- 1Department of Psychiatry, Beth Israel Deaconess Medical Center, Boston, MA, United States
- 2Department of Psychiatry, Harvard Medical School, Boston, MA, United States
- 3Schizophrenia and Bipolar Disorder Program, McLean Hospital, Belmont, MA, United States
- 4School of Social Work and Department of Psychiatry, University of Pittsburgh, Pittsburgh, PA, United States
Background: Psychotic disorders are characterized by impairment in social cognitive processing, which is associated with poorer community functioning. However, the neural mechanisms of social impairment in psychosis remain unclear. Social impairment is a hallmark of other psychiatric illnesses as well, including autism spectrum disorders (ASD), and the nature and degree of social cognitive impairments across psychotic disorders and ASD are similar, suggesting that mechanisms that are known to underpin social impairments in ASD may also play a role in the impairments seen in psychosis. Specifically, in both humans and animal models of ASD, a cerebellar–parietal network has been identified that is directly related to social cognition and social functioning. In this study we examined social cognition and resting-state brain connectivity in people with psychosis and in neurotypical adults. We hypothesized that social cognition would be most strongly associated with cerebellar–parietal connectivity, even when using a whole-brain data driven approach.
Methods: We examined associations between brain connectivity and social cognition in a trans-diagnostic sample of people with psychosis (n = 81) and neurotypical controls (n = 45). Social cognition was assessed using the social cognition domain score of the MATRICS Consensus Cognitive Battery. We used a multivariate pattern analysis to correlate social cognition with resting-state functional connectivity at the individual voxel level.
Results: This approach identified a circuit between right cerebellar Crus I, II and left parietal cortex as the strongest correlate of social cognitive performance. This connectivity-cognition result was observed in both people with psychotic disorders and in neurotypical adults.
Conclusions: Using a data-driven whole brain approach we identified a cerebellar–parietal circuit that was robustly associated with social cognitive ability, consistent with findings from people with ASD and animal models. These findings suggest that this circuit may be marker of social cognitive impairment trans-diagnostically and support cerebellar–parietal connectivity as a potential therapeutic target for enhancing social cognition.
Introduction
Psychotic disorders such as schizophrenia (SZ) spectrum disorders and bipolar disorder (BD) with psychosis are characterized by substantial impairment in social cognitive processing (1–3), which is associated with poorer community functioning (4–7). Social cognitive impairments have been reported in people with SZ and BD across multiple domains including various aspects of emotion processing such as facial affect recognition and “higher level” emotional reasoning (8–12), theory of mind (8, 13–15) and attributional style (16–18). Some aspects of social cognition appear to be more severely impaired in people with SZ compared to those with BD including “higher level” emotion processing (19, 20), theory of mind, and attributional style (10, 21), although in general differences appear more quantitative than qualitative, and empirical methods such as cluster analysis have revealed subgroups of patients cross-diagnostically who share similar levels of social cognitive functioning ranging from intact to more severely impaired (11).
Social cognitive impairments are not unique to psychosis but represent hallmark symptoms in other psychiatric disorders as well, including autism spectrum disorders (ASD). Recent evidence indicates that, behaviorally, people with psychotic disorders and ASD exhibit similar widespread social cognitive impairment relative to controls (22–24). Thus, it is possible that neural mechanisms believed to underpin social cognitive impairment in ASD may offer clues to neural substrates underlying similar deficits in SZ and BD. However, the extent to which similar behavioral phenotypes are underpinned by common neurobiological mechanisms across diagnoses is unclear.
Trans-diagnostic studies of neuroimaging and social cognitive impairment in people with SZ-spectrum disorders and ASD participants have been mixed, with some showing similar activation or connectivity patterns and others showing only partial overlap. In studies using fMRI-measured task-based activation, reduced frontolimbic and superior temporal sulcus (STS) engagement during social cognition tasks was a shared feature across diagnoses (25–27). Cortical connectivity abnormalities in default mode network (DMN) and salience networks were also common to both SZ-spectrum and ASD in adults and adolescents (28, 29) which were associated with abnormalities during mentalizing (29) and associated with severity of social impairment (30). However, some findings report diagnostic differences in regional activation even when task performance is similar (31) suggesting that similar behavioral phenotypes may result from different underlying mechanisms. Similarly, the above meta-analysis (27) found diagnosis-specific activation abnormalities including reduced thalamic and amygdala activation and ventrolateral prefrontal dysfunction primarily in SZ, decreased somatosensory engagement in ASD, and some task-specific differences in activation patterns. Overall, these findings suggest that some regional activation and network connectivity abnormalities may be common trans-diagnostically and associated with social cognition and functioning, although no clear mechanistic pathway has been identified within or across disorders. These studies have largely been based on purely correlational experiments, however, making it difficult to determine whether these associations are causal or are reflective of diagnosis-related epiphenomena.
While much work on the neurobiology of social cognition in psychosis has focused on cortical and limbic activation and connectivity (3, 32, 33), abnormalities of the cerebellum have consistently been reported in psychiatric disorders characterized by social cognitive impairments including SZ (34–38) and ASD (39–42). While cerebellum is commonly considered in terms of motor behavior, the cerebellum appears to play an important role in social cognition and emotion processing [e.g., (43–46)] and may be associated with social and emotional processing impairments seen in psychotic disorders and ASD. Few empirical reports have linked cerebellar abnormalities to social cognitive impairments in SZ [see (47)], and there are no such reports we are aware of in BD. However, recent evidence of associations between cerebellum and social cognition in ASD provide evidence of a specific cerebellar-cortical circuit directly related to social cognition.
Stoodley et al. used neuromodulation in humans and mice to demonstrate a causal association between connectivity of the Right Crus I (R Crus I) region of the cerebellum (commonly implicated in ASD) and the inferior parietal lobule and social behavior (48). Using neuromodulation, they identified a cerebellar–parietal circuit in neurotypical humans, and abnormalities of functional connectivity in this same circuit in children with ASD. They then went on to demonstrate that chemogenetically mediated inhibition of R Crus I activity in mice produced social behavioral impairment, whereas stimulation of R Crus I in a transgenic ASD mouse model rescued aberrant social behaviors. These novel findings are consistent with previous evidence from lesion studies in humans and animal models (49, 50), and suggest that this cerebellar parietal circuit may be directly and causally associated with social cognition in ASD. Whether this circuit is associated with social cognition in humans with psychotic disorders and thereby represents a trans-diagnostic mechanism for social processing impairments remains unknown.
In this report we aimed to examine whether previous findings of social cognition-connectivity associations in ASD were also present in people with psychotic disorders including SZ and BD. Specifically, we examined social cognition in association with resting-state (rsfMRI) brain connectivity in a trans-diagnostic sample of people with psychotic disorders as well as neurotypical controls using a data-driven, whole brain approach. We hypothesized that (1) people with psychosis would perform worse than neurotypical controls on an emotion management/emotion regulation task of social cognition; (2) social cognitive performance would be positively correlated with connectivity in the cerebellar–parietal circuit identified in people with ASD (48); and (3) associations between social cognitive performance and cerebellar–parietal connectivity would be similar across groups, indicating that this circuit is a common pathway underpinning social cognition.
Materials and Methods
Participants
Participants included people with a diagnosis of SZ or BD with psychosis (n = 81) and neurotypical controls (n = 45). Participants were recruited at three collaborating health centers via clinical programs including early psychosis specialty care, and through community referral networks, in the context of several separate research studies. Participants recruited from the Boston and Pittsburgh sites participated in a clinical trial (BICEPS, NCT01561859). Only the baseline (pre-intervention) evaluation data for these participants were included for this analysis. At the McLean site participants were recruited in the context of two separate but related studies including a study of cognitive remediation in bipolar disorder (TREC-BD, NCT01470781) and a study of clinical and cognitive characterization of psychosis. For subjects who participated in the cognitive remediation intervention study, only baseline cognitive and imaging data were included here. All procedures were approved by the Institutional Review Boards of the University of Pittsburgh (Pittsburgh, PA), McLean Hospital (Belmont, MA), and Beth Israel Deaconess Medical Center (Boston, MA). Every participant provided written informed consent prior to their participation. A subset of the data analyzed here was previously presented in Ling et al. (51).
Across sites, diagnosis was determined using the Structured Clinical Interview for the DSM-IV (SCID) (52), administered by trained raters of the SCID and confirmed by a doctoral-level clinician. All participants were clinically stable outpatients at the time of assessment. Inclusion criteria for participants at the Pittsburgh and Boston sites were: (1) between 18 and 45 years old; (2) current IQ ≥ 80, assessed by the WASI-II (53); and (3) fluent English speaker with the ability read at a sixth grade level or higher. Additional inclusion criteria for the participants with a psychotic disorder were: (1) a SZ or schizoaffective disorder diagnosis, verified using the SCID interview (54); (2) time since first psychotic symptoms of <10 years; and (3) clinically stabilized on antipsychotic medication. Inclusion criteria for psychotic disorder participants at the Belmont site were: (1) age 18–60 years; (2) diagnosis of SZ, schizoaffective disorder, or BD with psychotic features; and (3) clinically stable defined as no psychiatric hospitalization or medication change in the past month. Across sites exclusion criteria included: (1) significant neurological or medical disorders that might cause cognitive impairment (e.g., seizure disorder, traumatic brain injury); (2) persistent suicidal or homicidal behavior; (3) substance abuse or dependence present within the past 3 months; (4) any MRI contraindications; and (5) decisional incapacity requiring a guardian.
Neurotypical participants had never met criteria for any Axis I psychiatric disorder and had no history of head injury resulting in a loss of consciousness, seizure or neurological disorder. Table 1 summarizes the sample's demographic, clinical, and medication regimen information.
Cognitive Testing
The MATRICS Consensus Cognitive Battery (MCCB) was used to assess cognition (55, 56). This testing battery yields a cognitive composite score and 7 domain scores including processing speed, attention, working memory, verbal learning, visual learning, problem solving, and social cognition. In the MCCB, social cognition is assessed using the Mayer-Salovey-Caruso Emotional Intelligence Test (57) Managing Emotions branch (MSCEIT-ME). The MSCEIT-ME includes a series of vignettes. The vignettes are read aloud to participants as they follow along in their printed materials. Each vignette proposes a series of possible actions related to its scenario. The participants are asked to assess the effects each action would have on the actor's or other characters' mood states or behaviors. Responses follow a Likert-type scale. The MSCEIT-ME and MCCB scoring packages were used to calculate age and sex normed T scores.
Participants at the Boston and Pittsburg sites had full-scale (FSIQ) assessed using the Wechsler Abbreviated Scale of Intelligence (WASI). Participants at the McLean site had FSIQ and verbal IQ (VIQ) assessed using the North American Adult Reading Test (NAART).
MRI Data Acquisition
Boston site: Data were acquired on 3T Siemens Trio (TIM upgrade) scanners using a standard head coil. The echoplanar imaging parameters were: repetition time, 3,000 ms; echo time, 30 ms; flip angle, 85°; 3 × 3 × 3-mm voxels; and 47 axial sections collected with interleaved acquisition and no gap. Structural data included a high-resolution T1 image. All participants underwent a resting-state fMRI run. Each functional run lasted 6.2 min (124 time points).
Pittsburgh site: Data were acquired on a 3T Siemens Verio scanner using a standard head coil. The echoplanar imaging parameters were: repetition time, 3,000 ms; echo time, 30 ms; flip angle, 85°; 3 × 3 × 3-mm voxels; and 45 axial sections collected with interleaved acquisition and no gap. Structural data included a high-resolution T1 image. The functional run lasted 6.2 min (124 time points).
McLean site (SZ): Data were acquired on 3T Siemens Trio (TIM upgrade) scanners using a standard head coil. The echoplanar imaging parameters were: repetition time, 3,000 ms; echo time, 30 ms; flip angle, 85°; 3 × 3 × 3-mm voxels; and 47 axial sections collected with interleaved acquisition and no gap. Structural data included a high-resolution T1 image. Each functional run lasted 6.2 min (124 time points) and the participants were given instructions to “remain still, stay awake, and keep your eyes open.”
McLean site (BP): Data were acquired on 3T Siemens Trio (TIM upgrade) scanners using a standard head coil. The echoplanar imaging parameters were: repetition time, 2,500 ms; echo time, 24 ms; flip angle, 82°; 3 × 3 × 3-mm voxels; and 42 axial sections collected with interleaved acquisition and no gap. Structural data included a high-resolution T1 image. Each resting-state functional run here lasted 10 min (240 time points) and the participants were given instructions to “remain still, stay awake, and keep your eyes open.”
MRI Data Processing
MRI image preprocessing was performed as in presented in Ling et al. (51). DPABI image processing software was used to preprocess the imaging data (58). To minimize the scanner signal stabilization effects, the first images were omitted from all analysis (the first 4 images from 124 time point scans and first 10 images from 240 time point scans). We discarded scans with head motion that exceeded a 3 mm or 3° of maximum rotation threshold during the resting-state run. Functional and structural images were co-registered. Using the DARTEL technique (59), the structural images were normalized and segmented into gray, white and CSF partitions. Head motion effects were regressed out from the realigned data using a Friston 24-parameter model (60). CSF and white matter signals along with the global signal and the linear trend were regressed out. We incorporated the global signal regression because prior demonstration showed that combining it with volume-wise “scrubbing” for head “micromovements” is an effective method to remove motion artifacts (61). Following realignment, slice timing correction and co-registration, framewise displacement (FD) was calculated for all resting state volumes (62). All volumes within a scan that had a FD >0.2-mm were censored. Scans that required censoring half, or more, of their volumes were discarded. After nuisance covariate regression, the resultant data were band-pass filtered to select low frequency (0.01–0.08 Hz) signals. DARTEL normalized the filtered data into MNI space and then the data were smoothed by a Gaussian kernel of 8 mm3 full-width at half maximum (FWHM). Voxels contained within a group derived gray matter mask were used for further analyses.
After preprocessing, 126 participants, across all sites, remained in the study. 51 participants diagnosed with SZ, 9 with schizoaffective disorder, 21 with BD, and 45 neurotypical participants comprise our sample (Table 1).
Functional Connectivity Analysis
Multivariate Distance Matrix Regression
We performed a connectome-wide association study using multivariate distance matrix regression (MDMR) as originally laid out in Shehzad et al. (63). In brief, MDMR tests every voxel to determine if whole-brain connectivity to that voxel is more similar in individuals with similar scores on an independent measure (MSCEIT-ME) than in individuals with dissimilar scores. As described (64–66), MDMR occurs in several stages: First, scan and MSCEIT-ME scores are collected from all participants (Figure 1A). Next, a seed-to-voxel connectivity map is generated for every participant. These maps are created by calculating the temporal Pearson's correlation coefficients between each voxel, using its BOLD signal time-course, and all other gray matter voxels (Figure 1B). Second, the temporal correlation coefficients for each voxel in the connectivity map are correlated with the values of corresponding voxels in the maps generated for the other participants. This Pearson's correlation coefficient, r, is a measure addressing how similar the whole-brain connectivity to a specific voxel is, for each voxel, between patients. This value is used to calculate between-subject distance (or dissimilarity) using the metric dij = where i and j are two subjects and r is the correlation coefficient above (Figure 1C) (67). Third, we test the relationship between the independent variable of interest, here, MSCEIT-ME score, and the inter-subject distances in connectivity generated in the previous stage. Broadly speaking, this process consists of an ANOVA-like hypothesis test between a variable of interest and a matrix of distances. This method was originally named multivariate distance matrix regression by Zapala and Schork while they focused on associations between gene expression and related variables (67). Shezhad et al. then shifted their analytic focus, and used this framework to test the relationship between variables of interest and a matrix of distances, the matrix being similarity between-subject's whole-brain functional connectivity. This test first creates a distance matrix among n participants where d = the between subject distance metric calculated above. Next, this matrix is used to create a Gower's centered matrix , in which n is the number of participants, I is the n × n identity matrix, and 1 is a vector of n 1s. The F statistic for assessing the relationship between a predictor variable (e.g., MSCEIT-ME score) and dissimilarities in connectivity is calculated as follows: For m predictor variables, let X be a n × m design matrix of predictor values, and let H = X(XTX)−1XT be the associated n × m “hat” matrix.
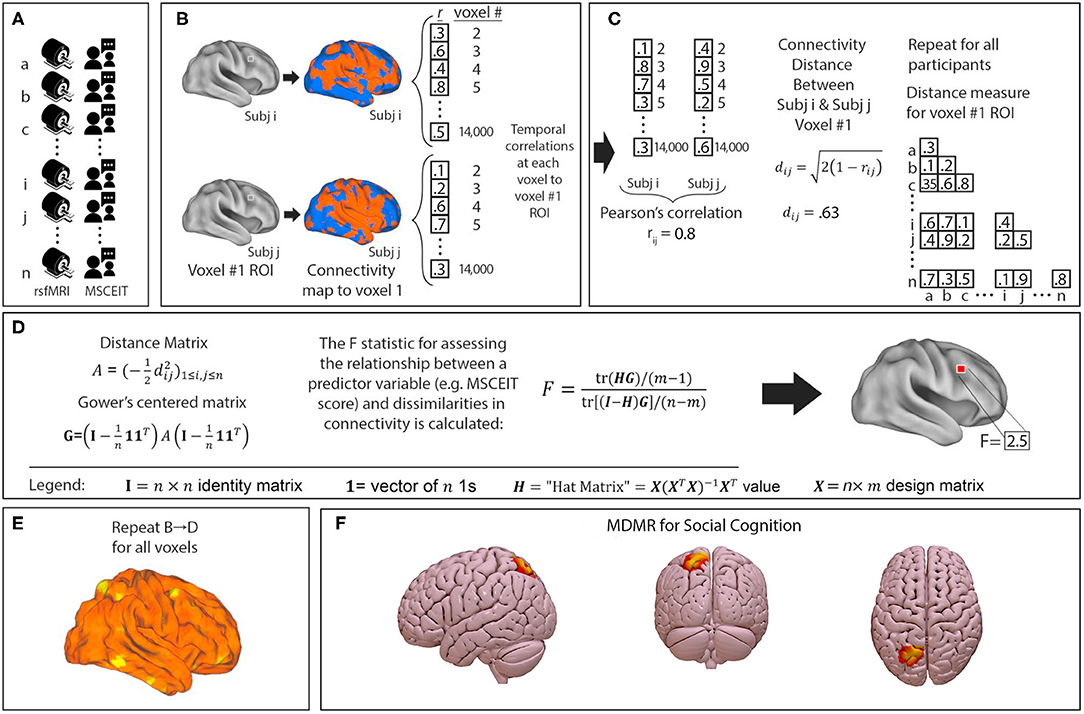
Figure 1. Multivariate distance matrix regression identifies left parietal connectivity as the strongest correlate of social cognitive ability in a trans-diagnostic sample. MDMR procedure: (A) rsfMRI and emotional intelligence testing are collected from each participant. (B) For each participant a functional connectivity map is generated to an individual voxel. (C) Voxelwise temporal correlations between participants are used to generate a Pearson's correlation r and a distance metric d. This is repeated for all participants to generate a matrix of between subject distances. (D) The distance matrix is centered and an ANOVA-like test is used to generate an F-statistic to assess the relationship between a predictor variable (MSCEIT-ME score) and dissimilarities in functional connectivity at that voxel. (E) This process is repeated for every voxel. This results in a whole brain map of how significantly functional connectivity is related to emotional intelligence. Permutation testing then identifies whole-brain significant clusters in connectivity-MSCEIT-ME relationships. (F) In our sample of 126 participants (n = 60 with schizophrenia or schizoaffective disorder, n = 21 with bipolar disorder with psychosis, and n = 45 neurotypical participants), we identified a single region in the left parietal lobule (centered at MNI coordinates x – 24 y – 69 z + 57) whose connectivity correlated significantly with emotional intelligence. In this image, connectivity is thresholded at a voxelwise level of p < 0.001 and extent threshold of p < 0.05.
(Figure 1D) (63). This process is repeated for every voxel. The result is a whole brain map showing how significant the relationship between MSCEIT-ME scores and functional connectivity is at every voxel (Figure 1E). From this generated map, ROIs for follow-up analysis are determined based on clusters of significant voxelwise F-statistics. To correct for multiple comparisons, a nonparametric permutation is calculated for voxels that exceed the significance threshold of p < 0.001 and clusters of such with an extent threshold of p < 0.05, with a null distribution calculated from 1,000 such permutations (Figure 1F). The voxelwise threshold was selected to maximize the replicability potential.
This MDMR analysis identifies anatomical regions where MSCEIT-ME score is significantly correlated with functional connectivity. Notably, this process does not consider spatial information about the voxels that give rise to between-individual distances. For example, two individuals may be very distant, or dissimilar, in the functional connectivity of a voxel in the precuneus. Such dissimilarity might be driven by differences in precuneus connectivity to the mPFC, temporal lobe, parietal lobe, or perhaps all three. MDMR, as implemented by Shehzad et al. (63), does not present this information. Visualizing this missing spatial information requires follow-on seed-based connectivity analysis. Shehzad et al. and others have defined this follow-on analysis as “post-hoc” testing to clarify that this alone, is not sufficient hypothesis testing nor an independent validation of the original MDMR finding (63–66). Following these prior manuscripts, we conducted the MDMR analysis to locate anatomical regions of interest where connectivity significantly correlated with MSCEIT-ME score and then performed follow-on seed-based connectivity analysis to detail the spatial distribution of these connectivity differences.
Seed Based Connectivity Analyses
We used DPABI for our seed-based connectivity analyses. This analysis extracted the BOLD signal time course in a 6 mm spherical ROI centered in the result of the MDMR (MNI x – 24 y – 69 z + 57). We then generated whole brain maps of z-transformed Pearson's correlation coefficients. We entered these maps into SPM12 (Statistical and Parametric Mapping, http://www.fil.ion.ucl.ac.uk/spm). Next, we regressed these maps against MSCEIT-ME scores. This process generated spatial maps that show how whole brain functional connectivity to the ROI varies with MSCEIT-ME score. We performed these analyses with sex, age, and scanner site as covariates to control for participant variables of non-interest.
In our sample, prescribed CPZE dosage was inversely correlated with MSCEIT-ME score (r = −0.445, p < 0.001). To control for possible medication regimen effects, this analysis was re-performed with the covariates above (age, scanner site, and sex) plus prescribed anti-psychotic dosage (in chlorpromazine equivalents, CPZE) as an additional covariate (Supplementary Figure 2).
ROI to ROI Analyses
To generate a scatter plot of the relationship between functional connectivity and MSCEIT score we extracted the BOLD signal time course between the MDMR centered ROI and the cerebellar cluster (thresholded at voxelwise p < 0.001).
Correlations between connectivity and MSCEIT and partial correlations with FSIQ or VIQ or CPZE as covariates were calculated using r.
Figure Generation
SurfIce was used to generate the projections of ROIs and T contrast maps onto cortical surfaces (www.nitrc.org/projects/surfice/).
Results
Functional Connectivity in the Superior Parietal Lobule Is Linked to Social Cognition
MDMR analysis performed across all 126 participants (51 SZ, 9 schizoaffective disorder, 21 BD with psychosis, 45 neurotypical) revealed a single region whose intrinsic functional connectivity correlated significantly with MSCEIT-ME social cognition scores. This identified a region in the left superior parietal lobule centered at MNI coordinates x – 24 y – 69 z + 57 (Figure 1F).
Parietal–Cerebellar Connectivity Is Linked to Social Cognitive Ability
We performed follow-on analysis using this parietal region in a seed-based connectivity analysis to determine the spatial distribution and directionality of connectivity that gave rise to this result. This analysis revealed that social cognition is positively correlated to functional connectivity between the left parietal lobe and other regions of the DMN including DMN nodes in both bilateral parietal lobes and bilateral cerebellum. This relationship was observed maximally between left superior parietal lobe and the Crus I, II region of the cerebellum (Figure 2 and Supplementary Figure 1).
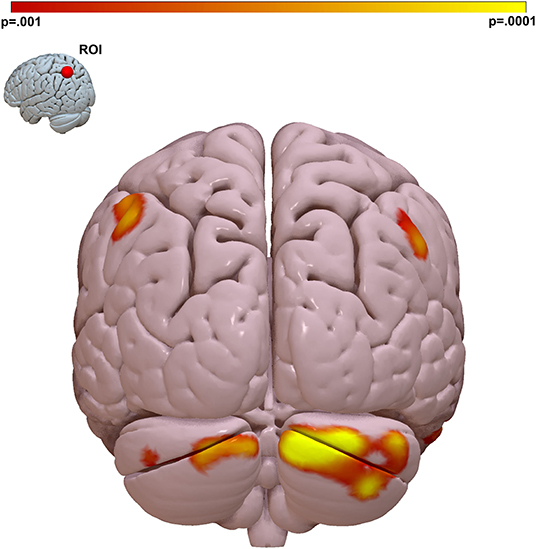
Figure 2. The strongest link between connectivity and social cognitive ability is a parietal Lobe-cerebellar Crus I, II circuit. We visualized the spatial distribution of connectivity that gave rise to the MDMR result in Figure 1. We placed a ROI in the left parietal region identified by MDMR and regressed connectivity to this region against MSCEIT-ME score. This identified the right cerebellar Crus I, II region as the region where functional connectivity correlates with social cognitive ability. Peak T-stat T = 4.99, p < 0.001, MNI x – 12, y – 90, z – 30. Cluster k = 695, pFWE < 0.001. Color bar = voxel connectivity p-value.
In our sample participants with a psychotic disorder demonstrated social cognitive ability a full standard deviation below the neurotypical participants (Table 1). When we examined individual diagnostic groups, we observed that the relationship between connectivity and cognition was similar for all groups: Neurotypical participants: r = 0.434, p = 0.003; BD participants: r = 0.448, p = 0.042. SZ/schizoaffective participants: r = 0.394, p = 0.002. Comparing the strength of correlation between groups did not reveal significant differences between neurotypical and BD groups (p = 0.952), between neurotypical and SZ /schizoaffective groups (p = 0.810), or between bipolar and SZ/schizoaffective groups (p = 0.810).
To isolate social cognition specific effects we calculated the partial correlation between parietal–cerebellar connectivity and MSCEIT with estimated IQ regressed out as a covariate. We continued to observe the same strong correlation between connectivity and MSCEIT score r = 0.410, p < 0.001. A subset of the participants (n = 56) also had verbal IQ estimated by NAART. In this subset of participants, the partial correlation of connectivity with MSCEIT score with VIQ as a covariate remained highly significant r = 0.555, p < 0.001.
As reported above (section “Seed Based Connectivity Analyses”) we observed a significant inverse correlation between prescribed CPZE dosage and MSCEIT score. We observed the same cerebellar–parietal connectivity-cognition relationship in all diagnostic subgroups (including neurotypical participants not taking antipsychotics) making it unlikely that observed connectivity is caused by medication effects. We calculated the partial correlation between parietal–cerebellar connectivity and MSCEIT with CPZE regressed out as a covariate. We continued to observe the same strong correlation between connectivity and MSCEIT score r = 0.364, p < 0.001. We also regressed maps of connectivity to the parietal ROI against MSCEIT score with CPZE as an additional covariate (in addition to age, sex, and site) and continued to identify a significant correlation to the right cerebellum (Supplementary Figure 2), albeit at a lower voxelwise significance threshold (p < 0.005).
Discussion
We present the results of our efforts to identify brain circuit correlates of social cognition. Our approach included a trans-diagnostic cohort of neurotypical adults and participants with psychotic disorders. As predicted, participants with psychosis exhibited significant impairment in social cognition compared to controls. We then used a fully data driven analysis of task-free connectivity at the individual voxel level to find the strongest correlates of social cognitive ability. This approach determined that functional connectivity between left superior parietal cortex and other nodes of the DMN are positively correlated with social cognitive ability. A link between cognition and SPL connectivity was observed in bilateral nodes of the DMN but there was a laterality to the strongest result observed. Specifically, the strongest relationship between functional connectivity and social cognitive ability was observed in a circuit between right cerebellar Crus I, II and left superior parietal cortex. The relationship between cognition and connectivity at those nodes was trans-diagnostic and observed in both neurotypical participants as well as those with psychotic disorders, despite the participants with psychotic disorders performing, on average, a full standard deviation worse than neurotypical adults. This is consistent with a model in which cerebellar–parietal connectivity mediates the relationship between diagnosis and social cognitive ability. This observation is in line with a recent consensus report highlighting the role of the cerebellum in social cognition (68). Interestingly, a recent large study in SZ found robust reductions in cerebellar gray matter volume with the strongest effects in regions that were functionally connected with frontoparietal cortical regions (69) suggesting that not only is cerebellar–parietal connectivity linked to social cognitive processing, but that it is strongly associated with abnormalities in psychosis.
Historically, hypothesis driven neuroimaging has focused on the prefrontal cortex in studies involving complex cognition such as working memory and social reasoning. How can our result be reconciled with the extant literature? Strikingly, this discovery is entirely consistent with prior findings in both human disorders of social cognition (e.g., autism) and in murine models. Case-control studies in ASD have consistently identified abnormalities in the Crus I, II region of the cerebellum but the functional consequence of this finding had been unclear. More recently, through innovative experiments, Stoodley et al. demonstrated that cerebellar neuromodulation in humans can manipulate cerebellar–parietal connectivity. Those investigators were able to extend this result by demonstrating with direct recording that right Crus I Purkinje neurons modulate activity in mouse parietal association cortex (48). Both that study and a subsequent paper demonstrated a critical role for Crus I in social preference in mice (48, 70).
Here we expand on these studies in two critical ways: First, while prior studies demonstrated R Crus I of the cerebellum can modulate parietal activity in humans, we demonstrate that communication between cerebellum and parietal lobe is directly related to human social cognitive ability. Second, we demonstrate that this circuit can account for individual variance in social cognitive ability in disorders of impaired social cognition (e.g., SZ) as well as in neurotypical humans.
Of particular note, we arrive at this circuit using a whole-brain, data-driven analysis, i.e., without limiting ourselves a priori to these candidate regions. This circuit is identified as the strongest link to social cognition in our sample. Thus, we observe a convergence of results from independent data in humans and mice identifying a trans-diagnostic and trans-species cerebellar-cortical circuit with evidence of a causal link to social cognitive ability.
We suggest that this convergence of results is also a product of the analytic approach used here. Specifically: The participants of this sample represented a spectrum of social cognitive ability. That variance is presumably linked to a variety of underlying causes, i.e., some participants had social cognitive deficits linked to a primarily genetic disorder (SZ) and other participants whose abilities represent normal population variation not linked to the genetic causes of SZ. In finding a common brain substrate for social cognitive ability irrespective of etiology, we propose that this connectivity-cognition link may be common pathway mediating social cognitive ability. That is, a circuit casually linked to cognition rather than epiphenomena linked to disease severity.
The results presented in these studies link circuit connectivity to social cognition as measured by tests in laboratory conditions. However, data suggest that there may be real-world outcomes linked to this circuit as well. Smith et al. analyzed human connectome project data using canonical correlation analysis to link a wide range of tests and life experiences to functional connectivity (71). This analysis revealed a broad range of outcomes organized along a “positive–negative” axis (e.g., life satisfaction as a positive outcome, and THC use as a negative outcome). Strikingly, the strongest brain link to this axis was functional connectivity between cerebellar Crus I, II and parietal lobule.
These results in murine behavioral tests, human social cognitive test performance, and real-world outcomes are independently derived but all converge on the same consensus cerebellar–parietal circuit. This allows the construction of an empirically derived model in which social cognition is critically dependent on this cerebellar-cortical circuit function (Figure 3).
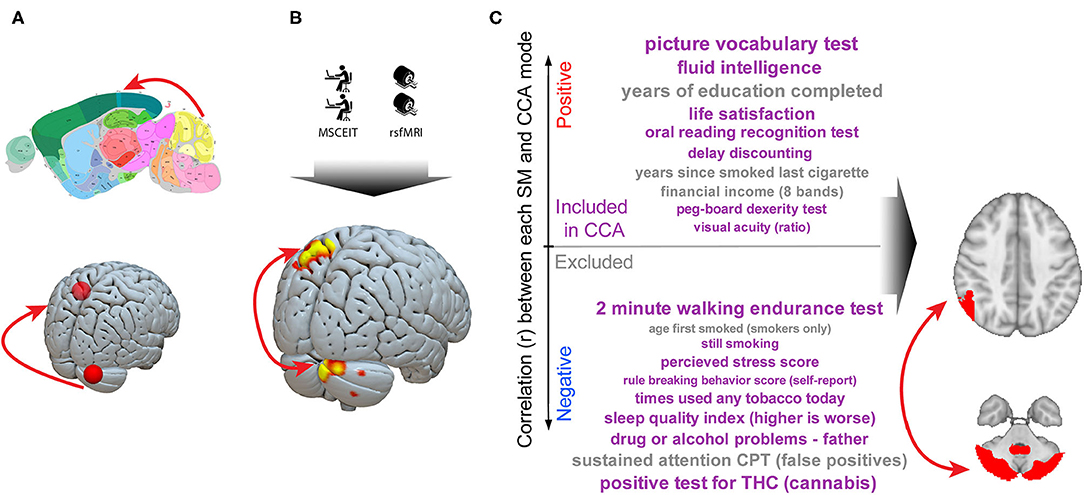
Figure 3. A cerebellar–parietal circuit is causally linked to social cognition both trans-diagnostically and trans-species. A series of murine and human experiments converge on a shared circuit causally linked to social cognition. (A) Imaging studies reliably identify cerebellar right Crus abnormalities in autism. Neuromodulation experiments in humans identify a circuit linking right Crus to the left parietal lobe and murine studies demonstrate this circuit is critical to normal social interaction (48). (B) We observe that, in a trans-diagnostic sample, connectivity between right cerebellar Crus and left parietal lobe connectivity is directly linked to social cognitive ability. (C) In a large (n = 461) dataset, connectivity in this cerebellar Crus–parietal circuit was the strongest link between a broad array of outcomes along a “positive–negative axis” (71). Taken together, these data are consistent with a critical role for a cerebellar–cortical circuit in complex social cognition. Murine image from the Allen Mouse Brain Atlas.
Prior evidence for a cerebellar role in organized cognition has come from lesions and correlational imaging studies (72). A wealth of recent murine studies has demonstrated a critical role for the cerebellum in multiple aspects of cognition (73–77). In this model we add social cognition to the growing list of cognitive domains dependent on cerebellar computation. In particular, the MSCEIT-ME branch requires participants to listen to vignettes and make predictions about the emotional or social consequences of various possible actions; the association between performance on this task and the identified cerebellar–parietal circuit is consistent with findings that the cerebellum, and specifically Crus I and II, plays a role in social cognition via social prediction (68).
What is the relevance of this result to disease? The evidence presented here link social cognitive impairment in psychotic disorders to this circuit. We previously demonstrated, in an independent data set, that hypoconnectivity in a cerebellar–Dorso-Lateral Pre-Frontal Cortex (DLPFC) circuit is causally linked to negative symptoms (e.g., apathy) in SZ (78). The cerebellar node of that circuit is the same Crus I, II region we link to social cognition in the current study. Specifically: connectivity between this Crus I, II region and different cortical regions is linked to different deficits in SZ: the left parietal lobe for social cognition and the right DLPFC for negative symptoms. This allows a mechanistic model for the co-occurrence of these deficits in SZ: Distinct deficits result from dysconnectivity in specific circuits, but all of these circuits have a shared node in the cerebellum.
Importantly, these findings have implications for targeted interventions to improve social cognitive functioning in people across diagnostic boundaries. Our study using transcranial magnetic stimulation (TMS) to target a cerebellar-cortical circuit associated with negative symptoms in psychosis found that neuromodulation at the cerebellar site was associated with both increased connectivity and reduction in negative symptoms (78). Our findings, together with others [e.g., (48)], identify a potential neural target for improving social cognition that may be both modifiable and associated with downstream pro-cognitive effects.
One limitation of our study was the use of a single test of social cognitive ability. The MSCEIT-ME test included in the MATRICS consensus cognitive battery was designed to measure a specific aspect of social cognition, higher-order emotional reasoning regarding emotion management and regulation, and does not measure other social cognitive domains such as theory of mind or emotion perception. That said, the managing emotions domain of MSCEIT-ME is linked to real world functional outcomes (79) and the broad adoption of the MATRICS allowed consolidation of samples from across multiple sites (80). The MSCEIT-ME branch was also the only branch of the MSCEIT in which people with psychosis continued to differ from controls after controlling for general cognitive ability (81), suggesting that it is tapping emotional intelligence in a way that is at least partially distinct from general cognitive skills. Additionally, the MSCEIT-ME was among the MSCEIT branches most strongly associated with brain volume measures in people with SZ and related disorders (82). However, associations between this circuit and other domains of social and emotional processing remain to be determined. Another limitation is that we did not have uniform data on social or other functional outcomes across the sample and were therefore unable to evaluate effects of our findings on real-world social functioning.
Despite these limitations, the convergence of results linking social cognition to a cerebellar–parietal circuit (Figure 3) argues (1) dysfunction in this circuit is linked to social cognition trans-diagnostically in psychotic disorders and (2) at the circuit level these deficits lie along a continuum with variation in social cognitive ability in a neurotypical population. Future studies can determine if individual variation in social cognitive ability in ASDs covaries with cerebellar–parietal connectivity. Evidence from murine experiments are consistent with a causal relationship between this circuit and social cognition. From a basic science perspective, the convergence of human and murine findings suggest that this circuit is a valid candidate for modeling how circuit dysfunction gives rise to social cognitive phenotypes in psychiatric disorders. Therapeutically, prior work has established that this circuit can be manipulated non-invasively (48) making it a promising candidate target for interventions designed to ameliorate social cognitive deficits.
Data Availability Statement
The raw data supporting the conclusions of this article will be made available by the authors, without undue reservation.
Ethics Statement
These studies were reviewed and approved by the University of Pittsburgh, BIDMC, and McLean Hospital IRBs.
Author Contributions
RB was involved in all aspects of this project including study design, statistical analyses, development of tables and figures, and drafting of the manuscript. AB was involved in statistical analyses, development of figures, and drafting of the results, and methods. MN was involved in data collection and analysis. SE was involved in development of studies in which the data were collected. RM-G was involved in design and interpretation of the cognitive data. MK was involved in development of studies in which the data were collected and the analysis plan. KL was involved in development of studies in which the data were collected, development of the present project, and drafting of the manuscript. All authors reviewed and approved the final version.
Funding
This work was supported by grants from the National Institute of Mental Health: R01MH116170 (RB), R01MH92440 (MK and SE), and R01MH117012 (KL).
Conflict of Interest
The authors declare that the research was conducted in the absence of any commercial or financial relationships that could be construed as a potential conflict of interest.
Acknowledgments
We wish to thank Ivy Lee for help with figure creation.
Supplementary Material
The Supplementary Material for this article can be found online at: https://www.frontiersin.org/articles/10.3389/fpsyt.2020.573002/full#supplementary-material
References
1. Mercer L, Becerra R. A unique emotional processing profile of euthymic bipolar disorder? A critical review. J Affect Disord. (2013) 146:295–309. doi: 10.1016/j.jad.2012.10.030
2. Penn DL, Sanna LJ, Roberts DL. Social cognition in schizophrenia: an overview. Schizophr Bull. (2008) 34:408–11. doi: 10.1093/schbul/sbn014
3. Green MF, Horan WP, Lee J. Social cognition in schizophrenia. Nat Rev Neurosci. (2015) 16:620–31. doi: 10.1038/nrn4005
4. Brekke J, Kay DD, Lee KS, Green MF. Biosocial pathways to functional outcome in schizophrenia. Schizophr Res. (2005) 80:213–25. doi: 10.1016/j.schres.2005.07.008
5. Horan WP, Green MF, Degroot M, Fiske A, Hellemann G, Kee K, et al. Social cognition in schizophrenia, Part 2: 12-month stability and prediction of functional outcome in first-episode patients. Schizophr Bull. (2012) 38:865–72. doi: 10.1093/schbul/sbr001
6. Kee KS, Green MF, Mintz J, Brekke JS. Is emotion processing a predictor of functional outcome in schizophrenia? Schizophr Bull. (2003) 29:487–97. doi: 10.1093/oxfordjournals.schbul.a007021
7. Ospina LH, Nitzburg GC, Shanahan M, Perez-Rodriguez MM, Larsen E, Latifoglu A, et al. Social cognition moderates the relationship between neurocognition and community functioning in bipolar disorder. J Affect Disord. (2018) 235:7–14. doi: 10.1016/j.jad.2018.03.013
8. Samame C, Martino DJ, Strejilevich SA. An individual task meta-analysis of social cognition in euthymic bipolar disorders. J Affect Disord. (2015) 173:146–53. doi: 10.1016/j.jad.2014.10.055
9. Van Rheenen TE, Rossell SL. Is the non-verbal behavioural emotion-processing profile of bipolar disorder impaired? A critical review. Acta Psychiatr Scand. (2013) 128:163–78. doi: 10.1111/acps.12125
10. Bora E, Pantelis C. Social cognition in schizophrenia in comparison to bipolar disorder: a meta-analysis. Schizophr Res. (2016) 175:72–8. doi: 10.1016/j.schres.2016.04.018
11. Varo C, Jimenez E, Sole B, Bonnin CM, Torrent C, Lahera G, et al. Social cognition in bipolar disorder: the role of sociodemographic, clinical, and neurocognitive variables in emotional intelligence. Acta Psychiatr Scand. (2019) 139:369–80. doi: 10.1111/acps.13014
12. Kohler CG, Walker JB, Martin EA, Healey KM, Moberg PJ. Facial emotion perception in schizophrenia: a meta-analytic review. Schizophr Bull. (2010) 36:1009–19. doi: 10.1093/schbul/sbn192
13. Sprong M, Schothorst P, Vos E, Hox J, Van Engeland H. Theory of mind in schizophrenia: meta-analysis. Br J Psychiatry. (2007) 191:5–13. doi: 10.1192/bjp.bp.107.035899
14. Bora E, Yucel M, Pantelis C. Theory of mind impairment in schizophrenia: meta-analysis. Schizophr Res. (2009) 109:1–9. doi: 10.1016/j.schres.2008.12.020
15. Bora E, Bartholomeusz C, Pantelis C. Meta-analysis of Theory of Mind (ToM) impairment in bipolar disorder. Psychol Med. (2016) 46:253–64. doi: 10.1017/S0033291715001993
16. Lahera G, Herrera S, Reinares M, Benito A, Rullas M, Gonzalez-Cases J, et al. Hostile attributions in bipolar disorder and schizophrenia contribute to poor social functioning. Acta Psychiatr Scand. (2015) 131:472–82. doi: 10.1111/acps.12399
17. Lyon HM, Startup M, Bentall RP. Social cognition and the manic defense: attributions, selective attention, and self-schema in bipolar affective disorder. J Abnorm Psychol. (1999) 108:273–82. doi: 10.1037/0021-843X.108.2.273
18. Mehl S, Landsberg MW, Schmidt AC, Cabanis M, Bechdolf A, Herrlich J, et al. Why do bad things happen to me? Attributional style, depressed mood, and persecutory delusions in patients with schizophrenia. Schizophr Bull. (2014) 40:1338–46. doi: 10.1093/schbul/sbu040
19. Dawson S, Kettler L, Burton C, Galletly C. Do people with schizophrenia lack emotional intelligence? Schizophr Res Treatment. (2012) 2012:495174. doi: 10.1155/2012/495174
20. Frajo-Apor B, Kemmler G, Pardeller S, Plass T, Muhlbacher M, Welte AS, et al. Emotional intelligence and non-social cognition in schizophrenia and bipolar I disorder. Psychol Med. (2017) 47:35–42. doi: 10.1017/S0033291716002324
21. Ruocco AC, Reilly JL, Rubin LH, Daros AR, Gershon ES, Tamminga CA, et al. Emotion recognition deficits in schizophrenia-spectrum disorders and psychotic bipolar disorder: findings from the Bipolar-Schizophrenia Network on Intermediate Phenotypes (B-SNIP) study. Schizophr Res. (2014) 158:105–12. doi: 10.1016/j.schres.2014.07.001
22. Fernandes JM, Cajao R, Lopes R, Jeronimo R, Barahona-Correa JB. Social cognition in schizophrenia and autism spectrum disorders: a systematic review and meta-analysis of direct comparisons. Front Psychiatry. (2018) 9:504. doi: 10.3389/fpsyt.2018.00504
23. Pinkham AE, Morrison KE, Penn DL, Harvey PD, Kelsven S, Ludwig K, et al. Comprehensive comparison of social cognitive performance in autism spectrum disorder and schizophrenia. Psychol Med. (2019) 1–9. doi: 10.1017/S0033291719002708
24. Eack SM, Bahorik AL, Mcknight SA, Hogarty SS, Greenwald DP, Newhill CE, et al. Commonalities in social and non-social cognitive impairments in adults with autism spectrum disorder and schizophrenia. Schizophr Res. (2013) 148:24–8. doi: 10.1016/j.schres.2013.05.013
25. Pinkham AE, Hopfinger JB, Pelphrey KA, Piven J, Penn DL. Neural bases for impaired social cognition in schizophrenia and autism spectrum disorders. Schizophr Res. (2008) 99:164–75. doi: 10.1016/j.schres.2007.10.024
26. Radeloff D, Ciaramidaro A, Siniatchkin M, Hainz D, Schlitt S, Weber B, et al. Structural alterations of the social brain: a comparison between schizophrenia and autism. PLoS ONE. (2014) 9:e106539. doi: 10.1371/journal.pone.0106539
27. Sugranyes G, Kyriakopoulos M, Corrigall R, Taylor E, Frangou S. Autism spectrum disorders and schizophrenia: meta-analysis of the neural correlates of social cognition. PLoS ONE. (2011) 6:e25322. doi: 10.1371/journal.pone.0025322
28. Nair A, Jolliffe M, Lograsso YSS, Bearden CE. A review of default mode network connectivity and its association with social cognition in adolescents with autism spectrum disorder and early-onset psychosis. Front Psychiatry. (2020) 11:614. doi: 10.3389/fpsyt.2020.00614
29. Hyatt CJ, Calhoun VD, Pittman B, Corbera S, Bell MD, Rabany L, et al. Default mode network modulation by mentalizing in young adults with autism spectrum disorder or schizophrenia. Neuroimage Clin. (2020) 27:102343. doi: 10.1016/j.nicl.2020.102343
30. Chen H, Uddin LQ, Duan X, Zheng J, Long Z, Zhang Y, et al. Shared atypical default mode and salience network functional connectivity between autism and schizophrenia. Autism Res. (2017) 10:1776–86. doi: 10.1002/aur.1834
31. Eack SM, Wojtalik JA, Keshavan MS, Minshew NJ. Social-cognitive brain function and connectivity during visual perspective-taking in autism and schizophrenia. Schizophr Res. (2017) 183:102–9. doi: 10.1016/j.schres.2017.03.009
32. Kronbichler L, Tschernegg M, Martin AI, Schurz M, Kronbichler M. Abnormal brain activation during theory of mind tasks in schizophrenia: a meta-analysis. Schizophr Bull. (2017) 43:1240–50. doi: 10.1093/schbul/sbx073
33. Olley AL, Malhi GS, Bachelor J, Cahill CM, Mitchell PB, Berk M. Executive functioning and theory of mind in euthymic bipolar disorder. Bipolar Disord. (2005) 7(Suppl. 5):43–52. doi: 10.1111/j.1399-5618.2005.00254.x
34. Andreasen NC, Pierson R. The role of the cerebellum in schizophrenia. Biol Psychiatry. (2008) 64:81–8. doi: 10.1016/j.biopsych.2008.01.003
35. Ho BC, Mola C, Andreasen NC. Cerebellar dysfunction in neuroleptic naive schizophrenia patients: clinical, cognitive, and neuroanatomic correlates of cerebellar neurologic signs. Biol Psychiatry. (2004) 55:1146–53. doi: 10.1016/j.biopsych.2004.02.020
36. Rasser PE, Schall U, Peck G, Cohen M, Johnston P, Khoo K, et al. Cerebellar grey matter deficits in first-episode schizophrenia mapped using cortical pattern matching. Neuroimage. (2010) 53:1175–80. doi: 10.1016/j.neuroimage.2010.07.018
37. Mothersill O, Knee-Zaska C, Donohoe G. Emotion and theory of mind in schizophrenia-investigating the role of the cerebellum. Cerebellum. (2016) 15:357–68. doi: 10.1007/s12311-015-0696-2
38. Moberget T, Ivry RB. Prediction, psychosis, and the cerebellum. Biol Psychiatry Cogn Neurosci Neuroimaging. (2019) 4:820–31. doi: 10.1016/j.bpsc.2019.06.001
39. Skefos J, Cummings C, Enzer K, Holiday J, Weed K, Levy E, et al. Regional alterations in Purkinje cell density in patients with autism. PLoS ONE. (2014) 9:E81255. doi: 10.1371/journal.pone.0081255
40. D'Mello AM, Crocetti D, Mostofsky SH, Stoodley CJ. Cerebellar gray matter and lobular volumes correlate with core autism symptoms. Neuroimage Clin. (2015) 7:631–9. doi: 10.1016/j.nicl.2015.02.007
41. Mosconi MW, Mohanty S, Greene RK, Cook EH, Vaillancourt DE, Sweeney JA. Feedforward and feedback motor control abnormalities implicate cerebellar dysfunctions in autism spectrum disorder. J Neurosci. (2015) 35:2015–25. doi: 10.1523/JNEUROSCI.2731-14.2015
42. Whitney ER, Kemper TL, Bauman ML, Rosene DL, Blatt GJ. Cerebellar Purkinje cells are reduced in a subpopulation of autistic brains: a stereological experiment using calbindin-D28k. Cerebellum. (2008) 7:406–16. doi: 10.1007/s12311-008-0043-y
43. Van Overwalle F, Baetens K, Marien P, Vandekerckhove M. Social cognition and the cerebellum: a meta-analysis of over 350 fMRI studies. Neuroimage. (2014) 86:554–72. doi: 10.1016/j.neuroimage.2013.09.033
44. Schmahmann JD, Caplan D. Cognition, emotion and the cerebellum. Brain. (2006) 129(Pt 2):290–2. doi: 10.1093/brain/awh729
45. Schmahmann JD, Sherman JC. The cerebellar cognitive affective syndrome. Brain. (1998) 121(Pt 4):561–79. doi: 10.1093/brain/121.4.561
46. Stoodley CJ, Schmahmann JD. Functional topography in the human cerebellum: a meta-analysis of neuroimaging studies. Neuroimage. (2009) 44:489–501. doi: 10.1016/j.neuroimage.2008.08.039
47. Andreasen NC, Calarge CA, O'Leary DS. Theory of mind and schizophrenia: a positron emission tomography study of medication-free patients. Schizophr Bull. (2008) 34:708–19. doi: 10.1093/schbul/sbn034
48. Stoodley CJ, D'Mello AM, Ellegood J, Jakkamsetti V, Liu P, Nebel MB, et al. Altered cerebellar connectivity in autism and cerebellar-mediated rescue of autism-related behaviors in mice. Nat Neurosci. (2017) 20:1744–51. doi: 10.1038/s41593-017-0004-1
49. Catsman-Berrevoets CE, Aarsen FK. The spectrum of neurobehavioural deficits in the posterior fossa syndrome in children after cerebellar tumour surgery. Cortex. (2010) 46:933–46. doi: 10.1016/j.cortex.2009.10.007
50. Tsai PT, Hull C, Chu Y, Greene-Colozzi E, Sadowski AR, Leech JM, et al. Autistic-like behaviour and cerebellar dysfunction in Purkinje cell Tsc1 mutant mice. Nature. (2012) 488:647–51. doi: 10.1038/nature11310
51. Ling G, Lee I, Guimond S, Lutz O, Tandon N, Nawaz U, et al. Individual variation in brain network topology is linked to emotional intelligence. Neuroimage. (2019) 189:214–23. doi: 10.1016/j.neuroimage.2019.01.013
52. First MB New York State Psychiatric Institute. Biometrics Research D. Structured Clinical Interview For DSM-IV-TR Axis I Disorders: SCID-I. New York, NY: Biometrics Research Department, New York State Psychiatric Institute (2007).
53. Hays JR, Reas DL, Shaw JB. Concurrent validity of the Wechsler abbreviated scale of intelligence and the Kaufman brief intelligence test among psychiatric inpatients. Psychological Reports. (2002) 90:355–9. doi: 10.2466/pr0.2002.90.2.355
54. First MB. Structured Clinical Interview for DSM-IV Axis I Disorders: Patient Edition (February 1996 Final), SCID-I/P. New York, NY: Biometrics Research Department, New York State Psychiatric Institute (1998).
55. Kern RS, Nuechterlein KH, Green MF, Baade LE, Fenton WS, Gold JM, et al. The MATRICS consensus cognitive battery, part 2: co-norming and standardization. Am J Psychiatry. (2008) 165:214–20. doi: 10.1176/appi.ajp.2007.07010043
56. Nuechterlein KH, Green MF, Kern RS, Baade LE, Barch DM, Cohen JD, et al. The MATRICS consensus cognitive battery, part 1: test selection, reliability, and validity. Am J Psychiatry. (2008) 165:203–13. doi: 10.1176/appi.ajp.2007.07010042
57. Mayer JD, Salovey P, Caruso DR, Sitarenios G. Measuring emotional intelligence with the MSCEIT V2.0. Emotion. (2003) 3:97–105. doi: 10.1037/1528-3542.3.1.97
58. Yan CG, Wang XD, Zuo XN, Zang YF. DPABI: data processing & analysis for (resting-state) brain imaging. Neuroinformatics. (2016) 14:339–51. doi: 10.1007/s12021-016-9299-4
59. Ashburner J. A fast diffeomorphic image registration algorithm. Neuroimage. (2007) 38:95–113. doi: 10.1016/j.neuroimage.2007.07.007
60. Friston KJ, Williams S, Howard R, Frackowiak RS, Turner R. Movement-related effects in fMRI time-series. Magn Reson Med. (1996) 35:346–55. doi: 10.1002/mrm.1910350312
61. Yan CG, Cheung B, Kelly C, Colcombe S, Craddock RC, Di Martino A, et al. A comprehensive assessment of regional variation in the impact of head micromovements on functional connectomics. Neuroimage. (2013) 76:183–201. doi: 10.1016/j.neuroimage.2013.03.004
62. Power JD, Barnes KA, Snyder AZ, Schlaggar BL, Petersen SE. Spurious but systematic correlations in functional connectivity MRI networks arise from subject motion. Neuroimage. (2012) 59:2142–54. doi: 10.1016/j.neuroimage.2011.10.018
63. Shehzad Z, Kelly C, Reiss PT, Cameron Craddock R, Emerson JW, Mcmahon K, et al. A multivariate distance-based analytic framework for connectome-wide association studies. Neuroimage. (2014) 93(Pt 1):74–94. doi: 10.1016/j.neuroimage.2014.02.024
64. Sharma A, Wolf DH, Ciric R, Kable JW, Moore TM, Vandekar SN, et al. Common dimensional reward deficits across mood and psychotic disorders: a connectome-wide association study. Am J Psychiatry. (2017) 174:657–66. doi: 10.1176/appi.ajp.2016.16070774
65. Shanmugan S, Wolf DH, Calkins ME, Moore TM, Ruparel K, Hopson RD, et al. Common and dissociable mechanisms of executive system dysfunction across psychiatric disorders in youth. Am J Psychiatry. (2016) 173:517–26. doi: 10.1176/appi.ajp.2015.15060725
66. Satterthwaite TD, Vandekar SN, Wolf DH, Bassett DS, Ruparel K, Shehzad Z, et al. Connectome-wide network analysis of youth with psychosis-spectrum symptoms. Mol Psychiatry. (2015) 20:1508–15. doi: 10.1038/mp.2015.66
67. Zapala MA, Schork NJ. Multivariate regression analysis of distance matrices for testing associations between gene expression patterns and related variables. Proc Natl Acad Sci USA. (2006) 103:19430–5. doi: 10.1073/pnas.0609333103
68. Van Overwalle F, Manto M, Cattaneo Z, Clausi S, Ferrari C, Gabrieli JDE, et al. Consensus paper: cerebellum and social cognition. Cerebellum. (2020) 19:833–68. doi: 10.1007/s12311-020-01155-1. [Epub ahead of print].
69. Moberget T, Doan NT, Alnaes D, Kaufmann T, Cordova-Palomera A, Lagerberg TV, et al. Cerebellar volume and cerebellocerebral structural covariance in schizophrenia: a multisite mega-analysis of 983 patients and 1349 healthy controls. Mol Psychiatry. (2018) 23:1512–20. doi: 10.1038/mp.2017.106
70. Badura A, Verpeut JL, Metzger JW, Pereira TD, Pisano TJ, Deverett B, et al. Normal cognitive and social development require posterior cerebellar activity. Elife. (2018) 7:e36401. doi: 10.7554/eLife.36401
71. Smith SM, Nichols TE, Vidaurre D, Winkler AM, Behrens TE, Glasser MF, et al. A positive–negative mode of population covariation links brain connectivity, demographics and behavior. Nat Neurosci. (2015) 18:1565–7. doi: 10.1038/nn.4125
72. Schmahmann JD, Guell X, Stoodley CJ, Halko MA. The theory and neuroscience of cerebellar cognition. Annu Rev Neurosci. (2019) 42:337–64. doi: 10.1146/annurev-neuro-070918-050258
73. Wagner MJ, Kim TH, Savall J, Schnitzer MJ, Luo L. Cerebellar granule cells encode the expectation of reward. Nature. (2017) 544:96–100. doi: 10.1038/nature21726
74. Wagner MJ, Kim TH, Kadmon J, Nguyen ND, Ganguli S, Schnitzer MJ, et al. Shared cortex-cerebellum dynamics in the execution and learning of a motor task. Cell. (2019) 177:669–82.e24. doi: 10.1016/j.cell.2019.02.019
75. Carta I, Chen CH, Schott AL, Dorizan S, Khodakhah K. Cerebellar modulation of the reward circuitry and social behavior. Science. (2019) 363:eaav0581. doi: 10.1126/science.aav0581
76. Tantra M, Guo L, Kim J, Zainolabidin N, Eulenburg V, Augustine GJ, et al. Conditional deletion of Cadherin 13 perturbs Golgi cells and disrupts social and cognitive behaviors. Genes Brain Behav. (2018) 17:E12466. doi: 10.1111/gbb.12466
77. Jackman SL, Chen CH, Offermann HL, Drew IR, Harrison BM, Bowman AM, et al. Cerebellar Purkinje cell activity modulates aggressive behavior. Elife. (2020) 9:e53229. doi: 10.7554/eLife.53229
78. Brady RO Jr, Gonsalvez I, Lee I, Ongur D, Seidman LJ, Schmahmann JD, et al. Cerebellar–prefrontal network connectivity and negative symptoms in schizophrenia. Am J Psychiatry. (2019) 176:512–20. doi: 10.1176/appi.ajp.2018.18040429
79. Detore NR, Mueser KT, Mcgurk SR. What does the managing emotions branch of the MSCEIT add to the MATRICS consensus cognitive battery? Schizophr Res. (2018) 197:414–20. doi: 10.1016/j.schres.2018.02.018
80. Green MF, Olivier B, Crawley JN, Penn DL, Silverstein S. Social cognition in schizophrenia: recommendations from the measurement and treatment research to improve cognition in schizophrenia new approaches conference. Schizophr Bull. (2005) 31:882–7. doi: 10.1093/schbul/sbi049
81. Frajo-Apor B, Pardeller S, Kemmler G, Welte AS, Hofer A. Emotional intelligence deficits in schizophrenia: the impact of non-social cognition. Schizophr Res. (2016) 172:131–6. doi: 10.1016/j.schres.2016.02.027
Keywords: bipolar disorder, schizophrenia, psychosis, connectivity, social cognition, cerebellum, imaging, resting state
Citation: Brady RO Jr, Beermann A, Nye M, Eack SM, Mesholam-Gately R, Keshavan MS and Lewandowski KE (2020) Cerebellar-Cortical Connectivity Is Linked to Social Cognition Trans-Diagnostically. Front. Psychiatry 11:573002. doi: 10.3389/fpsyt.2020.573002
Received: 15 June 2020; Accepted: 05 October 2020;
Published: 04 November 2020.
Edited by:
Tim Ziermans, University of Amsterdam, NetherlandsReviewed by:
Aarti Nair, University of California, Los Angeles, United StatesAmy Pinkham, The University of Texas at Dallas, United States
Copyright © 2020 Brady, Beermann, Nye, Eack, Mesholam-Gately, Keshavan and Lewandowski. This is an open-access article distributed under the terms of the Creative Commons Attribution License (CC BY). The use, distribution or reproduction in other forums is permitted, provided the original author(s) and the copyright owner(s) are credited and that the original publication in this journal is cited, in accordance with accepted academic practice. No use, distribution or reproduction is permitted which does not comply with these terms.
*Correspondence: Roscoe O. Brady Jr, cm9icmFkeSYjeDAwMDQwO2JpZG1jLmhhcnZhcmQuZWR1
†These authors have contributed equally to this work