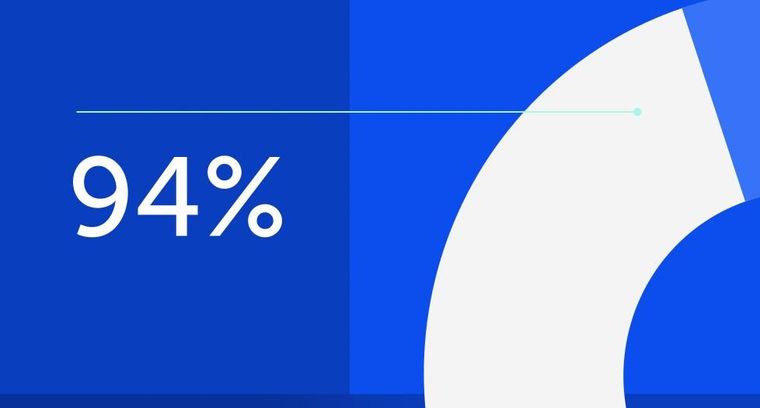
94% of researchers rate our articles as excellent or good
Learn more about the work of our research integrity team to safeguard the quality of each article we publish.
Find out more
SYSTEMATIC REVIEW article
Front. Psychiatry, 10 July 2020
Sec. Child and Adolescent Psychiatry
Volume 11 - 2020 | https://doi.org/10.3389/fpsyt.2020.00632
This article is part of the Research TopicChildren, Adolescents and Families with Severe Mental Illness: Towards a Comprehensive Early Identification of RiskView all 22 articles
Children, adolescents, and young adults with at least one first-degree relative [familial high-risk (FHR)] with either schizophrenia (SZ) or bipolar disorder (BD) have a one-in-two risk of developing a psychiatric disorder. Here, we review functional magnetic resonance imaging (fMRI) studies which examined task-related brain activity in young individuals with FHR-SZ and FHR-BD. A systematic search identified all published task-related fMRI studies in children, adolescents, and young adults below an age of 27 years with a first-degree relative with SZ or BD, but without manifest psychotic or affective spectrum disorder themselves. The search identified 19 cross-sectional fMRI studies covering four main cognitive domains: 1) working memory (n = 3), 2) cognitive control (n = 4), 3) reward processing (n = 3), and 4) emotion processing (n = 9). Thirteen studies included FHR-BD, five studies included FHR-SZ, and one study included a pooled FHR group. In general, task performance did not differ between the respective FHR groups and healthy controls, but 18 out of the 19 fMRI studies revealed regional alterations in task-related activation. Brain regions showing group differences in peak activation were regions associated with the respective task domain and showed little overlap between FHR-SZ and FHR-BD. The low number of studies, together with the low number of subjects, and the substantial heterogeneity of employed methodological approaches within the domain of working memory, cognitive control, and reward processing impedes finite conclusions. Emotion processing was the most investigated task domain in FHR-BD. Four studies reported differences in activation of the amygdala, and two studies reported differences in activation of inferior frontal/middle gyrus. Together, these studies provide evidence for altered brain processing of emotions in children, adolescents, and young adults at FHR-BD. More studies of higher homogeneity, larger sample sizes and with a longitudinal study design are warranted to prove a shared or specific FHR-related endophenotypic brain activation in young first-degree relatives of individuals with SZ or BD, as well as to pinpoint specific alterations in brain activation during cognitive-, emotional-, and reward-related tasks.
Schizophrenia (SZ) and bipolar disorder (BD) are severe and highly heritable (1) mental illnesses with a substantial impact on the individuals concerned, their families, and the society. By early adulthood, the offspring of parents with severe mental illnesses, including SZ, BD, and major depressive disorder, have a one-in-three risk of developing a psychotic or major mood disorder and a one-in-two risk of developing any mental disorder (2). Heritability shows partial phenotypical specificity with largest risk ratios for SZ among offspring of parents with SZ and largest risk ratios for BD among offspring of parents with BD. Additionally, offspring of parents with SZ have a significantly increased risk of BD and offspring of parents with BD have a significantly increased risk of SZ (2). According to data extracted from Danish registries, child and adolescent offspring of parents with severe mental illness express increased incidence rates for all diagnoses of child and adolescent mental disorders compared to reference offspring of parents without severe mental illness (3).
SZ is characterized as a neurodevelopmental disorder and manifests in adolescence or early adulthood (4, 5), whereas the developmental nature of BD is less understood (6). However, genome-wide association studies of SZ and BD have shown overlapping genetic risk loci (7, 8). Approximately, two-thirds of the genetic expression profile are shared across SZ and BD (9, 10). Thus, the genetic risk profile for SZ and BD may also be shared across the offspring of parents with these severe mental illnesses. Whether these disorders also share phenotypic expression profiles, e.g., brain responses, during early stages of pathogenesis is unclear.
First-degree relatives of individuals with SZ or BD, referred to as individuals with familial high-risk (FHR), show impairments on a variety of neurocognitive and motor functions on a group level, even at young age (11–17). Moreover, deficits in neurocognitive functioning in individuals with the manifest disorder and in adult FHR individuals have been linked to altered brain functioning and have been suggested as endophenotypic of the disorders (18–22). This, in turn, may reflect an increasing dysfunction during brain maturation in critical brain regions. Various childhood mental disorders including ADHD, autism, and childhood onset schizophrenia (COS) are associated with abnormal developmental trajectories for cortical thickness (23). Interestingly, healthy siblings of patients with COS show significant reductions in regional gray and white matter volume, suggesting a trait marker (24, 25). In keeping with this, characteristics of cortical morphology in child and adolescent offspring of SZ patients show cross-sectional decrease in global and parieto-occipital surface area compared to a control group, and a decrease in occipital surface area compared to offspring of BD patients (26). In that study, global and parietal surface area scaled with the expression of positive and negative prodromal symptoms in offsprings of SZ patients (26).
Throughout post-natal development, the brain undergoes continuous maturation (27–29). This is also the case for the frontal cortex, (30), but the maturational trajectories of frontal cortical areas differ from the trajectories of other inter-connected brain regions, such as the basal ganglia (31, 32). This discrepancy may render the brain vulnerable and favor the emergence of mental disorders during adolescence and early adulthood (32, 33).
Studies on the brain development in young individuals at high risk of severe mental disorders may not only reveal underlying neurobiological mechanisms but also identify markers associated with risk and resilience. Multiple studies of FHR individuals have investigated cognitive, motor or social capabilities in vulnerable populations (2, 11, 13, 17) also in combination with neuroimaging methods (34, 35), which has resulted in several systematic reviews and meta-analyses (18, 19, 36, 37). No systematic review or meta-analysis to date, however, has exclusively focused on neuroimaging studies in children, adolescents, and young adults at FHR of severe mental disorders. This is surprising, because the inclusion of adult individuals with FHR substantially impacts the interpretation of the results, as these individuals may already have passed the peak onset period and alterations may, thus, rather represent factors of resilience or compensation than vulnerability and risk.
Here, we systematically reviewed the existing literature investigating children, adolescents, and young adults at FHR for SZ (FHR-SZ) or FHR for BD (FHR-BD) with task-related functional magnetic resonance imaging (fMRI). We only considered studies of FHR individuals who had not yet manifested signs of serious mental illness. Half of all lifetime mental disorders start by the mid-teenage years and three quarters by the mid-20s (38). Therefore, we narrowed our search to studies including individuals with a group mean age under or equal to 21 years and did not include studies with participants above the age of 27 years. We aimed to answer the following questions; Firstly, do brain activation patterns associated with task-related activity in children, adolescents, and young adults at FHR-SZ or FHR-BD, differ from the patterns observed in healthy controls (HC)? Secondly, do the two FHR groups show shared or specific differences in brain activation patterns? Lastly, we relate the findings to earlier reports in patients with the manifest disorders, as well as adult FHR populations and clinical high-risk populations. Our approach aims at identifying early neurobiological alterations associated with FHR for severe mental disorders with fMRI, knowledge that may assist the optimization of diagnostic tools and improve the design of early interventions and preventive measures. Neurobiological approaches of child, adolescent and young adult offspring of patients with severe mental illnesses offer the opportunity to assess the early neural imprint of a genetic vulnerability to disease and make way for the study of preclinical features and the interaction between illness-related progressions and normal brain maturation.
We combined the search terms “MRI”, “fMRI”, “children”, “adolescents”, “bipolar disorder”, “schizophrenia”, “risk”, “first-degree relative”, and “genetic predisposition” in PubMed, EMBASE, and PsychINFO followed by a screening of inclusion criteria to identify fMRI studies including children and adolescents at FHR-SZ or FHR-BD (Table 1). The time-period covered in our search included all publications published in this area until September 10th, 2019. The protocol for this systematic review was registered on Prospective Register for Systematic Reviews (PROSPERO; registration ID: CRD42018086995) and is available on their International website (https://www.crd.york.ac.uk/prospero/display_record.php?ID=CRD42018086995). Identification, screening, eligibility, and inclusion procedures followed the Preferred Reporting Items for Systematic Reviews and Meta-analyses (Prisma) 2009 flow diagram (39) (Figure 1). We screened and included articles based on the following inclusion criteria: 1) peer-reviewed articles in English; 2) study population of children, adolescents and/or young adults at FHR (familial high-risk defined as having a first-degree relative diagnosed with SZ or BD) of developing SZ or BD; 3) absence of a diagnosis in the spectrum of psychotic or affective disorders as well as no reported symptoms in these areas; 4) mean age of study population below or equal to 21 years, with no individuals above 27 years of age; 5) direct comparison with a HC group; and 6) employment of task-related fMRI. Screening, eligibility and study selection procedures were completed according to the inclusion criteria by authors AV and LJ, independently. These procedures were completed by following EndNote specific review procedures (40). Any disagreement was followed up by KP. Data extraction was done by LJ under supervision from KL and HS. Summary measures include differences in mean values for behavioral variables as well as peak activation differences between the respective FHR group and HC.
Table 1 Detailed overview of the three database-specific search strings applied in PubMed, EMBASE, and PsychINFO, respectively.
Figure 1 The Preferred Reporting Items for Systematic Reviews and Meta-analyses (Prisma) flow-chart illustrating the systematic review procedure. FHR, familial high-risk; HC, healthy control; MR, magnetic resonance; COS, childhood-onset schizophrenia; DWI, Diffusion-weighted imaging; sMRI, Structural magnetic resonance imaging (MRI); fMRI, functional MRI; BOLD, Blood-oxygen-level-dependent.
A total of 1,137 articles were screened by title and abstract according to the inclusion criteria listed in the Methods section, resulting in exclusion of 562 articles. During the eligibility procedure, we assessed 575 articles by full-text reading of which 556 full-text articles were excluded (Figure 1). As a result of the exclusion process, 19 task-related fMRI articles were included of which five studies included individuals at FHR-SZ, 13 studies included individuals at FHR-BD, and one study pooled FHR-SZ and FHR-BD into one FHR sample (Table 2). All studies employed blood oxygen level–dependent (BOLD) fMRI and identified task-related changes in regional activity at the voxel level by specifying a general linear model (GLM). Three studies performed additional psycho-physiologic interaction (PPI) analysis (41, 42, 49) and a single study applied dynamic causal modeling (53). This review only concerns task-related brain activation as revealed by a GLM based approach. We divided the included articles into four main task domains: 1) Working memory (WM), 2) Cognitive control, 3) Reward processing, and 4) Emotion processing. Note that two articles have been included in two different task domains, i.e., Ladouceur, Diwadkar (55) in WM and emotion processing, and Hart, Bizzell (54) in cognitive control and emotion processing, as the applied tasks contrasts expand both domains. Division of studies into these four main task domains was based on the empirical evidence, when regarding the study specific contrasts applied in the respective GLM designs. We employed this pragmatic and bottom-up approach when reviewing the finally included papers. For details about the study specific contrast of interest see Supplementary Tables S1–S4. Due to the limited total number of articles identified at this point, we included all articles concerning task-related fMRI findings in children, adolescents, and young adults at FHR-SZ or FHR-BD independent of behavioral differences between groups. We have, however, explicitly reported the behavioral differences present in the respective studies.
In addition to the low number of existing articles within this specific field of research, we observed a high heterogeneity in terms of behavioral tasks, analytic methods, and reporting practices within the four main task domains. This heterogeneity precluded the use of quantitative meta-analytic methods, such as activation likelihood estimation (ALE) (60).
Four studies were identified in young individuals at FHR-SZ or FHR-BD within the WM domain. An overview of the brain peak activity differences between individuals at FHR-SZ or FHR-BD relative to HC within the WM domain are shown in Figure 2. Two studies investigated individuals at FHR-SZ. In the first study, the authors found hypo-activation in the left parietal cortex in 19 FHR-SZ offspring (mean age: 14.3) relative to 25 HC (mean age: 14.6) during high versus low WM demand (Table 2 and Figure 2). Furthermore, FHR-SZ relative to HC displayed lower response latencies but did not differ from HC with respect to hit rate and the effect of WM load on performance (41). In a second study, during correct versus incorrect memory performance in a WM task, it was found that 19 FHR-SZ offspring (mean age: 14.3) showed hyper-activation in the right dorsal prefrontal cortex (PFC) and left head of caudate relative to 25 HC (mean age: 14.6) (42) (Table 2 and Figure 2). Of the two studies in FHR-BD, one study reported hypo-activation during high versus low WM load in 10 individuals (mean age: 18.4) in the left cerebellum, bilateral insula, as well as in the right brainstem and right para-hippocampal gyrus/amygdala relative to 10 HC (mean age: 17.1). The same task contrast in this study also elicited hyper-activation in FHR-BD relative to HC in the left frontopolar cortex (43) (Table 2 and Figure 2). Another study also reported hypo-activation during high versus low WM load, though in a different area, namely the left ventro-lateral prefrontal cortex (VLPFC) in 16 FHR-BD offspring (mean age: 14.2) compared with 15 HC (mean age: 13.8) (55). None of the studies in FHR-BD summarized above found behavioral differences relative to HC.
Figure 2 Working memory-related brain activity differences between children and adolescents at familial high-risk (FHR) for schizophrenia (SZ) and healthy control (HC, yellow circles), and for bipolar disorder (BD) and healthy control (green circles). Circles mark the peak coordinate activation difference but do not reflect the extend of activation. The coordinates of peak activation reported in Talairach space were translated to Montreal Neurologic Institute (MNI) coordinates using the MNI<->Talairach with Brodmann Areas (1.09) website (http://sprout022.sprout.yale.edu/mni2tal/mni2tal.html). Using FSLview (version 4.0.1 ©2004-2009 Oxford University), the reported peak coordinates in each article were entered to localize brain area on a standard MNI152 brain. For visualization of areas of peak activation differences between FHR-SZ, FHR-BD, and HC, we created figures using FSLview and Mango (Version: 4.1, Jack L. Lancaster, Ph.D., Michael J. Martinez © 2019 Research Imaging Institute, UTHS CSA). Exact coordinates and details on contrasts and statistics can be found in Supplementary Table 1.
In summary, no behavioral differences were found between FHR-BD and HC, whereas one study in FHR-SZ reported differences between FHR-SZ and HC on response latency with FHR-SZ being faster (41). Brain activity during WM performance differed between both FHR groups and HC groups in brain regions normally associated with WM processing. Although three out of four studies applied a similar high WM load versus low WM load contrast (41, 43, 55), no common altered brain activity patterns between FHR and HC were apparent between these studies. Likewise, no commonalities between FHR-SZ and FHR-BD were seen. However, without taking directionality or magnitude of the observed brain activity differences into account, the three studies, two in FHR-BD individuals and one in FHR-SZ individuals, reported altered frontal activity compared to HC. More specifically, differences were present in the left VLPFC (55), the left frontopolar cortex (43) and the right dorsal PFC (42).
The five included studies of FHR within the cognitive control domain have focused on attention, response inhibition, and cognitive flexibility (Table 2). Differences in peak brain activation in FHR-SZ and FHR-BD relative to HC within the cognitive control domain are depicted in Figure 3. One study pooled FHR-SZ and FHR-BD offspring into one high-genetic-risk group (n = 22, mean age: 14.1). During both high and low attention load versus passive viewing, respectively, the FHR group showed hypo-activation in the dorsal PFC and hyper-activation in the parietal cortex relative to 24 HC (mean age: 15.4). Moreover, the FHR group showed a lack of additional activation during higher attentional demand as opposed to HC (44). A second study investigated selective attention in 21 individuals at FHR-SZ (mean age: 14.4) relative to 21 HC (mean age: 14.1) with FHR-SZ individuals showing lower accuracy on identification of non-targets. In terms of brain activation, FHR-SZ relative to HC displayed hypo-activation during target identification versus task-irrelevant stimuli in the right middle frontal gyrus, left frontal operculum cortex, right supplementary motor area, left insula, right precentral gyrus, right postcentral gyrus, right superior temporal gyrus, right precuneus, and left occipital cortex. Hyper-activation during the same contrast was found in the left inferior frontal cortex, bilateral caudate, left inferior temporal gyrus, and bilateral frontal pole in FHR-SZ relative to HC (54). In a third study, using a similar selective attention task, 29 individuals at FHR-BD (mean age: 14.9) showed larger reaction time variability and displayed hyper-activation in a group-by-reaction time interaction analysis in the left medial frontal gyrus and in the left superior frontal gyrus in comparison with 53 HC (mean age: 18.7) (47). Investigating response inhibition, one study of 13 individuals at FHR-BD (mean age: 13.5) relative to 21 HC (mean age: 13.8), showed hyper-activation during successful motor inhibition (stop incorrect versus stop correct) and unsuccessful inhibition (stop incorrect versus go) in the left and bilateral putamen, respectively (45). Lastly, in a study investigating cognitive flexibility, 13 individuals at FHR-BD (mean age: 13.9) relative to 21 HC (mean age: 13.7) showed hyper-activity in the right inferior parietal gyrus, the right inferior frontal gyrus (IFG) and in the left cerebellum in change versus go trials (i.e., successful cognitive flexibility). Unsuccessful change versus go trials, i.e., unsuccessful cognitive flexibility, evoked hyper-activation in the right caudate and left cerebellum in FHR-BD relative to HC. Successful cognitive flexibility relative to unsuccessful showed hyper-activation in FHR-BD relative to HC in the right IFG (46).
Figure 3 Cognitive control-related brain activity differences between children and adolescents at familial high-risk (FHR) for schizophrenia (SZ) and healthy control (yellow circles), and for bipolar disorder (BD) and healthy control (HC, green circles). Circles mark the peak coordinate activation difference but do not reflect the extend of activation. The coordinates of peak activation reported in Talairach space were translated to Montreal Neurologic Institute (MNI) coordinates using the MNI<->Talairach with Brodmann Areas (1.09) website (http://sprout022.sprout.yale.edu/mni2tal/mni2tal.html). Using FSLview (version 4.0.1 ©2004-2009 Oxford University), the reported peak coordinates in each article were entered to localize brain area on a standard MNI152 brain. For visualization of areas of peak activation differences between FHR-SZ, FHR-BD, and HC, we created figures using FSLview and Mango (Version: 4.1, Jack L. Lancaster, Ph.D., Michael J. Martinez © 2019 Research Imaging Institute, UTHS CSA). Exact coordinates and details on contrasts and statistics can be found in Supplementary Table 2.
In summary, two studies found behavioral differences on selective attention, one between FHR-SZ and HC (54), the other between FHR-BD and HC (47). In both studies, the FHR group performed worse than HC. Interestingly, all studies on FHR-BD groups found hyper-activity relative to HC, independent of paradigm design and task contrast (44–47) (Figure 3). Finally, when comparing young individuals at FHR-SZ or FHR-BD with HC during diverse cognitive control tasks, observed differences in brain activity generally involved areas within frontal, temporal, parietal, midbrain areas, as well as cerebellar sub-areas. Of note, only one study with FHR-SZ individuals contributed to these finding (54), while in another study FHR-SZ individuals were pooled with FHR-BD individuals into one high-risk group (44).
Three studies investigated reward processing in children, adolescents, and young adults at FHR-BD and HC (Table 2). Independent of winning or losing versus control trials, 29 FHR-BD offspring (mean age: 13.8) showed hyper-activation in the right frontal pole relative to 23 HC (mean age: 13.7) (48) (Figure 4). In another study, hyper-activation was reported in the right posterior insula in 25 FHR-BD offspring (mean age: 14.2) relative to 21 HC (mean age: 14.0) when contrasting the winning versus the control condition (50) (Figure 4). On the other hand, anticipation of loss versus non-loss resulted in hypo-activation in 20 FHR-BD offspring (mean age: 12.7) in the right pregenual cingulate cortex relative to 25 HC (mean age: 11.8). Furthermore, FHR-BD offspring also showed hyper-activation during reward feedback versus non-reward feedback in the left lateral orbitofrontal cortex relative to HC (49) (Figure 4).
Figure 4 Reward-related brain activity differences between children and adolescents at familial high-risk (FHR) for schizophrenia (SZ) and healthy control (yellow circles), and for bipolar disorder (BD) and healthy control (HC, green circles). Circles mark the peak coordinate activation difference but do not reflect the extend of activation. The coordinates of peak activation reported in Talairach space were translated to Montreal Neurologic Institute (MNI) coordinates using the MNI<->Talairach with Brodmann Areas (1.09) website (http://sprout022.sprout.yale.edu/mni2tal/mni2tal.html). Using FSLview (version 4.0.1 ©2004-2009 Oxford University), the reported peak coordinates in each article were entered to localize brain area on a standard MNI152 brain. For visualization of areas of peak activation differences between FHR-SZ and HC, and between FHR-BD and HC, we created figures using FSLview and Mango (Version: 4.1, Jack L. Lancaster, Ph.D., Michael J. Martinez © 2019 Research Imaging Institute, UTHS CSA). Exact coordinates and details on contrasts and statistics can be found in Supplementary Table 3.
In summary, differences in brain activity during reward processing in young individuals at FHR-BD relative to HC were mainly found in frontal cortical areas (Figure 4). Depending on the contrast of interest, i.e., anticipation or feedback, and winning or losing trials, these activation differences consisted of both hypo- and hyper-activity relative to HC. Although two studies applied similar number guessing reward tasks in FHR-BD [i.e., Manelis, Ladouceur (48) and Soehner, Bertocci (50)], activity differences were present in distinct areas and of opposite direction leaving little overlap in findings. Of note, we did not identify any studies investigating reward processing in young individuals at FHR-SZ.
We identified nine studies in young individuals at FHR-SZ or FHR-BD, dealing with brain responses related to the processing of faces showing emotional expressions or pictures with affective valence. This makes the emotion processing domain the most investigated domain in the present reviewed literature (Table 2). An overview of the emotion-related peak brain activity differences between young individuals at FHR-SZ and HC, and young individuals at FHR-BD and HC are depicted in Figure 5. None of the included studies reported behavioral differences between any FHR group and HC. One study did not find activation-related differences between 19 FHR-SZ offspring (mean age: 14.3) compared with 24 HC (mean age: 14.6) when viewing faces with emotional expressions versus distorted images (53). On the contrary, other studies employing similar emotion processing paradigms found that young FHR-BD individuals relative to HC show amygdala hyper-activation during viewing of emotional faces versus shapes (56), when viewing faces and rating them as fearful versus passive viewing (57), as well as during successful versus unsuccessful encoding of emotional faces (58). Further, distraction versus no distraction by happy faces during WM performance elicited hyper-activation in the right VLPFC in 16 FHR-BD (mean age: 14.2) relative to 15 HC (mean age: 13.8) (55). Moreover, 15 FHR-BD (mean age: 14.2) relative to 29 HC (mean age: 14.9) displayed hypo-activation in bilateral amygdala and in the left IFG with increasing anger intensity in face expressions, as well as in the left IFG during increasing happiness intensity in face expressions (51). In line with this, 19 FHR-SZ offspring (mean age: 14.3) relative to 25 HC (mean age: 14.9) showed hypo-activation in the left amygdala (centro-medial nuclei) in response to happy face expressions (52). Another study, reported hypo-activation in the left anterior cingulate cortex (ACC) and the left precuneus but hyper-activation in the central opercular cortex in 21 FHR-SZ (mean age: 14.4) relative to 21 HC (mean age: 14.1) in response to unpleasant images (53). Finally, 32 FHR-BD offspring (mean age: 15.3) relative to 32 HC (mean age: 14.6) showed hyper-activation in the left IFG during the viewing of unpleasant and pleasant images versus neutral images (59).
Figure 5 Emotion-related brain activity differences between children and adolescents at familial high-risk (FHR) for schizophrenia (SZ) and healthy control (yellow circles), and between bipolar disorder (BD) and healthy control (HC, green circles). Circles mark the peak coordinate activation difference but do not reflect the extend of activation. The coordinates of peak activation reported in Talairach space were translated to Montreal Neurologic Institute (MNI) coordinates using the MNI<->Talairach with Brodmann Areas (1.09) website (http://sprout022.sprout.yale.edu/mni2tal/mni2tal.html). Using FSLview (version 4.0.1 ©2004-2009 Oxford University), the reported peak coordinates in each article were entered to localize brain area on a standard MNI152 brain. For visualization of areas of peak activation differences between FHR-SZ, FHR-BD, and HC, we created figures using FSLview and Mango (Version: 4.1, Jack L. Lancaster, Ph.D., Michael J. Martinez © 2019 Research Imaging Institute, UTHS CSA). Exact coordinates and details on contrasts and statistics can be found in Supplementary Table 4.
In summary, young individuals at FHR-BD and FHR-SZ did not show behavioral differences compared with HC in the studies investigating emotional processing included in the present review. Across emotion processing paradigms, four studies reported altered activation (i.e., hypo- or hyper-activation) of amygdala in FHR-BD individuals compared with HC (51, 56–58), although each study applied individual task contrasts. FHR-BD individuals also showed altered activation in inferior/middle FG across two different studies and task contrasts (51, 59). Findings in FHR-SZ were less clear; one study showed amygdala hyper-activation (52), while another study showed ACC hypo-activation and central opercular cortex hyper-activation (54), which may relate to differences in task setup as well as applied analytical methods.
We have reviewed the existing literature to clarify whether children, adolescents, and young adults at familial high risk for either schizophrenia (FHR-SZ) or bipolar disorder (FHR-BD) show altered brain activation during task performance when compared to HC, and to determine shared or distinct patterns of brain activation in FHR-SZ versus FHR-BD. In this review, we have focused on task-based functional brain alterations with a specific emphasis on the susceptibility to SZ and BD, given the significant heritability for SZ or BD. The genetic variations that have shown to confer increased life-time risk to develop SZ or BD show a large overlap (7–10). This explains why a child’s vulnerability is not exclusively associated with a high-risk to develop the same illnesses as the parent, but vulnerability applies to a broader range of psychiatric and neurodevelopmental disorders (2, 3).
The main results of this review revealed that young individuals at FHR-SZ or FHR-BD did not show behavioral impairments in the experimental setting of a neuroimaging environment. Normal task performance in fMRI studies contrasts with several neuropsychological studies that assessed the behavioral performance only (11–17). Only two fMRI studies reported performance differences in FHR-SZ in a WM-related task and in an attention-related task, respectively, and only one study found impairments of performance in FHR-BD in an attention-related task. Across all task domains, FHR-SZ and FHR-BD showed altered brain activation when compared to HC. In the absence or the presence of a minimal overlap in altered brain activation between FHR-SZ and FHR-BD, the existing fMRI data suggest that FHR-SZ and FHR-BD are associated with distinct patterns of aberrant brain activation. This evidence, however, is of circumstantial character as most studies did not directly contrast brain activation patterns between the two groups.
Within the WM domain, young individuals with FHR-SZ or FHR-BD show altered brain activation patterns in frontal cortex, including the left VLPFC and left frontopolar regions compared to HC. These findings are in line with the existing neuroimaging literature in adult individuals at FHR-SZ (61), as well as in individuals at clinical high-risk (e.g., first-episode patients) of SZ (62) and confirmed SZ and BD patients (63). WM encompasses the ability to maintain information in an easily accessible state over short periods of time to enable goal-directed behavior (64). Lesion studies, transcranial magnetic stimulation (TMS) studies, and neuroimaging studies in humans, as well as single-cell recordings in monkeys have pinpointed the DLPFC, areas in the parietal cortex (superior, ventral and inferior), the cerebellum, striatum, and the medial temporal lobe (MTL) as being the significant brain regions involved in WM processes (65). Impairment of WM is a well-known feature in individuals with SZ spectrum and has been shown to be associated with PFC and fronto-striatal dysfunction in adult individuals with SZ and their unaffected adult first-degree relatives (66). WM deficits in patients with BD are one of the most frequently observed cognitive impairments and have been linked to brain dysfunction (i.e., hyper-activation) in frontal areas (67). Similarly, WM impairments have been shown in healthy adult first-degree relatives of BD patients (15). We did not find evidence of an impaired WM performance in young individuals at FHR-SZ or FHR-BD, and the alterations in WM-related brain activation in frontal regions resembled the pattern present in adult FHR individuals (18, 36). Taken together, these findings may indicate a compensatory mechanism or reflect neural inefficiency.
In the cognitive control domain, FHR individuals showed widespread altered brain activation in areas supporting cognitive control compared to HC. However, this finding was almost exclusively obtained in FHR-BD, and the number of studies and participants producing these widespread differences in activation patterns was low. Of note, cognitive control unifies several top-down cognitive processes supporting attention, problem-solving abilities and making appropriate decisions (64). Due to the small number of studies, however, we pooled all studies related to cognitive control, although some studies were more focused on attentional demands while others tapped into inhibitory abilities. Therefore, it is not surprising that there was little to no overlap between reported results in terms of brain areas that showed peak activation differences. Decreased attention span and poor inhibitory control are well-characterized deficits associated with SZ (68), which has been linked to abnormal brain activation in the DLPFC, ACC, thalamus, and in inferior/posterior parietal areas (69). These brain areas are known to support cognitive control task performance in HC (70). Further, a study on adult FHR-SZ (mean age >21) also found abnormalities in prefrontal activation during cognitive control tasks (22). One study included in the present review investigated young individuals at FHR-SZ, showing that altered brain activation in frontal, medial and parietal brain regions associated to cognitive control performance is already expressed in young individuals at FHR-SZ (54). Similarly, symptomatic individuals with BD show impaired cognitive control, such as sustained attention and inhibitory control, which persists in remission (15, 71). During cognitive control-related task performance, altered brain activation in IFG and limbic areas have been observed in individuals with confirmed BD as well as in adult individuals at FHR-BD (mean age >21) (18, 72). In summary, the abnormal fMRI activation patterns in the cognitive domain in young individuals with FHR match the findings reported in studies of individuals with confirmed SZ or BD as well as adults at FHR-BD and adults at FHR-SZ.
Studies in the reward processing domain only investigated young individuals at FHR-BD and showed differences in brain activity in frontal and medial areas during reward processing relative to HC. Depending on the investigated contrast of interest in the different studies reflecting brain responses to, e.g., anticipation, feedback, winning or losing, these activation differences in individuals at FHR-BD consisted of hypo- and hyper-activation relative to HC. Reward is a central component for facilitating motivation-based learning and the learning of appropriate responses to stimuli, as well as the formation of habits (73). The foundation of the reward system consist of circuits connecting specific frontal- and basal ganglia regions, including the ACC, the orbitofrontal cortex, the ventral striatum, the ventral pallidum, and the midbrain regions (74, 75). Manic episodes, a core symptom in BD, have been associated with impulsive decision making and risk taking that may arise from hyper-sensitivity to reward or general reward dysregulation (76). In the absence of reward-related behavioral impairments in young individuals at FHR-BD, the altered activation patterns of reward-related brain regions observed in the present review may indicate hyper-sensitivity or dysregulation in response to reward-related cues or compensatory mechanisms. We did not identify any studies investigating reward-related brain activity in young individuals at FHR-SZ compared with HC although negative and positive symptoms in SZ may relate to dysfunction of the reward system in the brain (77).
Emotion processing was the most investigated cognitive domain in children, adolescents, and young adults at FHR-BD. Altered amygdala function in individuals at FHR-BD was reported across different emotion processing studies (51, 56–58). Also, IFG/middle FG showed altered activation in FHR-BD compared with HC across two different studies and task contrasts (51, 59). These alterations are consistent with literature investigating BD patient populations, underlining these deficiencies as possible endophenotypic markers of the disorder (78). The two studies focusing on FHR-SZ yielded less consistent results, showing hypo-activation in amygdala in response to positive faces (52), and in ACC in response to aversive images (54). Viewing of aversive images also elicited hyper-activation in the central opercular cortex in FHR-SZ compared to HC (54). The neurobiological alterations in FHR-SZ youth compared to HC is adding to a growing body of evidence for endophenotypic traits of SZ which is also present in individuals at ultra-high risk (UHR) for psychosis, showing hyper-activation of frontal areas compared to controls when viewing negative pictures (79). The ability to regulate and process emotional responses depending on affective and social cues enables appropriate adaptiveness in various events throughout the lifespan (80). Subcortical structures, such as the amygdala and other limbic areas, play a key role in the processing of emotions. Emotion dysregulation is a core feature of almost every severe mental disorder, causing maladaptive decision making and social interactions (81). Patients with SZ and BD show an affective bias toward erroneous interpretation of emotional stimuli and general emotion dysregulation (82, 83). Individuals with SZ are impaired when making affective judgment and regulation, which may lead to misinterpretation of social cues and poor social skills (84). Individuals with BD show deficits in emotional processing even during euthymic periods (85). Of note, individual studies included in the emotion processing domain in the present review spanned several behavioral paradigm designs, but individual analytical approaches incorporated an emotion processing contrast (see Supplementary Table S4). Despite heterogenous task setups, reported results were fairly converging on differences between FHR-BD and HC on amygdala and IFG acivation.
A between-group comparison, including confirmed SZ or BD, FHR, and HC groups, may expand the current knowledge in three directions. First of all, brain-based measures found in FHR individuals and in individuals with confirmed SZ or BD, but not in HC, may reflect neurobiological markers of risk for severe mental illness, and thus may be classified as potential risk endophenotypes (86). Second, the comparison may also identify potential biomarkers for resilience to severe mental illness (87). This may be the case for brain regions where FHR individuals showed regional increases in brain activity, relative to confirmed SZ, BD, and HC, and where task-related brain activity scales positively with task performance. Finally, regions where individuals with confirmed SZ or BD showed dysfunction relative to FHR and HC may reflect potential illness-related adaptations. In these areas, regional brain activity should not reflect high quality of performance, but may rather scale with measures of task impairment. These neurobiological illness-related adaptations may be heavily influenced by important factors, such as duration of illness, medication, illness onset time, etc. As earlier stated, attenuated symptoms in psychosis may precede the manifest disorder.
An alternative way to investigate this hypothesis is by examining whether behavioral and neurobiological impairments are already present in individuals with an At-Risk-Mental-State (ARMS), also known as individuals at UHR for psychosis. This UHR category was introduced with the goal of developing preventive strategies of psychotic disorders and requires individuals to present with either (a) positive symptoms that are typical of psychotic disorders but of subthreshold severity or duration or (b) genetic high risk accompanied by functional decline (88). Individuals at UHR have an increased risk for developing psychosis with transition rates of 29% after 2 years (89, 90). As all UHR criteria rely on help-seeking individuals, the prevalence of the UHR state in the general population is not known to date. Widespread mild cognitive deficits are present in UHR individuals, falling at a level in between that of healthy individuals and those with confirmed SZ, and the magnitude of deficits is comparable with those at FHR (91). Further, abnormalities in brain activity and/or functional connectivity during a variety of cognitive tasks, including verbal memory and WM, verbal fluency, social cognition, as well as in the context of functions directly associated to the emergence of psychotic symptoms has also been shown [for a review, see Andreou and Borgwardt (92)]. Of note and as was the results of the present review, neuroimaging abnormalities in UHR is observed even in the absence of differences in behavioral performance (92), which may point to a compensatory mechanisms for the brain circuits to uphold sufficient behavior.
Behavioral differences between FHR individuals and HC were limited to the domains of WM and cognitive control. FHR-SZ showed lower response latency during an n-back task (41), and poorer performance in detecting non-targets compared to HC during an emotional odd ball task (54). Further, individuals with FHR-BD compared with HC displayed higher reaction time variability in a global attention task (47). The lack of behavioral differences in most studies summarized in the present review may be due to the chosen behavioral variables (e.g., accuracy and reaction time) that may lack sufficient sensitivity. Previous discussions have focused on the limitations of measuring high-level cognitive processes by simply inferring on accuracy and reaction time variables, and argue that some aspects of cognitive processes may be detectable with fMRI but not with these crude behavioral variables (93). Recent computational efforts and behavioral modeling approaches such as Bayesian modeling could help disentangle, explain, and even predict the different contributions from various behavioral sub-parameters within behavioral domains and task paradigms, which may then show a behavioral separation between children, adolescents, and young adults at FHR-SZ or FHR-BD, and HC. An important factor to consider may also be the effect of specific paradigm designs to fit them into an fMRI setting. During the recording of fMRI, a collection of images covering the whole brain is generally acquired every 1–3 s, and hundreds of brain volumes are gathered during completion of an entire fMRI scan, lasting around 2–15 min (94). In this setting, the time constraints for the cognitive tasks need adjustment to this temporality. The limited temporal resolution inherent in fMRI acquisition thus narrows the complexity and speed with which the cognitive paradigm may be carried out and is different from the cognitive paradigms completed outside a neuroimaging environment.
Sample sizes in the included studies were in general small to moderate of size with the smallest being 10 and the largest being 56. The mean sample size of all included studies was 26 for HC and 20 for FHR. Large effect sizes (Cohen’s d > 0.8) have been found in individuals with manifest BD when tested on different cognitive domains, but in first-degree relatives effect sizes are generally small to medium (Cohen’s d < 0.5) yet still significantly different from HC (15). In addition, individuals with SZ perform on average about 0.92 standard deviations worse than controls across many cognitive tasks, whereas the average effect size in healthy relatives on the same metric compared with appropriate control groups is approximately 0.35 (19). Hence, patient studies generally require smaller sample sizes, whereas studies of relatives require larger sample sizes to observe statistically significant group differences. Therefore, valuable evidence may have been missed due to insufficient statistical power inherent in small sample sizes. Also, when investigating a heterogeneous group of individuals (i.e., age span, sex, FHR disorder, comorbidities, etc.) small sample sizes could further obscure significant findings. In addition to small sample sizes in included studies, overlapping of study populations may present a potential confounding factor. Several studies may have originated from the same overall study and/or shared recruitment details and methods, and may thus not stem from independent populations, however, we did not investigate this further.
To fully establish a link between task-related activity and risk or resilience factors of psychiatric disorders, longitudinal fMRI data needs to be acquired. Indeed, longitudinal studies following symptom-free at risk or vulnerable individuals through maturation and possible development of severe mental illnesses would allow for identification of relevant pre-clinical and clinical markers of vulnerability, disease onset and/or resilience. The cross-sectional design of the studies included in the present review is thus a major limitation. While cross-sectional studies allow mapping of neurobiological differences between young individuals at FHR and typically developing controls, observed group differences may reflect vulnerability, brain alterations leading to disorder, or compensational mechanisms as well as variability in developmental stage. As an example of variation, grey matter volume in the frontal cortex peaks between age 7 to 11, but total cortical volume can vary up to 50% between typically developing individuals which enhances the relevance of inter-individual variability (95). Likewise, the subcortical structures undergo developmental changes in volume and shape in a non-linear fashion (96). General for the studies included here is that they do not report other environmental factors than socio-economic status and parental educational level. Since FHR studies cannot disentangle the effects of shared genes from shared environmental influences, designing studies with focus on, e.g., epigenetic analysis as well as home-, school-, and work- environment, and adverse lifetime events might contribute to a better understanding of contributing factors in the complex development of both SZ and BD.
In line with the longitudinal perspective mentioned above, the age of the included participants, as well as the age-range in the groups are of importance when evaluating vulnerability factors. Investigating young individuals at FHR for severe mental illnesses is of importance given the brain’s susceptibility to maladaptive changes within this period of the lifespan (33). The studies reviewed herein included individuals within an age range of at least seven years [age span 8–15 (49)] and at maximum 17 years [age span 8–25 (47)] This is a significant age span when considering neurodevelopment, since both grey and white matter undergoes substantial maturation throughout childhood and adolescence, with different structures reaching adult maturity level at different time points in different individuals (97). Including individuals within a broad age span during development may therefore include unwanted variability, which subsequently may impede clear conclusions. Future studies should thus strive to establish longitudinal cohorts with a limited age span.
Inclusion of FHR individuals with comorbid diagnoses, such as ADHD, anxiety disorder, phobia, major depressive disorder, etc. (see Table 2 for details) varied considerably across the different included studies and consequently some of the investigated individuals at FHR were on medication. Specifically, 13 of the included studies in the present review investigated FHR individuals with existing diagnoses (41, 42, 47, 48, 50, 54, 56, 59). Of importance, the reported diagnoses could be associated with behavioral and neurological changes not related directly and exclusively to the FHR status, but to the specific diagnoses or medications. For example, it has been proposed that whole-brain immature functional connections may underlie ADHD (98) and medication-naïve children with ADHD display reduced error-signaling within cingulo-opercular regions (99). However, it is also important to consider that individuals at FHR-SZ with ADHD might constitute a subgroup with enhanced risk for psychosis compared to FHR-SZ without diagnoses (100). Hence, these participants constitute an important group when studying underlying neural vulnerability factors for psychosis. Most of the studies that included FHR individuals with existing diagnoses performed exploratory or post hoc analyses in which participants with existing diagnoses/non-medication naïve were excluded. The post hoc analyses ruled out effects of diagnoses on the main results (41, 42, 47, 48, 50, 51, 54–58). Three studies did not perform such post hoc/exploratory analyses (52, 53, 59) and the effect of existing diagnoses on these particular results is therefore speculative.
Here, we specifically focused on reviewing studies including young individuals with a mean age ranging from 12.7 to 18.4 years of age (11.8 to 18.7 years of age for controls). Even though this is an advantage, given that this age is before the usual onset of SZ or BD, it narrowed the number of included articles substantially. Lastly, the decision to include only publications in English further reduced the number of included articles in this systematic review.
Mapping functional brain alterations in offspring of parents with confirmed SZ or BD may provide important insights into the underlying neurobiological processes that convey vulnerability to these disorders. Given the heterogeneity of the measures, methods and findings, supplementary fMRI studies are needed. These studies should preferentially aim at a longitudinal design and include large groups of individuals with a narrow age range to facilitate the interpretation of altered activity patterns during a specific cognitive task. Our literature search yielded no study that directly compared task-related brain activation between young individuals at FHR-SZ and young individuals at FHR-BD and HC. Given the empirical evidence by Lichtenstein et al. (7) and Schulze et al. (8) mentioned in the introduction section of the present review, the comparison between studies investigating FHR-SZ and studies investigating FHR-BD in larger groups and with more homogeneous methods will hopefully allow for a dissociation between the early stages of pathogenesis of these severe mental illnesses in the future. Ultimately, the understanding of the neurobiological mechanisms will guide and optimize future treatment and prevention practices toward higher precision.
The raw data supporting the conclusions of this article will be made available by the authors, without undue reservation, to any qualified researcher.
LJ, AV, BB, HS, and KP contributed to conception and design of the review. LJ and AV organized the database. LJ wrote the first draft of the manuscript. KL and KP wrote sections of the manuscript. All authors contributed to the article and approved the submitted version.
HS has received honoraria as speaker from Sanofi Genzyme, Denmark and Novartis, Denmark, as consultant from Sanofi Genzyme, Denmark and as senior editor (NeuroImage) and editor-in-chief (NeuroImage Clinical) from Elsevier Publishers, Amsterdam, The Netherlands. HS has also received royalties as book editor from Springer Publishers, Stuttgart, Germany and Gyldendahl Publishers, Copenhagen, Denmark.
The remaining authors declare that the research was conducted in the absence of any commercial or financial relationships that could be construed as a potential conflict of interest.
The authors would like to acknowledge the help provided by information-specialists at The Royal Danish Library, Copenhagen in designing the final search strings.
LJ is supported by a grant given to KP by the Independent Research Fund, Denmark (Grant Nr. DFF – 6110-00139) as well as a grant given to the Danish high risk and resilience study – VIA11 by The Innovation Fund, Denmark (Grant Nr. 6152-00002B).
AV and KL are funded by individual stipends from the Mental health services in the Capital Region of Denmark.
HS holds a 5-year professorship in precision medicine at the Faculty of Health and Medical Sciences, University of Copenhagen which is sponsored by the Lundbeck Foundation (Grant Nr. R186-2015-2138).
The Supplementary Material for this article can be found online at: https://www.frontiersin.org/articles/10.3389/fpsyt.2020.00632/full#supplementary-material
1. Martin AR, Daly MJ, Robinson EB, Hyman SE, Neale BM. Predicting Polygenic Risk of Psychiatric Disorders. Biological psychiatry. (2019) 86:97–109.
2. Rasic D, Hajek T, Alda M, Uher R. Risk of mental illness in offspring of parents with schizophrenia, bipolar disorder, and major depressive disorder: a meta-analysis of family high-risk studies. Schizophr Bull (2014) 40(1):28–38. doi: 10.1093/schbul/sbt114
3. Thorup AAE, Laursen TM, Munk-Olsen T, Ranning A, Mortensen PB, Plessen KJ, et al. Incidence of child and adolescent mental disorders in children aged 0-17 with familial high risk for severe mental illness - A Danish register study. Schizophr Res (2018) 197:298–304. doi: 10.1016/j.schres.2017.11.009
4. Howes OD, Murray RM. Schizophrenia: an integrated sociodevelopmental-cognitive model. Lancet (2014) 383(9929):1677–87. doi: 10.1016/S0140-6736(13)62036-X
5. Murray RM, Lewis SW. Is schizophrenia a neurodevelopmental disorder? Br Med J (Clin Res Ed) (1987) 295(6600):681–2. doi: 10.1136/bmj.295.6600.681
6. Sanches M, Keshavan MS, Brambilla P, Soares JC. Neurodevelopmental basis of bipolar disorder: A critical appraisal. Prog Neuropsychopharmacol Biol Psychiatry (2008) 32(7):1617–27. doi: 10.1016/j.pnpbp.2008.04.017
7. Lichtenstein P, Yip BH, Bjork C, Pawitan Y, Cannon TD, Sullivan PF, et al. Common genetic determinants of schizophrenia and bipolar disorder in Swedish families: a population-based study. Lancet (2009) 373(9659):234–9. doi: 10.1016/S0140-6736(09)60072-6
8. Schulze TG, Akula N, Breuer R, Steele J, Nalls MA, Singleton AB, et al. Molecular genetic overlap in bipolar disorder, schizophrenia, and major depressive disorder. World J Biol Psychiatry (2014) 15(3):200–8. doi: 10.3109/15622975.2012.662282
9. Cross-Disorder Group of the Psychiatric Genomics Consortium. Identification of risk loci with shared effects on five major psychiatric disorders: a genome-wide analysis. Lancet (2013) 381(9875):1371–9. doi: 10.1016/S0140-6736(12)62129-1
10. Cross-Disorder Group of the Psychiatric Genomics Consortium. Genetic relationship between five psychiatric disorders estimated from genome-wide SNPs. Nat Genet (2013) 45:984. doi: 10.1038/ng.2711
11. Keshavan M, Kulkarni S, Bhojraj T, Francis A, Diwadkar V, Montrose D, et al. Premorbid cognitive deficits in young relatives of schizophrenia patients. Front Hum Neurosci (2010) 3:62. doi: 10.3389/neuro.09.062.2009
12. Hemager N, Vangkilde S, Thorup A, Christiani C, Ellersgaard D, Spang KS, et al. Visual attention in 7-year-old children at familial high risk of schizophrenia or bipolar disorder: The Danish high risk and resilience study VIA 7. J Affect Disord (2019) 258:56–65. doi: 10.1016/j.jad.2019.07.079
13. Snitz BE, MacDonald AW III, Carter CS. Cognitive Deficits in Unaffected First-Degree Relatives of Schizophrenia Patients: A Meta-analytic Review of Putative Endophenotypes. Schizophr Bull (2005) 32(1):179–94. doi: 10.1093/schbul/sbi048
14. Agnew-Blais J, Seidman L. Neurocognition in youth and young adults under age 30 at familial risk for schizophrenia: A quantitative and qualitative review. Cogn Neuropsychiatry (2013) 18(1/2):44–82. doi: 10.1080/13546805.2012.676309
15. Arts B, Jabben N, Krabbendam L, van Os J. Meta-analyses of cognitive functioning in euthymic bipolar patients and their first-degree relatives. psychol Med (2008) 38(6):771–85. doi: 10.1017/S0033291707001675
16. Burton BK, Vangkilde S, Petersen A, Skovgaard LT, Jepsen JR, Hemager N, et al. Sustained Attention and Interference Control Among 7-Year-Old Children With a Familial High Risk of Schizophrenia or Bipolar Disorder-A Nationwide Observational Cohort Study. Biol Psychiatry Cogn Neurosci Neuroimaging (2018) 3(8):704–12. doi: 10.1016/j.bpsc.2018.04.012
17. Burton BK, Hjorthøj C, Jepsen JR, Thorup A, Nordentoft M, Plessen KJ. Research Review: Do motor deficits during development represent an endophenotype for schizophrenia? A meta-analysis. J Child Psychol Psychiatry (2016) 57(4):446–56. doi: 10.1111/jcpp.12479
18. Piguet C, Fodoulian L, Aubry JM, Vuilleumier P, Houenou J. Bipolar disorder: Functional neuroimaging markers in relatives. Neurosci Biobehav Rev (2015) 57:284–96. doi: 10.1016/j.neubiorev.2015.08.015
19. MacDonald A, Thermenos HW, Barch DM, Seidman LJ. Imaging genetic liability to schizophrenia: systematic review of FMRI studies of patients’ nonpsychotic relatives. Schizophr Bull (2009) 35(6):1142–62. doi: 10.1093/schbul/sbn053
20. Lee MS, Anumagalla P, Talluri P, Pavuluri MN. Meta-analyses of developing brain function in high-risk and emerged bipolar disorder. Front Psychiatry (2014) 5:141. doi: 10.3389/fpsyt.2014.00141
21. Fusar-Poli P, Howes O, Bechdolf A, Borgwardt S. Mapping vulnerability to bipolar disorder: a systematic review and meta-analysis of neuroimaging studies. J Psychiatry Neurosci Jpn (2012) 37(3):170–84. doi: 10.1503/jpn.110061
22. Goghari VM. Executive functioning-related brain abnormalities associated with the genetic liability for schizophrenia: an activation likelihood estimation meta-analysis. Psychol Med (2011) 41(6):1239–52. doi: 10.1017/S0033291710001972
23. Shaw P, Gogtay N, Rapoport J. Childhood psychiatric disorders as anomalies in neurodevelopmental trajectories. Hum Brain Mapp (2010) 31(6):917–25. doi: 10.1002/hbm.21028
24. Gogtay N, Hua X, Stidd R, Boyle CP, Lee S, Weisinger B, et al. Delayed white matter growth trajectory in young nonpsychotic siblings of patients with childhood-onset schizophrenia. Arch Gen Psychiatry (2012) 69(9):875–84. doi: 10.1001/archgenpsychiatry.2011.2084
25. Gogtay N, Sporn A, Clasen LS, Greenstein D, Giedd JN, Lenane M, et al. Structural brain MRI abnormalities in healthy siblings of patients with childhood-onset schizophrenia. Am J Psychiatry (2003) 160(3):569–71. doi: 10.1176/appi.ajp.160.3.569
26. Sugranyes G, de la Serna E, Borras R, Sanchez-Gistau V, Pariente JC, Romero S, et al. Clinical, cognitive, and neuroimaging evidence of a neurodevelopmental continuum in offspring of probands with schizophrenia and bipolar disorder. Schizophr Bull (2017) 43(6):1208–19. doi: 10.1016/S0924-977X(17)31623-1
27. Dennis EL, Thompson PM. Typical and atypical brain development: a review of neuroimaging studies. Dialogues Clin Neurosci (2013) 15(3):359–84.
28. Ostby Y, Tamnes CK, Fjell AM, Westlye LT, Due-Tonnessen P, Walhovd KB. Heterogeneity in subcortical brain development: A structural magnetic resonance imaging study of brain maturation from 8 to 30 years. J Neurosci Off J Soc Neurosci (2009) 29(38):11772–82. doi: 10.1523/JNEUROSCI.1242-09.2009
29. Raznahan A, Shaw P, Lalonde F, Stockman M, Wallace GL, Greenstein D, et al. How does your cortex grow? J Neurosci Off J Soc Neurosci (2011) 31(19):7174–7. doi: 10.1523/JNEUROSCI.0054-11.2011
30. Tamnes CK, Herting MM, Goddings AL, Meuwese R, Blakemore SJ, Dahl RE, et al. Development of the Cerebral Cortex across Adolescence: A Multisample Study of Inter-Related Longitudinal Changes in Cortical Volume, Surface Area, and Thickness. J Neurosci Off J Soc Neurosci (2017) 37(12):3402–12. doi: 10.1523/JNEUROSCI.3302-16.2017
31. Casey BJ, Jones RM, Somerville LH. Braking and Accelerating of the Adolescent Brain. J Res Adolesc (2011) 21(1):21–33. doi: 10.1111/j.1532-7795.2010.00712.x
32. Casey BJ, Heller AS, Gee DG, Cohen AO. Development of the emotional brain. Neurosci Lett (2019) 693:29–34. doi: 10.1016/j.neulet.2017.11.055
33. Paus T, Keshavan M, Giedd JN. Why do many psychiatric disorders emerge during adolescence? Nat Rev Neurosci (2008) 9(12):947–57. doi: 10.1038/nrn2513
34. Karlsgodt KH, Glahn DC, van Erp TGM, Therman S, Huttunen M, Manninen M, et al. The relationship between performance and fMRI signal during working memory in patients with schizophrenia, unaffected co-twins, and control subjects. Schizophr Res (2007) 89(1):191–7. doi: 10.1016/j.schres.2006.08.016
35. Sambataro F, Mattay VS, Thurin K, Safrin M, Rasetti R, Blasi G, et al. Altered cerebral response during cognitive control: a potential indicator of genetic liability for schizophrenia. Neuropsychopharmacol Off Publ Am Coll Neuropsychopharmacol (2013) 38(5):846–53. doi: 10.1038/npp.2012.250
36. Thermenos HW, Keshavan MS, Juelich RJ, Molokotos E, Whitfield-Gabrieli S, Brent BK, et al. A review of neuroimaging studies of young relatives of individuals with schizophrenia: a developmental perspective from schizotaxia to schizophrenia. Am J Med Genet Part B Neuropsychiatr Genet Off Publ Int Soc Psychiatr Genet (2013) 162B(7):604–35. doi: 10.1002/ajmg.b.32170
37. Cooper D, Barker V, Radua J, Fusar-Poli P, Lawrie SM. Multimodal voxel-based meta-analysis of structural and functional magnetic resonance imaging studies in those at elevated genetic risk of developing schizophrenia. Psychiatry Res: Neuroimaging (2014) 221(1):69–77. doi: 10.1016/j.pscychresns.2013.07.008
38. Kessler CR, Amminger PG, Aguilar-Gaxiola BS, Alonso BJ, Lee BS, Üstün BT. Age of onset of mental disorders: a review of recent literature. Curr Opin Psychiatry (2007) 20(4):359–64. doi: 10.1097/YCO.0b013e32816ebc8c
39. Moher D, Liberati A, Tetzlaff J, Altman DG, Group P. Preferred reporting items for systematic reviews and meta-analyses: the PRISMA statement. PloS Med (2009) 6(7):e1000097. doi: 10.1371/journal.pmed.1000097
40. Bramer WM, Milic J, Mast F. Reviewing retrieved references for inclusion in systematic reviews using EndNote. J Med Libr Assoc (2017) 105(1):84–7. doi: 10.5195/JMLA.2017.111
41. Bakshi N, Pruitt P, Radwan J, Keshavan MS, Rajan U, Zajac-Benitez C, et al. Inefficiently increased anterior cingulate modulation of cortical systems during working memory in young offspring of schizophrenia patients. J Psychiatr Res (2011) 45(8):1067–76. doi: 10.1016/j.jpsychires.2011.01.002
42. Diwadkar VA, Pruitt P, Zhang A, Radwan J, Keshavan MS, Murphy E, et al. The neural correlates of performance in adolescents at risk for schizophrenia: Inefficiently increased cortico-striatal responses measured with fMRI. J Psychiatr Res (2012b) 46(1):12–21. doi: 10.1016/j.jpsychires.2011.09.016
43. Thermenos HW, Makris N, Whitfield-Gabrieli S, Brown AB, Giuliano AJ, Lee EH, et al. A functional MRI study of working memory in adolescents and young adults at genetic risk for bipolar disorder: Preliminary findings. Bipolar Disord (2011) 13(3):272–86. doi: 10.1111/j.1399-5618.2011.00920.x
44. Diwadkar VA, Bakshi N, Gupta G, Pruitt P, White R. Dysfunction and dysconnection in cortical-striatal networks during sustained attention: Genetic risk for schizophrenia or bipolar disorder and its impact on brain network function. Front Psychiatry (2014) 5:50. doi: 10.3389/fpsyt.2014.00050
45. Deveney CM, Connolly ME, Jenkins SE, Kim P, Fromm SJ, Brotman MA, et al. Striatal dysfunction during failed motor inhibition in children at risk for bipolar disorder. Prog Neuropsychopharmacol Biol Psychiatry (2012) 38(2):127–33. doi: 10.1016/j.pnpbp.2012.02.014
46. Kim P, Jenkins SE, Connolly ME, Deveney CM, Fromm SJ, Brotman MA, et al. Neural correlates of cognitive flexibility in children at risk for bipolar disorder. J Psychiatr Res (2012) 46(1):22–30. doi: 10.1016/j.jpsychires.2011.09.015
47. Pagliaccio D, Wiggins JL, Adleman NE, Harkins E, Curhan A, Towbin KE, et al. Behavioral and Neural Sustained Attention Deficits in Bipolar Disorder and Familial Risk of Bipolar Disorder. Biol Psychiatry (2017) 82(9):669–78. doi: 10.1016/j.biopsych.2016.09.006
48. Manelis A, Ladouceur CD, Graur S, Monk K, Bonar LK, Hickey MB, et al. Altered functioning of reward circuitry in youth offspring of parents with bipolar disorder. psychol Med (2016) 46(1):197–208. doi: 10.1017/S003329171500166X
49. Singh MK, Kelley RG, Howe ME, Reiss AL, Gotlib IH, Chang KD. Reward processing in healthy offspring of parents with bipolar disorder. JAMA Psychiatry (2014) 71(10):1148–56. doi: 10.1001/jamapsychiatry.2014.1031
50. Soehner AM, Bertocci MA, Manelis A, Bebko G, Ladouceur CD, Graur S, et al. Preliminary investigation of the relationships between sleep duration, reward circuitry function, and mood dysregulation in youth offspring of parents with bipolar disorder. J Affect Disord (2016) 205:144–53. doi: 10.1016/j.jad.2016.03.074
51. Brotman MA, Deveney CM, Thomas LA, Hinton K, Yi J, Pine DS, et al. Parametric modulation of neural activity during face emotion processing in unaffected youth at familial risk for bipolar disorder. Neuropsychopharmacology (2013) 38(SUPPL. 2):S132–S3. doi: 10.1111/bdi.12193
52. Barbour T, Murphy E, Pruitt P, Eickhoff SB, Keshavan MS, Rajan U, et al. Reduced intra-amygdala activity to positively valenced faces in adolescent schizophrenia offspring. Schizophr Res (2010) 123(2-3):126–36. doi: 10.1016/j.schres.2010.07.023
53. Diwadkar VA, Wadehra S, Pruitt P, Keshavan MS, Rajan U, Zajac-Benitez C, et al. Disordered corticolimbic interactions during affective processing in children and adolescents at risk for schizophrenia revealed by functional magnetic resonance imaging and dynamic causal modeling. Arch Gen Psychiatry (2012) 69(3):231–42. doi: 10.1001/archgenpsychiatry.2011.1349
54. Hart SJ, Bizzell J, McMahon MA, Gu H, Perkins DO, Belger A. Altered fronto–limbic activity in children and adolescents with familial high risk for schizophrenia. Psychiatry Res: Neuroimaging (2013) 212(1):19–27. doi: 10.1016/j.pscychresns.2012.12.003
55. Ladouceur CD, Diwadkar VA, White R, Bass J, Birmaher B, Axelson DA, et al. Fronto-limbic function in unaffected offspring at familial risk for bipolar disorder during an emotional working memory paradigm. Dev Cogn Neurosci (2013) 5:185–96. doi: 10.1016/j.dcn.2013.03.004
56. Manelis A, Ladouceur CD, Graur S, Monk K, Bonar LK, Hickey MB, et al. Altered amygdala-prefrontal response to facial emotion in offspring of parents with bipolar disorder. Brain: A J Neurol (2015) 138(9):2777–90. doi: 10.1093/brain/awv176
57. Olsavsky AK, Brotman MA, Rutenberg JG, Muhrer EJ, Deveney CM, Fromm SJ, et al. Amygdala Hyperactivation During Face Emotion Processing in Unaffected Youth at Risk for Bipolar Disorder. J Am Acad Child Adolesc Psychiatry (2012) 51(3):294–303. doi: 10.1016/j.jaac.2011.12.008
58. Tseng WL, Bones BL, Kayser RR, Olsavsky AK, Fromm SJ, Pine DS, et al. An fMRI study of emotional face encoding in youth at risk for bipolar disorder. Eur Psychiatry (2015) 30(1):94–8. doi: 10.1016/j.eurpsy.2014.05.004
59. Welge JA, Saliba LJ, Strawn JR, Eliassen JC, Patino LR, Adler CM, et al. Neurofunctional differences among youth with and at varying risk for developing mania. J Am Acad Child Adolesc Psychiatry (2016) 55(11):980–9. doi: 10.1016/j.jaac.2016.08.006
60. Eickhoff SB, Bzdok D, Laird AR, Kurth F, Fox PT. Activation likelihood estimation meta-analysis revisited. NeuroImage (2012) 59(3):2349–61. doi: 10.1016/j.neuroimage.2011.09.017
61. Zhang R, Picchioni M, Allen P, Toulopoulou T. Working Memory in Unaffected Relatives of Patients With Schizophrenia: A Meta-Analysis of Functional Magnetic Resonance Imaging Studies. Schizophr Bull (2016) 42(4):1068–77. doi: 10.1093/schbul/sbv221
62. Schneider F, Habel U, Reske M, Kellermann T, Stöcker T, Shah NJ, et al. Neural correlates of working memory dysfunction in first-episode schizophrenia patients: An fMRI multi-center study. Schizophr Res (2007) 89(1):198–210. doi: 10.1016/j.schres.2006.07.021
63. Brandt CL, Eichele T, Melle I, Sundet K, Server A, Agartz I, et al. Working memory networks and activation patterns in schizophrenia and bipolar disorder: comparison with healthy controls. Br J Psychiatry (2014) 204(4):290–8. doi: 10.1192/bjp.bp.113.129254
64. Diamond A. Executive functions. Annu Rev Psychol (2013) 64:135–68. doi: 10.1146/annurev-psych-113011-143750
65. Eriksson J, Vogel Edward K, Lansner A, Bergström F, Nyberg L. Neurocognitive Architecture of Working Memory. Neuron (2015) 88(1):33–46. doi: 10.1016/j.neuron.2015.09.020
66. Glahn DC, Ragland JD, Abramoff A, Barrett J, Laird AR, Bearden CF, et al. Beyond hypofrontality: A quantitative meta-analysis of functional neuroimaging studies of working memory in schizophrenia. Hum Brain Mapp (2005) 25(1):60–9. doi: 10.1002/hbm.20138
67. Dell'Osso B, Cinnante C, Di Giorgio A, Cremaschi L, Palazzo MC, Cristoffanini M, et al. Altered prefrontal cortex activity during working memory task in Bipolar Disorder: A functional Magnetic Resonance Imaging study in euthymic bipolar I and II patients. J Affect Disord (2015) 184:116–22. doi: 10.1016/j.jad.2015.05.026
68. Albus M, Hubmann W, Mohr F, Tiedemann TV, Pechler S, Drießlein D, et al. Neurocognitive functioning in patients with first-episode schizophrenia: results of a prospective 15-year follow-up study. Eur Arch Psychiatry Clin Neurosci (2019). doi: 10.1007/s00406-019-01030-z
69. Minzenberg MJ, Laird AR, Thelen S, Carter CS, Glahn DC. Meta-analysis of 41 functional neuroimaging studies of executive function in schizophrenia. Arch Gen Psychiatry (2009) 66(8):811–22. doi: 10.1001/archgenpsychiatry.2009.91
70. Niendam TA, Laird AR, Ray KL, Dean YM, Glahn DC, Carter CS. Meta-analytic evidence for a superordinate cognitive control network subserving diverse executive functions. Cogn Affect Behav Neurosci (2012) 12(2):241–68. doi: 10.3758/s13415-011-0083-5
71. Quraishi S, Frangou S. Neuropsychology of bipolar disorder: a review. J Affect Disord (2002) 72(3):209–26.
72. Fleck DE, Eliassen JC, Durling M, Lamy M, Adler CM, DelBello MP, et al. Functional MRI of sustained attention in bipolar mania. Mol Psychiatry (2012) 17(3):325–36. doi: 10.1038/mp.2010.108
73. Stephen BKE, Robert RME. Contemporary learning theories. Pavlovian conditioning and the status of traditional learning theory. Klein SB, Mowrer RR, editors. New York, New York: Psychology Press (2014).
74. Haber SN, Knutson B. The reward circuit: linking primate anatomy and human imaging. Neuropsychopharmacol Off Publ Am Coll Neuropsychopharmacol (2010) 35(1):4–26. doi: 10.1038/npp.2009.129
75. Hollerman JR, Tremblay L, Schultz W. Involvement of basal ganglia and orbitofrontal cortex in goal-directed behavior. Prog Brain Res (2000) 126:193–215. doi: 10.1016/S0079-6123(00)26015-9
76. Mason L, O’Sullivan N, Blackburn M, Bentall R, El-Deredy W. I Want It Now! Neural Correlates of Hypersensitivity to Immediate Reward in Hypomania. Biol Psychiatry (2012) 71(6):530–7. doi: 10.1016/j.biopsych.2011.10.008
77. Nielsen M, Rostrup E, Wulff S, Bak N, Lublin H, Kapur S, et al. Alterations of the Brain Reward System in Antipsychotic Naïve Schizophrenia Patients. Biol Psychiatry (2012) 71(10):898–905. doi: 10.1016/j.biopsych.2012.02.007
78. Delvecchio G, Fossati P, Boyer P, Brambilla P, Falkai P, Gruber O, et al. Common and distinct neural correlates of emotional processing in Bipolar Disorder and Major Depressive Disorder: a voxel-based meta-analysis of functional magnetic resonance imaging studies. Eur Neuropsychopharmacol J Eur Coll Neuropsychopharmacol (2012) 22(2):100–13. doi: 10.1016/j.euroneuro.2011.07.003
79. van der Velde J, Opmeer EM, Liemburg EJ, Bruggeman R, Nieboer R, Wunderink L, et al. Lower prefrontal activation during emotion regulation in subjects at ultrahigh risk for psychosis: an fMRI-study. NPJ Schizophr (2015) 1(1):15026. doi: 10.1038/npjschz.2015.26
80. Barrett LF, Mesquita B, Ochsner KN, Gross JJ. The Experience of Emotion. Annu Rev Psychol (2007) 58(1):373–403. doi: 10.1146/annurev.psych.58.110405.085709
81. Beauchaine TP, Crowell SE, Leshin JC, Lindquist KA. Neuroimaging of Emotion Dysregulation. In: The Oxford Handbook of Emotion Dysregulation. Oxford University Press: Oxford Handbooks Online (2020).. doi: 10.1093/oxfordhb/9780190689285.013.14
82. Cohen AS, Minor KS. Emotional Experience in Patients With Schizophrenia Revisited: Meta-analysis of Laboratory Studies. Schizophr Bull (2008) 36(1):143–50. doi: 10.1093/schbul/sbn061
83. Summers M, Papadopoulou K, Bruno S, Cipolotti L, Ron MA. Bipolar I and bipolar II disorder: cognition and emotion processing. psychol Med (2006) 36(12):1799–809. doi: 10.1017/S0033291706008804
84. Phillips ML, Drevets WC, Rauch SL, Lane R. Neurobiology of emotion perception II: implications for major psychiatric disorders. Biol Psychiatry (2003) 54(5):515–28. doi: 10.1016/S0006-3223(03)00171-9
85. Mercer L, Becerra R. A unique emotional processing profile of euthymic bipolar disorder? A critical review. J Affect Disord (2013) 146(3):295–309. doi: 10.1016/j.jad.2012.10.030
86. Gottesman II, Gould TD. The Endophenotype Concept in Psychiatry: Etymology and Strategic Intentions. Cogn Affect Behav Neurosci (2003) 160(4):636–45. doi: 10.1176/appi.ajp.160.4.636
87. Frangou S. Brain structural and functional correlates of resilience to Bipolar Disorder. Front Hum Neurosci (2012) 5:184. doi: 10.3389/fnhum.2011.00184
88. Klosterkötter J, Schultze-Lutter F, Bechdolf A, Ruhrmann S. Prediction and prevention of schizophrenia: what has been achieved and where to go next? World Psychiatry (2011) 10(3):165–74. doi: 10.1002/j.2051-5545.2011.tb00044.x
89. Fusar-Poli P, Bonoldi I, Yung AR, Borgwardt S, Kempton MJ, Valmaggia L, et al. Predicting Psychosis: Meta-analysis of Transition Outcomes in Individuals at High Clinical Risk. Arch Gen Psychiatry (2012) 69(3):220–9. doi: 10.1001/archgenpsychiatry.2011.1472
90. Schultze-Lutter F, Michel C, Schmidt SJ, Schimmelmann BG, Maric NP, Salokangas RKR, et al. EPA guidance on the early detection of clinical high risk states of psychoses. Eur Psychiatry (2015) 30(3):405–16. doi: 10.1016/j.eurpsy.2015.01.010
91. Fusar-Poli P, Borgwardt S, Bechdolf A, Addington J, Riecher-Rössler A, Schultze-Lutter F, et al. The Psychosis High-Risk State: A Comprehensive State-of-the-Art Review. JAMA Psychiatry (2013) 70(1):107–20. doi: 10.1001/jamapsychiatry.2013.269
92. Andreou C, Borgwardt S. Structural and functional imaging markers for susceptibility to psychosis. Mol Psychiatry (2020). doi: 10.1038/s41380-020-0679-7
93. Wilkinson D, Halligan P. The relevance of behavioural measures for functional-imaging studies of cognition. Nat Rev Neurosci (2004) 5(1):67–73. doi: 10.1038/nrn1302
94. Amaro E, Barker GJ. Study design in fMRI: Basic principles. Brain Cogn (2006) 60(3):220–32. doi: 10.1016/j.bandc.2005.11.009
95. Aubert-Broche B, Fonov VS, García-Lorenzo D, Mouiha A, Guizard N, Coupé P, et al. A new method for structural volume analysis of longitudinal brain MRI data and its application in studying the growth trajectories of anatomical brain structures in childhood. NeuroImage (2013) 82:393–402. doi: 10.1016/j.neuroimage.2013.05.065
96. Wierenga LM. The Development of Brain Structure and Connectivity. Utrecht, Utrecht, The Netherlands: the Brain Center Rudolf Magnus, Department of Psychiatry, University Medical Center (2016).
97. Brown Timothy T, Kuperman Joshua M, Chung Y, Erhart M, McCabe C, Hagler Donald J, et al. Neuroanatomical Assessment of Biological Maturity. Curr Biol (2012) 22(18):1693–8. doi: 10.1016/j.cub.2012.07.002
98. Marcos-Vidal L, Martínez-García M, Pretus C, Garcia-Garcia D, Martínez K, Janssen J, et al. Local functional connectivity suggests functional immaturity in children with attention-deficit/hyperactivity disorder. Hum Brain Mapp (2018) 39(6):2442–54. doi: 10.1002/hbm.24013
99. Plessen KJ, Allen EA, Eichele H, van Wageningen H, Hovik MF, Sorensen L, et al. Reduced error signalling in medication-naive children with ADHD: associations with behavioural variability and post-error adaptations. J Psychiatry Neurosci Jpn (2016) 41(2):77–87. doi: 10.1503/jpn.140353
Keywords: fMRI—functional magnetic resonance imaging, neurocognitive function, familial high-risk, schizophrenia, bipolar disorder, children, adolescents
Citation: Johnsen LK, Ver Loren van Themaat AH, Larsen KM, Burton BK, Baaré WFC, Madsen KS, Nordentoft M, Siebner HR and Plessen KJ (2020) Alterations in Task-Related Brain Activation in Children, Adolescents and Young Adults at Familial High-Risk for Schizophrenia or Bipolar Disorder - A Systematic Review. Front. Psychiatry 11:632. doi: 10.3389/fpsyt.2020.00632
Received: 24 February 2020; Accepted: 17 June 2020;
Published: 10 July 2020.
Edited by:
Frauke Schultze-Lutter, Heinrich Heine University Düsseldorf, GermanyReviewed by:
Jochen Kindler, University of Bern, SwitzerlandCopyright © 2020 Johnsen, Ver Loren van Themaat, Larsen, Burton, Baaré, Madsen, Nordentoft, Siebner and Plessen. This is an open-access article distributed under the terms of the Creative Commons Attribution License (CC BY). The use, distribution or reproduction in other forums is permitted, provided the original author(s) and the copyright owner(s) are credited and that the original publication in this journal is cited, in accordance with accepted academic practice. No use, distribution or reproduction is permitted which does not comply with these terms.
*Correspondence: Line Korsgaard Johnsen, bGluZS5rb3JzZ2FhcmQuam9obnNlbkByZWdpb25oLmRr; Kerstin Jessica Plessen, a2Vyc3Rpbi5qZXNzaWNhLnBsZXNzZW5AcmVnaW9uaC5kaw==
Disclaimer: All claims expressed in this article are solely those of the authors and do not necessarily represent those of their affiliated organizations, or those of the publisher, the editors and the reviewers. Any product that may be evaluated in this article or claim that may be made by its manufacturer is not guaranteed or endorsed by the publisher.
Research integrity at Frontiers
Learn more about the work of our research integrity team to safeguard the quality of each article we publish.