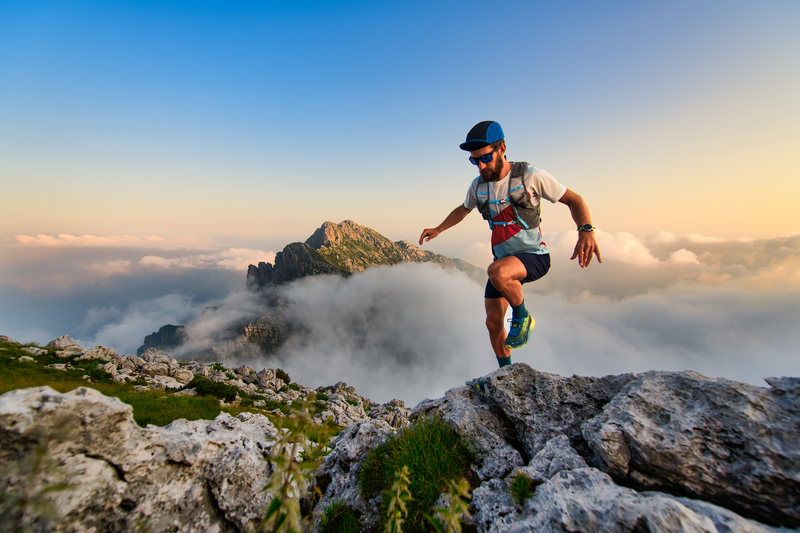
94% of researchers rate our articles as excellent or good
Learn more about the work of our research integrity team to safeguard the quality of each article we publish.
Find out more
ORIGINAL RESEARCH article
Front. Psychiatry , 07 May 2020
Sec. Neuroimaging
Volume 11 - 2020 | https://doi.org/10.3389/fpsyt.2020.00388
Objectives: Noninvasive brain stimulation (NIBS) is an emerging tool for treating autism spectrum disorder (ASD). Exploring new stimulation targets may improve the efficacy of NIBS for ASD.
Materials and Methods: We first conducted a meta-analysis on 170 functional magnetic resonance imaging studies to identify ASD-associated brain regions. We then performed resting state functional connectivity analysis on 70 individuals with ASD to investigate brain surface regions correlated with these ASD-associated regions and identify potential NIBS targets for ASD.
Results: We found that the medial prefrontal cortex, angular gyrus, dorsal lateral prefrontal cortex, inferior frontal gyrus, superior parietal lobe, postcentral gyrus, precentral gyrus, middle temporal gyrus, superior temporal sulcus, lateral occipital cortex, and supplementary motor area/paracentral gyrus are potential locations for NIBS in ASD.
Conclusion: Our findings may shed light on the development of new NIBS targets for ASD.
Autism spectrum disorder (ASD) is a highly prevalent disorder (1). Despite decades of research, the treatment of ASD is far from satisfactory (2). To date, only a handful of treatment options have been shown to ameliorate the symptoms associated with the disorder (3). Developing new intervention methods for ASD is therefore urgently needed.
Noninvasive brain stimulation (NIBS) is an emerging tool for the treatment of ASD. Such a technique may include repetitive transcranial magnetic stimulation (rTMS), transcranial direct current stimulation (tDCS), and transcranial alternating current stimulation (tACS) (4, 5). Preliminary evidence has demonstrated the potentials of rTMS and tDCS in the treatment of ASD (6–8). However, results from recent meta-analyses regarding the effectiveness of these NIBS techniques in ASD treatment are inconsistent (9–11), necessitating that researchers further investigate and improve NIBS treatment.
Refining stimulation targets may be a promising way to improve the treatment effect of NIBS. Currently, multiple NIBS targets for ASD treatment have been explored, including the dorsal lateral prefrontal cortex (DLPFC), motor cortex, inferior frontal gyrus (IFG), dorsal medial prefrontal cortex (dmPFC), and temporoparietal junction (TPJ) (9, 10, 12–14). However, the rationale for choosing these areas is often ambiguous, significantly limiting the optimization of NIBS and its application in ASD.
Neuroimaging studies have discovered a large number of ASD-associated brain regions and have expanded our understanding of ASD pathophysiology (15–18). However, clinical translation is still limited. One way of selecting target brain regions for NIBS from neuroimaging research is through meta-analysis of previous studies. However, some brain regions identified from these meta-analyses, such as the fusiform gyrus and amygdala, are located in areas that are inaccessible for certain NIBS technologies such as tDCS.
Recently, investigators have started to apply resting state functional connectivity methods to optimize the locations of NIBS for depression treatment and have achieved some encouraging results, demonstrating the potential of resting state functional connectivity in refining NIBS target locations (19–21). Nevertheless, few studies have systematically investigated potential locations for ASD using brain imaging. This study aims to explore new potential target locations for NIBS in ASD treatment by combining meta-analysis and resting state functional connectivity methods. Specifically, we first performed an automated meta-analysis of ASD and defined regions of interest (ROI). Then, we investigated the resting state functional connectivity of those ROIs in 70 ASD patients to identify easily accessible locations for NIBS, particularly tDCS.
To extract ASD-associated ROIs, we used Neurosynth (22) (http://neurosynth.org/; accessed 11 March 2020) as a metadata reference of the neuroimaging literature. Under the search string “autism spectrum,” 170 fMRI studies were identified, and a uniformity test map was generated to identify ASD-associated brain regions. A complete list of the 170 fMRI studies extracted from Neurosynth can be found in Supplementary Material Table S1.
To create ASD-associated ROIs for further analysis, we first used xjView toolbox (http://www.alivelearn.net/xjview/) to identify the coordinates with peak z-scores within all clusters larger than 50 voxels on the uniformity test map. Then, 6-mm radius spherical masks centered on the identified peak coordinates were created using WFU_PickAtlas toolbox (version 3.0.5b, http://fmri.wfubmc.edu/software/PickAtlas). The ROIs were further refined by taking the overlap of the uniformity test map with the whole brain cortical masks (see Supplementary Material Figure S1) from the WFU_PickAtlas for the purpose of maintaining regional specificity.
Data were extracted from Autism Brain Imaging Data Exchange II. Subjects were selected from three data sites: Georgetown University, New York University (sample 1 and sample 2), and Kennedy Krieger Institute from Autism Brain Imaging Data Exchange (ABIDEII) (23). We selected subjects based on the following criteria: (1) 5–12 years old; (2) full-scale IQ (FIQ) scores > 80; (3) diagnosis of ASD based on DSM-IV-TR and assessed with the Autism Diagnostic Observation Schedule (ADOS) (24) and/or the Autism Diagnostic Interview–Revised (ADI-R) (25); (4) no history of attention-deficit hyperactivity disorder, oppositional defiant disorder, or phobia. All procedures from these three sites were approved by their local Institutional Review Boards.
The resting-state fMRI and high-resolution T1-weighted brain structural images were acquired on 3T MRI scanners (see details of the acquisition parameters in http://fcon_1000.projects.nitrc.org/indi/abide/abide_II.html).
The images were preprocessed in CONN version 18a (https://sites.google.com/view/conn/) (26) and SPM 12 (http://www.fil.ion.ucl.ac.uk/spm/) using CONN's default preprocessing pipeline. The preprocessing steps included slice-timing correction, realignment, normalization (3×3×3 mm3 in MNI space), and smoothing (6×6×6 mm3). During preprocessing, the Artifact Detection Tool (https://www.nitrc.org/projects/artifact_detect/) was used to detect outliers (> 3 SD and/or >0.5mm). The outliers were used for subsequent scrubbing regression. The structural images were segmented and used to create gray matter, white matter (WM), and cerebral spinal fluid (CSF) masks of each subject. Then, linear regression using WM & CSF signals (CompCor; 5 components for WM and CSF), linear trend, subject motion (six rotation/translation motion parameters and six first-order temporal derivatives), and outliers (scrubbing) was conducted to remove confounding effects. Afterwards, the residual BOLD time series were band-pass filtered (0.008–0.09 Hz).
Similar to our previous study (27, 28), to explore potential brain surface regions related to ASD, 21 ROIs identified from the meta-analysis were used to conduct seed-to-voxel functional connectivity analyses on resting-state fMRI data from all selected ASD subjects. The residual BOLD time course was extracted from the 21 ROIs, and Pearson's correlation coefficients were computed between the ROIs and all other brain voxels for each subject to create subject-level seed maps. The resulting correlation coefficients were subsequently transformed into z-scores to increase normality. At the group level, all subject-level seed maps were included in a one-sample t-test to obtain a group-level correlation map.
After the one-sample t-test, a brain surface mask was created to exclude brain regions that are not on the brain surface (inaccessible to NIBS). The regions included in the brain surface mask were the bilateral pre and postcentral gyri; superior and middle frontal gyri; superior, inferior, and middle occipital gyri; superior and inferior parietal lobules; supramarginal gyrus; angular gyrus; superior temporal gyrus; superior temporal pole; middle temporal gyrus; middle temporal pole; inferior temporal gyrus; opercular inferior frontal gyrus; Rolandic operculum; triangular inferior frontal gyrus; superior medial frontal gyrus; calcarine sulcus; orbital middle, superior, and inferior frontal gyri; orbital medial frontal gyrus; supplementary motor area; paracentral lobule; precuneus; and cuneus (see Supplementary Material Figure S1 for detailed mask image).
Similar to our previous study (27, 28), three different pipelines were applied to identify potential brain surface regions for NIBS in ASD (Figure 1). The most straightforward approach was pipeline 1, which used the meta-analysis to identify brain areas associated with ASD. Unfortunately, most of these brain areas are not located on the brain surface and therefore may be inaccessible to neuromodulation methods. We thus also employed pipelines 2 and 3 to identify surface brain areas that are functionally linked to deep brain structures associated with ASD, and hopefully stimulating these surface areas may influence the function of the deep brain areas.
Figure 1 Methods and tools. ROI, regions of interest; ASD, autism spectrum disorder; FC, functional connectivity.
Pipeline 1. We selected brain surface clusters in the uniformity test map from the meta-analysis by applying the whole brain cortical mask. These clusters represent potential brain surface regions (that are accessible to NIBS) directly involved in the pathophysiology of ASD.
Pipeline 2. The 21 refined ASD-associated ROIs from the meta-analysis were combined to form one ROI, which represented the ASD network for seed-to-voxel connectivity analysis in CONN. Then, we selected 4–6 brain surface clusters with the largest peak z-scores among all clusters with a voxel size larger than 50 and intensity larger than 4 on the group-level correlation map (positive and negative correlation maps separately). These clusters represent the brain surface regions that have the strongest correlations with the ASD functional network. A voxel-wise level threshold of p < 0.001 and a cluster level family-wise error (FWE) of p < 0.05 were applied to obtain group-level correlation maps of the ROIs.
Pipeline 3. We saved the group-level correlation maps of each ASD-associated ROI to a binary mask. The binary masks of all ROIs were added together to form a third-level map, positive correlation map, and negative correlation map separately. The intensity of each voxel in the third-level map may represent the number of ASD ROIs correlated to the voxel. Then, we selected 4–6 brain surface clusters with the largest peak z-scores among all clusters with a voxel size larger than 50 and intensity larger than 4 on the third-level correlation map as potential regions. These clusters represent the brain surface regions correlated with the largest number of ASD ROIs. A voxel-wise level threshold of p < 0.001 and a cluster level family-wise error (FWE) of p < 0.05 were applied to obtain group-level correlation maps of the ROIs.
The results from the three pipelines were mapped onto a standard brain using Surf Ice (https://www.nitrc.org/projects/surfice/) and a standard head using MRIcroGL (http://www.mccauslandcenter.sc.edu/mricrogl/) with the international 10-20 system in MNI space. The MNI coordinates of the 10-20 system were extracted from a previous study (29).
Demographic and clinical characteristics of the study groups are summarized in Table 1. In total, 70 ASD subjects (58 males) were included in the study. The mean age of the study group was 8.98 ± 1.97 (SD) years old with an average FIQ of 112.79 ± 16.67 (SD).
Twenty-one peak coordinates were identified from the uniformity test map of the meta-analysis (Table 2). The 21 coordinates were then used to create 6-mm radius spherical masks (see Supplementary Material Figure S2 for the 21 spherical masks). The masks included the bilateral hippocampus/amygdala, bilateral fusiform gyrus, medial prefrontal cortex (mPFC), bilateral insula, bilateral ventral lateral prefrontal cortex (vlPFC), bilateral dorsal lateral prefrontal cortex (dlPFC), bilateral supplementary motor area (SMA), bilateral caudate, bilateral angular gyrus (AG), posterior cingulate gyrus (PCC), left middle temporal gyrus (MTG), left lateral occipital gyrus, right superior temporal gyrus (STG), left frontal eye field (FEF), left superior parietal gyrus, left postcentral gyrus, and left precentral gyrus. The masks were refined by taking the overlap of the masks and the original forward inference map. Then, the refined masks were used as ROIs in the seed-to-voxel connectivity analysis.
The results of the three pipelines were mapped onto a standard brain and a standard head in MNI space (Table 3 and Figure 2).
Figure 2 Potential locations for noninvasive brain stimulation (NIBS) in autism spectrum disorder (ASD) identified from the three pipelines. IFG, inferior frontal gyrus; AG, angular gyrus; LOC, lateral occipital cortex; mPFC, medial prefrontal cortex; STS, anterior superior temporal sulcus; MTG, middle temporal gyrus; dlPFC, dorsolateral prefrontal cortex; SMG, supramarginal gyrus; SPL, superior parietal lobule.
In Pipeline 1, the mPFC, bilateral AG, bilateral dlPFC, inferior frontal gyrus (IFG), precentral gyrus, MTG/superior temporal sulcus (STS), lateral occipital cortex (LOC), left SMA/FEF, superior parietal lobe (SPL), and postcentral gyrus were identified as brain surface regions that may be directly involved in the pathophysiology of ASD (see Supplementary Material Figure S3 for statistical maps of pipeline 1). The 10-20 system coordinates corresponding to the centers of these regions were located approximately at Fz, P3, and P4 (left and right AG), posterior to F3 and F4 (left and right dlPFC), posterior to F7 and F8 (left and right IFG), anterior to C3 and C4 (left and right precentral gyrus), center at T3 and T4 (left and right MTG), center at T5 and T6 (left and right LOC), posterior to Fz, anterior to P3, and posterior to C3.
In Pipeline 2, the bilateral SPL, SMA / paracentral gyrus, right dlPFC, AG, and postcentral gyrus were identified as brain surface regions positively correlated with the ASD network (see Supplementary Material Figure S4 for statistical maps of pipeline 2). The 10-20 system coordinates corresponding to the centers of these regions were located approximately anterior to P3 and P4 (left and right SPL), posterior to Cz, posterior to F4, center at P4, and posterior to C4, respectively. No cluster was found to be negatively correlated with the ASD network.
In pipeline 3, the mPFC, bilateral precuneus, temporal pole (TPO)/MTG/STS, AG, dlPFC, IFG, SPL, postcentral gyrus, LOC, right supramarginal gyrus (SMG), and left precentral gyrus were identified as brain surface regions positively correlated with ASD ROIs (see Supplementary Material Figure S5 for positive statistical maps of pipeline 3). The 10-20 system coordinates corresponding to the centers of these regions were located approximately at Fz, posterior to Cz, T3, and T4 (left and right TPO/MTG/STS), P3 and P4 (left and right AG), posterior to F3 and F4 (left and right dlPFC), posterior to F7 and F8 (left and right IFG), anterior to P3 and P4 (left and right SPL), posterior to C3 and C4 (left and right postcentral gyrus), center at T5 and T6 (left and right LOC), anterior to P4, and anterior to C3, respectively. The mPFC, bilateral dlPFC, precuneus, and right SMG were found to be brain surface regions negatively correlated with ASD ROIs (see Supplementary Material Figure S6 for negative statistical maps of pipeline 3). The 10-20 system coordinates corresponding to the centers of these regions were located approximately at Fz, posterior to F3 and F4 (left and right dlPFC), posterior to Cz, and anterior to P4.
In this study, we combined resting-state functional connectivity and meta-analysis to identify potential NIBS targets for ASD. We found the brain regions overlapping between the meta-analysis and seed-based analysis to be potential targets, including the mPFC, bilateral AG, dlPFC, IFG, MTG/STS, LOC, left SPL, postcentral gyrus, precentral gyrus, and SMA. Stimulation locations on the scalp corresponding to these brain targets were also provided based on the EEG 10-20 system.
Consistent with the general consensus on cortical stimulation sites for ASD (10, 12), we identified the bilateral dlPFC, IFG, and AG as potential NIBS targets. The effectiveness of stimulating these three regions for ASD has been tested in previous studies (7, 30, 31).
Several neuropsychology studies have revealed impaired executive function in patients with pervasive developmental disorders such as ASD (32). Executive function encompasses a set of mental processes involved in planning, working memory, attention, problem solving, verbal reasoning, and mental flexibility (33), all of which are highly associated with activation of the dlPFC (34). As the most preferable target region, stimulation of the dlPFC may induce reduction in comorbid depression (35) and social-related impairments (36, 37) and yield improvement in attention (36) in individuals with ASD (38, 39). Sokhadze and colleagues reported improved executive functioning in individuals with ASD as evidence of normalization of event-related potential responses and behavioral accuracy after 1 Hz rTMS to the dlPFC (6). Thus, the dlPFC may be a potential target to treat symptoms associated with executive function and depression.
The IFG has been extensively associated with functions such as language processing, response inhibition, and social cognition and may be directly involved in the pathophysiology of ASD (40–46). For instance, Grace and her colleagues reported that activation in the left IFG was reduced in individuals with ASD compared to typically developing controls during speech stimulation (47). Moreover, Leehe et al. showed that anodal tDCS targeting the right IFG (rIFG) can modulate participants' emotional ratings to social touch.
As part of the TPJ, the AG integrates multi-sensory and cognitive processes that are implicated in the theory of mind and the attention network (48, 49). A recent study found that tDCS on the TPJ can influence social-cognitive performance as assessed by the Autism Spectrum Quotient (AQ) score (50). These studies demonstrate the functional alterations of the IFG and AG in ASD and provide support for using these regions as treatment targets, particularly in language and attention-related symptoms.
The motor system is another commonly used target for ASD (5). We found the left precentral gyrus and SMA in both the meta-analysis and seed-based analysis pipelines. A recent systematic review (11) on TMS neurophysiology revealed motor cortex excitatory and inhibitory imbalances in ASD, thereby providing a basis for targeting these regions. Thus, we speculate that the region may be associated with repetitive behavior and other motor-related symptoms.
In addition, our results suggest that the mPFC, bilateral MTG/STS, LOC, left SPL, and postcentral gyrus as other overlapping results of the meta-analysis and seed-based analysis should also be considered as potential targets for ASD treatment. Numerous functional imaging studies have demonstrated the important role of the prefrontal cortex in social cognition (51, 52), and the mPFC is particularly highlighted in the pathophysiology of ASD in both functional imaging and gene expression studies (53). Indeed, Enticott et al. found that deep rTMS to the bilateral mPFC can reduce social-related impairment and social-related anxiety in ASD patients (37), indicating that the mPFC may be a target region for these symptoms.
An extensive body of literature has shown the STS to be an established node of a “social network” (54) involved in language processing and social perception (55, 56), and it is a key region of numerous functional differences between ASD and typically developing individuals (57, 58). Beauchamp et al. reported that applying single-pulse TMS to the STS could significantly disrupt the McGurk effect (59), a perceptual phenomenon integrating hearing and vision in speech perception. Moreover, Daniel et al. found that theta burst TMS to the STS could produce a significant change in resting-state functional connectivity across the face-processing network (60). As findings have indicated that individuals with ASD may suffer from a weak McGurk effect and impaired face processing in daily settings (61, 62), these studies provide an interesting basis and guide for future stimulation of the STS in ASD treatment. Adjacent to the STG, the MTG is a brain area that is unique to humans (63). Previous studies have demonstrated the function of the MTG in language processing (64), and Acheson et al. reported a modulation effect on the language network when applying rTMS to the posterior MTG (64–67). These findings suggest that the STG and MTG may be potential targets for treating ASD symptoms related to language processing and social perception.
The SPL plays an important role in many cognitive, perceptive, and motor-related processes (68). In particular, event-related fMRI studies have shown that the SPL is critical for sensorimotor integration (69, 70), highlighting its potential role in the pathophysiology of repetitive and restrictive behaviors identified as additional core traits of ASD. Travers and colleagues reported that individuals with ASD had reduced fMRI activation in the SPL compared to typically developing individuals during motor-linked implicit learning, and they found that the more severe the traits of repetitive and restrictive behaviors, the greater the decrease in activation in the SPL (71). Furthermore, transcranial magnetic stimulation to the SPL was found to affect the planning of reaching movements (72). The SPL may therefore be considered a potential target for repetitive and restrictive behaviors.
As part of the lateral occipital-temporal complex (73), the LOC is an important region involved in object recognition (74) and multiple sensory integration. Studies have indicated that individuals with ASD may have difficulties integrating verbal and nonverbal cues during social interactions (75). Our previous study also found that reduced structural connectivity and resting-state brain activity in the LOC is associated with social communication deficits in boys with ASD (18).
Activation of the postcentral gyrus has been widely reported in previous studies when subjects were observing another person being touched (76, 77), suggesting the role of the postcentral gyrus in empathetic sharing of somatosensations. These findings suggest that this region may be used to relieve sensory-related symptoms in children with ASD (76, 77).
Finally, it is worth noting that identifying these locations may not necessarily be limited to NIBS, but may also be applied in other interventions such as scalp acupuncture (stimulating the area of scalp corresponding to brain regions believed to be involved in disorder pathology using acupuncture needles) and transcutaneous electrical nerve stimulation. Thus, results obtained from this study may facilitate the development of acupuncture and other therapeutic methods for the treatment of ASD.
There are several limitations to our study. First, the excitatory and inhibitory natures of these identified regions are indefinable using methods in this study. For some NIBS techniques like TMS, it is important to know the direction of the stimulation. How to apply and optimize different treatment modalities to target the brain areas identified in our study is beyond the scope of this manuscript. Investigators should consider the characteristics of different tools when attempting to stimulate these areas. Second, we do not know which pipeline is optimal for identifying potential NIBS targets for ASD. Regions identified from pipeline 1 are directly associated with ASD pathophysiology, regions identified from pipeline 2 have the strongest correlation with the ASD network, and regions identified from pipeline 3 correlate with the largest number of ASD ROIs. Understanding the derivative of these locations may help researchers choose target regions during clinical practice. Third, the meta-analysis conducted by Neurosynth is not flawless. Potential errors may occur during automatic extraction and synthesis of fMRI activation coordinates. However, several supporting analyses have been conducted to confirm the validity and sensitivity of Neurosynth-based meta-analysis and may provide evidence for the feasibility of this method. Finally, we applied peak z-scores to create spherical ROIs within these clusters. As a result, each ROI should have a similar size (voxel number), thus avoiding the potential influence of cluster size. Nevertheless, using original clusters as ROIs is also a reasonable method. One potential limitation of using the cluster derived from Neurosynth is that the cluster size may vary when different thresholds are applied.
In conclusion, we identified several potential NIBS targets and their corresponding stimulation locations on the scalp for the treatment of ASD. As ASD displays significant clinical heterogeneity with respect to stimulation sites, a clear link between neurobiological targets and clinical outcome measurements may be a future step toward optimizing NIBS for ASD treatment. Although further testing of these identified targets is needed, these results may help clinicians optimize the application of NIBS therapy in individuals with ASD.
Publicly available datasets were analyzed in this study. This data can be found at: http://fcon_1000.projects.nitrc.org/indi/abide/abide_II.html.
The study used public datasets from 3 centers. Please refer to http://fcon_1000.projects.nitrc.org/indi/abide/abide_II.html for details. Written informed consent to participate in this study was provided by the participants' legal guardian/next of kin.
YH contributed to data analysis and manuscript preparation. JK conceived the idea, contributed to data analysis and manuscript preparation, BZ contributed to data analysis and manuscript preparation, contributed to study design and manuscript preparation. JC contributed to manuscript revision. SY contributed to data analysis. GW and JP contributed to manuscript preparation.
JK holds equity in a startup company, MNT, and has pending patents to develop new neuromodulation tools.
The remaining authors declare that the research was conducted in the absence of any commercial or financial relationships that could be construed as a potential conflict of interest.
YH is supported by Chinese Scholarship Council (201706557085). JK is supported by R01AT008563, R33AT009310, R33AT009341, R34DA046635, and R21AT008707 from NIH.
The Supplementary Material for this article can be found online at: https://www.frontiersin.org/articles/10.3389/fpsyt.2020.00388/full#supplementary-material
1. Muhle RA, Reed HE, Stratigos KA, Veenstra-VanderWeele J. The Emerging Clinical Neuroscience of Autism Spectrum Disorder: A Review. JAMA Psychiatry (2018) 75(5):514–23. doi: 10.1001/jamapsychiatry.2017.4685
2. Lord C, Elsabbagh M, Baird G, Veenstra-Vanderweele J. Autism spectrum disorder. Lancet (2018) 392(10146):508–20. doi: 10.1016/S0140-6736(18)31129-2
3. Masi A, DeMayo MM, Glozier N, Guastella AJ. An Overview of Autism Spectrum Disorder, Heterogeneity and Treatment Options. Neurosci Bull (2017) 33(2):183–93. doi: 10.1007/s12264-017-0100-y
4. Obeso I, Oliviero A, Jahanshahi M. Editorial: Non-invasive Brain Stimulation in Neurology and Psychiatry. Front Neurosci (2016) 10:574. doi: 10.3389/fnins.2016.00574
5. Finisguerra A, Borgatti R, Urgesi C. Non-invasive Brain Stimulation for the Rehabilitation of Children and Adolescents With Neurodevelopmental Disorders: A Systematic Review. Front Psychol (2019) 10:135. doi: 10.3389/fpsyg.2019.00135
6. Sokhadze EM, Lamina EV, Casanova EL, Kelly DP, Opris I, Tasman A, et al. Exploratory Study of rTMS Neuromodulation Effects on Electrocortical Functional Measures of Performance in an Oddball Test and Behavioral Symptoms in Autism. Front Syst Neurosci (2018) 12:20. doi: 10.3389/fnsys.2018.00020
7. Gómez L, Vidal B, Maragoto C, Morales LM, Berrillo S, Vera Cuesta H, et al. Non-Invasive Brain Stimulation for Children with Autism Spectrum Disorders: A Short-Term Outcome Study. Behav Sci (Basel Switzerland) (2017) 7(3):63. doi: 10.3390/bs7030063
8. van Steenburgh JJ, arvaris M, Schretlen DJ, Vannorsdall TD, Gordon B. Balanced bifrontal transcranial direct current stimulation enhances working memory in adults with high-functioning autism: a sham-controlled crossover study. Mol Autism (2017) 8(1):40. doi: 10.1186/s13229-017-0152-x
9. Barahona-Correa JB, Velosa A, Chainho A, Lopes R, Oliveira-Maia AJ. Repetitive Transcranial Magnetic Stimulation for Treatment of Autism Spectrum Disorder: A Systematic Review and Meta-Analysis. Front Integr Neurosci (2018) 12:27. doi: 10.3389/fnint.2018.00027
10. Osorio AAC, Brunoni AR. Transcranial direct current stimulation in children with autism spectrum disorder: a systematic scoping review. Dev Med Child Neurol (2018) 61:298–304. doi: 10.1111/dmcn.14104
11. Masuda F, Nakajima S, Miyazaki T, Yoshida K, Tsugawa S, Wada M, et al. Motor cortex excitability and inhibitory imbalance in autism spectrum disorder assessed with transcranial magnetic stimulation: a systematic review. Trans Psychiatry (2019) 9(1):110. doi: 10.1038/s41398-019-0444-3
12. Cole EJ, Enticott PG, Oberman LM, Gwynette MF, Casanova MF, Jackson SLJ, et al. The Potential of Repetitive Transcranial Magnetic Stimulation for Autism Spectrum Disorder: A Consensus Statement. Biol Psychiatry (2019) 85(4):e21–2. doi: 10.1016/j.biopsych.2018.06.003
13. Enticott PG, Rinehart NJ, Tonge BJ, Bradshaw JL, Fitzgerald PB. Repetitive transcranial magnetic stimulation (rTMS) improves movement-related cortical potentials in autism spectrum disorders. Brain Stimul (2012) 5(1):30–7. doi: 10.1016/j.brs.2011.02.001
14. Panerai S, Tasca D, Lanuzza B, Trubia G, Ferri R, Musso S, et al. Effects of repetitive transcranial magnetic stimulation in performing eye-hand integration tasks: four preliminary studies with children showing low-functioning autism. Autism (2014) 18(6):638–50. doi: 10.1177/1362361313495717
15. Anagnostou E, Taylor MJ. Review of neuroimaging in autism spectrum disorders: what have we learned and where we go from here. Mol Autism (2011) 2(1):4–4. doi: 10.1186/2040-2392-2-4
16. Ecker C, Bookheimer SY, Murphy DG. Neuroimaging in autism spectrum disorder: brain structure and function across the lifespan. Lancet Neurol (2015) 14(11):1121–34. doi: 10.1016/S1474-4422(15)00050-2
17. Jung M, Tu Y, Park J, Jorgenson K, Lang C, Song W, et al. Surface-based shared and distinct resting functional connectivity in attention-deficit hyperactivity disorder and autism spectrum disorder. Br J Psychiatry (2018) 214(6):339–44. doi: 10.1192/bjp.2018.248
18. Jung M, Tu Y, Lang CA, Ortiz A, Park J, Jorgenson K, et al. Decreased structural connectivity and resting-state brain activity in the lateral occipital cortex is associated with social communication deficits in boys with autism spectrum disorder. NeuroImage (2019) 190:205–12. doi: 10.1016/j.neuroimage.2017.09.031
19. Fox MD, Buckner RL, White MP, Greicius MD, Pascual-Leone A. Efficacy of transcranial magnetic stimulation targets for depression is related to intrinsic functional connectivity with the subgenual cingulate. Biol Psychiatry (2012) 72(7):595–603. doi: 10.1016/j.biopsych.2012.04.028
20. Williams NR, Sudheimer KD, Bentzley BS, Pannu J, Stimpson KH, Duvio D, et al. High-dose spaced theta-burst TMS as a rapid-acting antidepressant in highly refractory depression. Brain (2018) 141(3):e18–8. doi: 10.1093/brain/awx379
21. Li C-T, Chen M-H, Juan C-H, Huang H-H, Chen L-F, Hsieh J-C, et al. Efficacy of prefrontal theta-burst stimulation in refractory depression: a randomized sham-controlled study. Brain (2014) 137(7):2088–98. doi: 10.1093/brain/awu109
22. Yarkoni T, Poldrack RA, Nichols TE, Van Essen DC, Wager TD. Large-scale automated synthesis of human functional neuroimaging data. Nat Methods (2011) 8(8):665–70. doi: 10.1038/nmeth.1635
23. Di Martino A, O'Connor D, Chen B, Alaerts K, Anderson JS, Assaf M, et al. Enhancing studies of the connectome in autism using the autism brain imaging data exchange II. Sci Data (2017) 4:170010. doi: 10.1038/sdata.2017.10
24. Lord C, Risi S, Lambrecht L, Cook EH Jr., Leventhal BL, DiLavore PC, et al. The autism diagnostic observation schedule-generic: a standard measure of social and communication deficits associated with the spectrum of autism. J Autism Dev Disord (2000) 30(3):205–23. doi: 10.1023/A:1005592401947
25. Lord C, Rutter M, Le Couteur A. Autism Diagnostic Interview-Revised: a revised version of a diagnostic interview for caregivers of individuals with possible pervasive developmental disorders. J Autism Dev Disord (1994) 24(5):659–85. doi: 10.1007/BF02172145
26. Whitfield-Gabrieli S, Nieto-Castanon A. Conn: a functional connectivity toolbox for correlated and anticorrelated brain networks. Brain Connect (2012) 2(3):125–41. doi: 10.1089/brain.2012.0073
27. Liu J, Zhang B, Wilson G, Kong J. New Perspective for Non-invasive Brain Stimulation Site Selection in Mild Cognitive Impairment: Based on Meta- and Functional Connectivity Analyses. Front Aging Neurosci (2019) 11:228. doi: 10.3389/fnagi.2019.00228
28. Zhang B, Liu J, Bao T, Wilson G, Park J, Zhao B. Locations for noninvasive brain stimulation in treating depressive disorders – a combination of meta-analysis and resting state functional connectivity analysis. Aust New Z J Psychiatry (2020). doi: 10.1177/0004867420920372
29. Cutini S, Scatturin, Zorzi M. A new method based on ICBM152 head surface for probe placement in multichannel fNIRS. Neuroimage (2011) 54(2):919–27. doi: 10.1016/j.neuroimage.2010.09.030
30. Hogeveen J, Obhi SS, Banissy MJ, Santiesteban I, Press C, Catmur C, et al. Task-dependent and distinct roles of the temporoparietal junction and inferior frontal cortex in the control of imitation. Soc Cognit Affect Neurosci (2015) 10(7):1003–9. doi: 10.1093/scan/nsu148
31. Santiesteban I, Banissy MJ, Catmur C, Bird G. Functional lateralization of temporoparietal junction - imitation inhibition, visual perspective-taking and theory of mind. Eur J Neurosci (2015) 42(8):2527–33. doi: 10.1111/ejn.13036
32. Craig F, Margari F, Legrottaglie AR, Palumbi R, de Giambattista C, Margari L. A review of executive function deficits in autism spectrum disorder and attention-deficit/hyperactivity disorder. Neuropsychiatr Dis Treat (2016) 12:1191–202. doi: 10.2147/NDT.S104620
33. Sawa T, Kodaira M, Oiji A, Sasayama D, Iwadare Y, Ushijima H. Dysfunction of orbitofrontal and dorsolateral prefrontal cortices in children and adolescents with high-functioning pervasive developmental disorders. Ann Gen Psychiatry (2013) 12(1):31–1. doi: 10.1186/1744-859X-12-31
34. Mars RB, Grol MJ. Dorsolateral Prefrontal Cortex, Working Memory, and Prospective Coding for Action. J Neurosci (2007) 27(8):1801–2. doi: 10.1523/JNEUROSCI.5344-06.2007
35. Gwynette MF, Lowe DW, Henneberry EA, Sahlem GL, Wiley MG, Alsarraf H, et al. Treatment of Adults with Autism and Major Depressive Disorder Using Transcranial Magnetic Stimulation: An Open Label Pilot Study. Autism Res (2020) 13(3):346–51. doi: 10.1002/aur.2266
36. Sokhadze EM, Baruth JM, Sears L, Sokhadze GE, El-Baz AS, Casanova MF. Prefrontal Neuromodulation Using rTMS Improves Error Monitoring and Correction Function in Autism. Appl Psychophysiol Biofeedback (2012) 37(2):91–102. doi: 10.1007/s10484-012-9182-5
37. Enticott PG, Fitzgibbon BM, Kennedy HA, Arnold SL, Elliot D, Peachey A, et al. A double-blind, randomized trial of deep repetitive transcranial magnetic stimulation (rTMS) for autism spectrum disorder. Brain Stimul (2014) 7(2):206–11. doi: 10.1016/j.brs.2013.10.004
38. Schneider HD, Hopp JP. The use of the Bilingual Aphasia Test for assessment and transcranial direct current stimulation to modulate language acquisition in minimally verbal children with autism. Clin Linguist Phon (2011) 25(6-7):640–54. doi: 10.3109/02699206.2011.570852
39. Gómez L, Vidal B, Denis M, Marín T, Maragoto C, Vera H, et al. Non-invasive brain stimulation in children with autism spectrum disorder. Brain Stimulation (2017) 10(2):347. doi: 10.1016/j.brs.2017.01.013
40. Nixon P, Lazarova J, Hodinott-Hill I, Gough P, Passingham R. The inferior frontal gyrus and phonological processing: an investigation using rTMS. J Cognit Neurosci (2004) 16(2):289–300. doi: 10.1162/089892904322984571
41. Tyler LK, Marslen-Wilson WD, Randall B, Wright P, Devereux BJ, Zhuang J, et al. Left inferior frontal cortex and syntax: function, structure and behaviour in patients with left hemisphere damage. Brain (2011) 134(Pt 2):415–31. doi: 10.1093/brain/awq369
42. Hampshire A, Chamberlain SR, Monti MM, Duncan J, Owen AM. The role of the right inferior frontal gyrus: inhibition and attentional control. Neuroimage (2010) 50(3):1313–9. doi: 10.1016/j.neuroimage.2009.12.109
43. Swick D, Ashley V, Turken ,U. Left inferior frontal gyrus is critical for response inhibition. BMC Neurosci (2008) 9:102–2. doi: 10.1186/1471-2202-9-102
44. Peled-Avron L, Glasner L, Gvirts HZ, Shamay-Tsoory SG. The role of the inferior frontal gyrus in vicarious social touch: A transcranial direct current stimulation (tDCS) study. Dev Cogn Neurosci (2019) 35:115–21. doi: 10.1016/j.dcn.2018.04.010
45. Kilner JM, Neal A, Weiskopf N, Friston KJ. Evidence of Mirror Neurons in Human Inferior Frontal Gyrus. J Neurosci (2009) 29(32):10153–9. doi: 10.1523/JNEUROSCI.2668-09.2009
46. Hartwright CE, Hansen PC, Apperly IA. Current knowledge on the role of the Inferior Frontal Gyrus in Theory of Mind - A commentary on Schurz and Tholen (2016). Cortex (2016) 85:133–6. doi: 10.1016/j.cortex.2016.10.005
47. Lai G, Pantazatos SP, Schneider H, Hirsch J. Neural systems for speech and song in autism. Brain (2012) 135(3):961–75. doi: 10.1093/brain/awr335
48. Saxe R, Wexler A. Making sense of another mind: The role of the right temporo-parietal junction. Neuropsychologia (2005) 43(10):1391–9. doi: 10.1016/j.neuropsychologia.2005.02.013
49. Vossel S, Geng JJ, Fink GR. Dorsal and Ventral Attention Systems:Distinct Neural Circuits but Collaborative Roles. Neurosci (2014) 20(2):150–9. doi: 10.1177/1073858413494269
50. Donaldson PH, Kirkovski M, Rinehart NJ, Enticott PG. Autism-relevant traits interact with temporoparietal junction stimulation effects on social cognition: a high-definition transcranial direct current stimulation and electroencephalography study. Eur J Neurosci (2018) 47(6):669–81. doi: 10.1111/ejn.13675
51. Forbes CE, Grafman J. The role of the human prefrontal cortex in social cognition and moral judgment. Annu Rev Neurosci (2010) 33:299–324. doi: 10.1146/annurev-neuro-060909-153230
52. Zahn R, Moll J, Paiva M, Garrido G, Krueger F, Huey ED, et al. The Neural Basis of Human Social Values: Evidence from Functional MRI. Cereb Cortex (2008) 19(2):276–83. doi: 10.1093/cercor/bhn080
53. Brumback AC, Ellwood IT, Kjaerby C, Iafrati J, Robinson S, Lee AT, et al. Identifying specific prefrontal neurons that contribute to autism-associated abnormalities in physiology and social behavior. Mol Psychiatry (2018) 23(10):2078–89. doi: 10.1038/mp.2017.213
54. Zahn R, Moll J, Krueger F, Huey ED, Garrido G, Grafman J. Social concepts are represented in the superior anterior temporal cortex. Proc Natl Acad Sci (2007) 104(15):6430–5. doi: 10.1073/pnas.0607061104
55. Bigler ED, Mortensen S, Neeley ES, Ozonoff S, Krasny L, Johnson M, et al. Superior temporal gyrus, language function, and autism. Dev Neuropsychol (2007) 31(2):217–38. doi: 10.1080/87565640701190841
56. Beauchamp MS. The social mysteries of the superior temporal sulcus. Trends Cognit Sci (2015) 19(9):489–90. doi: 10.1016/j.tics.2015.07.002
57. Redcay E. The superior temporal sulcus performs a common function for social and speech perception: implications for the emergence of autism. Neurosci Biobehav Rev (2008) 32(1):123–42. doi: 10.1016/j.neubiorev.2007.06.004
58. Lee JE, Bigler ED, Alexander AL, Lazar M, DuBray MB, Chung MK, et al. Diffusion tensor imaging of white matter in the superior temporal gyrus and temporal stem in autism. Neurosci Lett (2007) 424(2):127–32. doi: 10.1016/j.neulet.2007.07.042
59. Beauchamp MS, Nath AR, Pasalar S. fMRI-Guided transcranial magnetic stimulation reveals that the superior temporal sulcus is a cortical locus of the McGurk effect. J Neurosci (2010) 30(7):2414–7. doi: 10.1523/JNEUROSCI.4865-09.2010
60. Handwerker DA, Ianni G, Gutierrez B, Roopchansingh V, Gonzalez-Castillo J, Chen G, et al. Thetaburst TMS to the posterior superior temporal sulcus decreases resting-state fMRI connectivity across the face processing network. bioRxiv (2019), 794578. doi: 10.1101/794578
61. Zhang J, Meng Y, He J, Xiang Y, Wu C, Wang S, et al. McGurk Effect by Individuals with Autism Spectrum Disorder and Typically Developing Controls: A Systematic Review and Meta-analysis. J Autism Dev Disord (2019) 49(1):34–43. doi: 10.1007/s10803-018-3680-0
62. Golarai G, Grill-Spector K, Reiss AL. Autism and the development of face processing. Clin Neurosci Res (2006) 6(3):145–60. doi: 10.1016/j.cnr.2006.08.001
63. Xu J, Wang J, Fan L, Li H, Zhang W, Hu Q, et al. Tractography-based Parcellation of the Human Middle Temporal Gyrus. Sci Rep (2015) 5:18883. doi: 10.1038/srep18883
64. Davey J, Thompson HE, Hallam G, Karapanagiotidis T, Murphy C, De Caso I, et al. Exploring the role of the posterior middle temporal gyrus in semantic cognition: Integration of anterior temporal lobe with executive processes. NeuroImage (2016) 137:165–77. doi: 10.1016/j.neuroimage.2016.05.051
65. Sato W, Toichi M, Uono S, Kochiyama T. Impaired social brain network for processing dynamic facial expressions in autism spectrum disorders. BMC Neurosci (2012) 13(1):99. doi: 10.1186/1471-2202-13-99
66. Goel V, Gold B, Kapur S, Houle S. Neuroanatomical Correlates of Human Reasoning. J Cogn Neurosci (1998) 10(3):293–302. doi: 10.1162/089892998562744
67. Patriquin MA, DeRamus T, Libero LE, Laird A, Kana RK. Neuroanatomical and neurofunctional markers of social cognition in autism spectrum disorder. Hum Brain Mapp (2016) 37(11):3957–78. doi: 10.1002/hbm.23288
68. Wang J, Yang Y, Fan L, Xu J, Li C, Liu Y, et al. Convergent functional architecture of the superior parietal lobule unraveled with multimodal neuroimaging approaches. Hum Brain Mapp (2015) 36(1):238–57. doi: 10.1002/hbm.22626
69. Iacoboni M, Zaidel E. Interhemispheric visuo-motor integration in humans: the role of the superior parietal cortex. Neuropsychologia (2004) 42(4):419–25. doi: 10.1016/j.neuropsychologia.2003.10.007
70. Buneo CA, Andersen RA. The posterior parietal cortex: Sensorimotor interface for the planning and online control of visually guided movements. Neuropsychologia (2006) 44(13):2594–606. doi: 10.1016/j.neuropsychologia.2005.10.011
71. Travers BG, Kana RK, Klinger LG, Klein CL, Klinger MR. Motor learning in individuals with autism spectrum disorder: activation in superior parietal lobule related to learning and repetitive behaviors. Autism Res (2015) 8(1):38–51. doi: 10.1002/aur.1403
72. Busan P, Barbera C, Semenic M, Monti F, Pizzolato G, Pelamatti G, et al. Effect of Transcranial Magnetic Stimulation (TMS) on Parietal and Premotor Cortex during Planning of Reaching Movements. PloS One (2009) 4(2):e4621. doi: 10.1371/journal.pone.0004621
73. Beauchamp MS. See me, hear me, touch me: multisensory integration in lateral occipital-temporal cortex. Curr Opin Neurobiol (2005) 15(2):145–53. doi: 10.1016/j.conb.2005.03.011
74. Grill-Spector K, Kushnir T, Edelman S, Avidan G, Itzchak Y, Malach R. Differential Processing of Objects under Various Viewing Conditions in the Human Lateral Occipital Complex. Neuron (1999) 24(1):187–203. doi: 10.1016/S0896-6273(00)80832-6
75. Hubbard AL, McNealy K, Scott-Van Zeeland AA, Callan DE, Bookheimer SY, Dapretto M. Altered integration of speech and gesture in children with autism spectrum disorders. Brain Behav (2012) 2(5):606–19. doi: 10.1002/brb3.81
76. Blakemore S-J, Bristow D, Bird G, Frith C, Ward J. Somatosensory activations during the observation of touch and a case of vision–touch synaesthesia. Brain (2005) 128(7):1571–83. doi: 10.1093/brain/awh500
Keywords: autism spectrum disorders, meta-analysis, fMRI, noninvasive brain stimulation
Citation: Huang Y, Zhang B, Cao J, Yu S, Wilson G, Park J and Kong J (2020) Potential Locations for Noninvasive Brain Stimulation in Treating Autism Spectrum Disorders—A Functional Connectivity Study. Front. Psychiatry 11:388. doi: 10.3389/fpsyt.2020.00388
Received: 24 January 2020; Accepted: 17 April 2020;
Published: 07 May 2020.
Edited by:
Alireza Mohammadi, Baqiyatallah University of Medical Sciences, IranReviewed by:
Thomas Nickl-Jockschat, University of Iowa, United StatesCopyright © 2020 Huang, Zhang, Cao, Yu, Wilson, Park and Kong. This is an open-access article distributed under the terms of the Creative Commons Attribution License (CC BY). The use, distribution or reproduction in other forums is permitted, provided the original author(s) and the copyright owner(s) are credited and that the original publication in this journal is cited, in accordance with accepted academic practice. No use, distribution or reproduction is permitted which does not comply with these terms.
*Correspondence: Jian Kong, amtvbmcyQG1naC5oYXJ2YXJkLmVkdQ==
†These authors have contributed equally to this work
Disclaimer: All claims expressed in this article are solely those of the authors and do not necessarily represent those of their affiliated organizations, or those of the publisher, the editors and the reviewers. Any product that may be evaluated in this article or claim that may be made by its manufacturer is not guaranteed or endorsed by the publisher.
Research integrity at Frontiers
Learn more about the work of our research integrity team to safeguard the quality of each article we publish.