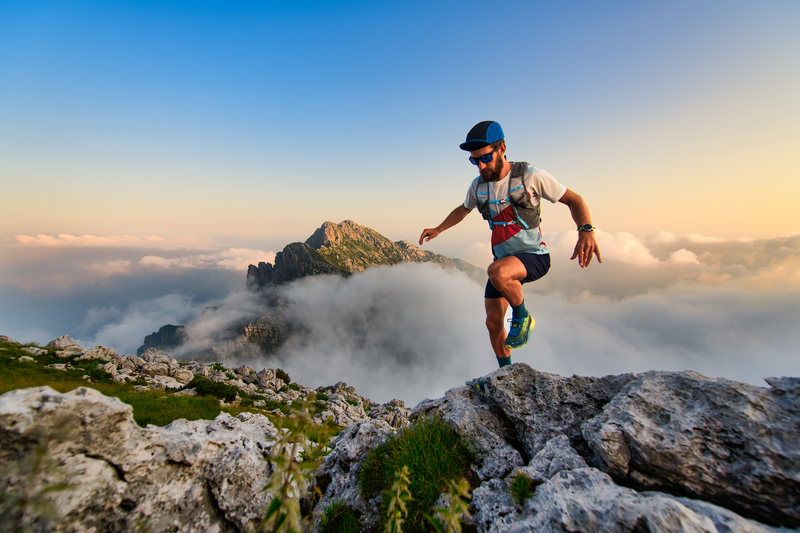
94% of researchers rate our articles as excellent or good
Learn more about the work of our research integrity team to safeguard the quality of each article we publish.
Find out more
ORIGINAL RESEARCH article
Front. Psychiatry , 06 November 2020
Sec. Psychopathology
Volume 11 - 2020 | https://doi.org/10.3389/fpsyt.2020.580503
This article is part of the Research Topic Clinical High Risk for Psychosis: From Epidemiological Findings to Neurobiological Underpinnings of Treatment Response and Outcome View all 5 articles
Introduction: Alterations in autonomic functioning in individuals diagnosed with schizophrenia are well-documented. Yet, it is currently unclear whether these dysfunctions extend into the clinical high-risk state. Thus, we investigated resting heart rate (RHR) and heart rate variability (HRV) indices in individuals at clinical high-risk for psychosis (CHR-P).
Methods: We recruited 117 CHR-P participants, 38 participants with affective disorders and substance abuse (CHR-N) as well as a group of 49 healthy controls. CHR-P status was assessed with the Comprehensive Assessment of At-Risk Mental States (CAARMS) and the Schizophrenia Proneness Instrument, Adult Version (SPI-A). We obtained 5 min, eyes-open resting-state MEG data, which was used for the extraction of cardiac field-related inter-beat-interval data and from which heart-rate and heart-rate variability measures were computed.
Results: Compared to both CHR-N and healthy controls, CHR-P participants were characterized by an increased RHR, which was not explained by differences in psychopathological comorbidity and medication status. Increased RHR correlated with the presence of subthreshold psychotic symptoms and associated distress. No differences between groups were found for heart-rate variability measures, however. Furthermore, there was an association between motor-performance and psychophysiological measures.
Conclusion: The current study provides evidence of alterations in autonomic functioning as disclosed by increased RHR in CHR-P participants. Future studies are needed to further evaluate this characteristic feature of CHR-P individuals and its potential predictive value for psychosis development.
Schizophrenia (SZ), the most severe manifestation of psychosis with a lifetime prevalence of ~1% (1), is accompanied by a significantly decreased life expectancy (2, 3). Recent evidence suggests cardiovascular diseases (CVD) may contribute significantly toward increased mortality in SZ (4). Furthermore, the prodromal phase of SZ has been associated with a substantially higher risk of physical conditions, such as hypertension, heart disease, and cerebrovascular disease (5). In addition to SZ, altered autonomic functioning has been associated with a range of psychiatric syndromes, including anxiety, depression and personality disorders (6–9).
Resting heart-rate (RHR) is a non-invasive measure of vagal tonic inhibitory control over autonomic function (10, 11) and is determined by intrinsic cardiac mechanisms as well as the activity of both branches of the autonomic nervous system (ANS)—the sympathetic and the parasympathetic (vagus) nerves. At rest, the vagal tone reduces RHR to 60–80 beats per minute (12). A heart rate (HR) higher than the RHR is a result of both the withdrawal of vagal tone and the activation of the sympathetic branch of the ANS (11, 13).
Animal and human data have suggested that cortical activity, especially in prefrontal areas, plays a modulatory role in cardiovascular function (10). A potential pathway for the modulatory role of frontal areas is through the tonic inhibition of the amygdala (14, 15). Specifically, inhibition increases the dominance of vagal influence on cardiovascular activity during rest.
There is emerging evidence that increased RHR may be associated with SZ. For example, a study by Latvala et al. (16) reported that elevated RHR is associated with increased risk for developing SZ. In addition, a number of studies have reported increased RHR in unmedicated first episode SZ patients (17–22). Medication status is important in light of substantial evidence found for effects of atypical antipsychotics on RHR and HR variability (HRV), although this relationship may not hold for other psychiatric disorders (23). Furthermore, psychotic symptom severity has been found to correlate with cardiac ANS disturbances (17). Reinertsen et al. (24) reported that cardio-vascular activity (HR) was associated with illness severity in SZ.
To date, few studies have investigated HR changes in participants that meet criteria for clinical high-risk of psychosis (CHR-P). CHR-P criteria include ultra-high-risk (UHR) criteria (i.e., based on attenuated or transient psychotic symptoms, in addition to genetic risk plus functional deterioration) (25), as well as basic symptoms (BS)—self-experienced perceptual and cognitive disturbances (26, 27). Clamor et al. (28) found a significant increase in RHR in participants with established psychotic disorders, but not in participants meeting UHR criteria. In contrast, Counotte et al. (29) reported associations between psychosis liability (based on UHR criteria and genetic risk for psychosis) and increased HR as well as decreased HRV during a virtual reality experiment with social stressors.
In the present study, we aimed to further investigate altered autonomic functioning in a population of CHR-P individuals (n = 117) to assess whether aberrant HR may be present in the psychosis-risk state. To examine the diagnostic specificity of alterations in ANS, we also assessed identically recruited participants who did not met CHR-P criteria but were characterized by substance abuse and affective disorders (CHR-N = 38) as well as a group of healthy controls (CON = 49).
The psychophysiological measures of cardio-vascular activity were extracted from a 5-min resting-state magnetoencephalography (MEG) recording. The contraction of the heart-muscle generates a strong electrical dipole resulting in a magnetic field [the cardiac field artifact, CFA (30)], which is visible in the PQRST cycle of the electrocardiogram. The CFA signal propagates throughout the body and can be recorded by electrodes positioned at any location. Independent component analysis on MEG data was used to visually identify and extract the heart-rate signal, that is, the inter-beat-interval (IBI) data. In line with recent reports of autonomic changes in the CHR-P individuals (31) and hypothalamic-pituitary-adrenal axis disturbances in first episode psychosis (FEP) patients (32), we expected a significant increase in RHR, as well as a decrease in HRV indices in the CHR-P sample but not in the CHR-N group.
A total of 204 participants were recruited as part of the “Youth Mental Health Risk and Resilience Study” [YouR-Study; (32)], a Medical Research Council funded study aiming at identifying biological and psychological markers of psychosis-risk. Participants were recruited through an online-screening approach [for more detail on the recruitment procedure, see (33)]. The Comprehensive Assessment of At-Risk Mental States (CAARMS) Interview (25) as well as the Schizophrenia Proneness Instrument (SPI-A) (26) were used for establishing CHR-P criteria. For the SPI-A, Cognitive-Perceptive Basic Symptoms (COPER) and Cognitive Disturbances (COGDIS) items were administered. CAARMS-criteria were established as follows: (a) criteria for the attenuated psychosis group (attenuated psychotic symptoms present in the last year without a decline in functioning); (b) criteria for genetic risk with functional decline (30% drop in global functioning score); and (c) criteria for the brief limited intermittent psychotic symptoms (BLIPS) group. Participants who did not meet a CHR-P criterion, were assigned to the CHR-N group (n = 38). CHR-N participants were recruited through the same pathway as CHR-P participants but did not meet BS/UHR-criteria. The rationale was to have a comparison group that is more closely matched for co-morbidity frequently associated with CHR-P participants, such as affective disorders, substance abuse and lower functioning. Finally, a group of 49 healthy participants (CON) were recruited without an Axis I diagnosis or family history of psychotic disorders. All participants were between 16 and 35 years of age and were excluded for current or past diagnosis with Axis I psychotic disorders [see (32) for more details].
The M.I.N.I. International Neuropsychiatric Interview [M.I.N.I. 6.0; (16)], the scales for premorbid adjustment (34), and social and role functioning (35) were administered. Neuropsychological assessment consisted of the Brief Assessment of Cognition in Schizophrenia [BACS (36)], as well as three tasks from the Penn Computerized Neurocognitive Battery [CNB (37); the Continuous Performance Test, the N-Back Task, and the Emotion Recognition Task].
Five-minute, eyes-open resting-state MEG data was acquired using a 248-channel 4D-BTI magnetometer system (MAGNES 3600 WH, 4D-Neuroimaging, San Diego), at a sampling frequency of 1,017.25 Hz, filtered online between DC and 400 Hz. Four minutes of MEG resting-state data were extracted, down sampled to 400 Hz and bandpass-filtered between 5 and 70 Hz.
A continuous single epoch data was created for each channel using the open-source MATLAB Fieldtrip Toolbox (38). The data were then submitted to Independent Component Analyses (ICA) to isolate the component best describing the R-peaks of the heartbeat signal. The resulting single vector of time-varying heartbeat signal was further analyzed using the validated HRV-analyses Matlab Toolbox ARTiiFACT (39).
Firstly, time-series data were low-pass filtered at a manually adjustable cut-off frequency, and R-peaks were detected using a global threshold detection method that was manually adjusted between 0 and 20 μV for each participant. Secondly, visual inspection of IBIs was performed in order to detect extra or missing IBIs and manually correct them. Thirdly, automatically detected artifacts were corrected using cubic spline interpolation.
Four parameters were extracted: (1) RHR, (2) the square root of the mean squared differences of successive normal-to-normal intervals (RMSSD), and (3) the standard deviation of normal-to-normal intervals (SDNN). Fast Fourier transformation was computed with frequency bands set as high frequency (HF, 0.15–0.4 Hz), low frequency (LF, 0.04–0.15 Hz), and very low frequency (VLF, <0.04 Hz). Finally, 4) the ratio of LF and HF (LF/HF), reflecting sympatho-vagal balance (40), was computed.
All statistical analyses were performed using the R statistical software (41). Demographic and clinical parameters were assessed using Welch based F-tests or Kruskal–Wallis H-tests. Independent-sample Kruskal–Wallis H-tests were used to investigate main effects (i.e., relationship between psychophysiological measures and clinical category (CON – CHR-N – CHR-P; with the psychophysiological measure as the outcome and the clinical category as the predictor: outcome ~ predictor). Eta-squared (η2) with bias-corrected and accelerated (BCa) 1,000 samples bootstrapped confidence interval (95% CI) is reported as an indicator of effect size. For significant group effects, Hochberg-corrected, 20% means-trimmed, and 3,000 samples bootstrapped post-hoc comparisons were calculated.
Covariates were included to investigate possible effects including smoking (i.e., number of cigarettes smoked per day, current or past), BMI (42), age, and sex (43), medication (7, 23) (antipsychotics, antidepressants, beta-blockers, anxiolytics, stimulants, mood stabilizers, and combinations of these were considered; for details (see Supplementary Table 1)]. We conducted a parametric ANCOVA with clinical status (CON – CHR-N – CHR-P) as the first and the covariates as remaining predictors. Orthogonal Helmert contrasts were used. The assumption of the homogeneity of regression slopes was examined by including the interaction terms in the model. Tukey post-hoc tests on adjusted means were carried out. Partial omega squared (ω2p) was reported as a measure of effect size. To further investigate the influence of covariates, we conducted within-group analysis for the CHR-N and CHR-P groups separately. When assumptions for within-group analysis of variance were met, we conducted parametric ANOVAs with η2 as effect size.
To investigate the relationship between psychophysiological measures and clinical symptomatology and presentation, CAARMS severity, CAARMS distress, and the comorbidity index were computed for each participant and entered into linear regression and correlational analyses. Spearman's two-sided correlation with BCa 2,000 samples bootstrap 0.95 CI, corrected for ties (ρ [95% CI], p), are reported.
A factor analysis was conducted to assess the effect of psychopathological comorbidity expressed through the comorbidity index variable that was obtained from the M.I.N.I. ratings. In the first step, all M.I.N.I. variables were used except “Antisocial personality disorder,” “Anorexia,” and “Anorexia Binge,” due to missing data. Additional variables were excluded from the factor analysis due to low r-drop values (“Panic No Agoraphobia,” r-drop = 0.14; “Agoraphobia,” r-drop = 0.13), resulting in the final test reliability expressed in standardized alpha of 0.83 (0.78, 0.85). The appropriateness of conducting a factor analysis on these data was confirmed via the Bartlett test (chi-square = 1,727.90, p < 0.001 ***, df = 276, det < 0.001***). Several factor analysis models were tested by performing Pearson's correlation and replacing missing values with medians [see (31)]. The best performing model was the maximum likelihood estimation (BIC = −252.07). Factor loadings were extracted for each participant (the “Comorbidity index” variable).
BACS and CBN Emotion Recognition raw scores were z-transformed to the CON and corrected for sex (44). Non-parametric Spearman's rho correlations were calculated for psychophysiological measures and both BACS and CBN Emotion Recognition data, separately for each group. As these analyses were explorative, no corrections for multiple comparisons were implemented.
Sample characteristics are presented in Table 1. The groups differed significantly with respect to years of education and psychopathological comorbidity, with CHR-N participants having significantly lower years of education and higher comorbidity scores. Furthermore, both the CHR-P and CHR-N groups had significantly higher medication scores than CON. Additionally, the groups were significantly different in terms of CAARMS severity and distress. For a detailed presentation of clinical characteristics (see Supplementary Table 2).
Psychophysiological measures indicated higher RHR for the CHR-P group (mean/SE: 71.63/1.03) compared to CON (67.16/1.37), but not to the CHR-N group (68.31/1.59). This was confirmed by Kruskal–Wallis H tests, revealing marginally significant group differences for RHR (χ2 = 5.98, p = 0.050, η2 = 0.19 [−0.01, 0.08]). Pairwise comparisons showed the expected significant increase in RHR only in the CHR-P group relative to CON (psihat = −3.97, p = 0.015, pcrit = 0.016; see Figure 1). In contrast, no significant group effects were found in HRV indices (RMSSD/SDNN) or changes in sympathovagal balance (LH/HF) (Table 2).
Figure 1. Analyses of variance (mean levels and standard error) and post-hoc group comparisons. CON, healthy controls; CHR-N, clinical high risk-negative; CHR-P, clinical high risk-positive; RHR, resting heart rate; RMSSD, square root of the mean squared differences of successive normal-to-normal intervals; SDNN, standard deviation of normal-to-normal heart beat intervals; LF/HF, ratio of low and high frequency power.
Table 2. Estimated means (standard error) and analysis of group differences for psychophysiological measurements.
A parametric ANCOVA that included age, sex, smoking, and BMI as covariates revealed a significant between-group effect for RHR [F(2, 168) = 3.93, p = 0.021, ω2p = 0.03] as well as a significant effect of sex [F(1, 168) = 7.48, p = 0.007, ω2p = 0.02] and age [F(1, 168) = 3.91, p = 0.05, ω2p = 0.02]. Tukey post-hoc tests on adjusted means revealed a significant CON vs. CHR-P difference (Δ = 5.12, t = 2.64, p = 0.024).
Within-group analysis of the relationship between psychophysiological measures and medication or comorbidity status revealed that both medication and comorbidity factor had a significant effect only on the LF/HF measurement and only in the CHR-P group (see Table 3). No effects were observed for RHR.
Table 3. Within-group effects of medication and comorbidity factor on psychophysiological measurements.
In addition, correlation analyses revealed no significant relationships between the comorbidity factor and any of our psychophysiological measures (Table 4). Yet, there were significant correlations between CAARMS distress and both SDNN (ρ = −0.27, p = 0.004) and RMSSD (ρ = −0.21, p = 0.027) in the CHR-P group only (Figure 2). However, these did not survive correction for multiple comparisons. Linear regression analyses showed a trend-level relationship between RHR and CAARMS severity [adjusted R2 = 0.013, F(1, 199) = 3.764, p = 0.053] as well as distress [adjusted R2 = 0.014, F(1, 201) = 3.887, p = 0.050]. In addition, CAARMS distress but not severity was associated with RMSSD [adjusted R2 = 0.014, F(1, 201) = 4.014, p = 0.046] and SDNN [adjusted R2 = 0.023, F(1, 201) = 5.692, p = 0.018]. There were no significant correlations between BS severity or distress with any of the psychophysiological measures (see Supplementary Table 3).
Table 4. Correlations of psychophysiological measurements with CAARMS severity and distress, and the comorbidity factor.
Figure 2. CAARMS Distress correlation analysis in the CHR-P group. Non-parametric Spearman's correlation is represented. RMSSD, square root of the mean squared differences of successive normal-to-normal intervals; SDNN, standard deviation of normal-to-normal heart beat intervals.
Correlation analysis revealed that the token-motor component of the BACS showed significant correlation with all psychophysiological measures, in particular with RMSSD (ρ = 0.45, p = < 0.001; see Figure 3). There were no significant results for the remaining neurocognitive measures (Table 5).
Figure 3. BACS Token Motor and psychophysiological measures correlation plots for the CHR-P group. Non-parametric Spearman's correlation is represented. RHR, resting heart rate; RMSSD, square root of the mean squared differences of successive normal-to-normal intervals; SDNN, standard deviation of normal-to-normal heart-beat intervals; LF/HF, ratio of low and high frequency power.
Table 5. Correlations of psychophysiological measurements with cognitive and emotion recognition tests.
The current study investigated RHR abnormalities in a sample of CHR-P participants. Cardiovascular activity indices were computed by analyzing the time-varying heartbeat signal obtained directly from resting-state MEG data, which contains the ballisto-cardiogram artifact that is likely caused by blood-flow in the vessels around the head/neck area (45). These indices are thus comparable to the values reported in previous studies using finger photoplethysmography (28, 29), a technique which measures HR indices from blood volume pulses. We found a significant increase in RHR in the CHR-P group compared to healthy controls but not to the CHR-N group. Importantly, the observed difference in RHR was not influenced by several covariates, including age, sex, smoking habits, medication, and BMI.
Previous studies that have investigated cardiovascular changes in smaller psychosis-risk samples have reported conflicting results. Clamor et al. (28) did not find an increase in RHR or a reduction in HRV in CHR-P participants. Interestingly, RHRs for the CHR-P group were similar to those found in our study. However, the HC group in the current study was characterized by lower RHR compared to Clamor et al. (28). Counotte et al. (29) reported both increased HR and reduced HRV in both 22 UHR and 44 first-degree relatives of patients with psychosis. It is important to note, however, that HR was measured during a virtual-reality experiment involving social stressors.
Interestingly, the Counotte study found no changes in recorded levels of skin conductance, a pure measure of sympathetic nervous-system (SNS) activation. This is in line with evidence suggesting that dysfunctional parasympathetic nervous-system (PNS) activity in SZ may be considered as the main contributor to sympathovagal imbalance (9, 46, 47). In the current study, we only found evidence for an increase in RHR, but not in HRV measures such as RMSSD, SDNN, or LF/HF in CHR-P participants. RHR is regarded as a tonic and much more stable measure of ANS functioning than HRV measures, which are highly influenced by breathing rhythm [for example see (48)]. RHR has been associated with severity of psychotic symptoms in chronic SZ patients (49) specifically in terms of changes in PNS functioning, evident in the cardiac vagal index. Specifically, baseline RHR predicted the changes in clinical state on subsequent assessment whereby SZ-patients with elevated RHR showed an increase in the severity of psychosis. The relationship between ANS dysregulation and severity of psychosis was also supported by our observation of a positive relationship between RHR and CAARMS severity and distress.
It has been suggested that ANS dysfunction in SZ is coupled with decreased resting PNS activity, mediated through impaired vagal control in the absence of changes in SNS activity [e.g., review by (46)]. However, the underlying mechanisms for this autonomic imbalance are still unclear. An increase in arousal would be the simplest explanation for increased RHR, but this is unlikely to occur without changes in SNS activity.
Recent findings suggest the vagal nerve has an important influence on immune functioning in SZ patients (50). According to recent meta-analyses, the low-grade inflammatory profile found in SZ patients is correlated with clinical symptom severity (51–53). Furthermore, this inflammatory profile has been reported in drug naïve FEP patients (53). Finally, increased CVD risk in SZ—typically linked to traditional risk factors such as lifestyle, or metabolic syndrome—has recently been shown to bear a relation to systemic inflammation, indicated by increases in leucocyte counts and cytokines concentration (54). Vagal nerve stimulation has been shown to have anti-inflammatory properties [see review (55)] and is potentially involved in inflammation in SZ (50).
A limitation of the present study is that we did not meet the recommended minimum of 5 min for the purposes of cross-study comparison (56), nor did we measure breathing rates. Some studies have shown increased RHRs in conjunction with increased breathing rates in SZ patients [e.g., (18)]. Finally, we also did not assess comorbidities in terms of potential physical illnesses that could alter RHR.
In conclusion, the current data suggest that increased RHR is associated with individuals meeting CHR-P criteria and correlates with severity and distress of increased APS. Given the importance of CVD in SZ (4), it is important to determine the underlying factors that give rise to alterations in RHR in CHR-P individuals. Given recent suggestions of a potential link with neuroinflammation (57), future studies should also further investigate the link between the CHR-P state, aberrant RHR activity, and neuroinflammatory markers. In addition, follow-up data are needed to evaluate its potential as a biomarker for the development of a psychotic disorder.
The raw data supporting the conclusions of this article will be made available by the authors, without undue reservation.
The study was approved by the ethical committees of University of Glasgow and the National Health Services Research Ethical Committee Glasgow and Greater Clyde. All participants provided written informed consent.
AK preformed the data analysis. AK, PU, and TG-'t-J wrote the manuscript. PU conceptualized the project, oversaw design, interpretation, analyses, and edited the manuscript. RG, JG, AG, SL, MS, and FS-L edited the manuscript. All authors contributed to the article and approved the submitted version.
The YouR-study has received research support from Medical Research Council (MR/L011689/1).
The authors declare that the research was conducted in the absence of any commercial or financial relationships that could be construed as a potential conflict of interest.
We thank Frances Crabbe, MSc, Institute of Neuroscience and Psychology, University of Glasgow, Glasgow, Scotland for help in the acquisition of MEG/MRI-data. The investigators also acknowledge the support of the Scottish Mental Health Research Network (http://www.smhrn.org.uk), now called the NHS Research Scotland Mental Health Network (http://www.nhsresearchscotland.org.uk/research-areas/mental-health), for providing assistance with participant recruitment, interviews, and cognitive assessments. We thank both the participants and patients who took part in the study and the research assistants of the YouR-study for supporting the recruitment and assessment of CHR participants.
The Supplementary Material for this article can be found online at: https://www.frontiersin.org/articles/10.3389/fpsyt.2020.580503/full#supplementary-material
1. Sisti DA, Calkins ME. Psychosis risk: what is it and how should we talk about it? AMA J Ethics. (2016) 18:624–32. doi: 10.1001/journalofethics.2016.18.6.msoc1-1606
2. Laursen TM. Life expectancy among persons with schizophrenia or bipolar affective disorder. Schizophr Res. (2011) 131:101–4. doi: 10.1016/j.schres.2011.06.008
3. Druss BG, Zhao L, Von Esenwein S, Morrato EH, Marcus SC. Understanding excess mortality in persons with mental illness: 17-year follow up of a nationally representative US survey. Med Care. (2011) 49:599–604. doi: 10.1097/MLR.0b013e31820bf86e
4. Hennekens CH, Hennekens AR, Hollar D, Casey DE. Schizophrenia and increased risks of cardiovascular disease. Am Heart J. (2005) 150:1115–21. doi: 10.1016/j.ahj.2005.02.007
5. Chen Y-L, Pan C-H, Chang C-K, Chen P-H, Chang H-M, Tai M-H, et al. Physical illnesses before diagnosed as schizophrenia: a nationwide case-control study. Schizophr Bull. (2020) 84:785–94. doi: 10.1093/schbul/sbaa009
6. Chalmers JA, Quintana DS, Abbott MJ, Kemp AH. Anxiety disorders are associated with reduced heart rate variability: a meta-analysis. Front Psychiatry. (2014) 5:80. doi: 10.3389/fpsyt.2014.00080
7. Kemp AH, Quintana DS, Gray MA, Felmingham KL, Brown K, Gatt JM. Impact of depression and antidepressant treatment on heart rate variability: a review and meta-analysis. Biol Psychiatry. (2010) 67:1067–74. doi: 10.1016/j.biopsych.2009.12.012
8. Koenig J, Kemp AH, Feeling NR, Thayer JF, Kaess M. Resting state vagal tone in borderline personality disorder: a meta-analysis. Prog Neuropsychopharmacol Biol Psychiatry. (2016) 64:18–26. doi: 10.1016/j.pnpbp.2015.07.002
9. Montaquila JM, Trachik BJ, Bedwell JS. Heart rate variability and vagal tone in schizophrenia: a review. J Psychiatr Res. (2015) 69:57–66. doi: 10.1016/j.jpsychires.2015.07.025
10. Thayer JF, Hansen AL, Saus-Rose E, Johnsen BH. Heart rate variability, prefrontal neural function, and cognitive performance: the neurovisceral integration perspective on self-regulation, adaptation, and health. Ann Behav Med. (2009) 37:141–53. doi: 10.1007/s12160-009-9101-z
11. Levy MN. Autonomic interactions in cardiac control. Ann N Y Acad Sci. (1990) 601:209–21. doi: 10.1111/j.1749-6632.1990.tb37302.x
12. Uijtdehaage SH, Thayer JF. Accentuated antagonism in the control of human heart rate. Clin Auton Res. (2000) 10:107–10. doi: 10.1007/BF02278013
13. Jose AD, Collison D. The normal range and determinants of the intrinsic heart rate in man. Cardiovasc Res. (1970) 4:160–7. doi: 10.1093/cvr/4.2.160
14. Barbas H, Saha S, Rempel-Clower N, Ghashghaei T. Serial pathways from primate prefrontal cortex to autonomic areas may influence emotional expression. BMC Neurosci. (2003) 4:25. doi: 10.1186/1471-2202-4-25
15. Shekhar A, Sajdyk TJ, Gehlert DR, Rainnie DG. The amygdala, panic disorder, cardiovascular responses. Ann N Y Acad Sci. (2003) 985:308–25. doi: 10.1111/j.1749-6632.2003.tb07090.x
16. Sheehan DV, Lecrubier Y, Sheehan KH, Amorim P, Janavs J, Weiller E, et al. The mini-international neuropsychiatric interview (MINI): the development and validation of a structured diagnostic psychiatric interview for DSM-IV and ICD-10. J Clin Psychiatry. (1998) 59(Suppl 20):22–33. doi: 10.1037/t18597-000
17. Bär K-J, Wernich K, Boettger S, Cordes J, Boettger MK, Löffler S, et al. Relationship between cardiovagal modulation and psychotic state in patients with paranoid schizophrenia. Psychiatry Res. (2008) 157:255–7. doi: 10.1016/j.psychres.2007.04.021
18. Schulz S, Bär K-J, Voss A. Analyses of heart rate, respiration and cardiorespiratory coupling in patients with schizophrenia. Entropy. (2015) 17:483–501. doi: 10.3390/e17020483
19. Schulz S, Bolz M, Bär K-J, Voss A. Central-and autonomic nervous system coupling in schizophrenia. Philos Trans R Soc Math Phys Eng Sci. (2016) 374:20150178. doi: 10.1098/rsta.2015.0178
20. Bär KJ, Letzsch A, Jochum T, Wagner G, Greiner W, Sauer H. Loss of efferent vagal activity in acute schizophrenia. J Psychiatr Res. (2005) 39:519–27. doi: 10.1016/j.jpsychires.2004.12.007
21. Chang H-A, Chang C-C, Tzeng N-S, Kuo TB, Lu R-B, Huang S-Y. Cardiac autonomic dysregulation in acute schizophrenia. Acta Neuropsychiatr. (2013) 25:155–64. doi: 10.1111/acn.12014
22. Schulz S, Tupaika N, Berger S, Haueisen J, Bär K-J, Voss A. Cardiovascular coupling analysis with high-resolution joint symbolic dynamics in patients suffering from acute schizophrenia. Physiol Meas. (2013) 34:883. doi: 10.1088/0967-3334/34/8/883
23. Alvares GA, Quintana DS, Hickie IB, Guastella AJ. Autonomic nervous system dysfunction in psychiatric disorders and the impact of psychotropic medications: a systematic review and meta-analysis. J Psychiatry Neurosci. (2016) 41:89–104. doi: 10.1503/jpn.140217
24. Reinertsen E, Osipov M, Liu C, Kane JM, Petrides G, Clifford GD. Continuous assessment of schizophrenia using heart rate and accelerometer data. Physiol Meas. (2017) 38:1456. doi: 10.1088/1361-6579/aa724d
25. Yung AR, Yuen HP, McGorry PD, Phillips LJ, Kelly D, Dell'Olio M, et al. Mapping the onset of psychosis: the comprehensive assessment of at-risk mental states. Aust N Z J Psychiatry. (2005) 39:964–71. doi: 10.1080/j.1440-1614.2005.01714.x
26. Schultze-Lutter F, Addington J, Ruhrmann S, Klosterkötter J. Schizophrenia Proneness Instrument, Adult Version (SPI-A). Rome: Giovanni Fioriti (2007).
27. Schultze-Lutter F, Debbané M, Theodoridou A, Wood SJ, Raballo A, Michel C, et al. Revisiting the basic symptom concept: toward translating risk symptoms for psychosis into neurobiological targets. Front Psychiatry. (2016) 7:9. doi: 10.3389/fpsyt.2016.00009
28. Clamor A, Sundag J, Lincoln TM. Specificity of resting-state heart rate variability in psychosis: a comparison with clinical high risk, anxiety, healthy controls. Schizophr Res. (2019) 206:89–95. doi: 10.1016/j.schres.2018.12.009
29. Counotte JR, Pot-Kolder R, van Roon AM, Hoskam OM, van der Gaag M, Veling W. High psychosis liability is associated with altered autonomic balance during exposure to virtual reality social stressors. Schizophr Res. (2017) 184:14–20. doi: 10.1016/j.schres.2016.11.025
30. Sander TH, Wubbeler G, Lueschow A, Curio G, Trahms L. Cardiac artifact subspace identification and elimination in cognitive MEG data using time-delayed decorrelation. IEEE Trans Biomed Eng. (2002) 49:345–54. doi: 10.1109/10.991162
31. Lahey BB, Applegate B, Hakes JK, Zald DH, Hariri AR, Rathouz PJ. Is there a general factor of prevalent psychopathology during adulthood? J Abnorm Psychol. (2012) 121:971. doi: 10.1037/a0028355
32. Uhlhaas PJ, Gajwani R, Gross J, Gumley AI, Lawrie SM, Schwannauer M. The youth mental health risk and resilience study (YouR-Study). BMC Psychiatry. (2017) 17:43. doi: 10.1186/s12888-017-1206-5
33. McDonald M, Christoforidou E, Van Rijsbergen N, Gajwani R, Gross J, Gumley AI, et al. Using online screening in the general population to detect participants at clinical high-risk for psychosis. Schizophr Bull. (2018) 45:600–9. doi: 10.1093/schbul/sby069
34. Cannon-Spoor HE, Potkin SG, Wyatt RJ. Measurement of premorbid adjustment in chronic schizophrenia. Schizophr Bull. (1982) 8:470–84. doi: 10.1093/schbul/8.3.470
35. Cornblatt BA, Auther AM, Niendam T, Smith CW, Zinberg J, Bearden CE, et al. Preliminary findings for two new measures of social and role functioning in the prodromal phase of schizophrenia. Schizophr Bull. (2007) 33:688–702. doi: 10.1093/schbul/sbm029
36. Keefe RS, Goldberg TE, Harvey PD, Gold JM, Poe MP, Coughenour L. The brief assessment of cognition in schizophrenia: reliability, sensitivity, and comparison with a standard neurocognitive battery. Schizophr Res. (2004) 68:283–97. doi: 10.1037/t38021-000
37. Moore TM, Reise SP, Gur RE, Hakonarson H, Gur RC. Psychometric properties of the penn computerized neurocognitive battery. Neuropsychology. (2015) 29:235. doi: 10.1037/neu0000093
38. Oostenveld R, Fries P, Maris E, Schoffelen J-M. FieldTrip: open source software for advanced analysis of MEG, EEG, and invasive electrophysiological data. Comput Intell Neurosci. (2011) 2011:156869. doi: 10.1155/2011/156869
39. Kaufmann T, Sütterlin S, Schulz SM, Vögele C. ARTiiFACT: a tool for heart rate artifact processing and heart rate variability analysis. Behav Res Methods. (2011) 43:1161–70. doi: 10.3758/s13428-011-0107-7
40. T.F. of the E.S. of Cardiology, Guidelines. Heart rate variability, standards of measurement, physiological interpretation, clinical use. Eur Heart J. (1996) 17:354–81. doi: 10.1093/oxfordjournals.eurheartj.a014868
41. R core Team. R: A Language and Environment for Statistical Computing. Vienna: R Foundation for Statistical Computing (2013). Available online at https://www.R-project.org/
42. Status WP. The Use and Interpretation of Anthropometry. WHO Technical Report Series (1995). 854.
43. Nanchen D. Resting Heart Rate: What Is Normal? BMJ Publishing Group Ltd and British Cardiovascular Society (2018). doi: 10.1136/heartjnl-2017-312731
44. Keefe RS, Harvey PD, Goldberg TE, Gold JM, Walker TM, Kennel C, et al. Norms and standardization of the brief assessment of cognition in schizophrenia (BACS). Schizophr Res. (2008) 102:108–15. doi: 10.1016/j.schres.2008.03.024
45. Litvak V, Eusebio A, Jha A, Oostenveld R, Barnes GR, Penny WD, et al. Optimized beamforming for simultaneous MEG and intracranial local field potential recordings in deep brain stimulation patients. Neuroimage. (2010) 50:1578–88. doi: 10.1016/j.neuroimage.2009.12.115
46. Guccione C, di Scalea GL, Ambrosecchia M, Terrone G, Di Cesare G, Ducci G, et al. Early signs of schizophrenia and autonomic nervous system dysregulation: a literature review. Clin Neuropsychiatry. (2019) 16:86–97.
47. Bär K-J, Boettger MK, Koschke M, Schulz S, Chokka P, Yeragani VK, et al. Non-linear complexity measures of heart rate variability in acute schizophrenia. Clin Neurophysiol. (2007) 118:2009–15. doi: 10.1016/j.clinph.2007.06.012
48. Quintana DS, Heathers JA. Considerations in the assessment of heart rate variability in biobehavioral research. Front Psychol. (2014) 5:805. doi: 10.3389/fpsyg.2014.00805
49. Toichi M, Kubota Y, Murai T, Kamio Y, Sakihama M, Toriuchi T, et al. The influence of psychotic states on the autonomic nervous system in schizophrenia. Int J Psychophysiol. (1999) 31:147–54. doi: 10.1016/S0167-8760(98)00047-6
50. das Corsi-Zuelli GFM, Brognara F, da Quirino SGF, Hiroki CH, Fais RS, Del-Ben CM, et al. Neuroimmune interactions in schizophrenia: focus on vagus nerve stimulation and activation of the alpha-7 nicotinic acetylcholine receptor. Front Immunol. (2017) 8:618. doi: 10.3389/fimmu.2017.00618
51. Goldsmith DR, Rapaport MH, Miller BJ. A meta-analysis of blood cytokine network alterations in psychiatric patients: comparisons between schizophrenia, bipolar disorder and depression. Mol Psychiatry. (2016) 21:1696–709. doi: 10.1038/mp.2016.3
52. Miller BJ, Buckley P, Seabolt W, Mellor A, Kirkpatrick B. Meta-analysis of cytokine alterations in schizophrenia: clinical status and antipsychotic effects. Biol Psychiatry. (2011) 70:663–71. doi: 10.1016/j.biopsych.2011.04.013
53. Upthegrove R, Manzanares-Teson N, Barnes NM. Cytokine function in medication-naive first episode psychosis: a systematic review and meta-analysis. Schizophr Res. (2014) 155:101–8. doi: 10.1016/j.schres.2014.03.005
54. Chung K-H, Chen P-H, Kuo C-J, Tsai S-Y, Huang S-H, Wu W-C. Risk factors for early circulatory mortality in patients with schizophrenia. Psychiatry Res. (2018) 267:7–11. doi: 10.1016/j.psychres.2018.05.044
55. Bonaz B, Sinniger V, Pellissier S. Anti-inflammatory properties of the vagus nerve: potential therapeutic implications of vagus nerve stimulation. J Physiol. (2016) 594:5781–90. doi: 10.1113/JP271539
56. Laborde S, Mosley E, Thayer JF. Heart rate variability and cardiac vagal tone in psychophysiological research–recommendations for experiment planning, data analysis, data reporting. Front Psychol. (2017) 8:213. doi: 10.3389/fpsyg.2017.00213
Keywords: autonomic functioning, clinical high risk for psychosis (CHR-P), heart-rate variability, resting heart rate, Schizophrenia
Citation: Kocsis A, Gajwani R, Gross J, Gumley AI, Lawrie SM, Schwannauer M, Schultze-Lutter F, Grent-‘t-Jong T and Uhlhaas PJ (2020) Altered Autonomic Function in Individuals at Clinical High Risk for Psychosis. Front. Psychiatry 11:580503. doi: 10.3389/fpsyt.2020.580503
Received: 06 July 2020; Accepted: 11 September 2020;
Published: 06 November 2020.
Edited by:
Maximus Berger, University of Melbourne, AustraliaReviewed by:
Cheryl Mary Corcoran, Mount Sinai Medical Center, United StatesCopyright © 2020 Kocsis, Gajwani, Gross, Gumley, Lawrie, Schwannauer, Schultze-Lutter, Grent-‘t-Jong and Uhlhaas. This is an open-access article distributed under the terms of the Creative Commons Attribution License (CC BY). The use, distribution or reproduction in other forums is permitted, provided the original author(s) and the copyright owner(s) are credited and that the original publication in this journal is cited, in accordance with accepted academic practice. No use, distribution or reproduction is permitted which does not comply with these terms.
*Correspondence: Peter J. Uhlhaas, cGV0ZXIudWhsaGFhc0BjaGFyaXRlLmRl
†These authors share senior authorship
Disclaimer: All claims expressed in this article are solely those of the authors and do not necessarily represent those of their affiliated organizations, or those of the publisher, the editors and the reviewers. Any product that may be evaluated in this article or claim that may be made by its manufacturer is not guaranteed or endorsed by the publisher.
Research integrity at Frontiers
Learn more about the work of our research integrity team to safeguard the quality of each article we publish.