- 1Mental Health Center, West China Hospital, Sichuan University, Chengdu, China
- 2West China Biomedical Big Data Center, West China Hospital, Sichuan University, Chengdu, China
- 3Wuyuzhang Honors College, Sichuan University, Chengdu, China
Background: Patients with major depressive disorder (MDD) often present with co-occurring anxiety symptoms. The network method provides a novel view on understanding the co-occurrence of depressive and anxiety symptoms. Thus, the purpose of our study was to explore it by applying network analysis methods.
Methods: We used electronic medical records from West China Hospital in China. In total, 3,424 patients who met the criteria for MDD were included. R-studio 3.6 was used to estimate the network structure. First, we estimated the network structure of depression and anxiety symptoms using the graphic LASSO algorithm. Then, we estimated the centrality indices of nodes to determine which symptoms are more central in the network. We then estimated the bridge centrality indices using the bridge function via the R package networktools.
Results: Some strong connections were found like “easy to wake up,” “wake up early,” and “difficulty falling asleep,” “suicidal thoughts,” and “hopelessness.” “Depressed mood,” “somatic anxiety,” “hopelessness,” “anxiety mood,” and “tension” have the higher centrality indices. Results revealed eight bridge symptoms (e.g., concentration/memory difficulty, gastrointestinal symptoms) in the co-occurrence network structure.
Conclusions: This research suggests that the described approach in mapping the presence of anxiety symptoms in individuals with major depression might potentially increase diagnostic precision and help choose more targeted interventions and potentially reduce the occurrence of treatment resistance.
Introduction
Major depressive disorder (MDD) is a debilitating psychiatric condition characterized by depressed mood, decreased energy level, and lack of interest in pleasurable activities (1). It has a significant burden on both individuals and society and is related to psychological impairments and health dysfunction. Among Chinese adults, MDD has a 12-month and lifetime prevalence rate of 3.6 and 6.9%, respectively (2). Gaspersz's study showed that 40–60% of patients with MDD also have anxiety symptoms (3). Another survey suggested that 50% of individuals with MDD meet the diagnostic criteria for anxiety disorders (4, 5).
The co-occurrence anxiety symptoms among patients with MDD have important clinical implications. Firstly, the co-occurrence anxiety symptoms predict a more chronic course and more severe disease progression (6). Secondly, compared with individuals who have MDD without anxiety symptoms, patients influenced by anxiety and depressive symptoms have shown greater functional disability, poorer quality of life, and higher risk of suicide behavior (7, 8). Thirdly, MDD with prominent coexisting anxiety symptoms is more difficult to treat than MDD without anxiety symptoms (9). Fourth, anxiety symptoms are associated with occupation of more health care resources among patients with MDD (10). Previous studies either concentrated on the count symptoms to clarify a diagnosis and calculate the prevalence rate of comorbidity or regarded the symptoms as indicators of latent dimensions, however, the between-symptom links are considered a byproduct of dimensional community (11).
Network theory provides us a novel perspective of mental disorders. Network analysis conceptualizes symptoms as constituents of mental disorders, as compared to traditional methods that assume an underlying disease in advance as the common cause of symptoms. Recently, network analysis models are rapidly growing, not only concerning methodological issues but also in offering an appealing interpretation of psychopathology (12, 13).
From the perceptive of topology, network structure consists of nodes(symptoms) and edges(association between symptoms) (14). The importance of nodes was evaluated via centrality indices. Edges represent the links between pairs of symptoms and thicker edges denote larger correlations (15). Specifically, centrality indices include strength, closeness, and betweenness that allow clinicians to discern the symptoms with the greatest importance in the network structure. Those with high centrality indices convey more clinical information (16, 17). Strength is one of the most commonly used centrality indices as it is easy to interpret and is the most stable centrality index (18). In the opinion of network theory, comorbidity is regarded as a constellation of symptom-level relationships (19). Symptoms that link two mental disorders are regarded as “bridge symptom.” Bridge symptoms indicated by bridge centrality indices mainly included bridge strength, bridge closeness, and bridge betweenness (20). Bridge symptoms may have an important role in the development and maintenance of co-occurring mental disorder (21). Thus, when one mental disorder presents, intervention on potential bridge symptoms may contribute to preventing co-occurrence (20). For example, if we suppose that sleep disturbance is a bridge symptom between depression and anxiety, then patients who suffer poor sleep quality as one of their MDD symptoms would be at greater risk for anxiety compared to those without sleep disturbance. Thus, it would be wise for psychiatrists to treat these bridge symptoms therapeutically to reduce the co-occurrence.
At present, 10 studies utilized network analysis to explore comorbidity and co-occurrence in depression (22–31). Previous studies have explored the comorbidity between anxiety and depression (23), posttraumatic stress disorder and co-morbidity depressive symptoms (31), and comorbidity between obsessive-compulsive disorder and depression (26). While previous network researches in MDD have broadened our knowledge of the field, several limitations remain. Firstly, most studies use self-reported questionnaires (e.g., Patient Health Questionnaire-9 and General Anxiety Disorder-7) to assess symptoms among the general population (29, 30), while self-report questionnaires have a risk for response biases (32). Few studies used a sample that comprised treatment-seeking patients with MDD (23). Secondly, while sample size plays an important role in establishing a reliable network structure, most studies used a small sample size that ranges from 296 (25) to 1,029 (23, 33). Thirdly, no studies to date have explored the co-occurrence between MDD and anxiety symptoms based on real-world evidence. Real-word evidence is important for establishing the network structure of co-occurrence between MDD and anxiety symptoms. In this regard, electronic medical records (EMRs) bring new chances in clinical research, providing the potential chance for low-cost and high-volume data on clinical research (34).
The main aim of this study, which was based on the retrospective of EMRs, was to establish the co-occurring network structure between MDD and anxiety symptoms to identify the bridge symptoms.
Methods
Study Population
This was a retrospective study based on pre-existing data derived from EMRs undertaken at West China Hospital. Eligible patients were those with a diagnosis of MDD, aged between 18 and 65. In this study, we identified MDD patients through recorded primary diagnosis at discharge and based on the International Classification of Disease, Tenth Revision (Clinical Modification Codes F32, single episode major depressive disorder and F33, recurrent major depressive disorder), which has been described in another study (35). We extracted anonymous clinical-related information. Exclusion criteria are the following 3 items. Patients' follow-up discharge diagnosis code is F30 or F31. Patients with MDD did not accomplish Hamilton Depression Scale-24 (HAMD-24) and Hamilton Anxiety Scale-14 (HAMA-14) at admission. ③ Age ≤ 18 or age > 65. The details are shown in Figure 1. The data used to support the findings of this manuscript are restricted by the West China Hospital in order to protect patient privacy and avoid legal and ethical risks. Data are available from the West China Hospital for researchers who meet the criteria for access to confidential data (data.cd120.com).
We obtained approval from the Ethics Committee of the West China Hospital, Sichuan University (2017 N0.185). As this is a retrospective study, Institute Review Board (IRB) waived the requirement for obtaining informed consent from the individual patients. We did not use information on the identity of patients and all related information was kept confidential. All procedures were in accordance with the ethical standards of the Ethics Committee and the revised Helsinki Declaration of 2008 (36, 37).
Measures
HAMD-24 is one of the most widely used scales in clinical practical assessment of depression. It is assessed by psychiatrists and takes about 15 to 20 min to finish (38). HAMA-14 is one of the most widely used scales in the clinical practical assessment of anxiety. HAMA-14 includes 14 items with each item divided into 5 levels from 0 to 4 (39). The Chinese versions of HAMD-24 and HAMA-14 have good reliability and validity (40).
Data Analysis
All analysis was accomplished using the R-3.6 studio. In this research, the missing data belong to the category of missing at random (MAR). So, we used the method of unconditional mean imputation to handle the missing data (41). The network structure consists of two elements: nodes and edges. Every node represents a symptom, and each edge demonstrates a relationship between the two symptoms. In our study, the nodes (symptoms) were represented the scale items of HAMD-24 and HAMA-14. We used a graphical Gaussian model to estimate the networks. In addition, we used the least absolute shrinkage and selection operator (LASSO) to regularize our model and used the qgraph package to visualize the network. Next, we computed the centrality indices (i.e., betweenness, strength, and closeness) of nodes to find which symptoms are more central in the network structure. Betweenness and closeness are often not reliably estimated (17). Thus, we only reported the strengths in this article, while other node centrality indices are provided in the Supplementary Material. We assessed the accuracy stability of the centrality using the bootstrap approach in the bootnet package. To gain a stable and interpretable centrality, the CS coefficient should be >0.25 (42). We estimated the stability of edge-weights by bootstrapping the 95% confidence intervals (CIs), where fewer overlaps in the CIs indicate higher stability. Jones uses the term community to demonstrate a theoretically based group of nodes that correspond to a mental disorder according to clinical criteria, instead of according to any network analytic procedure (20). This method contributes to identifying bridge nodes, especially when networks are large, complex, or difficult to account for visually. The networktools package (43) were used to calculate the values and create plots. You can get the code from the Git hub (https://github.com/fenfenge/Network-structure).
Results
Descriptive Statistics
We included 3,424 patients with MDD comprising 2,349 females and 1,075 males, with ages ranging from 18 to 65 (M = 42.5, SD = 13.25). Table 1 shows the items, item content, sample means, standard deviation, and missing items/percentage.
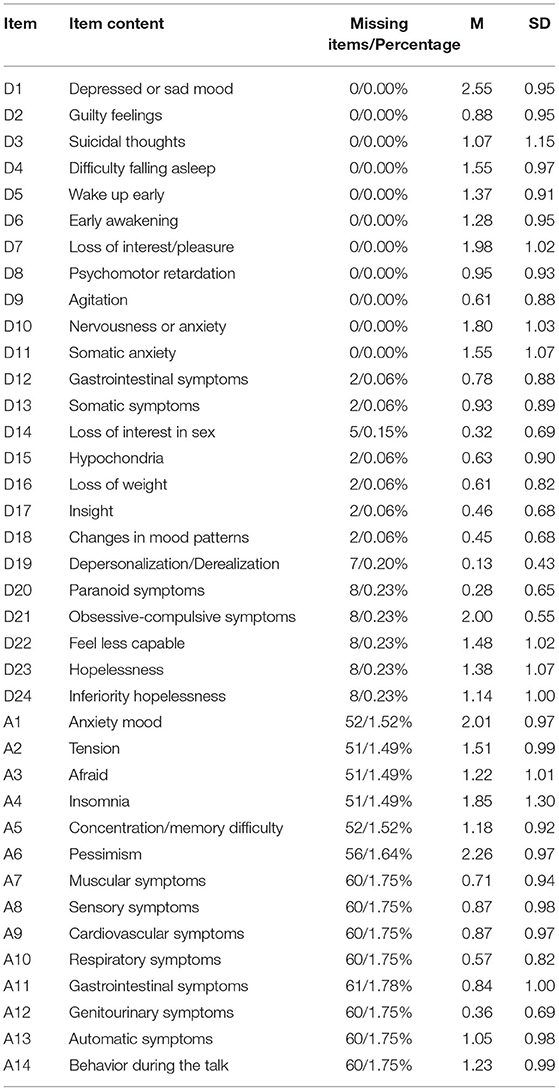
Table 1. Items, item content, missing items, means, and standard deviations for HAMD-24 and HAMD-14.
Network Estimation
The graphical LASSO network is shown in Figure 2. A thicker edge indicates a stronger association between the symptoms. Green edges represent positive regularized partial correlations, and red edges represent negative regularized partial correlations. Network analysis demonstrated that five strong connections edges were among the HAMD-24 items. The top edge was between the items “easy to wake up,” “wake up early,” and “difficulty falling asleep” (D4: D5: D6). Additionally, HAMD-24 items “suicidal thoughts” and “hopelessness” (D3:D23) and “hopelessness” and “inferiority” (D23:D24) were among the strongest. There were two strongest edges between the HAMA-14 items. The strongest edge was between items “tension” and “afraid” (A2:A3), followed by “cardiovascular symptoms” and “respiratory symptoms” (A9:A10).
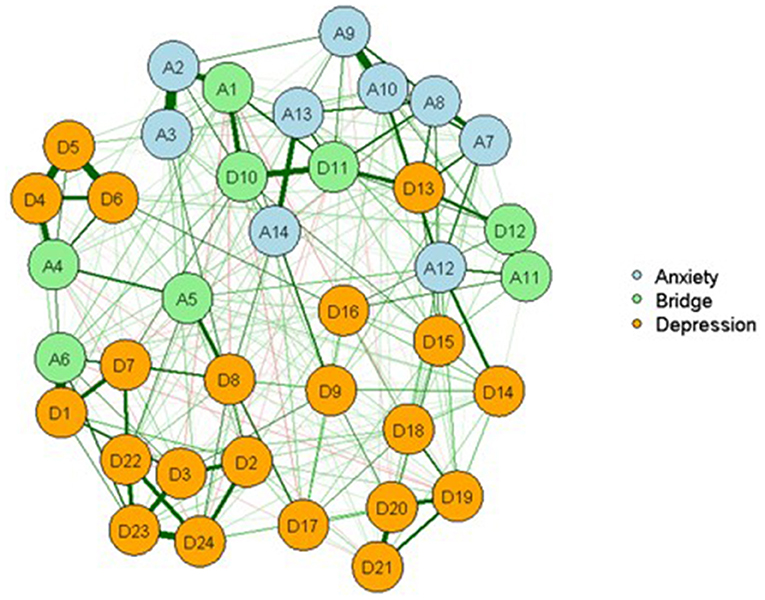
Figure 2. Network of MDD and anxiety symptoms showing bridge symptoms among clinical samples. D1: Depressed or sad mood; D2: Guilty feelings; D3: Suicidal thoughts; D4: Difficulty falling asleep; D5: Easy to wake up; D6: Early awakening; D7: Loss of interest/pleasure; D8: Psychomotor retardation; D9: Agitation; D10: Nervousness or anxiety; D11: Somatic anxiety; D12: Gastrointestinal symptoms; D13: Somatic symptoms; D14: Loss of interest in sex; D15: Hypochondria; D16: Loss of weight; D17: Insight; D18: Changes in mood patterns; D19: Depersonalization/Derealization; D20: Paranoid symptom; D21: Obsessive-compulsive symptom; D22: Feel less capable; D23: Hopelessness; D24: Inferiority hopelessness; A1: Anxiety mood; A2: Tension; A3: Afraid; A4: Insomnia; A5: Concentration/memory difficulty; A6: Pessimism; A7: Muscular symptoms; A8: Sensory symptoms; A9: Cardiovascular symptoms; A10: Respiratory symptoms; A11: Gastrointestinal symptoms; A12: Genitourinary symptoms; A13: Automatic symptoms; A14: Behavior during the talk.
Network Inference and Stability
Strength centrality is shown in Figure 3. Firstly, nodes demonstrating “depressed or sad mood” (D1), “somatic anxiety” (D11), and “hopelessness” (D23) were among the MDD symptoms exhibiting higher levels of strength. Secondly, “anxiety mood” (A1) and “tension” (A2) were among the anxiety symptoms exhibiting higher levels of strength. In terms of stability of network analysis, bootstrap 95% CI demonstrated moderate stability for the strength index (Supplementary Materials).
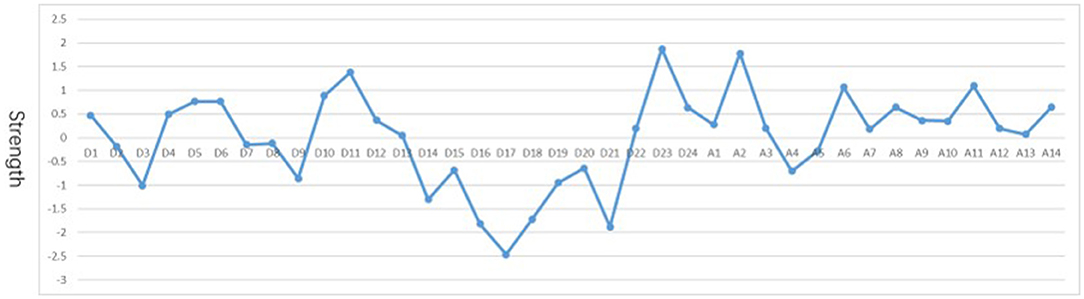
Figure 3. Plot of standardized centrality indices for network. D1: Depressed or sad mood; D2: Guilty feelings; D3: Suicidal thoughts; D4: Difficulty falling asleep; D5: Easy to wake up; D6: Early awakening; D7: Loss of interest/pleasure; D8: Psychomotor retardation; D9: Agitation; D10: Nervousness or anxiety; D11: Somatic anxiety; D12: Gastrointestinal symptoms; D13: Somatic symptoms; D14: Loss of interest in sex; D15: Hypochondria; D16: Loss of weight; D17: Insight; D18: Changes in mood patterns; D19: Depersonalization/Derealization; D20: Paranoid symptom; D21: Obsessive-compulsive symptom; D22: Feel less capable; D23: Hopelessness; D24: Inferiority hopelessness; A1: Anxiety mood; A2: Tension; A3: Afraid; A4: Insomnia; A5: Concentration/memory difficulty; A6: Pessimism; A7: Muscular symptoms; A8: Sensory symptoms; A9: Cardiovascular symptoms; A10: Respiratory symptoms; A11: Gastrointestinal symptoms; A12: Genitourinary symptoms; A13: Automatic symptoms; A14: Behavior during the talk.
Bridge strength is shown in Figure 4. Nodes demonstrating “anxiety mood” (A1), “insomnia” (A4), “concentration/memory difficulty” (A5), “pessimism” (A6), and “gastrointestinal symptoms” (A11) were anxiety symptoms displaying higher levels of bridge strength on all MDD symptoms. The MDD symptoms “nervousness or anxiety” (D10), “somatic anxiety” (D11), and “gastrointestinal symptoms” (D12) exert a strong bridging effect on anxiety symptoms.
Discussion
Many patients with MDD also experience anxiety symptoms. As far as we know, this is the first research to explore network connectivity among treat-seeking patients with MDD and co-occurrence anxiety symptoms based on real-world evidence. Perhaps the most prominent result was that not all nodes(symptoms) were equally important in the network with co-occurrence anxiety symptoms among patients with MDD (44, 45). Besides, we also found some strong linkage between symptoms, such as “easy to wake up” (D4), “wake up early” (D5), and “difficulty falling asleep” (D6), which were closely associated with each other.
Moreover, “depressed or sad mood” (D1) and “hopelessness” (D23) have the highest centrality in the network structure. Such results do not come as a surprise; previous studies that used self-report questionnaires and composite international diagnostic interviews also suggested that sad mood and hopelessness carry more weight than other symptoms of MDD (14, 46–48). “Somatic anxiety” (D11) had one of the highest centrality indices, making it a hallmark symptom of MDD among Chinese. “Somatic anxiety” is involved via overt signs of excessive autonomic activity and/or skeletal/muscle-motor tension. Due to the following three reasons that including (1) emotional symptoms are stigmatized, (2) thinking deviated far from internal experiences, (3)a special perspective of the self is emphasized, (49) somatization has been a general symptom in the Chinese population (50, 51). It is worthy that higher somatization was related to poorer performance in the cognitive and poorer antidepressant treatment clinical outcome (52). Anxiety symptoms (e.g., anxiety mood and tension) also had higher centrality. Goghari's 2-year longitudinal study found that patients with MDD reported higher levels of anxiety than those with other mental disorders. To some extent, easing the patient's anxiety level helps improve the outcome and daily function of depression (53).
Network theory helps to understand the co-occurrence of psychopathology. We could reduce co-occurrence by effectively “burning the bridge symptoms” between disorders (45). In this study, the MDD nodes that displayed the strongest association with anxiety symptoms were “nervousness” (D10), “somatic anxiety” (D11), and “gastrointestinal symptoms” (D12). Conversely, the anxiety nodes that displayed the strongest connection with MDD symptoms were “anxiety mood” (A1), “insomnia” (A4), “concentration/memory difficulty” (A5), “pessimism” (A6), and “gastrointestinal symptoms” (A11). Gastrointestinal symptoms were bridge symptoms that link MDD to anxiety symptoms and similarly linked anxiety symptoms to MDD. Gastrointestinal symptoms are common features for both MDD and anxiety disorders. They are also prominent features in posttraumatic stress disorder, schizophrenia, and autism spectrum disorder (54–56), demonstrating that gastrointestinal symptoms may be a common feature associated with a multitude of mental disorders. Simpson's review indicated that the role of gut microbiota in mood regulation and emotional processing may be of particular relevance to depression and anxiety etiology (57). In this study, concentration/memory difficulty was a bridge symptom that linked anxiety to MDD. Pettit's research found that computer-based attention training can reduce anxiety symptoms among the youth (58). These results suggested that attention training could be used to ease anxiety symptoms among MDD patients.
We found a strong connection between “easy to wake up,” “wake up early,” and “hard to fall asleep” (D4: D5: D6). Interestingly, the three symptoms above belong to the diagnostic criteria for insomnia (59) and MDD patients with sleep complaints are prone to more severe symptoms (60). Thus, insomnia is a valuable therapeutic target in MDD patients. The theoretical promise of network analysis is that psychiatric symptoms are not isolated and may accentuate each other (61). Symptoms may reinforce one another via creating a feedback loop, such as, “inferior helplessness” (D24) could lead to “hopelessness” (D23), and at the same time prompt “suicidal thoughts” (D3). These links may contribute to the build of a self-sustained symptom constellation. Thus, it is necessary to find potential self-sustaining mechanisms and timely interventions for feedback loops (13). Somatic symptoms (e.g., cardiovascular symptoms and respiratory symptoms) were closely connected. This can be explained by the mind-body interaction model, which states that information flows not only from the body to the brain but also from the brain to the body. In the brain, “prediction” is derived from metacognition (conceptual knowledge), namely higher-order thoughts and cognition, which helps estimate the generation of behavioral commands (62).
Clinical Implication
The network model provides a novel view to investigate the potential mechanisms underlying the etiology and maintenance of mental disorders. We can understand how the symptoms are interrelated via networks and how to intervene on comorbidities. Firstly, when depressive patients are admitted to the hospital, psychiatrists should prioritize the evaluation of identified bridging MDD symptoms to screen patients with a higher risk of suffering anxiety symptoms. Secondly, from the viewpoint of network structure, we can intervene mental disorders from the following aspects: (1) symptoms (nodes) interventions: direct intervention of one or more symptoms; (2) network interventions: intervention symptom-symptom connections (61); (3) bridge symptoms: intervention of bridge symptoms to prevent co-occurrence. For example, we can intervene on closely connected sleep symptoms to avoid using certain antidepressants that may cause or even worsen sleep problems (63), since improving sleep contributes to improving the outcomes of MDD (64, 65). Our findings may be used to demonstrate which symptoms (e.g., gastrointestinal symptoms, insomnia) drive the association, and which should be handled first to reduce MDD and anxiety co-occurrence. These findings emphasize the importance of assessing anxiety symptoms among patients with MDD. Owing to the limitations of earlier versions of the Diagnostic and Statistical Manual of Mental Disorder (DSM) on grading targeting rules, anxiety in mental disorders has been underestimated, underdiagnosed, and undertreated. CBT is very effective for the treatment of anxiety symptoms and sleep disturbance (66). Thus, patients with MDD can be treated with suitable medicines and CBT.
Strengths and Limitations
This research has several key strengths. First, we employed a sample comprised of treatment-seeking patients diagnosed with MDD according to the ICD-10. Moreover, HAMD and HAMA are evaluated by psychiatrists; thus, ambiguous/vague answers to the self-assessment scale were avoided. Second, we used a relatively large sample that contributed to establishing a reliable network structure with robust edge weights and centrality (42). To date, only one study has used large psychiatric samples of over 1,000 participants (23).
Despite the strengths, some limitations should be considered in this study. Firstly, this is a cross-sectional study, and we cannot explore changes in the co-occurrence network structure over time. Specifically, we are not sure whether the connections between symptoms appear temporarily or continuously, and what will happen to those strong connections under the intervention of strategy. Considering that network modes require the estimation of many parameters and these models need power to reliably detect small coefficients, exploration of larger data sets is necessary (67, 68). Thus, in this study, we do not compare the network structure in different severity of subgroup. Secondly, in our study, the target population was patients with MDD diagnosis so that the results could be generalized to the whole population. Specifically, the results do not suit individuals who suffer from certain depression symptoms, while failing to meet the diagnostic criteria for MDD. Third, Gureje's study showed that the presentation of mental disorders is influenced by culture and social milieu (69), while we only focused on Chinese patients. Future studies should consider the cross-cultural variation and explore how culture influences the presentation of mental disorders. Finally, we included patients with ICD-10 F32 and F33 diagnoses only. We did not check whether the patients had a comorbidity diagnosis with an anxiety disorder or any other co-morbidity. However, Wise's study (70) may support the notion that comorbidities might not be so important, at least concerning functional connectivity. Specifically, Wise's research supports the notion that biological abnormalities in functional connectivity in major depression across independent samples might overlap irrespective of the presence of anxiety comorbidities.
Conclusion
This research is the first to explore the association between depressive and anxiety symptoms among MDD patients based on EMRs, thus offering an essential basis on how the two disorders co-vary. We found that some high central symptoms (e.g., hopelessness, somatic anxiety, and tension) and some bridge symptoms (e.g., concentration/memory difficulty, pessimism, and gastrointestinal symptoms). We summarize the evidence from the current research that treatment for co-occurring anxiety symptoms among MDD patients at symptom level may be efficacious. Multiple interventions, such as improving sleep, CBT, or attention training, could be applied to address these co-occurring symptoms.
Data Availability Statement
The data analyzed in this study is subject to the following licenses/restrictions: The dataset belong to the West China Hospital, Sichuan University. Requests to access these datasets should be directed to Jingwen Jiang, jiangjingwen@wchscu.cn.
Ethics Statement
The studies involving human participants were reviewed and approved by Ethics Committee of the West China Hospital, Sichuan University. The ethics committee waived the requirement of written informed consent for participation.
Author Contributions
FG participated in the study design, data analysis, interpretation of findings, literature search, writing, implementation, and approval of the final manuscript. WZ conceived and designed the study. JJ, YW, and MW participated in the study data analysis. All authors have approved the final manuscript.
Funding
This study was financially supported by The Special Project on Natural Chronic Non-infectious Diseases (grant no. 2016YFC1307201).
Conflict of Interest
The authors declare that the research was conducted in the absence of any commercial or financial relationships that could be construed as a potential conflict of interest.
Supplementary Material
The Supplementary Material for this article can be found online at: https://www.frontiersin.org/articles/10.3389/fpsyt.2021.595418/full#supplementary-material
References
2. Huang Y, Wang Y, Wang H, Liu Z, Yu X, Yan J, et al. Prevalence of mental disorders in China: a cross-sectional epidemiological study. Lancet Psychiatry. (2019) 6:211–24. doi: 10.1016/s2215-0366(18)30511-x
3. Gaspersz R, Lamers F, Kent JM, Beekman AT, Smit JH, van Hemert AM, et al. Longitudinal predictive validity of the DSM-5 anxious distress specifier for clinical outcomes in a large cohort of patients with major depressive disorder. J Clin Psychiatry. (2017) 78:207–13. doi: 10.4088/JCP.15m10221
4. Nutt D. Management of patients with depression associated with anxiety symptoms. J Clin Psychiatry. (1997) 58(Suppl. 8):11–6.
5. Kessler RC, Berglund P, Demler O, Jin R, Koretz D, Merikangas KR, et al. The epidemiology of major depressive disorder: results from the National Comorbidity Survey Replication (NCS-R). JAMA. (2003) 289:3095–105. doi: 10.1001/jama.289.23.3095
6. Goldberg D, Fawcett J. The importance of anxiety in both major depression and bipolar disorder. Depress Anxiety. (2012) 29:471–8. doi: 10.1002/da.21939
7. Pfeiffer PN, Ganoczy D, Ilgen M, Zivin K, Valenstein M. Comorbid anxiety as a suicide risk factor among depressed veterans. Depress Anxiety. (2009) 26:752–7. doi: 10.1002/da.20583
8. Zimmerman M, Chelminski I, Young D, Dalrymple K, Walsh E, Rosenstein L. A clinically useful self-report measure of the DSM-5 anxious distress specifier for major depressive disorder. J Clin Psychiatry. (2014) 75:601–7. doi: 10.4088/JCP.13m08961
9. Gaspersz R, Lamers F, Kent JM, Beekman ATF, Smit JH, van Hemert AM, et al. Anxious distress predicts subsequent treatment outcome and side effects in depressed patients starting antidepressant treatment. J Psychiatr Res. (2017) 84:41–8. doi: 10.1016/j.jpsychires.2016.09.018
10. McLaughlin TP, Khandker RK, Kruzikas DT, Tummala R. Overlap of anxiety and depression in a managed care population: prevalence and association with resource utilization. J Clin Psychiatry. (2006) 67:1187–93. doi: 10.4088/jcp.v67n0803
11. Borsboom D. Psychometric perspectives on diagnostic systems. J Clin Psychiatry. (2008) 64:1089–108. doi: 10.1002/jclp.20503
12. Cramer AO, Waldorp LJ, van der Maas HL, Borsboom D. Comorbidity: a network perspective. Behav Brain Sci. (2010) 33:137–50. doi: 10.1017/s0140525x09991567
13. Fried EI, van Borkulo CD, Cramer AO, Boschloo L, Schoevers RA, Borsboom D. Mental disorders as networks of problems: a review of recent insights. Soc Psychiatry Psychiatr Epidemiol. (2017) 52:1–10. doi: 10.1007/s00127-016-1319-z
14. Boschloo L, van Borkulo CD, Borsboom D, Schoevers RA. A prospective study on how symptoms in a network predict the onset of depression. Psychother Psychosom. (2016) 85:183–4. doi: 10.1159/000442001
15. McNally RJ, Robinaugh DJ, Wu GWY, Wang L, Deserno MK, Borsboom D. Mental disorders as causal systems: a network approach to posttraumatic stress disorder. Clin Psychol Sci. (2014) 3:836–49. doi: 10.1177/2167702614553230
16. Fava GA, Fau RC, Tomba E, Tomba E. The clinical process in psychiatry: a clinimetric approach. J Clin Psychiatry. (2012) 73:177–84. doi: 10.4088/JCP.10r06444
17. Bringmann LA-O, Elmer T, Epskamp S, Krause RW, Schoch DA-O, Wichers M, et al. What do centrality measures measure in psychological networks? J Abnorm Psychol. (2019) 128:892–903. doi: 10.1037/abn0000446
18. Phillips RD, Wilson SM, Sun D, Morey R. Posttraumatic stress disorder symptom network analysis in U.S. military veterans: examining the impact of combat exposure. Front Psychiatry. (2018) 9:608. doi: 10.3389/fpsyt.2018.00608
19. Borsboom D, Epskamp S, Kievit RA, Cramer AO, Schmittmann VD. Transdiagnostic networks: commentary on Nolen-Hoeksema and Watkins (2011). Perspect Psychol Sci. (2011) 6:610–4. doi: 10.1177/1745691611425012
20. Jones PJ, Ma R, McNally RJ. Bridge centrality: a network approach to understanding comorbidity. Multivariate Behav Res. (2019) 1–15. doi: 10.1080/00273171.2019.1614898. [Epub ahead of print].
21. Cramer AOJ, Waldorp LJ, van der Maas HLJ, Borsboom D. Complex realities require complex theories: refining and extending the network approach to mental disorders. Behav Brain Sci. (2010) 33:178–93. doi: 10.1017/S0140525X10000920
22. Kozel D, Marusić A. Individuals with diabetes mellitus with and without depressive symptoms: could social network explain the comorbidity? Psychiatr Danub. (2006) 18:12–8.
23. Beard C, Millner AJ, Forgeard MJ, Fried EI, Hsu KJ, Treadway MT, et al. Network analysis of depression and anxiety symptom relationships in a psychiatric sample. Psychol Med. (2016) 46:3359–69. doi: 10.1017/s0033291716002300
24. Afzali MH, Sunderland M, Teesson M, Carragher N, Mills K, Slade T. A network approach to the comorbidity between posttraumatic stress disorder and major depressive disorder: the role of overlapping symptoms. J Affect Disord. (2017) 208:490–6. doi: 10.1016/j.jad.2016.10.037
25. Choi KW, Batchelder AW, Ehlinger PP, Safren SA, O'Cleirigh C. Applying network analysis to psychological comorbidity and health behavior: depression, PTSD, and sexual risk in sexual minority men with trauma histories. J Consult Clin Psychol. (2017) 85:1158–70. doi: 10.1037/ccp0000241
26. McNally RJ, Mair P, Mugno BL, Riemann BC. Co-morbid obsessive-compulsive disorder and depression: a Bayesian network approach. Psychol Med. (2017) 47:1204–14. doi: 10.1017/s0033291716003287
27. Boschloo L. Zooming in and zooming out: a network perspective on the comorbidity of depression and anxiety. J Am Acad Child Adolesc Psychiatry. (2018) 57:914–5. doi: 10.1016/j.jaac.2018.07.880
28. Zanirati G, Azevedo PN, Venturin GT, Greggio S, Alcará AM, Zimmer ER, et al. Depression comorbidity in epileptic rats is related to brain glucose hypometabolism and hypersynchronicity in the metabolic network architecture. Epilepsia. (2018) 59:923–34. doi: 10.1111/epi.14057
29. Garabiles MR, Lao CK, Xiong Y, Hall BJ. Exploring comorbidity between anxiety and depression among migrant Filipino domestic workers: a network approach. J Affect Disord. (2019) 250:85–93. doi: 10.1016/j.jad.2019.02.062
30. Price M, Legrand AC, Brier ZMF, Hébert-Dufresne L. The symptoms at the center: examining the comorbidity of posttraumatic stress disorder, generalized anxiety disorder, and depression with network analysis. J Psychiatr Res. (2019) 109:52–8. doi: 10.1016/j.jpsychires.2018.11.016
31. Lazarov A, Suarez-Jimenez B, Levi O, Coppersmith DDL, Lubin G, Pine DS, et al. Symptom structure of PTSD and co-morbid depressive symptoms - a network analysis of combat veteran patients. Psychol Med. (2020) 50:2154–70. doi: 10.1017/s0033291719002034
32. Artino AR Jr, Phillips AW, Utrankar A, Ta AQ, Durning SJ. “The Questions Shape the Answers”: assessing the quality of published survey instruments in health professions education research. Acad Med. (2018) 93:456–63. doi: 10.1097/acm.0000000000002002
33. Epskamp S, Fried EI. A tutorial on regularized partial correlation networks. Psychol Methods. (2018) 23:617–34. doi: 10.1037/met0000167
34. Berger ML, Sox H, Willke RJ, Brixner DL, Eichler HG, Goettsch W, et al. Good practices for real-world data studies of treatment and/or comparative effectiveness: recommendations from the Joint ISPOR-ISPE Special Task Force on real-world evidence in health care decision making. Value Health. (2017) 20:1003–8. doi: 10.1016/j.jval.2017.08.3019
35. Ge F, Jiang J, Wang Y, Yuan C, Zhang W. Identifying suicidal ideation among Chinese patients with major depressive disorder: evidence from a real-world hospital-based study in China. Neuropsychiatr Dis Treat. (2020) 16:665–72. doi: 10.2147/ndt.s238286
36. World Medical Association declaration of Helsinki. Recommendations guiding physicians in biomedical research involving human subjects. JAMA. (1997) 277:925–6.
37. World Medical Association declaration of Helsinki. Recommendations guiding physicians in biomedical research involving human subjects. J Med Liban. (1994) 42:88–9.
38. Hamilton M. A rating scale for depression. J Neurol Neurosurg Psychiatry. (1960) 23:56–62. doi: 10.1136/jnnp.23.1.56
40. Yan M. XS, Hu M. The Chinese translation, reliability and validity of some depression scales in China. Chinese Mental Health J. (2016) 30:501–5. doi: 10.3969/j.issn.1000-6729.2016.07.005
41. Donders AR, van der Heijden GJ, Stijnen T, Moons KG. Review: a gentle introduction to imputation of missing values. J Clin Epidemiol. (2006) 59:1087–91. doi: 10.1016/j.jclinepi.2006.01.014
42. Epskamp S, Borsboom D, Fried EI. Estimating psychological networks and their accuracy: a tutorial paper. Behav Res Methods. (2018) 50:195–212. doi: 10.3758/s13428-017-0862-1
43. Jones PJ. Networktools: Tools for Identifying Important Nodes in Networks. R Package Version 1.1.0 (2017). Available online at: https://CRAN.R-project.org/package=networktools
44. Knefel M, Tran US, Lueger-Schuster B. The association of posttraumatic stress disorder, complex posttraumatic stress disorder, and borderline personality disorder from a network analytical perspective. J Anxiety Disord. (2016) 43:70–8. doi: 10.1016/j.janxdis.2016.09.002
45. Jones PJ, Mair P, Riemann BC, Mugno BL, McNally RJ. A network perspective on comorbid depression in adolescents with obsessive-compulsive disorder. J Anxiety Disord. (2018) 53:1–8. doi: 10.1016/j.janxdis.2017.09.008
46. Fried EI, Epskamp S, Nesse RM, Tuerlinckx F, Borsboom D. What are 'good' depression symptoms? Comparing the centrality of DSM and non-DSM symptoms of depression in a network analysis. J Affect Disord. (2016) 189:314–20. doi: 10.1016/j.jad.2015.09.005
47. Boschloo L, Schoevers RA, van Borkulo CD, Borsboom D, Oldehinkel AJ. The network structure of psychopathology in a community sample of preadolescents. J Abnorm Psychol. (2016) 125:599–606. doi: 10.1037/abn0000150
48. Cramer AOJ, van der Sluis S, Noordhof A, Wichers M, Geschwind N, Aggen SH, et al. Dimensions of normal personality as networks in search of equilibrium: you can't like parties if you don't like people. Eur J Pers. (2012) 26:414–31. doi: 10.1002/per.1866
49. Ryder AG. Cross-cultural Differences in the Presentation of Depression: Chinese Somatization and Western Psychologization. Vancouver, BC: University of British Columbia (2004).
50. Zhou X, Dere J, Zhu X, Yao S, Chentsova-Dutton YE, Ryder AG. Anxiety symptom presentations in Han Chinese and Euro-Canadian outpatients: is distress always somatized in China? J Affect Disord. (2011) 135:111–4. doi: 10.1016/j.jad.2011.06.049
51. Dere J, Sun J, Zhao Y, Persson TJ, Zhu X, Yao S, et al. Beyond “somatization” and “psychologization”: symptom-level variation in depressed Han Chinese and Euro-Canadian outpatients. Front Psychol. (2013) 4:377. doi: 10.3389/fpsyg.2013.00377
52. Braund TA, Palmer DM, Williams LM, Harris AWF. Dimensions of anxiety in Major depressive disorder and their use in predicting antidepressant treatment outcome: an iSPOT-D report. Psychol Med. (2020) 50:1032–42. doi: 10.1017/s0033291719000941
53. Goghari VM, Harrow M. Anxiety symptoms across twenty-years in schizoaffective disorder, bipolar disorder, and major depressive disorder. Psychiatry Res. (2019) 275:310–4. doi: 10.1016/j.psychres.2019.03.050
54. Virtanen T, Eskelinen S, Sailas E, Suvisaari J. Dyspepsia and constipation in patients with schizophrenia spectrum disorders. Nordic J Psychiatry. (2017) 71:48–54. doi: 10.1080/08039488.2016.1217044
55. Wernersson R, Carlsson J. Posttraumatic stress disorder is correlated to irritable bowel syndrome. Ugeskrift Laeger. (2015) 177:1248–52.
56. Holingue C, Newill C, Lee LC, Pasricha PJ, Daniele Fallin M. Gastrointestinal symptoms in autism spectrum disorder: a review of the literature on ascertainment and prevalence. Autism Res. (2018) 11:24–36. doi: 10.1002/aur.1854
57. Simpson CA, Diaz-Arteche C, Eliby D, Schwartz OS, Simmons JG, Cowan CSM. The gut microbiota in anxiety and depression - a systematic review. Clin Psychol Rev. (2020) 83:101943. doi: 10.1016/j.cpr.2020.101943
58. Pettit JW, Bechor M, Rey Y, Vasey MW, Abend R, Pine DS, et al. A randomized controlled trial of attention bias modification treatment in youth with treatment-resistant anxiety disorders. J Am Acad Child Adolesc Psychiatry. (2020) 59:157–65. doi: 10.1016/j.jaac.2019.02.018
59. Medicine AAoS. International Classification of Sleep Disorders, 3rd ed. Darien: American Academy of Sleep Medicine (2014).
60. Hinkelmann K, Moritz S, Botzenhardt J, Muhtz C, Wiedemann K, Kellner M, et al. Changes in cortisol secretion during antidepressive treatment and cognitive improvement in patients with major depression: a longitudinal study. Psychoneuroendocrinology. (2012) 37:685–92. doi: 10.1016/j.psyneuen.2011.08.012
61. Borsboom D. A network theory of mental disorders. World Psychiatry. (2017) 16:5–13. doi: 10.1002/wps.20375
62. Mallorquí-Bagué N, Bulbena A, Pailhez G, Garfinkel SN, Critchley HD. Mind-body interactions in anxiety and somatic symptoms. Harvard Rev Psychiatry. (2016) 24:53–60. doi: 10.1097/hrp.0000000000000085
63. Fang H, Tu S, Sheng J, Shao A. Depression in sleep disturbance: a review on a bidirectional relationship, mechanisms and treatment. J Cell Mol Med. (2019) 23:2324–32. doi: 10.1111/jcmm.14170
64. McCall WV, Blocker JN, D'Agostino R Jr., Kimball J, Boggs N, et al. Treatment of insomnia in depressed insomniacs: effects on health-related quality of life, objective and self-reported sleep, and depression. J Clin Sleep Med. (2010) 6:322–9. doi: 10.5664/jcsm.27872
65. Manber R, Edinger JD, Gress JL, San Pedro-Salcedo MG, Kuo TF, Kalista T. Cognitive behavioral therapy for insomnia enhances depression outcome in patients with comorbid major depressive disorder and insomnia. Sleep. (2008) 31:489–95. doi: 10.1093/sleep/31.4.489
66. Frueh BC, Grubaugh AL, Cusack KJ, Kimble MO, Elhai JD, Knapp RG. Exposure-based cognitive-behavioral treatment of PTSD in adults with schizophrenia or schizoaffective disorder: a pilot study. J Anxiety Disord. (2009) 23:665–75. doi: 10.1016/j.janxdis.2009.02.005
67. Epskamp S, Rhemtulla M, Borsboom D. Generalized network psychometrics: combining network and latent variable models. Psychometrika. (2017) 82:904–27. doi: 10.1007/s11336-017-9557-x
68. Epskamp S, Kruis J, Marsman M. Estimating psychopathological networks: be careful what you wish for. PLoS ONE. (2017) 12:e0179891. doi: 10.1371/journal.pone.0179891
69. Gureje O, Lewis-Fernandez R, Hall BJ, Reed GM. Systematic inclusion of culture-related information in ICD-11. World Psychiatry. (2019) 18:357–8. doi: 10.1002/wps.20676
Keywords: major depressive disorder, anxiety, co-occurrence, network analysis, psychopathology
Citation: Ge F, Jiang J, Wang Y, Wan M and Zhang W (2021) Mapping the Presence of Anxiety Symptoms in Adults With Major Depressive Disorder. Front. Psychiatry 12:595418. doi: 10.3389/fpsyt.2021.595418
Received: 16 August 2020; Accepted: 07 April 2021;
Published: 19 May 2021.
Edited by:
Danilo Arnone, United Arab Emirates University, United Arab EmiratesReviewed by:
Casimiro Cabrera Abreu, Queens University, CanadaYauhen Statsenko, United Arab Emirates University, United Arab Emirates
Copyright © 2021 Ge, Jiang, Wang, Wan and Zhang. This is an open-access article distributed under the terms of the Creative Commons Attribution License (CC BY). The use, distribution or reproduction in other forums is permitted, provided the original author(s) and the copyright owner(s) are credited and that the original publication in this journal is cited, in accordance with accepted academic practice. No use, distribution or reproduction is permitted which does not comply with these terms.
*Correspondence: Wei Zhang, weizhanghx@163.com