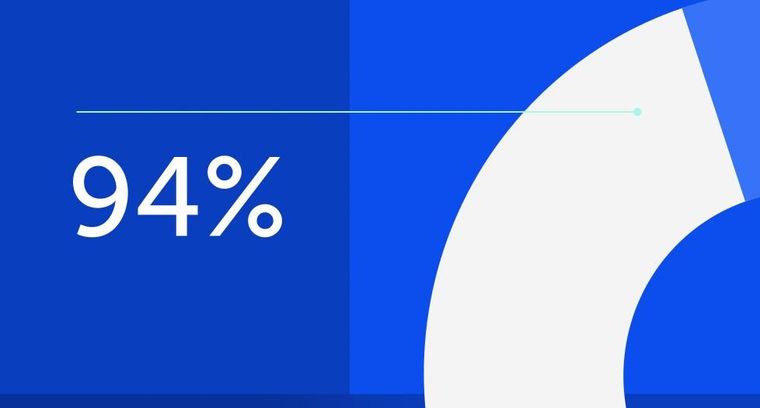
94% of researchers rate our articles as excellent or good
Learn more about the work of our research integrity team to safeguard the quality of each article we publish.
Find out more
SYSTEMATIC REVIEW article
Front. Psychiatry, 24 March 2020
Sec. Public Mental Health
Volume 11 - 2020 | https://doi.org/10.3389/fpsyt.2020.00131
Background: Individuals with mental disorders need continuous and efficient collaboration between different sectors of care. In 2012, a new law in Germany enabled the implementation of novel budgets in psychiatry (flexible and integrated treatment = FIT). Hospitals implementing FIT programs have been evaluated in controlled cohort studies. We present first results based on a meta-analysis from 13 FIT hospitals.
Methods/Design: We undertook a series of claims-data-based controlled cohort studies. Data from over 70 statutory health insurance (SHI) funds in Germany were analyzed. All patients insured by any of the participating SHI funds and treated in one of the FIT hospitals for any of 16 predefined mental disorders were compared with matched control patients from routine care. The patient collective was subdivided into hospital-new and hospital-known patients. Analyses included utilization of inpatient care, day care, outpatient PIA (psychiatrische Institutsambulanz) care, outpatient care with established practitioners, and durations of sick leave. Individual treatment effects of the 13 FIT hospitals were pooled in a random-effects meta-analysis. Meta-regression analysis was used to explore potential reasons for heterogeneity in model effectiveness.
Results: The meta-analysis revealed a significant reduction by over 5 days of inpatient care in hospital-new patients in FIT hospitals compared to control hospitals. This effect was stronger among FIT hospitals with a preexisting FIT-like environment. There was no overall significant effect regarding sick leave between the two groups. Further meta-regression for hospital-new patients revealed a significantly reduced duration of sick leave by almost 13 days for patients in FIT hospitals with a preexisting FIT-like contract compared to FIT hospitals without such a contract.
Conclusions: This study suggests positive effects of FIT programs for patients with mental disorders pointing toward a shorter duration of inpatient treatment. Furthermore, contracts already existent prior to initialization of FIT programs appear to have facilitated the transition into the new treatment environment. For FIT hospitals without such contracts, supposedly there is a certain implementation phase for effects to be apparent. The results should still be interpreted with caution as this manuscript only covers the first year of the 5 year evaluation period in 13 of 18 FIT hospitals.
Clinical Trial Registration: This study was registered in the database “Health Services Research Germany” (trial number: VVfD_EVA64_15_003713).
Mental disorders are predominantly characterized by an early onset in patients' lives and by persisting over long periods of time (1, 2). However, utilization of treatment in psychiatric patients is low with a long delay between the onset of illness and initial treatment (3–5). Efficient patient-centered treatment of these disorders demands for a continuous and close collaboration between different sectors and professions of care (3, 6). As early as in the 1970s, the Study Commission of the German Bundestag urged for new approaches to health care particularly aiming at—whenever possible—a preference of outpatient over inpatient treatment and, in addition, a regionalized health care (7).
However, current standard care for patients with mental disorders in Germany is still characterized by a strong focus on inpatient treatment (8). Furthermore, the German healthcare system currently suffers from insufficient interfaces between different sectors of health care, particularly in the field of psychiatric care (9). This situation results in an inadequate integration of inpatient and outpatient treatment, psychotherapy, and psychosocial services and might even obstruct joint care approaches involving multiple medical specialists (10, 11). Additionally, there is also a great fragmentation of the financing system in German psychiatric health care: budgets for inpatient and day care services are strictly separated from the budget of the psychiatric outpatient departments (PIA, psychiatrische Institutsambulanz). These departments treat patients in need of particularly intensive and complex near-hospital care due to the nature, severity, or longevity of the mental disorder. This financial separation constitutes an additional obstacle for efforts toward an efficient trans-sectoral treatment (12), potentially even resulting in misguided incentives, such as maximizing inpatient occupancies by admitting as many patients as possible with the highest possible retention time (13).
In response, there have been a number of initiatives promoting new financing budgets (from now on called global treatment budgets) aiming to promote patient-centered, cross-sectoral health care for mentally ill patients (14–17). The most recent taking on this issue is based on a German law launched in 2012 [§ 64b, German Social Code V, (18)]. It enabled statutory health insurance (SHI) funds, together with selected hospitals, to jointly establish contracts that represent a hybrid installment of both capitation budget (19–22) and block contract (23–25). According to these contracts, each hospital has an overall fixed annual budget for all patients including inpatient care, day care, and outpatient care. This budget covers all treatment expenses leaving room for the provider to apply an individual treatment strategy. These novel programs will from now on be called FIT programs [FIT = flexible and integrated treatment (26, 27)], and hospitals implementing this type of programs will from now on be referred to as FIT hospitals. FIT hospitals are free to tailor specific models of care that suit the regional peculiarities and meet the needs of community members (28). Thus, they tend to differ tremendously in terms of starting conditions as well as treatment and process structures. All of the FIT programs, however, share the common goal of providing a continuous, flexible, and integrated treatment to patients.
As part of the legal requirement, the authors of this manuscript are in charge of conducting an evaluation of almost all FIT hospitals. This study was, in accordance with requests formulated in prior research (6, 29), designed to provide a standardized evaluation procedure. In this overall evaluation study, called EVA64, patients being treated in 1 of a total of 18 FIT hospitals constitute the intervention group (IG) and are compared to patients from routine care hospitals, which form the control group (CG).
This manuscript describes first results of effectivity measures based on meta-analyses and meta-regressions over the intermediate reports of the 13 FIT hospitals that have been evaluated so far. Analyses included utilization of inpatient care, day care, outpatient PIA care, as well as outpatient care with established practitioners. Furthermore, we analyzed durations of sick leave. Based on the primary goals of FIT programs, we hypothesized a reduction in inpatient care and in the duration of sick leave among patients in FIT hospitals compared to patients from control hospitals.
We undertook a series of controlled cohort studies (1 for each of the 13 FIT hospitals evaluated so far) employing a pre–post design based on health insurance data from each of the intervention hospitals and its matched control hospitals (30). We used anonymized patient data from German statutory SHI funds covering a total time span of 2 years with 1 year prior and 1 year subsequent to inclusion in the evaluation. FIT programs started between January 2013 and December 2014 in all analyzed hospitals. FIT hospitals differed with respect to starting conditions: four hospitals transitioned from standard care to FIT, while the remaining nine hospitals transitioned into the FIT environment with a preexisting contract. Those contracts had goals similar to those of the FIT programs (=FIT-like structures) and could include a regional budget for mental health care, a contract for integrated care, or both options combined (17).
All patients insured by any of the participating SHI funds and treated in one of the FIT hospitals (IG) for any of 16 predefined mental disorders (see Table 1) were compared with control patients from routine care (CG). For each individual hospital population, subcohorts of hospital-known and hospital-new patients were defined. Hospital-new patients had no contact to the psychiatric ward or PIA in the corresponding FIT or control hospital in the 2 years prior to being included. Hospital-known patients had to have at least one such contact during those 2 years. Our reasoning behind the subdivision of the cohort into hospital-new and hospital-known patients was that potential intervention effects would have a different impact for IG/CG patients who already had a treatment history at an FIT/control hospital compared to IG/CG patients whose initial treatment took place after the onset of the FIT intervention. This differentiation gains even more importance considering that 9 out of 13 FIT hospitals already have had specific contracts that to a certain extent exhibited FIT-like structures prior to initiation of the FIT programs. These already preexisting contracts are likely to have facilitated the transition into the new FIT environment and could have already forestalled some of the intended intervention effects before FIT initiation (15, 17, 31, 32). Hence, we expected more unbiased intervention effects to occur in the subcohort of hospital-new patients.
Table 1. Inclusion criteria, diagnoses, International Classification of Diseases, 10th revision (ICD−10).
To minimize the likelihood of selection bias on the provider and patient level, a two-level matching algorithm was applied. On the hospital level, we were primarily concerned with assigning comparable control hospitals to each FIT hospital. Therefore, up to 10 control hospitals were allocated in ranking order to each FIT hospital based on a priori defined knockout criteria (i.e., same region, institutionalized structures, such as specialist departments, and PIA), criteria based on patients (i.e., number of cases per diagnosis) with a weighting of 50%, structural features of hospitals (e.g., number of beds or number of personnel) with a weighting of 25%, and regional factors (e.g., unemployment rate, household income) with a weighting of 25%. More details can be found in the already-published study protocol (33). Once we identified best-fitting control hospitals for each FIT hospital, we applied a second matching algorithm on the level of patients. This was done in order to reduce the impact of possible confounding variables by leveling out IG and CG patient distributions regarding these exact variables. Patients were matched exactly according to the variables: year of study inclusion, hospital-known and hospital-new patient, and type of mental disorder diagnosed at study inclusion. Thus, for these variables, twin pairs of IG and CG patients had to exhibit the exact same value. Furthermore, propensity score matching was applied on variables sex, age at study inclusion, and health care utilization before study inclusion (amount of inpatient care, day care, and outpatient utilization in PIA and established practitioners, all in the area of mental health care). The propensity matching procedure is based on a patient's probability (i.e., propensity score) of group membership (IG/CG), which is calculated by logistic regression for the entire population. Patients' propensity scores were utilized to determine twin pairs of IG and CG members based on a nearest neighbor algorithm (caliper = 0.25 standard deviation, without replacement). Hence, each patient of the IG was assigned its best-fitting twin from the CG based on the least difference in value defined by the propensity score. Note that one CG can contain patients from more than one hospital.
The standardized dataset provided by the participating SHI funds covered inpatient care, day care, and outpatient care (diagnoses and procedures) including PIA and established practitioners, pharmaceutical and non-pharmaceutical treatments (i.e., psychotherapy), and information on sick leave. As all analyzed data were anonymous, the ethical committee of the University of Magdeburg confirmed that no ethical approval was necessary. Data were handled, analyzed, and reported according to Good Epidemiological Practice (GEP) (34), Good Practice of Secondary Data Analysis (GPS) (35), and the German Reporting Standard for Secondary Data Analyses, Version 2 (STROSA 2) (36).
Outcome parameters between IG and CG were compared with respect to the patient–individual pre-time, which was 1 year prior to study entrance. We report first results from inception until the end of the first year of intervention in 13 out of the total 18 FIT hospitals. Primary outcomes were duration of inpatient care and sick leave. The first outcome describes the average cumulated length of hospitalization days. For the parameter sick leave, we aggregated the average cumulated number of days in sick leave. The inclusion diagnosis, in this case, was based on inpatient and outpatient sick leave prescriptions. In addition, every day a patient spent in inpatient care was counted as sick leave day. The analysis was restricted to patients with “member” status as reported by the corresponding SHI. This was done in order to exclude retirees and people who were not capable of working, who have a different status of health insurance. Secondary outcomes included the cumulated duration of day care (number of days) as well as the cumulated number of contacts in outpatient care. We split outpatient care into (i) contacts to the PIA and (ii) contacts to established medical specialists for psychiatry or psychotherapy. We assumed that a potentially reduced inpatient care in the initial treatment phase could be compensated for through either day care or outpatient treatment. To this end, we analyzed day care and outpatient utilization (for each PIA and established practitioner) in addition to the duration of inpatient care. To further analyze the association of duration of inpatient and duration of day care treatment for the cohort of hospital-new patients, we calculated a Pearson correlation coefficient on the level of hospitals between these two measures.
Estimators for outcomes of interest were computed using generalized linear Poisson models. Models contained variables for factors group (IG vs. CG) and time (pre vs. 1st year after inclusion into the study) as well as an additional variable for the interaction term group x time. As the interaction term approximates the difference-in-difference (DiD) coefficient, and hence the actual effect of treatment, regression coefficients for that interaction term were later used in the meta-analysis. The DiD coefficient compares the average change over time in the outcome variable for the IG in contrast to the average change over time for the CG. Thus, greater changes over time in the IG compared to the CG are associated with a positive DiD coefficient and vice versa.
The DiD coefficients for every single FIT hospital were used in a meta-analysis regarding the primary outcomes duration of inpatient care as well as duration of sick leave, as well as the secondary outcomes duration of day care and amount of outpatient contacts for each PIA and established practitioner, respectively. The meta-analysis was done utilizing the R package metaphor (37). The heterogeneity measure I2 between the individual entities was expected to be high since hospitals recruited different populations and had varying starting conditions. Taking these considerations into account, the pooled estimator was modeled as random effect. The heterogeneity parameter τ2 was estimated using the DerSimonian–Laird estimator. Due to insufficient pre-period data of outpatient contacts in the PIA in 6 of the 13 FIT hospitals, this meta-analysis only compared mean differences in the first year after onset of the project. Regarding contacts to medical specialists, we were able to apply the usual procedure of calculating a pooled effect over individual DiD estimates. In addition, we carried out a meta-regression for selected outcomes to investigate the impact of preexisting FIT–like structures. Therefore, we included a predictor variable into the meta-regression that dummy-coded hospitals with a preexisting contract (0 = no existing contract vs. 1 = existing contract). The result of the meta-regression would give an estimate on how FIT hospitals with preexisting contracts would contrast against those without such a contract. All statistical analyses were done using statistical software R V. 3.3.2 (38).
The number of patients per group ranged between 153 and 852 in hospital-new, and 164 and 1,207 in hospital-known patients in different hospitals. The overall cohort consisted of 26,398 patients (13,199 each in IG and CG) with 12,468 (6,234 each) being hospital-new and 13,930 (6,965 each) being hospital-known. Mean age and sex were highly comparable between IG and CG (see Table 2). Throughout all hospitals, regardless of group, more women were included (56%).
Among hospital-new patients, the number of days spent in hospital was low before inclusion and sharply increased within the first year after inclusion (see Table 3). However, across all FIT hospitals, the sharp increase in hospital stay was lower in the IG (from 2 to 15.6 days) compared to the CG (from 2.2 to 21.3 days).
Hospital-known patients had fewer inpatient days in the IG compared to the CG. The increase in inpatient care days was slightly lower in the IG (10.9 to 12.7 days) compared to the CG (from 13.9 to 16.4 days; Table 3).
The amount of days of sick leave sharply increased within the first year after inclusion (Table 4). There was no notable overall difference in the increase of days of sick leave between the two groups (see Table 4).
The aggregated average number of days of sick leave was lower in the IG compared to the CG in the first year of the evaluation. Overall, there was a slight increase in the duration of sick leave in both groups with the increase in the IG being slightly lower compared to the CG.
In the cohort of hospital-new patients, the pooled effect of the overall difference in cumulative number of inpatient care days per person between IG and CG exceeded the significance level (p < 0.001). The pooled DiD showed an average decrease of 5.4 days (95% CI: −7.41; −3.44) in the IG compared to the CG (see Table 5 and Figure 1). The meta-regression unfolded that this effect was especially driven by FIT hospitals that already had a preexisting contract. When contrasted against the remaining four FIT hospitals without such a contract, hospitals with a preexisting contract had a significantly reduced DiD of ~4.7 days in the meta-regression (see Table 6).
Figure 1. Forest plot depicting effects of cumulated duration of inpatient care in hospital-new patients.
Table 6. Meta-regression effect sizes of FIT-like pre-contracts (existing vs. nonexisting) in different outcomes.
In the cohort of hospital-known patients, the pooled estimate displayed a decrease of 0.77 days (95% CI: −2.5; 0.97) in the IG compared to the CG (see Table 5 and Figure 2). However, this effect did not exceed the significance level. The following meta-regression revealed no significant difference in FIT hospitals that already had a preexisting contract compared to hospitals without a contract (see Table 6). In general, coefficients of individual DiD effects in the cohort of hospital-known patients revealed smaller absolute values compared to the cohort of hospital-new patients, especially in FIT hospitals with preexisting FIT-like contracts. This is mainly due to already-existent baseline differences in the pre-period between IG and CG. Those baseline differences might lead to possible ceiling effects by leaving not much room for further improvement in the post-period. This pre-period difference between the two groups was not that pronounced in FIT hospitals without already-existing FIT-like structure (see Table 3).
Figure 2. Forest plot depicting effects of cumulated duration of inpatient care in hospital-known patients.
Analysis for outpatient contacts in PIA or with an established practitioner did not yield statistically significant results in both hospital-new and hospital-known patients. Neither the number of contacts in the PIA nor the number of contacts to medical specialists were considerably increased in intervention patients compared to patients of the CG (see Table 5).
In the meta-analysis of cumulated day care duration for hospital-new patients, the pooled estimate did not exceed the significance level as well (p = 0.15, see Table 5). However, the IG showed a trend toward a higher utilization (~2 days) compared to the CG (see Figure 3).
We identified a negative correlation coefficient of −0.31 between the utilization of inpatient care and day care. The more inpatient treatment days were reduced in the IG of a specific FIT hospital, the more the number of days in day care treatment was increased compared to the CG and vice versa (see Figure 4). This correlation did not yet exceed the significance level (p = 0.15), which is mainly due to the lack of statistical power as only data pairs from 13 FIT hospitals were available up to that point. In hospital-known patients, there was no significant difference in day care utilization between IG and CG.
Figure 4. Regression line depicting the association between duration of inpatient treatment and duration of day care treatment in hospital-new patients (n.s.) 1 DiD, difference-in-difference.
In the cohort of hospital-new patients, there was no significant pooled effect in the overall difference in cumulative number of sick leave days per person between IG and CG (pooled estimate = −0.02; p = 0.994, see Table 5 and Figure 5). There is a significant individual effect in almost every FIT hospital contrasted against its respective CG. However, the direction of these effects is quite diverse. When contrasting hospitals who already had a preexisting FIT-like contract against the remaining hospitals without such a contract as reference in the meta-regression, we found a massive effect of an increased negative DiD (see Table 6). Hence, whether or not an FIT hospital already had a preexisting FIT-like structure plays a huge role in explaining the large heterogeneity of the individual DiD effects. Hospitals with a preexisting contract had a reduced duration of sick leave of almost 13 days compared to hospitals without such a contract. Based on this result, we additionally performed a meta-analysis only including the nine FIT hospitals with a preexisting contract. The pooled estimate did not also exceed the significance level, but, however, showed a stronger tendency toward a reduced duration of sick leave of over 4 days in IG patients compared to CG patients (pooled estimate = −4.34; p = 0.076).
Figure 5. Forest plot depicting effects of cumulated duration of sick leave in hospital-new patients.
In the cohort of hospital-known patients, the pooled estimate displayed a decrease in days of sick leave of 1.27 days in the IG compared to the CG (see Table 5 and Figure 6). This effect did not exceed the significance level. The meta-regression revealed no significant difference in FIT hospitals that already had a preexisting contract compared to hospitals without a contract (see Table 6).
Figure 6. Forest plot depicting effects of cumulated duration of sick leave in hospital-known patients.
The EVA64-study evaluates 18 different nationwide FIT hospitals, which aim to improve the health care of patients with mental disorders in Germany on the basis of novel global treatment budgets. This manuscript presents results of the EVA64-study based on a meta-analysis over the first intermediate evaluative reports of 13 of these FIT projects.
The meta-analysis showed a significantly lower increase in the cumulated duration of inpatient care in hospital-new intervention patients (IG) compared to control patients (CG) indicating positive effects on one of the primary goals of the FIT intervention (30). This effect was even stronger when only considering FIT hospitals that transitioned from an already-existing FIT-like contract into the new environment. The overall sharp increase in inpatient care from pre-period to the end of the first year in both groups was expected, as the hospital-new patient's inclusion diagnosis is likely to be an incident diagnosis resulting in a high initial demand of care. In hospital-known patients, there was already a substantial baseline difference between IG and CG in those FIT hospitals. This was not the case when only considering FIT hospitals without any preexisting contract in the pre-period. These results may lead to various conclusions with high impact for the organization of clinical care in mental health settings. First, the FIT intervention is effective in terms of reducing fully inpatient care. Secondly, preexisting FIT-like contracts in the pre-period may have already forestalled some of the intended effects. This presumption is supported by findings from other authors who explicitly examined the effects of some of these contracts in various hospitals (16, 17, 39). On the other hand, pre-contracts possibly facilitated a faster and smoother implementation of the new FIT environment. Intervention hospitals starting from scratch are likely to undergo a longer transition and implementation period. Thus, potential FIT effects are not likely to be already present in the first year after onset in those clinics. Interestingly, we found tendencies of overall increased day care duration but not an increase in outpatient contacts in hospital-new patients. Moreover, there seems to be a correlation between the reduction of inpatient care and day care. The more inpatient care is reduced in an FIT hospital, day care treatment increases. This may give rise to the interpretation that reduced inpatient days in FIT hospitals are rerouted into day care treatment but not necessarily into outpatient treatment per se.
Analogously to inpatient treatment, there was a sharp increase in sick leave duration from pre-period to the end of the first year in both groups. As hospital-new patients' inclusion diagnosis is likely to be an incident diagnosis, this may lead to an increased number of sick leave days. On first sight, there seems to be no considerable difference regarding trends in sick leave duration between FIT patients and control patients. The pooled estimator showed no significant difference in the average cumulated number of sick leave days between IG and CG in both hospital-new and hospital-known patients. With the individual effects being very heterogeneous, a further meta-regression for hospital-new patients revealed that sick leave duration was significantly lower by almost 13 days in FIT hospitals with an already preexisting FIT-like contract in the pre-period compared to FIT hospitals without such a contract. A concluding meta-analysis containing only FIT hospitals with an FIT-like contract in the pre-period showed a clear tendency of reduced sick leave duration in intervention patients compared to control patients, albeit without being significant.
The study presented gathers data from FIT hospitals from locations all over Germany in total representing more than 300,000 patients (40). Yet, follow-up of the results described is still limited to only the first year of the all in all five-year evaluation period. Thus, this manuscript can only cover potential short-term effects, leaving out effects that occur only over a longer time period. Further reports with data spanning over a longer observation period will reveal whether positive intervention effects will be present or strengthened in FIT hospitals, particularly in those without any preexisting contract. So far, only 13 of a total out of 18 FIT hospitals have been analyzed. For a final assessment, data covering all FIT hospitals over the entire evaluation period have to be evaluated. The scientific use of claims data from SHI funds for the evaluation of new health care concepts has been established during the last years including analysis and reporting standards (35, 36). However, validity of information on diagnoses in claims data can be a potential issue, especially regarding outpatient data (41). However, please note that due to the CG design of the study, we expect potential biases of diagnosis quality to affect each, IG and CG to equal amounts. While claims data offer essential information and have the great advantage of being less prone to selection bias (42), they do not contain preference-based and patient-centered information such as symptom severity or functional level measures. Hence, it is important to stress that this study cannot evaluate treatment success in FIT programs per se.
In order to close this gap and gain such information, the complementary evaluation project PsychCare has been established. This project is conducted in 10 FIT hospitals and consecutive controls and will give access to patient-reported outcomes and patient-reported experience measures by means of questionnaires and qualitative surveys. First results by another research group implementing a mixed methods design suggest that changes caused in FIT hospitals are rated mostly positive by patients, while relatives stated to fear a certain extra effort caused by increased outpatient treatment. Employees in FIT hospitals, especially in nursing professions, described to have a higher work load in response to the initiation of the FIT program (43).
The indirect aim of FIT programs is to develop a system where the treatment can be adjusted flexibly to the patient in contrast to adjusting the patient to the treatment. In accordance with our hypothesis, the meta-analyses presented here suggest positive effects for models of care based on global treatment budgets for patients with mental disorders. Results point toward a shorter duration of inpatient treatment and a trend toward a reduced duration of sick leave days in FIT hospitals with a preexisting FIT–like structure in the pre-period. Moreover, the results suggest that (a) preexisting contracts are likely to have facilitated the transition into the new FIT environment and (b) implementation of FIT programs in hospitals without such a pre-contract demands for a certain transition period until positive effects will be visible. Further reports will answer the question if positive effects of intervention observed so far will persist on a long-term perspective. If FIT hospitals in psychiatric care continue to be efficient compared to routine care, this evaluation will provide arguments for a new structuring of routine care for patients with mental disorders in Germany.
The data supporting the findings of the study is provided by more than 70 participating SHI funds. These data are not publicly available and were used under license for the current study.
The ethical committee of the University of Magdeburg has been notified. The ethical committee stated in April 2016 that no vote is necessary, as the EVA64-study is an analysis of anonymous data.
FB wrote the manuscript, conceptualized, and conducted the statistical analyses. OS conceptualized the statistical analyses. AN coordinated the study and defined the outcome parameters. MS contributed substantially to data handling, selection of control hospitals, and evaluation of outcome parameters. RK contributed substantially to the economic outcomes and patient–individual matching. SM contributed substantially to the data management. ES was responsible for data management. DH was responsible for economic outcomes. AP advised substantially in regard to psychiatric care and outcome parameters. JS was principal investigator and responsible for the description of the study design and effectivity estimation. All authors have read and commented on the manuscript and approved the final version of the manuscript.
This study was funded by a cooperation of the following statutory health insurance funds [as of May 2019, later mergers or name changes are not considered; the current list of all SHI funds can be found elsewhere (44)]. The funding bodies collected and provided the raw claims data, which were later processed and aggregated by the authors. The funding bodies did not exert influence on the design, the analysis, and the interpretation of the data, nor were they involved in the writing process of the manuscript. actimonda krankenkasse; AOK Nordost—Die Gesundheitskasse für Berlin, Brandenburg und Mecklenburg-Vorpommen; AOK Rheinland/Hamburg—Die Gesundheitskasse; AOK PLUS—Die Gesundheitskasse für Sachsen und Thüringen; AOK NORDWEST—Die Gesundheitskasse; AOK—Die Gesundheitskasse in Hessen; AOK—Die Gesundheitskasse für Niedersachsen; AOK Bayern—Die Gesundheitskasse, Zentrale; AOK Sachsen-Anhalt—Die Gesundheitskasse; AOK Bremen/Bremerhaven—Die Gesundheitskasse; AOK Rheinland-Pfalz/Saarland—Die Gesundheitskasse; AOK Baden-Württemberg; Audi BKK; BAHN-BKK; BARMER (merged with Deutsche BKK as of 01.01.2017); Bertelsmann BKK; Betriebskrankenkasse der BMW AG (BMW BKK); Betriebskrankenkasse der MTU Friedrichshafen GmbH (BKK MTU); Betriebskrankenkasse Herford Minden Ravensburg (BKK HMR); Betriebskrankenkasse Mobil Oil; BKK Achenbach Buschhütten; BKK advita; BKK Aesculap; BKK Akzo Nobel; BKK B. Braun Melsungen AG; BKK BPW Bergische Achsen KG; BKK der Maschinenfabrik und Eisengießerei Meuselwitz (BKK MEM); BKK Deutsche Bank AG; BKK_DürkoppAdler; BKK evm; BKK EWE; BKK exklusiv; BKK Faber-Castell & Partner; BKK firmus; BKK GILDEMEISTER SEIDENSTICKER; BKK Groz-Beckert; BKK Herkules; BKK KRONES; BKK Linde (merged with HEAG BKK as of 01.01.2016); BKK MAHLE; BKK Melitta Plus; BKK Pfaff; BKK Pfalz (merged with BKK Vital as of 01.01.2018); BKK ProVita (merged with BKK family as of 01.01.2016); BKK PwC; BKK Rieker Ricosta Weisser; BKK Scheufelen; BKK Schwarzwald-Baar-Heuberg (BKK SBH); BKK Stadt Augsburg; BKK Technoform; BKK VDN; BKK Verkehrsbau Union (BKK VBU) (merged with Vereinigten BKK as of 01.01.2017 and with BKK DEMAG KRAUSS-MAFFEI, BKK Schleswig-Holstein, BKK Basell as of 01.01.2016) BKK VerbundPlus; BKK Voralb HELLER LEUZE TRAUB; BKK Werra-Meissner; BKK Wirtschaft und Finanzen; BKK Würth; BKK ZF & Partner; Bosch BKK; Brandenburgische BKK; Continentale Betriebskrankenkasse; DAK-Gesundheit (merged with BKK Beiersdorf as of 01.07.2016); Daimler Betriebskrankenkasse; Debeka Betriebskrankenkasse; Deutsche Rentenversicherung Knappschaft-Bahn-See; DIE BERGISCHE KRANKENKASSE; Die Schwenninger Krankenkasse; energie-BKK (merged with E.ON BKK as of 01.01.2017) Ernst & Young BKK; HEK – Hanseatische Krankenkasse; hkk; IKK Südwest; IKK classic; Kaufmännische Krankenkasse – KKH; Merck BKK; mhplus Betriebskrankenkasse (merged with Metzinger BKK as of 01.01.2019); Novitas BKK; pronova BKK (merged with BKK Braun-Gillette as of 01.01.2017); R+V BKK; SBK Siemens-Betriebskrankenkasse; SIEMAG BKK; SKD BKK; Sozialversicherung für Landwirtschaft, Forsten und Gartenbau; Südzucker BKK; Techniker Krankenkasse (TK); Thüringer Betriebskrankenkasse (TBK); Vaillant BKK; Wieland BKK; WMF Betriebskrankenkasse. The Technische Universität Dresden, the University of Magdeburg, and WIG2 provided funding for this study. This funding did not have any influence on the design, methods, and evaluation of the study.
The authors declare that the research was conducted in the absence of any commercial or financial relationships that could be construed as a potential conflict of interest.
The EVA64 study group acknowledges the contribution of Katrin Arnold, Franziska Claus, Nils Kossack, Denise Küster, Thomas Petzold, Ines Weinhold, and Jessika Weiß to the conception of the study. Furthermore, we thank Veronika Bierbaum for critically counterchecking the manuscript for spelling and grammar as well as providing us with lots of helpful comments. Publication of this manuscript was funded by the Publication Fund of the TU Dresden.
CG, control group; DiD, difference in difference; ICD-10, International Classification of Diseases, 10th revision; IG, intervention group; PIA, psychiatric outpatient department; SGB, Social Security Code (Sozialgesetzbuch); SHI, statutory health insurance.
1. Karow A, Bock T, Naber D, Lowe B, Schulte-Markwort M, Schafer I, et al. [Mental health of children, adolescents and young adults–part 2: burden of illness, deficits of the German health care system and efficacy and effectiveness of early intervention services]. FortschrNeurolPsychiatr. (2013) 81:628–38. doi: 10.1055/s-0033-1355840
2. Lambert M, Bock T, Naber D, Lowe B, Schulte-Markwort M, Schafer I, et al. [Mental health of children, adolescents and young adults–part 1: prevalence, illness persistence, adversities, service use, treatment delay and consequences]. FortschrNeurolPsychiatr. (2013) 81:614–27. doi: 10.1055/s-0033-1355843
3. Brieger P, Bode L, Urban R, Pfennig A. [Psychiatric care for subjects with bipolar disorder: results of the new German S3 guidelines]. Nervenarzt. (2012) 83:595–603. doi: 10.1007/s00115-011-3417-1
4. DGBS and DGPPN. S3-Leitlinie zur Diagnostik und Therapie Bipolarer Störungen. Berlin: Springer Verlag (2012).
5. Pfennig A, Bschor T, Falkai P, Bauer M. The diagnosis and treatment of bipolar disorder: recommendations from the current s3 guideline. DtschArzteblInt. (2013) 110:92–100. doi: 10.3238/arztebl,.2013.0092
6. Wilms B, Becker T, Lambert M, Deister A. Modelle für eine zukunftsfähige psychiatrische Versorgung. Psychiatrie. (2012) 9:4–13. doi: 10.1055/s-0038-1671750034
7. Kunze H. Personenzentrierter Ansatz in der psychiatrischen Versorgung in Deutschland. Psycho. (1999) 25:728–35.
8. Salize HJ, Kilian R. Gesundheitsökonomie in der Psychiatrie - Konzepte, Methoden, Analysen. Stuttgart: Verlag W. Kohlhammer (2010).
9. Schmitt J, Petzold T, Nellessen-Martens G, Pfaff H. [Prioritization and consentation of criteria for the appraisal, funding and evaluation of projects from the german innovationsfonds: a multi-perspective delphi study]. Gesundheitswesen. (2015) 77:570–9. doi: 10.1055/s-0035-1555898
11. Schneider F, Falkai P, Maier W. Psychiatrie 2020 Plus. Perspektiven, Chancen und Herausforderungen. Berlin, Heidelberg: Springer (2011).
12. Deister A. Erlösqualität optimieren. Führen und Wirtschaften im Krankenhaus. Melsungen: Bibliomed-Verlag (2015) 32:248–51.
13. Becker T, Hoffmann H, Puschner B, Weinmann S, Gaebel W, Müller-Spahn F, et al. Versorgungsmodelle in der Psychiatrie und Psychotherapie. Stuttgart: Kohlhammer Verlag (2008).
14. Deister A, Zeichner D, Witt T, Forster HJ. [Changes in mental health care by a regional budget: results of a pilot Project in Schleswig-Holstein (Germany)]. Psychiatr Prax. (2010) 37:335–42. doi: 10.1055/s-0030-1248438
15. Konig HH, Heinrich S, Heider D, Deister A, Zeichner D, Birker T, et al. [The regional psychiatry budget (RPB): a model for a new payment system of hospital based mental health care services]. Psychiatr Prax. (2010) 37:34–42. doi: 10.1055/s-0029-1223418
16. Roick C, Deister A, Zeichner D, Birker T, Konig HH, Angermeyer MC. [The regional budget for mental health care: a new approach to combine inpatient and outpatient care]. Psychiatr Prax. (2005) 32:177–84. doi: 10.1055/s-2004-834736
17. Schmid P, Steinert T, Borbé R. Systematische Literaturübersicht zur Implementierung der sektorübergreifenden Versorgung (Regionalbudget, integrierte Versorgung) in Deutschland. Psychiatrische Praxis. (2013) 40:414–24. doi: 10.1055/s-0033-1343192
18. Sozialgesetzbuch (SGB) Fünftes Buch (V) - Gesetzliche Krankenversicherung - (Artikel 1 des Gesetztes v. 20. Dezebmer 1988, BGBI. S. 2477), zuletzt geändert durch Art. 7 G v. 23.5.2017 | 1228 (1998).
19. Bloom JR, Wang H, Kang SH, Wallace NT, Hyun JK, Hu TW. Capitation of public mental health services in Colorado: a five-year follow-up of system-level effects. Psychiatr Serv. (2011) 62:179–85. doi: 10.1176/ps.62.2.pss6202_0179
20. Freeman DS, Manson L, Howard J, Hornberger J. Financing the primary care behavioral health model. J Clin Psychol Med Settings. (2018) 25:197–209. doi: 10.1007/s10880-017-9529-4
21. Jacobs R, Chalkley M, Aragon MJ, Bohnke JR, Clark M, Moran V. Funding approaches for mental health services: is there still a role for clustering? BJPsych Adv. (2018) 24:412–21. doi: 10.1192/bja.2018.34
22. Konig HH, Heider D, Rechlin T, Hoffmann P, Birker T, Heinrich S, et al. [How does the Regional Psychiatry Budget (RPB) work in an area with initially low capacity of psychiatric hospital beds?]. Psychiatr Prax. (2013) 40:430–8. doi: 10.1055/s-0033-1343186
23. Estes CL, Wood JB. A preliminary assessment of the impact of block grants on community mental health centers. Hosp Community Psychiatry. (1984) 35:1125–9.
24. Hargrove DS, Melton GB. Block grants and rural mental health services. J Rural Commun Psychol. (1987). 8:4–11.
25. Sharfstein SS. Medicaid cutbacks and block grants - crisis or opportunity for community mental-health. Am J Psychiatry. (1982) 139:466–70. doi: 10.1176/ajp.139.4.466
26. Johne J, von Peter S, Schwarz J, Timm J, Heinze M, Ignatyev Y. Evaluation of new flexible and integrative psychiatric treatment models in Germany-assessment and preliminary validation of specific program components. BMC Psychiatry. (2018) 18:278. doi: 10.1186/s12888-018-1861-1
27. von Peter S, Ignatyev Y, Johne J, Indefrey S, Kankaya OA, Rehr B, et al. Evaluation of flexible and integrative psychiatric treatment models in germany—a mixed-method patient and staff-oriented exploratory study. Front Psychiatry. (2019) 9:785. doi: 10.3389/fpsyt.2018.00785
28. Berghofer A, Hubmann S, Birker T, Hejnal T, Fischer F. Evaluation of quality indicators of integrated care in a regional psychiatry budget - a pre-post comparison by secondary data analysis. Int J Integr Care. (2016) 16:17. doi: 10.5334/ijic.2479
29. Nolting HD, Hackmann T. Bestandsaufnahme von komplexen lokalen, regionalen und überregionalen sektorübergreifenden Modellprojekten zur Versorgung von Menschen mit psychischen Erkrankungen - Abschlussberichtv. Berlin: IGES Institut GmbH (2012).
30. Neumann A, Swart E, Hackl D, Kliemt R, March S, Kuster D, et al. The influence of cross-sectoral treatment models on patients with mental disorders in Germany: study protocol of a nationwide long-term evaluation study (EVA64). BMC Psychiatry. (2018) 18:139. doi: 10.1186/s12888-018-1721-z
31. Coleman M, Schnapp W, Hurwitz D, Hedberg S, Cabral L, Laszlo A, et al. Overview of publicly funded managed behavioral health care. Adm Policy Ment Health. (2005) 32:321–40. doi: 10.1007/s10488-004-1662-3
32. Roick C, Heinrich S, Deister A. Das Regionale Psychiatriebudget: kosten und Effekte eines neuen sektorübergreifenden Finanzierungsmodells für die psychiatrische Versorgung. Psychiatr Prax. (2008) 35:285. doi: 10.1055/s-2008-1067432
33. Petzold T, Neumann A, Seifert M, Kuster D, Pfennig A, Weiss J, et al. [Identification of control hospitals for the implementation of the nationwide and standardized evaluation of model projects according to section sign 64b SGB V: analysis of data from structured quality reports]. Gesundheitswesen. (2019) 81:63–71. doi: 10.1055/s-0042-116436
34. Hoffmann W, Latza U, Baumeister SE, Brunger M, Buttmann-Schweiger N, Hardt J, et al. Guidelines and recommendations for ensuring Good Epidemiological Practice (GEP): a guideline developed by the German Society for Epidemiology. Eur J Epidemiol. (2019) 34:301–17. doi: 10.1007/s10654-019-00500-x
35. Swart E, Gothe H, Geyer S, Jaunzeme J, Maier B, Grobe T, et al. Gute Praxis Sekundärdatenanalyse (GPS): Leitlinien und Empfehlungen [Goode practice secondary data analysis: guidelines and recommendations]. Gesundheitswesen. (2015) 77:120–6. doi: 10.1055/s-0034-1396815
36. Swart E, Bitzer EM, Gothe H, Harling M, Hoffmann F, Horenkamp-Sonntag D, et al. A consensus german reporting standard for secondary data analyses, version 2 (STROSA-STandardisierte BerichtsROutine fur SekundardatenAnalysen). Gesundheitswesen. (2016) 78:e145–60. doi: 10.1055/s-0042-108647
37. Viechtbauer W. Conducting meta-analyses in R with the metafor package. J Stat Softw. (2010) 36:1–48. doi: 10.18637/jss.v036.i03
38. R Core Team. R: A Language and Environment for Statistical Computing. Vienna: R Foundation for Statistical Computing (2019). Available online at: https://www.R-project.org/
39. Sander K, Albus M. Innovative Projekte im Gefolge der Gesundheitsmodernisierungsgesetze: Erfahrungen mit einem Projekt der integrierten Versorgung in der Psychiatrie. Psychiatr Forsch. (2010) 1:92–5. doi: 10.5283/pf.32
40. March S, Zimmermann L, Kubat D, Neumann A, Schmitt J, Baum F, et al. Methodische Herausforderungen bei der Nutzung von Daten von mehr als 70 gesetzlichen Krankenkassen - Ein Werkstattbericht aus der EVA64-Studie. Gesundheitswesen. (2020) 82(Suppl 1):S4–12. doi: 10.1055/a-1036-6364
41. Erler A, Beyer M, Muth C, Gerlach FM, Brennecke R. [Garbage in - garbage out? Validity of coded diagnoses from GP claims records]. Gesundheitswesen. (2009) 71:823–31. doi: 10.1055/s-0029-1214399
42. Swart E. Claims data for evaluation. In: Amelung E, Stein V, Goodwin N, Balicer R, Nolte E, Suter E, editors. Handbook Integrated Care. New York, NY: Springer. (2017). p. 333–349.
43. von Peter S, Ignatyev Y, Heinze M. Evaluation der Modellprojekte nach § 64b SGB V - ein Mixed Method Ansatz. Paper presented at the Deutscher Kongress Versorgungsforschung. Berlin (2017).
44. GKV Spitzenverband. Krankenkassenliste Deutschland (2020). Retrieved from: http://www.gkv-spitzenverband.de/service/versicherten_service/krankenkassenliste/krankenkassen.jsp
Keywords: claims data, psychiatric health care, effectiveness, statutory health insurance, inpatient and outpatient treatment, setting approach, health care system, health services research
Citation: Baum F, Schoffer O, Neumann A, Seifert M, Kliemt R, March S, Swart E, Häckl D, Pfennig A and Schmitt J (2020) Effectiveness of Global Treatment Budgets for Patients With Mental Disorders—Claims Data Based Meta-Analysis of 13 Controlled Studies From Germany. Front. Psychiatry 11:131. doi: 10.3389/fpsyt.2020.00131
Received: 27 September 2019; Accepted: 13 February 2020;
Published: 24 March 2020.
Edited by:
Tilman Steinert, ZfP Südwürttemberg, GermanyReviewed by:
Anke Bramesfeld, Hannover Medical School, GermanyCopyright © 2020 Baum, Schoffer, Neumann, Seifert, Kliemt, March, Swart, Häckl, Pfennig and Schmitt. This is an open-access article distributed under the terms of the Creative Commons Attribution License (CC BY). The use, distribution or reproduction in other forums is permitted, provided the original author(s) and the copyright owner(s) are credited and that the original publication in this journal is cited, in accordance with accepted academic practice. No use, distribution or reproduction is permitted which does not comply with these terms.
*Correspondence: Fabian Baum, RmFiaWFuLkJhdW1AdW5pa2xpbmlrdW0tZHJlc2Rlbi5kZQ==
Disclaimer: All claims expressed in this article are solely those of the authors and do not necessarily represent those of their affiliated organizations, or those of the publisher, the editors and the reviewers. Any product that may be evaluated in this article or claim that may be made by its manufacturer is not guaranteed or endorsed by the publisher.
Research integrity at Frontiers
Learn more about the work of our research integrity team to safeguard the quality of each article we publish.