain development (56); it has shown a potential therapeutic role for ASD in vitro (57) and in individuals with ASD (58) and Rett syndrome (59). KMT2A, KMT2C, KMT5B, and NSD1 all participate in post-translational chromatin modification such as histone lysine methylation and are thus linked to transcriptional activation or repression (60). These genes are also involved in lysine degradation and play an important role in both normal human development and developmental disorders, including ASD (61). A recent study showed that miR-27a-3p has protective effects on the blood–brain barrier, brain injury, and hippocampal neuron injury (62). Therefore, its dysregulation may result in neurologic dysfunction. miR-23a-3p and miR-27a-3p are dysregulated not only in brain samples (10) but also in the saliva of autistic persons (38). Thus, they may be potential biomarkers of ASD.
hsa-miR-106b-5p and hsa-miR-93-5p belong to the microRNA-25-93-106b cluster; they are vital for “neural stem/progenitor cell proliferation and neuronal differentiation” and are involved in various psychiatric or neurologic disorders (63, 64). They both target many ASD risk genes including MECP2, PTEN, and SMAD4, and they are involved in various pathways, including adherens junction, circadian clock, long-term depression, mTOR, and estrogen signaling pathway (Supplementary Materials 7, 8). Methyl CpG binding protein 2 (MECP2) is abundantly expressed in neurons and is essential for neuronal function and development (65). MECP2 under-expression and overexpression have been associated with Rett syndrome (3, 66) and MECP2 duplication syndrome, respectively, and many neurologic disorders including ASD (3, 67). Besides, many cases of Rett syndrome and MECP2 duplication syndrome fit the diagnostic criteria for ASD (3, 68). As a multifunctional protein, MECP2 affects various metabolites (including glutamate and catecholamine catabolites), genes (including BDNF), and pathways (including AKT/mTOR and neurotrophin signaling pathway). Therefore, more research is needed to investigate the target genes and pathways of hsa-miR-106b-5p and hsa-miR-93-5p to better understand neurologic disorders including ASD and Rett syndrome.
Notably, miR-146a-5p (11, 17) and miR-155-5p (11, 13, 18) were both consistently upregulated in brain tissues of ASD persons (Supplementary Material 6), and they play a critical role in regulating immune response (69) and neuroinflammation (70). In line with this, our results showed that they target immune/inflammation-associated genes (including IL6 and SMAD4) and pathways (such as NF-kappa B, TGF-beta, and Toll-like receptor signaling pathway) (Figure 2C; Supplementary Material 7). miR-146a-5p has also been consistently upregulated in lymphoblastoid cell line (34), skin fibroblasts (42), and olfactory mucosal stem cell (42) samples from people diagnosed with ASD. This may be an anti-inflammatory compensatory reaction since miR-146a-5p primarily acts as a negative inflammation regulator (70). However, evidence showed that miR-146a-5p dysregulation can affect neuronal development and differentiation and may thus lead to ASD (17, 42, 71).
miR-7-5p is abundant in neurons and neuroendocrine organs and is a prototypical neuroendocrine miRNA. It regulates many genes in the brain (Supplementary Material 7) (72, 73). For example, miR-7-5p inhibits the expression of ASD risk gene PAX6 (74), a crucial transcription factor for neural tissue development, and regulates dopaminergic neuron differentiation (75). miR-7-5p also regulated the expression of ASD risk gene SHANK3 and affected the dendritic spines in hippocampal neurons (76). Moreover, miR-7 could regulate cerebral cortex development through the p53 pathway (77). Our bioinformatic analysis shows that its related pathways include fatty acid biosynthesis, biotin metabolism, lysine degradation, and the Hippo signaling pathway (Supplementary Material 7, Figure 2B). Therefore, it is reasonable to speculate that miR-7 dysregulation may lead to neurologic disorders including ASD.
Each ASD-miRNA was validated to target several ASD risk genes and involves many signaling pathways (Supplementary Materials 7–9). TNRC6B, PTEN, AGO1, AGO2, SKI, and SMAD4 are among the most commonly targeted ASD genes (Supplementary Materials 7–9). TNRC6B, AGO1, and AGO2 participate in miRNA-mediated translational inhibition (78), which can affect a variety of cellular functions, given the various targets in this pathway. PTEN gene has been identified as a strong candidate gene for ASD (79). Defective PTEN protein interacts with tumor protein p53 to suppress energy production in neurons, causing detrimental changes in mitochondrial DNA and abnormal levels of energy production in the cerebellum and hippocampus, regions of the brain vital to social function and cognition, and Pten haploinsufficient mice exhibit autistic-like behavior with brain mitochondrial dysfunction and accumulation of mitochondrial DNA loss (80). Moreover, PTEN antagonizes PI3K-AKT signaling, which is essential for axon guidance and dendritic outgrowth (81). So PTEN-deficiency may affect neuronal connectivity, synaptic plasticity, and the development of autistic behavior (81). The SKI protein regulates the TGF-beta pathway by interacting with SMAD proteins, such as SMAD4, and plays a key role in tissue development (including the brain) during embryogenesis (82).
Tissue subgroup analysis showed that ASD-miRNAs in the brain, blood, and saliva participate in the FoxO signaling pathway (Figures 2A–C; Supplementary Materials 7–9), and several ASD risk genes are present in this pathway (Supplementary Materials 7–9). Recently, FoxO transcription factors have emerged as important regulators of cell development and function in the nervous system (83) and are implicated in neurological diseases such as Parkinson's disease and Huntington's disease (83, 84). A growing body of evidence demonstrates that miRNAs can directly regulate FoxO transcripts in various physiological and pathological conditions (85). However, there is still a lack of literature on the FoxO signaling pathway in ASD.
Notably, ASD-miRNAs were implicated in many cancer pathways (Supplementary Materials 7–9; Figures 2A–C). This supports evidence that ASD shares overlapping genes and pathways with cancers (86). For example, several ASD-miRNAs (miR-21-3p, miR-106a-5p, miR-155-5p, miR-92a-3p, miR-23a-3p, miR-106b-5p, and miR-19b-3p) have been shown to target PTEN (Supplementary Materials 7–9), a ubiquitously expressed tumor suppressor that is commonly inactivated in human cancers (87). Additionally, several ASD risk genes, including PTEN, are regulators of p53, which act as a tumor suppressor. Besides, the p53 tumor suppressor network cross-talks with the miRNA regulation system (88). For example, dysregulated microRNAs in ASD (miR-15a-5p, miR-19b-3p, and miR-92a-3p) target the p53 pathway (Supplementary Material 8; Figure 2A). In contrast, the p53 pathway could downregulate microRNAs such as let-7a and miR-17/92 cluster (miR-19b-1, miR-20a, and miR-92a) and upregulates microRNAs such as miR-107, miR-34c, miR-145, miR-15a, miR-23b, and miR-486 (89, 90). These miRNAs mediating p53 function in tumor suppression are also dysregulated in ASD. Evidence showed that the p53 gene is more deficit in ASD children and their fathers (91). So dysregulated miRNAs and the p53 signaling pathway may impact each other mutually and contribute to ASD. Since ASD and cancer have mechanistic similarities, these have clinical importance, such as repurposing feasible cancer drugs for ASD-targeted therapies (86).
It should be highlighted that ASD-miRNAs are implicated in various metabolic pathways (Supplementary Materials 7–9; Figures 2A–C). This is consistent with the clinical finding that ASD persons have various metabolic problems (92–94). One explanation is mitochondria dysfunction since mitochondria are vital for bioenergetic and biosynthetic processes, including lipids, amino acids, and nucleotide metabolism (95). Many microRNAs including miR-23a-3p, miR-23b-3p, miR-107, and miR-103 are found in mitochondria (96); they may regulate various biological pathways, such as p53 signaling, neurotrophin signaling, TGF cycle, cell cycle, and ubiquitin-mediated proteolysis (97). For example, miR-23a-3p and miR-23b-3p bind to (glutaminase) GLS-mRNA and suppress its translation; thus, they are involved in the mitochondrial amino acid metabolism (96, 98). miR-107 and miR-103 regulate insulin signaling and glucose homeostasis (99). Cellular nutrient and energy-sensing by mTOR signaling regulates almost all aspects of metabolism and mitochondrial biogenesis and plays an important role in glucose homeostasis, lipid homeostasis, immune function, brain function, cancer, etc. (100, 101). Notably, several ASD risk genes are present in mTOR signaling, including IGF1, MTOR, PIK3R2, PTEN, RHEB, TSC1, and TSC2. Some of them (PTEN and TSC2) are direct target genes of p53, which regulates mTOR signaling and many metabolic activities (102). Taken together, microRNA, metabolism, and mitochondria are closely interconnected and associated with other cellular pathways, which may partially explain the etiology of mitochondrial dysfunction and diverse clinical manifestations of ASD.
It is well-known that immune dysregulation/inflammation participates in the pathogenesis of ASD. Reinforcing this perspective, our pathway enrichment analysis shows that ASD-miRNAs are involved in immune/inflammation-associated pathways, such as the NF-κB, Hippo, TGF-beta, and mTOR signaling pathways (Figures 2A–C; Supplementary Materials 7–9). The TGF-beta signaling pathway is a crucial regulator of the immune system and plays a critical role in the regulation of inflammation and embryo development (103). Evidence shows that TGF-beta1 regulates the PI3K/Akt/Wnt/beta-catenin signaling pathway and restores hippocampal synaptic plasticity and memory (104). TGF-beta1 also activates the MAPK signaling pathway and modulates neurogenesis (105). TGF-beta can also activate other pathways including mTOR signaling pathways and the NF-κB pathway (106). Moreover, TGF-beta signaling cross-talks with miRNAs and upregulates miRNAs such as the miR-181 family, the miR-17/92 cluster, miR-155, and the miR-23/24/27 cluster; downregulates the miR-200 family, miR-203, let-7, miR-34a, and miR-584 (107). On the other hand, miRNAs including the miR-106b/205 cluster (including miR-106b-5p and miR-93-5p) regulate TGF-beta signaling by convergently suppressing a range of TGF-beta signaling components, such as SMAD4 (107). This may partly explain the reason why microRNAs are dysregulated in ASD. However, the functions of TGF-beta in ASD are little understood (108). Further research is needed to investigate the role of microRNAs and TGF-beta signaling in immune dysregulation in ASD.
The cadherin genes (including PCDH10, CDH5, CDH8, CDH9, and CDH15) are ASD risk genes and function as essential cell adhesion molecules. Some of them may interact with beta-catenin, which is encoded by the CTNNB1 gene (a validated target of miR-155-5p, miR-27a-3p, and miR-106a-5p, etc.). Cadherins act as an intracellular signal transducer in various signaling pathways including the adherens junctions and the Hippo pathway and play a critical role in the development and cellular function (109).
Adherens junctions are cadherin-based protein complexes, which exist at intercellular adhesions of endothelial and epithelial tissues and play a critical role in embryogenesis and cortical development (110). Evidence confirmed the links between adherens junction and ASD (111). Interestingly, a functional local RNA interference system has been recently found in the epithelial adhesion junctions (112), which affects miRNAs and mRNAs, indicating that miRNAs play an important role in regulating epithelial and endothelial cell functions in development and disease (113). For example, miR-155 adversely affected brain–blood-barrier function during neuroinflammation by targeting cell–cell complex molecules, such as AA2, claudin-1, and molecules that are critical in cell-to-extracellular matrix (ECM) interactions including dedicator of cytokinesis 1 and syntenin-1 (114). Thus, miRNAs may contribute to adherens junctions dysfunction and brain–blood-barrier and intestinal epithelial barriers impairment in ASD (115, 116).
The extracellular matrix and its receptors are essential for neuronal migration during brain development and are involved in the maintenance of stable neuronal connections and regulation of synaptic plasticity (117). ASD risk genes including RELN, LAMB1, and THBS1 are involved in ECM-receptor interaction. However, there is still a lack of literature on ECM-receptor interaction in ASD.
Adherens junctions, extracellular matrix, or cadherins could also regulate the Hippo pathway activity (118, 119), which plays a critical role in regulating development and organ size (120). Hippo signaling also cross-talks with innate immunity and regulates inflammation (121). Several ASD risk genes are implicated in Hippo signaling, including CTNNB1, SMAD4, and PPP2R1B. However, there is still little research in the literature regarding the Hippo signaling pathway in ASD.
Thyroid hormones are important regulators of development, growth, and metabolism and crucial for normal brain development. Thyroid hormones regulate AMPK activity and fatty acid metabolism in the central nervous system (122). Maternal thyroid disorders during pregnancy were found to be associated with an increased risk of ASD in offspring (123), and brain genes were altered (124). However, few studies have examined whether postnatal thyroid hormone levels influence the risk of ASD and the underlying mechanisms remain unclear (125). The thyroid hormone and its receptor-related genes (including THRA, TPO, TRIP12, and NR4A2) have been linked with ASD, and miRNAs are necessary for thyroid hormone production (126). Further studies are needed to investigate the microRNA regulation of thyroid hormones in ASD during pregnancy and postnatal.
Estrogen and estrogen receptors play critical roles in brain development and functions, including synaptogenesis, corticogenesis, cognition, and learning (127). Evidence showed that prenatal and postnatal estrogen signaling impairment may contribute to ASD (128, 129). Consistent with this, several ASD-miRNAs (including miR-142-3p, miR-151a-3p, miR-19b-3p, miR-32-5p, miR-221-3p, miR-320a, miR-338-5p, and miR-423-5p) target ASD risk genes including PIK3R2, ADCY3, ITPR1, and GNAI1 (Supplementary Materials 7–9), which are also implicated in estrogen signaling.
Interestingly, the ASD-miRNAs target circadian rhythm genes including RORA, RORB, PER1, PER2, BTRC, NPAS2, NR1D1, and CSNK1E, which are also ASD risk genes (Supplementary Materials 7–9). Circadian rhythm disorders are associated with neurological (130) and other functional impairments in ASD (131). Evidence suggests that circadian rhythm genes may be correlated with ASD (132) and cause sleep disorders that are common in ASD (133). Moreover, salivary microRNAs (miR-24-3p, miR-200b-3p, miR-203a-3p, and miR-26a-5p) are associated with sleep disorders in children with ASD, so microRNAs in biofluids such as saliva could have diagnostic and therapeutic values in circadian rhythm disorder and ASD (134).
Collectively, this study clarified overlapping altered microRNAs in ASD and explored their target genes and associated pathways. We also highlight pathways that are significantly enriched while being less studied in ASD, such as adherens junctions, ECM receptor interaction, FoxO, Hippo, and TGF-beta signaling pathway.
Some limitations of this study exist. First, as microRNA profiling and analysis methods are heterogeneous among studies and much raw data are not available, it is difficult to perform a quantitative meta-analysis. Second, various diagnostic approaches were employed, and six studies did not report diagnostic tools (10, 14, 18, 33, 35, 53). The change of diagnostic criteria, for example, from DSM-IV to DSM-V, may lead to the inconsistency of research participants. The heterogeneity of diagnostic criteria and participants made comparisons between studies challenging. Subgroup analysis stratifying by homogeneous characteristics dimensionally or categorically within ASD would be helpful. However, this was not practicable as the included studies did not give sufficient phenotype/behavioral information on ASD individuals. It should also be noticed that some dysregulated microRNAs are located on the X chromosome and miR-29c-3p (27), miR-4732-5p, and miR-423-3p show gender differences (32). However, this link has not been identified in other studies (19, 22, 33, 38, 39). In addition, some research only included male or female ASD participants. Thus, it is unclear if particular miRNA dysregulation in ASD is gender-specific. Given the heterogeneity and gender differences in ASD, future microRNA expression research with more female participants and stratification by ASD subtype or gender will be valuable.
Conclusion
In conclusion, our systematic review on miRNA expression profiling studies identified a number of altered microRNAs in ASD, especially miR-23a-3p, miR-27a-3p, miR-106b-5p, miR-93-5p, miR-7-5p, miR-146a-5p, and miR-155-5p. Some of these microRNAs have the potential to serve as biomarkers for ASD. Each of these altered microRNAs was validated to target many ASD risk genes. The target genes of these microRNAs are implicated in various pathways associated with ASD and form very rich networks, which further highlights the importance of these microRNAs in ASD etiology. However, few studies have reported the implication of microRNAs and some associated pathways in ASD pathogenesis, such as adherens junctions, ECM receptor interaction, FoxO, Hippo, TGF-beta signaling pathway, etc. More research is needed to examine microRNA expression in ASD and their associated target genes and pathways to better understand ASD pathogenesis. This may contribute to the use of microRNAs as potential personalized biomarkers and therapeutic targets.
Data Availability Statement
The original contributions presented in the study are included in the article/Supplementary Material, further inquiries can be directed to the corresponding author.
Author Contributions
Z-XH: conceptualization, methodology, software, formal analysis, bioinformatic analysis, investigation, resources, data extraction, data curation, writing - original draft, writing - review and editing, visualization, and funding acquisition (Qihang Funds of Fujian Medical University). YC: conceptualization, methodology, writing - review and editing, supervision, project administration, and funding acquisition. H-RG: data extraction and data curation. G-FC: data extraction. All authors read and approved the final manuscript.
Funding
This research was supported by the National Key Research and Development Program of China (Grant No. 2016YFC1306204), the Joint Funds for the innovation of science and Technology, Fujian province (Grant No. 2017Y9043), and Qihang Funds of Fujian Medical University (Grant No. 2018QH2032). The funding sources had no role in the design and conduct of the study; collection, management, analysis, and interpretation of the data; preparation, review, or approval of the manuscript; and decision to submit the manuscript for publication.
Conflict of Interest
The authors declare that the research was conducted in the absence of any commercial or financial relationships that could be construed as a potential conflict of interest.
Publisher's Note
All claims expressed in this article are solely those of the authors and do not necessarily represent those of their affiliated organizations, or those of the publisher, the editors and the reviewers. Any product that may be evaluated in this article, or claim that may be made by its manufacturer, is not guaranteed or endorsed by the publisher.
Acknowledgments
We thank the associate editor and the reviewers for their useful feedback that improved this paper.
Supplementary Material
The Supplementary Material for this article can be found online at: https://www.frontiersin.org/articles/10.3389/fpsyt.2021.630876/full#supplementary-material
Abbreviations
ADCY3, adenylate cyclase 3; ADI-R, Autism Diagnostic Interview-Revised; ADOS, Autism Diagnostic Observation Schedule; AGO1, argonaute RISC component 1; Akt, also known as protein kinase B; AMPK, 5′ adenosine monophosphate-activated protein kinase; ASD, Autism spectrum disorder; BTRC, beta-transducin repeat containing E3 ubiquitin protein ligase; CARS, Childhood Autism Rating Scale; CARS-II, Childhood Autism Rating Scale, Second edition; CDH5, cadherin 5; CDH8, cadherin 8; CDH9, cadherin 9; CHD8, chromodomain helicase DNA binding protein 8; CSNK1E, casein kinase 1 epsilon; Ct, cycles-to-threshold; CTNNB1, catenin beta 1; CUL3, cullin 3; DSM-5, Diagnostic and Statistical Manual of Mental Disorders, 5th Edition; DSM-IV, Diagnostic and Statistical Manual of Mental Disorders, 4th Edition; DSM-IV-TR, Diagnostic and Statistical Manual of Mental Disorders, 4th Edition, Text Revision; ECM, extracellular matrix; FC, fold change; FDR, false discovery rate correction; FoxO, forkhead box O; GNAI1, G protein subunit alpha i1; IGF1, insulin like growth factor 1; IL6, interleukin 6; ITPR1, Inositol 1, 4, 5-trisphosphate receptor type 1; KDM3B, Lysine Demethylase 3B; KDM6B, lysine demethylase 6B; KEGG, Kyoto Encyclopedia of Genes and Genomes; KMT2A, lysine methyltransferase 2A; KMT2C, lysine methyltransferase 2C; KMT5B, lysine methyltransferase 5B; LAMB1, laminin subunit beta 1; MAPK, mitogen-activated protein kinase; MECP2, Methyl CpG binding protein 2; MED13, mediator complex subunit 13; mTOR, mammalian (mechanistic) target of rapamycin; MTOR, mechanistic target of rapamycin kinase; N/A, not available; NF-kappa B, Nuclear factor-kappa B; NPAS2, neuronal PAS domain protein 2; NR1D1, nuclear receptor subfamily 1 group D member 1; NR4A2, nuclear receptor subfamily 4 group A member 2; NSD1, nuclear receptor binding SET domain protein 1; PAX6, paired box 6; PBMC, peripheral blood monocytes; PCDH10, protocadherin 10; PER1, period circadian regulator 1; PER2, period circadian regulator 2; PI3K, phosphatidylinositol 3′ -kinase; PIK3R2, phosphoinositide-3-kinase regulatory subunit 2; PPP2R1B, protein phosphatase 2 scaffold subunit Abeta; PRISMA, Preferred Reporting Items for Systematic reviews and Meta-Analyses; PTEN, phosphatase and tensin homolog; qRT-PCR, quantitative reverse-transcription/real time polymerase chain reaction; RELN, reelin; RHEB, Ras homolog, mTORC1binding; RNA-Seq, ribonucleic acid sequencing; RORA, RAR related orphan receptor A; RORB, RAR related orphan receptor B; SETD1B, SET domain containing 1B; SHANK3, SH3 and multiple ankyrin repeat domains 3; SKI, Sloan–Kettering Institute proto-oncogene; SMAD, mothers against decapentaplegic homolog; TBL1XR1, transducin beta like 1 X-linked receptor 1; TCF4, transcription factor 4; TGF, transforming growth factor; THBS1, thrombospondin 1; THRA, thyroid hormone receptor alpha; TNRC6B, trinucleotide repeat containing adaptor 6B; TPO, thyroid peroxidase; TRIP12, thyroid hormone receptor interactor 12; TSC1, tuberous sclerosis complex 1; TSC2, tuberous sclerosis complex 2; Wnt, Wingless-type/Int-1.
References
1. Diagnostic and Statistical Manual of Mental Disorders (DSM-5®). Washington, DC: American Psychiatric Association Publishing (2013). doi: 10.1176/appi.books.9780890425596
2. Zhou J, Park CY, Theesfeld CL, Wong AK, Yuan Y, Scheckel C, et al. Whole-genome deep-learning analysis identifies contribution of noncoding mutations to autism risk. Nat Genet. (2019) 51:973–80. doi: 10.1038/s41588-019-0420-0
3. Yuen RK, Merico D, Bookman M, L Howe J, Thiruvahindrapuram B, Patel RV, et al. Whole genome sequencing resource identifies 18 new candidate genes for autism spectrum disorder. Nat Neurosci. (2017) 20:602–11. doi: 10.1038/nn.4524
4. Vorstman JAS, Parr JR, Moreno-De-Luca D, Anney RJL, Nurnberger JI, Hallmayer JF. Autism genetics: opportunities and challenges for clinical translation. Nat Rev Genet. (2017) 18:362–76. doi: 10.1038/nrg.2017.4
5. Quesnel-Vallières M, Weatheritt RJ, Cordes SP, Blencowe BJ. Autism spectrum disorder: insights into convergent mechanisms from transcriptomics. Nat Rev Genet. (2019) 20:51–63. doi: 10.1038/s41576-018-0066-2
6. O'Brien J, Hayder H, Zayed Y, Peng C. Overview of MicroRNA biogenesis, mechanisms of actions, and circulation. Front Endocrinol (Lausanne). (2018) 9:402. doi: 10.3389/fendo.2018.00402
7. Lai X, Wolkenhauer O, Vera J. Understanding microRNA-mediated gene regulatory networks through mathematical modelling. Nucleic Acids Res. (2016) 44:6019–35. doi: 10.1093/nar/gkw550
8. Yapijakis C. Regulatory role of MicroRNAs in brain development and function. Adv Exp Med Biol. (2020) 1195:237–47. doi: 10.1007/978-3-030-32633-3_32
9. Rajman M, Schratt G. MicroRNAs in neural development: from master regulators to fine-tuners. Development. (2017) 144:2310–22. doi: 10.1242/dev.144337
10. Abu-Elneel K, Liu T, Gazzaniga FS, Nishimura Y, Wall DP, Geschwind DH, et al. Heterogeneous dysregulation of microRNAs across the autism spectrum. Neurogenetics. (2008) 9:153–61. doi: 10.1007/s10048-008-0133-5
11. Mor M, Nardone S, Sams DS, Elliott E. Hypomethylation of miR-142 promoter and upregulation of microRNAs that target the oxytocin receptor gene in the autism prefrontal cortex. Mol Autism. (2015) 6:46. doi: 10.1186/s13229-015-0040-1
12. Ander BP, Barger N, Stamova B, Sharp FR, Schumann CM. Atypical miRNA expression in temporal cortex associated with dysregulation of immune, cell cycle, and other pathways in autism spectrum disorders. Mol Autism. (2015) 6:37. doi: 10.1186/s13229-015-0029-9
13. Wu YE, Parikshak NN, Belgard TG, Geschwind DH. Genome-wide, integrative analysis implicates microRNA dysregulation in autism spectrum disorder. Nat Neurosci. (2016) 19:1463–76. doi: 10.1038/nn.4373
14. Wright C, Shin JH, Rajpurohit A, Deep-Soboslay A, Collado-Torres L, Brandon NJ, et al. Altered expression of histamine signaling genes in autism spectrum disorder. Transl Psychiat. (2017) 7:e1126. doi: 10.1038/tp.2017.87
15. Schumann CM, Sharp FR, Ander BP, Stamova B. Possible sexually dimorphic role of miRNA and other sncRNA in ASD brain. Mol Autism. (2017) 8:4. doi: 10.1186/s13229-017-0117-0
16. Pagan C, Goubran-Botros H, Delorme R, Benabou M, Lemière N, Murray K, et al. Disruption of melatonin synthesis is associated with impaired 14-3-3 and miR-451 levels in patients with autism spectrum disorders. Sci Rep. (2017) 7:2096. doi: 10.1038/s41598-017-02152-x
17. Nguyen LS, Fregeac J, Bole-Feysot C, Cagnard N, Iyer A, Anink J, et al. Role of miR-146a in neural stem cell differentiation and neural lineage determination: relevance for neurodevelopmental disorders. Mol Autism. (2018) 9:38. doi: 10.1186/s13229-018-0219-3
18. Almehmadi KA, Tsilioni I, Theoharides TC. Increased expression of miR-155p5 in amygdala of children with autism spectrum disorder. Autism Res. (2020) 13:18–23. doi: 10.1002/aur.2205
19. Mundalil Vasu M, Anitha A, Thanseem I, Suzuki K, Yamada K, Takahashi T, et al. Serum microRNA profiles in children with autism. Mol Autism. (2014) 5:40. doi: 10.1186/2040-2392-5-40
20. Cirnigliaro M, Barbagallo C, Gulisano M, Domini CN, Barone R, Barbagallo D, et al. Expression and regulatory network analysis of miR-140-3p, a new potential serum biomarker for autism spectrum disorder. Front Mol Neurosci. (2017) 10:250. doi: 10.3389/fnmol.2017.00250
21. Yu D, Jiao XQ, Cao T, Huang FS. Serum miRNA expression profiling reveals miR-486-3p may play a significant role in the development of autism by targeting ARID1B. Neuroreport. (2018) 29:1431–6. doi: 10.1097/WNR.0000000000001107
22. Zamil BM, Ali-Labib R, Youssef WY, Khairy E. Evaluation of miR-106a and ADARB1 in autistic children. Gene Reports. (2020) 18:100586. doi: 10.1016/j.genrep.2019.100586
23. Popov NT, Minchev DS, Naydenov M, Minkov IN, Vachev TI. Investigation of circulating serum MicroRNA-328-3p and MicroRNA-3135a expression as promising novel biomarkers for autism spectrum disorder. Balkan J Med Genet. (2018) 21:5–12. doi: 10.2478/bjmg-2018-0026
24. Popov NT, Madjirova NP, Minkov IN, Vachev TI. Micro RNA HSA-486-3P gene expression profiling in the whole blood of patients with autism. Biotechnol Biotechnol Equip. (2012) 26:3385–8. doi: 10.5504/BBEQ.2012.0093
25. Vachev T, Minkov I, Stoyanova V, Popov N. Down regulation of miRNA let-7b-3p and let-7d-3p in the peripheral blood of children with autism spectrum disorder. Int J Curr Microbiol App Sci. (2013) 2:384–8.
26. Huang F, Long Z, Chen Z, Li J, Hu Z, Qiu R, et al. Investigation of gene regulatory networks associated with autism spectrum disorder based on miRNA expression in China. PLoS ONE. (2015) 10:e0129052. doi: 10.1371/journal.pone.0129052
27. Eftekharian MM, Komaki A, Oskooie VK, Namvar A, Taheri M, Ghafouri-Fard S. Assessment of apoptosis pathway in peripheral blood of autistic patients. J Mol Neurosci. (2019) 69:588–96. doi: 10.1007/s12031-019-01387-9
28. Kichukova TM, Popov NT, Ivanov IS, Vachev TI. Profiling of circulating serum microRNAs in children with autism spectrum disorder using stem-loop qRT-PCR assay. Mol Autism. (2017) 59:43–52. doi: 10.1515/folmed-2017-0009
29. Vaccaro TDS, Sorrentino JM, Salvador S, Veit T, Souza DO, De Almeida RF. Alterations in the MicroRNA of the blood of autism Spectrum disorder patients: effects on epigenetic regulation and potential biomarkers. Behav Sci. (2018) 8:75. doi: 10.3390/bs8080075
30. Nakata M, Kimura R, Funabiki Y, Awaya T, Murai T, Hagiwara M. MicroRNA profiling in adults with high-functioning autism spectrum disorder. Mol Brain. (2019) 12:1–4. doi: 10.1186/s13041-019-0508-6
31. Jyonouchi H, Geng L, Streck DL, Dermody JJ, Toruner GA. MicroRNA expression changes in association with changes in interleukin-1ß/interleukin10 ratios produced by monocytes in autism spectrum disorders: their association with neuropsychiatric symptoms and comorbid conditions (observational study). J Neuroinflammation. (2017) 14:229. doi: 10.1186/s12974-017-1003-6
32. Jyonouchi H, Geng L, Toruner GA, Rose S, Bennuri SC, Frye RE. Serum microRNAs in ASD: association with monocyte cytokine profiles and mitochondrial respiration. Front Psychiatry. (2019) 10:614. doi: 10.3389/fpsyt.2019.00614
33. Atwan H, Assarehzadegan MA, Shekarabi M, Jazayeri SM, Barfi S, Shokouhi Shoormasti R, et al. Assessment of miR-181b-5p, miR-23a-3p, BCL-2, and IL-6 in peripheral blood mononuclear cells of autistic patients; likelihood of reliable biomarkers. Iran J Allergy Asthma Immunol. (2020) 19:74–83. doi: 10.18502/ijaai.v19i1.2420
34. Talebizadeh Z, Butler MG, Theodoro MF. Feasibility and relevance of examining lymphoblastoid cell lines to study role of microRNAs in autism. Autism Res. (2008) 1:240–50. doi: 10.1002/aur.33
35. Sarachana T, Zhou R, Chen G, Manji HK, Hu VW. Investigation of post-transcriptional gene regulatory networks associated with autism spectrum disorders by microRNA expression profiling of lymphoblastoid cell lines. Genome Med. (2010) 2:23. doi: 10.1186/gm144
36. Seno MMG, Hu PZ, Gwadry FG, Pinto D, Marshall CR, Casallo G, et al. Gene and miRNA expression profiles in autism spectrum disorders. Brain Res. (2011) 1380:85–97. doi: 10.1016/j.brainres.2010.09.046
37. Bleazard TP. Investigating the Role of MicroRNAs in Autism. The University of Manchester, United Kingdom (2018).
38. Hicks SD, Ignacio C, Gentile K, Middleton FA. Salivary miRNA profiles identify children with autism spectrum disorder, correlate with adaptive behavior, and implicate ASD candidate genes involved in neurodevelopment. BMC Pediatr. (2016) 16:52. doi: 10.1186/s12887-016-0586-x
39. Hicks SD, Carpenter RL, Wagner KE, Pauley R, Barros M, Tierney-Aves C, et al. Saliva MicroRNA differentiates children with autism from peers with typical and atypical development. J Am Acad Child Adolesc Psychiatry. (2020) 59:296–308. doi: 10.1016/j.jaac.2019.03.017
40. Sehovic E, Spahic L, Smajlovic-Skenderagic L, Pistoljevic N, Dzanko E, Hajdarpasic A. Identification of developmental disorders including autism spectrum disorder using salivary miRNAs in children from Bosnia and Herzegovina. PLoS ONE. (2020) 15:e0232351. doi: 10.1371/journal.pone.0232351
41. Moore D, Meays BM, Madduri LSV, Shahjin F, Chand S, Niu M, et al. Downregulation of an evolutionary young miR-1290 in an iPSC-derived neural stem cell model of autism spectrum disorder. Stem Cells Int. (2019) 2019:8710180. doi: 10.1155/2019/8710180
42. Nguyen LS, Lepleux M, Makhlouf M, Martin C, Fregeac J, Siquier-Pernet K, et al. Profiling olfactory stem cells from living patients identifies miRNAs relevant for autism pathophysiology. Mol Autism. (2016) 7:1. doi: 10.1186/s13229-015-0064-6
43. Kozomara A, Birgaoanu M, Griffiths-Jones S. miRBase: from microRNA sequences to function. Nucleic Acids Res. (2019) 47:D155–62. doi: 10.1093/nar/gky1141
44. Hicks SD, Middleton FA. A comparative review of microRNA expression patterns in autism spectrum disorder. Front Psychiatry. (2016) 7:176. doi: 10.3389/fpsyt.2016.00176
45. Moher D, Liberati A, Tetzlaff J, Altman DG. Preferred reporting items for systematic reviews and meta-analyses: the PRISMA statement. BMJ. (2009) 339:b2535. doi: 10.1136/bmj.b2535
46. Huang H-Y, Lin Y-C-D, Li J, Huang K-Y, Shrestha S, Hong H-C, et al. miRTarBase 2020: updates to the experimentally validated microRNA-target interaction database. Nucleic Acids Res. (2020) 48:D148–54. doi: 10.1093/nar/gkz896
47. Abrahams BS, Arking DE, Campbell DB, Mefford HC, Morrow EM, Weiss LA, et al. SFARI Gene 2.0: a community-driven knowledgebase for the autism spectrum disorders (ASDs). Mol Autism. (2013) 4:36. doi: 10.1186/2040-2392-4-36
48. Pereanu W, Larsen EC, Das I, Estévez MA, Sarkar AA, Spring-Pearson S, et al. AutDB: a platform to decode the genetic architecture of autism. Nucleic Acids Res. (2018) 46:D1049–54. doi: 10.1093/nar/gkx1093
49. Zhou Y, Zhou B, Pache L, Chang M, Khodabakhshi AH, Tanaseichuk O, et al. Metascape provides a biologist-oriented resource for the analysis of systems-level datasets. Nat Commun. (2019) 10:1523. doi: 10.1038/s41467-019-09234-6
50. Uhlén M, Fagerberg L, Hallström BM, Lindskog C, Oksvold P, Mardinoglu A, et al. Proteomics. Tissue-based map of the human proteome. Science. (2015) 347:1260419. doi: 10.1126/science.1260419
51. Vlachos IS, Zagganas K, Paraskevopoulou MD, Georgakilas G, Karagkouni D, Vergoulis T. DIANA-miRPath v3.0: deciphering microRNA function with experimental support. Nucleic Acids Res. (2015) 43:W460–6. doi: 10.1093/nar/gkv403
52. Ottawa Hospital Research Institute. The Newcastle-Ottawa Scale (NOS) for assessing the quality of nonrandomised studies in meta-analyses. Ottawa, Ontario, Canada (2020). Available online at: http://www.ohri.ca/programs/clinical_epidemiology/oxford.asp (accessed August 3, 2020).
53. Lin C-Y, Chang K-W, Lin C-Y, Wu J-Y, Coon H, Huang P-H, et al. Allele-specific expression in a family quartet with autism reveals mono-to-biallelic switch and novel transcriptional processes of autism susceptibility genes. Sci Rep. (2018) 8:4277. doi: 10.1038/s41598-018-22753-4
54. Winden KD, Ebrahimi-Fakhari D, Sahin M. Abnormal mTOR activation in autism. Annu Rev Neurosci. (2018) 41:1–23. doi: 10.1146/annurev-neuro-080317-061747
55. Lin S-T, Huang Y, Zhang L, Heng MY, Ptácek LJ, Fu Y-H. MicroRNA-23a promotes myelination in the central nervous system. Proc Natl Acad Sci USA. (2013) 110:17468–73. doi: 10.1073/pnas.1317182110
56. Wrigley S, Arafa D, Tropea D. Insulin-like growth factor 1: at the crossroads of brain development and aging. Front Cell Neurosci. (2017) 11:14. doi: 10.3389/fncel.2017.00014
57. Chen S-T, Lai W-J, Zhang W-J, Chen Q-P, Zhou L-B, So K-F, et al. Insulin-like growth factor 1 partially rescues early developmental defects caused by SHANK2 knockdown in human neurons. Neural Regener Res. (2020) 15:2335–43. doi: 10.4103/1673-5374.285002
58. Linker SB, Mendes APD, Marchetto MC. IGF-1 treatment causes unique transcriptional response in neurons from individuals with idiopathic autism. Mol Autism. (2020) 11:55. doi: 10.1186/s13229-020-00359-w
59. O'Leary HM, Kaufmann WE, Barnes KV, Rakesh K, Kapur K, Tarquinio DC, et al. Placebo-controlled crossover assessment of mecasermin for the treatment of Rett syndrome. Ann Clin Transl Neur. (2018) 5:323–32. doi: 10.1002/acn3.533
60. Husmann D, Gozani O. Histone lysine methyltransferases in biology and disease. Nat Struct Mol Biol. (2019) 26:880–9. doi: 10.1038/s41594-019-0298-7
61. Faundes V, Newman WG, Bernardini L, Canham N, Clayton-Smith J, Dallapiccola B, et al. Histone lysine methylases and demethylases in the landscape of human developmental disorders. Am J Hum Genet. (2018) 102:175–87. doi: 10.1016/j.ajhg.2017.11.013
62. Xi T, Jin F, Zhu Y, Wang J, Tang L, Wang Y, et al. miR-27a-3p protects against blood-brain barrier disruption and brain injury after intracerebral hemorrhage by targeting endothelial aquaporin-11. J Biol Chem. (2018) 293:20041–50. doi: 10.1074/jbc.RA118.001858
63. Camkurt MA, Karababa F, Erdal ME, Bayazit H, Kandemir SB, Ay ME, et al. Investigation of dysregulation of several MicroRNAs in peripheral blood of schizophrenia patients. Clin Psychopharm Neu. (2016) 14:256–60. doi: 10.9758/cpn.2016.14.3.256
64. Zadehbagheri F, Hosseini E, Bagheri-Hosseinabadi Z, Rekabdarkolaee HM, Sadeghi I. Profiling of miRNAs in serum of children with attention-deficit hyperactivity disorder shows significant alterations. J Psychiatr Res. (2019) 109:185–92. doi: 10.1016/j.jpsychires.2018.12.013
65. Lyst MJ, Bird A. Rett syndrome: a complex disorder with simple roots. Nat Rev Genet. (2015) 16:261–75. doi: 10.1038/nrg3897
66. Balicza P, Varga NÁ, Bolgár B, Pentelényi K, Bencsik R, Gál A, et al. Comprehensive analysis of rare variants of 101 autism-linked genes in a hungarian cohort of autism spectrum disorder patients. Front Genet. (2019) 10:434. doi: 10.3389/fgene.2019.00434
67. Husson T, Lecoquierre F, Cassinari K, Charbonnier C, Quenez O, Goldenberg A, et al. Rare genetic susceptibility variants assessment in autism spectrum disorder: detection rate and practical use. Transl Psychiat. (2020) 10:77. doi: 10.1038/s41398-020-0760-7
68. Liu Z, Li X, Zhang JT, Cai YJ, Cheng TL, Cheng C, et al. Autism-like behaviours and germline transmission in transgenic monkeys overexpressing MeCP2. Nature. (2016) 530:98–102. doi: 10.1038/nature16533
69. Testa U, Pelosi E, Castelli G, Labbaye C. miR-146 and miR-155: two key modulators of immune response and tumor development. Non-coding RNA. (2017) 3:22. doi: 10.3390/ncrna3030022
70. Gaudet AD, Fonken LK, Watkins LR, Nelson RJ, Popovich PG. MicroRNAs: roles in regulating neuroinflammation. Neuroscientist. (2018) 24:221–45. doi: 10.1177/1073858417721150
71. Fregeac J, Moriceau S, Poli A, Nguyen LS, Oury F, Colleaux L. Loss of the neurodevelopmental disease-associated gene miR-146a impairs neural progenitor differentiation and causes learning and memory deficits. Mol Autism. (2020) 11:22. doi: 10.1186/s13229-020-00328-3
72. Zhao J, Zhou Y, Guo M, Yue D, Chen C, Liang G, et al. MicroRNA-7: expression and function in brain physiological and pathological processes. Cell Biosci. (2020) 10:77. doi: 10.1186/s13578-020-00436-w
73. Piwecka M, GlaŽar P, Hernandez-Miranda LR, Memczak S, Wolf SA, Rybak-Wolf A, et al. Loss of a mammalian circular RNA locus causes miRNA deregulation and affects brain function. Science. (2017) 357:eaam8526. doi: 10.1126/science.aam8526
74. Needhamsen M, White RB, Giles KM, Dunlop SA, Thomas MG. Regulation of Human PAX6 Expression by miR-7. Evol Bioinform Online. (2014) 10:107–13. doi: 10.4137/EBO.S13739
75. de Chevigny A, Coré N, Follert P, Gaudin M, Barbry P, Béclin C, et al. miR-7a regulation of Pax6 controls spatial origin of forebrain dopaminergic neurons. Nat Neurosci. (2012) 15:1120–6. doi: 10.1038/nn.3142
76. Choi S-Y, Pang K, Kim JY, Ryu JR, Kang H, Liu Z, et al. Post-transcriptional regulation of SHANK3 expression by microRNAs related to multiple neuropsychiatric disorders. Mol Brain. (2015) 8:74. doi: 10.1186/s13041-015-0165-3
77. Pollock A, Bian S, Zhang C, Chen Z, Sun T. Growth of the developing cerebral cortex is controlled by microRNA-7 through the p53 pathway. Cell Biosci. (2014) 7:1184–96. doi: 10.1016/j.celrep.2014.04.003
78. Briskin D, Wang PY, Bartel DP. The biochemical basis for the cooperative action of microRNAs. Proc Natl Acad Sci USA. (2020) 117:17764–74. doi: 10.1073/pnas.1920404117
79. Frazier TW. Autism spectrum disorder associated with germline heterozygous PTEN mutations. Cold Spring Harb Perspect Med. (2019) 9:a037002. doi: 10.1101/cshperspect.a037002
80. Napoli E, Ross-Inta C, Wong S, Hung C, Fujisawa Y, Sakaguchi D, et al. Mitochondrial dysfunction in Pten haplo-insufficient mice with social deficits and repetitive behavior: interplay between Pten and p53. PLoS ONE. (2012) 7:e42504. doi: 10.1371/journal.pone.0042504
81. Rademacher S, Eickholt BJ. PTEN in autism and neurodevelopmental disorders. Cold Spring Harb Perspect Med. (2019) 9:a036780. doi: 10.1101/cshperspect.a036780
82. Tecalco-Cruz AC, Ríos-López DG, Vázquez-Victorio G, Rosales-Alvarez RE, Macías-Silva M. Transcriptional cofactors Ski and SnoN are major regulators of the TGF-β/Smad signaling pathway in health and disease. Signal Transduct Tar. (2018) 3:15. doi: 10.1038/s41392-018-0015-8
83. Schäffner I, Minakaki G, Khan MA, Balta E-A, Schlötzer-Schrehardt U, Schwarz TJ, et al. FoxO function is essential for maintenance of autophagic flux and neuronal morphogenesis in adult neurogenesis. Neuron. (2018) 99:1188–203. doi: 10.1016/j.neuron.2018.08.017
84. Santo EE, Paik J. FOXO in neural cells and diseases of the nervous system. Curr Top Dev Biol. (2018) 127:105–18. doi: 10.1016/bs.ctdb.2017.10.002
85. Urbánek P, Klotz LO. Posttranscriptional regulation of FOXO expression: microRNAs and beyond. Br J Pharmacol. (2017) 174:1514–32. doi: 10.1111/bph.13471
86. Crawley JN, Heyer W-D, LaSalle JM. Autism and cancer share risk genes, pathways, and drug targets. Trends Genet. (2016) 32:139–46. doi: 10.1016/j.tig.2016.01.001
87. Álvarez-Garcia V, Tawil Y, Wise HM, Leslie NR. Mechanisms of PTEN loss in cancer: it's all about diversity. Semin Cancer Biol. (2019) 59:66–79. doi: 10.1016/j.semcancer.2019.02.001
88. Luo Z, Cui R, Tili E, Croce C. Friend or foe: microRNAs in the p53 network. Cancer Lett. (2018) 419:96–102. doi: 10.1016/j.canlet.2018.01.013
89. Goeman F, Strano S, Blandino G. MicroRNAs as key effectors in the p53 network. Int Rev Cell Mol Biol. (2017) 333:51–90. doi: 10.1016/bs.ircmb.2017.04.003
90. Liu J, Zhang C, Zhao Y, Feng Z. MicroRNA control of p53. J Cell Biochem. (2017) 118:7–14. doi: 10.1002/jcb.25609
91. Wong S, Napoli E, Krakowiak P, Tassone F, Hertz-Picciotto I, Giulivi C. Role of p53, mitochondrial DNA deletions, and paternal age in autism: a case-control study. Pediatrics. (2016) 137:e20151888. doi: 10.1542/peds.2015-1888
92. Orozco JS, Hertz-Picciotto I, Abbeduto L, Slupsky CM. Metabolomics analysis of children with autism, idiopathic-developmental delays, and Down syndrome. Transl Psychiat. (2019) 9:243. doi: 10.1038/s41398-019-0578-3
93. Liu A, Zhou W, Qu L, He F, Wang H, Wang Y, et al. Altered urinary amino acids in children with autism spectrum disorders. Front Cell Neurosci. (2019) 13:7. doi: 10.3389/fncel.2019.00007
94. Khemakhem AM, Frye RE, El-Ansary A, Al-Ayadhi L, Bacha AB. Novel biomarkers of metabolic dysfunction is autism spectrum disorder: potential for biological diagnostic markers. Metab Brain Dis. (2017) 32:1983–97. doi: 10.1007/s11011-017-0085-2
95. Spinelli JB, Haigis MC. The multifaceted contributions of mitochondria to cellular metabolism. Nat Cell Biol. (2018) 20:745–54. doi: 10.1038/s41556-018-0124-1
96. Geiger J, Dalgaard LT. Interplay of mitochondrial metabolism and microRNAs. Cell Mol Life Sci. (2017) 74:631–46. doi: 10.1007/s00018-016-2342-7
97. Sripada L, Tomar D, Prajapati P, Singh R, Singh AK, Singh R. Systematic analysis of small RNAs associated with human mitochondria by deep sequencing: detailed analysis of mitochondrial associated miRNA. PLoS ONE. (2012) 7:e44873. doi: 10.1371/journal.pone.0044873
98. Gao P, Tchernyshyov I, Chang T-C, Lee Y-S, Kita11 K. c-Myc suppression of miR-23 enhances mitochondrial glutaminase and glutamine metabolism. Nature. (2009) 458:762–5. doi: 10.1038/nature07823
99. Trajkovski M, Hausser J, Soutschek J, Bhat B, Akin A, Zavolan M, et al. MicroRNAs 103 and 107 regulate insulin sensitivity. Nature. (2011) 474:649–53. doi: 10.1038/nature10112
100. Kim J, Guan K-L. mTOR as a central hub of nutrient signalling and cell growth. Nat Cell Biol. (2019) 21:63–71. doi: 10.1038/s41556-018-0205-1
101. Saxton RA, Sabatini DM. mTOR signaling in growth, metabolism, and disease. Cell. (2017) 168:960–76. doi: 10.1016/j.cell.2017.02.004
102. Lacroix M, Riscal R, Arena G, Linares LK, Le Cam L. Metabolic functions of the tumor suppressor p53: implications in normal physiology, metabolic disorders, and cancer. Mol Metab. (2020) 33:2–22. doi: 10.1016/j.molmet.2019.10.002
103. Sanjabi S, Oh SA, Li MO. Regulation of the immune response by TGF-β: from conception to autoimmunity and infection. Cold Spring Harb Perspect Biol. (2017) 9:a022236. doi: 10.1101/cshperspect.a022236
104. Hu Y, Chen W, Wu L, Jiang L, Liang N, Tan L, et al. TGF-β1 restores hippocampal synaptic plasticity and memory in Alzheimer model via the PI3K/Akt/Wnt/β-catenin signaling pathway. J Mol Neurosci. (2019) 67:142–9. doi: 10.1007/s12031-018-1219-7
105. Stipursky J, Francis D, Gomes FCA. Activation of MAPK/PI3K/SMAD pathways by TGF-β(1) controls differentiation of radial glia into astrocytes in vitro. Dev Neurosci. (2012) 34:68–81. doi: 10.1159/000338108
106. Tzavlaki K, Moustakas A. TGF-β signaling. Biomolecules. (2020) 10:487. doi: 10.3390/biom10030487
107. Suzuki HI. MicroRNA control of TGF-β signaling. Int J Mol Sci. (2018) 19:1901. doi: 10.3390/ijms19071901
108. Baranova J, Dragunas G, Botellho MCS, Ayub ALP, Bueno-Alves R, Alencar RR, et al. Autism spectrum disorder: signaling pathways and prospective therapeutic targets. Cell Mol Life Sci. (2020) 41:619–49. doi: 10.1007/s10571-020-00882-7
109. Yulis M, Kusters DHM, Nusrat A. Cadherins: cellular adhesive molecules serving as signalling mediators. J Physiol. (2018) 596:3883–98. doi: 10.1113/JP275328
110. Veeraval L, O'Leary CJ, Cooper HM. Adherens junctions: guardians of cortical development. Front Cell Dev Biol. (2020) 8:6. doi: 10.3389/fcell.2020.00006
111. Folmsbee SS, Wilcox DR, Tyberghein K, De Bleser P, Tourtellotte WG, van Hengel J, et al. αT-catenin in restricted brain cell types and its potential connection to autism. J Mol Psychiat. (2016) 4: 2. doi: 10.1186/s40303-016-0017-9
112. Kourtidis A, Necela B, Lin W-H, Lu R, Feathers RW, Asmann YW, et al. Cadherin complexes recruit mRNAs and RISC to regulate epithelial cell signaling. J Cell Biol. (2017) 216:3073–85. doi: 10.1083/jcb.201612125
113. Kourtidis A, Anastasiadis PZ. Close encounters of the RNAi kind: the silencing life of the adherens junctions. Curr Opin Cell Biol. (2018) 54:30–6. doi: 10.1016/j.ceb.2018.03.001
114. Lopez-Ramirez MA, Wu D, Pryce G, Simpson JE, Reijerkerk A, King-Robson J, et al. MicroRNA-155 negatively affects blood-brain barrier function during neuroinflammation. FASEB J. (2014) 28:2551–65. doi: 10.1096/fj.13-248880
115. Lopez-Ramirez MA, Reijerkerk A, de Vries HE, Romero IA. Regulation of brain endothelial barrier function by microRNAs in health and neuroinflammation. FASEB J. (2016) 30:2662–72. doi: 10.1096/fj.201600435RR
116. Fiorentino M, Sapone A, Senger S, Camhi SS, Kadzielski SM, Buie TM, et al. Blood-brain barrier and intestinal epithelial barrier alterations in autism spectrum disorders. Mol Autism. (2016) 7:49. doi: 10.1186/s13229-016-0110-z
117. Kerrisk ME, Cingolani LA, Koleske AJ. ECM receptors in neuronal structure, synaptic plasticity, and behavior. Prog Brain Res. (2014) 214:101–31. doi: 10.1016/B978-0-444-63486-3.00005-0
118. Misra JR, Irvine KD. The hippo signaling network and its biological functions. Annu Rev Genet. (2018) 52:65–87. doi: 10.1146/annurev-genet-120417-031621
119. Yang C-C, Graves HK, Moya IM, Tao C, Hamaratoglu F, Gladden AB, et al. Differential regulation of the Hippo pathway by adherens junctions and apical-basal cell polarity modules. Proc Natl Acad Sci USA. (2015) 112:1785–90. doi: 10.1073/pnas.1420850112
120. Ma S, Meng Z, Chen R, Guan KL. The hippo pathway: biology and pathophysiology. Annu Rev Biochem. (2019) 88:577–604. doi: 10.1146/annurev-biochem-013118-111829
121. Liu B, Zheng Y, Yin F, Yu J, Silverman N, Pan D. Toll receptor-mediated hippo signaling controls innate immunity in drosophila. Cell. (2016) 164:406–19. doi: 10.1016/j.cell.2015.12.029
122. López M, Varela L, Vázquez MJ, Rodríguez-Cuenca S, González CR, Velagapudi VR, et al. Hypothalamic AMPK and fatty acid metabolism mediate thyroid regulation of energy balance. Nat Med. (2010) 16:1001–8. doi: 10.1038/nm.2207
123. Rotem RS, Chodick G, Shalev V, Davidovitch M, Koren G, Hauser R, et al. Maternal thyroid disorders and risk of autism spectrum disorder in progeny. Epidemiology. (2020) 31:409–17. doi: 10.1097/EDE.0000000000001174
124. Martinez ME, Duarte CW, Stohn JP, Karaczyn A, Wu ZF, DeMambro VE, et al. Thyroid hormone influences brain gene expression programs and behaviors in later generations by altering germ line epigenetic information. Mol Psychiatry. (2020) 25:939–50. doi: 10.1038/s41380-018-0281-4
125. Ames JL, Windham GC, Lyall K, Pearl M, Kharrazi M, Yoshida CK, et al. Neonatal thyroid stimulating hormone and subsequent diagnosis of autism spectrum disorders and intellectual disability. Autism Res. (2020) 13:444–55. doi: 10.1002/aur.2247
126. Fuziwara CS, Kimura ET. MicroRNAs in thyroid development, function and tumorigenesis. Mol Cell Endocrinol. (2017) 456:44–50. doi: 10.1016/j.mce.2016.12.017
127. Crider A, Pillai A. Estrogen signaling as a therapeutic target in neurodevelopmental disorders. J Pharmacol Exp Ther. (2017) 360:48–58. doi: 10.1124/jpet.116.237412
128. Crider A, Thakkar R, Ahmed AO, Pillai A. Dysregulation of estrogen receptor beta (ERβ), aromatase (CYP19A1), and ER co-activators in the middle frontal gyrus of autism spectrum disorder subjects. Mol Autism. (2014) 5:46. doi: 10.1186/2040-2392-5-46
129. Baron-Cohen S, Tsompanidis A, Auyeung B, Nørgaard-Pedersen B, Hougaard DM, Abdallah M, et al. Foetal oestrogens and autism. Mol Psychiatry. (2020) 25:2970–8. doi: 10.1038/s41380-019-0454-9
130. Logan RW, McClung CA. Rhythms of life: circadian disruption and brain disorders across the lifespan. Nat Rev Neurosci. (2019) 20:49–65. doi: 10.1038/s41583-018-0088-y
131. Yavuz-Kodat E, Reynaud E, Geoffray M-M, Limousin N, Franco P, Bonnet-Brilhault F, et al. Disturbances of continuous sleep and circadian rhythms account for behavioral difficulties in children with autism spectrum disorder. J Clin Med. (2020) 9:1978. doi: 10.3390/jcm9061978
132. Khanzada NS, Butler MG, Manzardo AM. Gene analytics pathway analysis and genetic overlap among autism spectrum disorder, bipolar disorder and schizophrenia. Int J Mol Sci. (2017) 18:527. doi: 10.3390/ijms18030527
133. Carmassi C, Palagini L, Caruso D, Masci I, Nobili L, Vita A, et al. Systematic review of sleep disturbances and circadian sleep desynchronization in autism spectrum disorder: toward an integrative model of a self-reinforcing loop. Front Psychiatry. (2019) 10:366. doi: 10.3389/fpsyt.2019.00366
Keywords: systematic review, microRNA, autism spectrum disorder, gene, signaling pathway, bioinformatic analysis
Citation: Huang Z-X, Chen Y, Guo H-R and Chen G-F (2021) Systematic Review and Bioinformatic Analysis of microRNA Expression in Autism Spectrum Disorder Identifies Pathways Associated With Cancer, Metabolism, Cell Signaling, and Cell Adhesion. Front. Psychiatry 12:630876. doi: 10.3389/fpsyt.2021.630876
Received: 18 November 2020; Accepted: 31 August 2021;
Published: 21 October 2021.
Edited by:
Rebecca Ann Muhle, Columbia University, United StatesReviewed by:
Richard Eugene Frye, Phoenix Children's Hospital, United StatesJeffrey C. Glennon, University College Dublin, Ireland
Copyright © 2021 Huang, Chen, Guo and Chen. This is an open-access article distributed under the terms of the Creative Commons Attribution License (CC BY). The use, distribution or reproduction in other forums is permitted, provided the original author(s) and the copyright owner(s) are credited and that the original publication in this journal is cited, in accordance with accepted academic practice. No use, distribution or reproduction is permitted which does not comply with these terms.
*Correspondence: Yanhui Chen, yanhui_0655@126.com