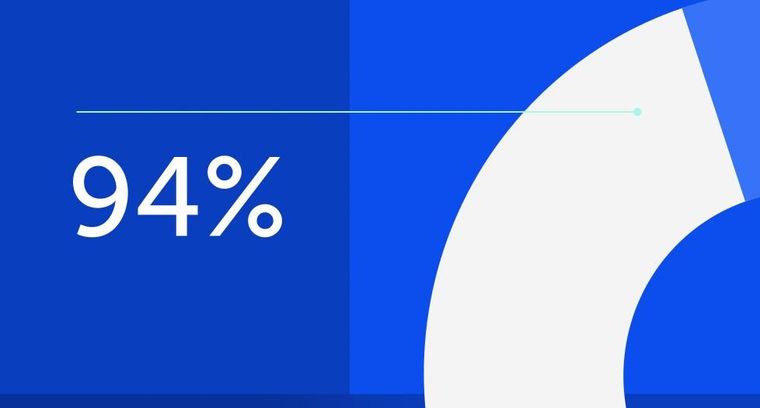
94% of researchers rate our articles as excellent or good
Learn more about the work of our research integrity team to safeguard the quality of each article we publish.
Find out more
SYSTEMATIC REVIEW article
Front. Psychiatry, 09 January 2020
Sec. Addictive Disorders
Volume 10 - 2019 | https://doi.org/10.3389/fpsyt.2019.00871
Background: In patients with alcohol use disorder, novel interventions to increase abstinence have attracted growing attention. Interventions aimed at modifying cognitive biases linked to alcohol use [i.e. cognitive bias modification (CBM)] may serve as an add-on to standard therapy. This systematic review thoroughly aggregates existing data on the effects of three alcohol-specific computerized interventions, namely attentional bias modification (AtBM), approach bias modification (ApBM), and inhibition training (IT). In doing so, each CBM’s effects on experimental tasks assessing the relevant biases, drinking behavior, and neurophysiology are summarized. Also, the influence of drinking behavior severity and motivation to change drinking behavior are discussed.
Methods: A literature search was conducted in four databases for original research articles published between 2000 and May 2019. Studies were eligible if investigating the effects of alcohol-specific computerized interventions (AtBM, ApBM, IT) on drinking behavior, bias change, and/or neurophysiology. Forty eligible articles were classified as being either a non-clinical experimental lab study (ELS) or clinical randomized-controlled trial (RCT) and summarized.
Results: While AtBM seems to influence attentional bias, its effects on drinking behavior are inconsistent. As for ApBM, the best effects on drinking behavior are obtained in clinical samples. Effects of ApBM on approach bias are mixed. Interestingly, those clinical RCTs which investigated ApBM effects on bias change as well as on drinking outcome, reported consistent effects in both measures (i.e. either effects on bias and drinking or no effects). Studies on IT are limited to non-clinical samples and show inconsistent effects on drinking behavior. Considering ITs effects on implicit semantic associations, most studies do not support the conceptualization of IT as a form of memory bias modification, while reports on IT’s effects on inhibitory control are still incomplete. Conclusions about the overall influence of drinking behavior severity are hampered by the non-uniform use of sample descriptions.
Conclusions: In clinical samples, ApBM has shown more consistent beneficial effects, while evidence on AtBM is more inconsistent, and data on IT still lacks important information. Conclusions about the influence of drinking behavior severity would be facilitated by a uniform use of clearly defined sample descriptions.
Alcohol use disorder (AUD) is a chronic health problem with serious biological, psychological, and social consequences (1). Despite sophisticated psychological and pharmacological treatment, patients frequently relapse (2), requiring the search for novel interventions to improve treatment. While cognitive behavioral treatments predominantly target conscious information processing, accumulating evidence for the importance of subconscious mechanisms is reflected less in treatment programs (3).
Certain alterations in the cognitive processing of alcohol-specific cues have been identified as being relevant in AUD. While the automatized tendency to process, memorize, and approach alcohol-related cues (4, 5) is pronounced in AUD patients, inhibitory processes to withhold from drinking alcohol are deficient (6). This behavioral research on cognitive processes in AUD is complemented by recent neuroscientific evidence for marked changes in brain activation while processing alcohol-related cues. Such changes are thought to play a pivotal role in the development and maintenance of AUD (7, 8). The automatic activation of both saliency and motivation-related networks is sensitized to alcohol-related cues, activation in brain areas linked to memory, interoception, and stress reactivity are altered, and the ability of brain regions involved in executive control to effectively exert that control function is impaired (9). Thus, both behavioral and neuroscientific evidence has highlighted the imbalance between automatized processes pushing patients toward alcohol-related cues and opposing control processes. Three specific substance-related maladaptive cognitive processes and the manipulation thereof have increasingly become the focus of research, namely biased selective attention (10) and approach tendencies (11) toward alcohol-related cues, as well as impaired inhibitory control (6).
The first of these processes, attentional bias (AtB), describes that alcohol-specific cues rapidly attract and subsequently hold selective attention (12). The extent of alcohol-specific AtB has been shown to be proportional both to alcohol consumption (13) and to predict future relapse rates in AUD (12). The second concept, approach bias (ApB) toward alcohol-specific stimuli, describes an automatic action tendency that biases a person to approach alcohol-related stimuli rather than avoid them. ApB has been shown to be pronounced in AUD, and to be related to the amount of alcohol consumption (14). Finally, inhibitory control is defined as the ability to suppress, delay, or change an inappropriate response (15). It represents a well-investigated component of impulsivity and executive functioning, which is deficient in individuals with AUD (6).
Motivated by cumulative evidence of altered cognitive processes in AUD, research has tested various interventions aimed at modifying attentional bias, approach tendencies, and inhibitory control impairments. The modification of such processes is commonly referred to as cognitive bias modification (CBM). Recently, there has been greater interest in alcohol-specific computerized interventions that target altering these deficits. On the one hand, the focus on alcohol-specific interventions is justified from a clinical perspective, since drinking behavior is often triggered by alcohol stimuli (5). On the other hand, the research perspective stresses the biased and misbalanced processing of such stimuli. The computerization of bias-altering interventions is not only cost-effective, but it also allows for repetitive training, an important prerequisite when automatized processing is to be targeted (16).
Several reviews and meta-analyses have covered literature on one or more of the three aforementioned alcohol-related CBMs, with some of them reaching a rather positive conclusion (17, 18), while others were more cautious (19–22). Most authors agreed that future studies should expand research to clinical samples, clarify which cognitive construct is best targeted, and enlarge knowledge about the moderating factors and mechanisms of action (17, 20, 23). In summarizing data on alcohol-related CBMs, it has been argued that it is necessary to disentangle experimental laboratory studies, which aim to investigate psychological mechanisms in non-clinical samples, from clinical trials, which test the efficacy in a clinical population (24). One important argument for this is the assumption that changing implicit processes will only translate into effective behavior change if participants are motivated to do so. Thus, motivation to change drinking behavior might be a prerequisite for CBM’s effects on drinking behavior. This argument is often heard in conjunction with the assumption that clinical populations are motivated to change their alcohol consumption, in contrast to heavily drinking students, for example. While this may be true when comparing means of both populations, the assumption that every AUD patient has a stable motivation to change alcohol consumption seems rather optimistic. Such an assumption is also challenged by research indicating that motivation in AUD patients varies over time and between subjects (e.g. 25).
This systematic review aims to extend and update those summaries as follows: First, the current database will be expanded by including the most recent research articles. Second, evidence will be thoroughly summarized separately for each of the computerized interventions targeting either attentional bias, approach bias, or inhibitory control processes. Conclusions are drawn for three outcomes, namely drinking behavior, experimental tasks, and neurophysiological effects, while distinguishing between non-clinical experimental lab studies and clinical randomized-controlled studies. In doing so, the potential role of motivation to change drinking behavior is acknowledged and systematically assessed. By visualizing whether an intervention affected the targeted bias and at the same time drinking behavior, light is shed on the assumption that drinking behavior change is associated with and driven by a bias change (see Figures 2 and 3).
Since the predictive value of non-clinical studies for potential benefits in a clinical sample is subject to question, we will compare the different studies’ effects while considering the investigated sample’s severity of drinking behavior. On the one hand, this will be a focus in the summary of each intervention. On the other hand, this review comprises an overall insight into the link between severity of drinking behavior and training effects across all three interventions.
Thorough literature searches in the databases PubMed Medline, Google Scholar, and PsycInfo were conducted in January and May 2019 to identify eligible publications papers on computerized alcohol-specific interventions for attentional and approach bias modification and inhibition training. The sample was to be alcohol-consuming, and studies were to include alcohol-specific interventions with the aim to alter the alcohol-specific biases (i.e. including alcohol-related stimuli). Both clinical and non-clinical samples were represented. The following keywords and all possible combinations thereof were entered into the search masks. Alcohol*, alcohol depend*, addict*, alcohol use disorder, harmful drinking, heavy alcohol use, heavy drink*, hazardous drink*, drink*, alcohol-specific computerized intervention, attention* bias, approach bias, approach-avoidance bias, cognitive bias modification, computerized training, inhibition, inhibitory control, and inhibition training. In order to identify additional papers, a search within Google Scholar was conducted for papers, in which already detected eligible papers for this review had been cited. Furthermore, the bibliographies of the included studies were reviewed. The literature search was restricted to original research articles including a computerized and alcohol-specific CBM intervention that had been published from 2000 through May 2019. Figure 1 shows the selection process of literature included in this review, which ultimately included 40 eligible original research papers.
Eligible studies met the following criteria: the publications (a) were peer-reviewed and published quantitative research studies; (b) involved computerized interventions aimed to alter either attentional or approach bias or inhibitory control; c) implemented alcohol-related stimuli in the computerized intervention; and (d) examined either the effect of interventions on experimental and neurophysiological outcomes in a longitudinal design including a pre- and post-measurement, or the effect of the intervention on drinking outcomes at post-intervention only (including group comparisons of a post-intervention measure, such as taste test, relapse rates 1 year after intervention), and e) were written in English. Studies assessing bias, drinking, or neurophysiology cross-sectionally only were excluded.
Articles found in the literature search were assessed for eligibility by the first author (HB). Once all duplicates were removed, the remaining articles were screened for eligibility based on the inclusion and exclusion criteria. If inclusion was debatable, another author (MS) also assessed the paper and the case was discussed until an agreement was reached.
Studies were categorized as either experimental laboratory study (ELS) if they investigated non-clinical samples, or clinical randomized-controlled trials (RCT) if they described an RCT conducted in a clinical sample containing treatment-seeking patients with AUD. For all three CBMs, this review will initially summarize effects on experimental tasks, i.e. whether the CBM intervention affected the according bias. Thereafter, effects on drinking behavior are reported, as are neurophysiological effects, whenever applicable.
For all studies included in this review, the following data were extracted (see Table 1):
1. name of the first author(s) and publication year
2. the sample description (e.g. heavy drinkers, AUD patients), the sample’s mean age and standard deviation
3. the type of CBM intervention: attentional bias modification (AtBM), approach bias modification (ApBM), or inhibition training (IT)
4. the study design: non-clinical experimental lab study (ELS) or clinical randomized-controlled trial (RCT)
5. the different groups, to which participants were allocated (including size)
6. which task the intervention is based on (e.g. VPT, AAT, etc.)
7. the number of training sessions conducted
8. the outcomes, separated into experimental tasks, drinking behavior, and neuronal activity. As for experimental tasks, all assessed tasks were extracted. Drinking behavior outcomes were summarized according to how long after the intervention they were assessed.
9. remarks on important characteristic of study (e.g. no control group)
Table 1A–C Detailed overview of original research articles included in review according to CBM type [AtBM (1A), ApBM (1B), or IT (1C)], and study design/sample included [non-clinical (ELS) or clinical (RCT)]. Abbreviations are summarized below each sub-table.
The quality of the included studies was assessed based on the Cochrane Collaboration’s Risk of Bias guidelines (65) by the first (HB) and last (FM) authors. Whenever unclear, the second author (MS) was included in the discussion until a conclusion was reached. All information is found in the Supplementary Table 1. Apart from Cochrane criteria, specific methodological factors were also extracted, so that the table contains the following information:
1. Sample composition (e.g. students only, general population, or clinical sample), mean age (+ standard deviation), sex ratio (% female participants)
2. Number of sessions and setting (e.g. lab or web/home-based intervention)
3. Whether motivation (regarded as moderator for CBM effectiveness) was assessed, and if so, if it was included in the analyses
4. Whether a power analysis was conducted prior to the study onset, and whether the calculated N was achieved
5. Adequate generation of allocation to conditions (randomization bias)
6. Blinding procedure (prevention of knowledge of the allocated intervention to participants and/or study member conducting the study: single- or double-blind design)
7. Attrition bias: Initial N and N for (sub-)analyses
Results are summarized first for each CBM separately, before an overall summary is given, which also addresses aspects of potentially moderating variables, such as drinking problem severity and motivation to change alcohol consumption. Both a detailed summarizing table (Table 1), and synoptic figures (Figures 2 and 3) accompany this summary; in Table 1, important details regarding the characteristics and results of each study are listed. Figures 2 and 3 offer a synoptic depiction indicating which study has yielded positive or negative results with respect to bias and drinking behavior change (note that Figure 2 is aligned according to CBM type while Figure 3 is aligned according to drinking problem severity).
Figure 2 Alignment of studies according to CBM type (AtBM, ApBM, or IT) and study type [non-clinical experimental laboratory study (ELS) or clinical randomized-controlled trial (RCT)] and overview of effects on bias change/experimental tasks as well as proximal and distal drinking outcomes. Notes. This figure is organized according to CBM type [i.e. Attentional bias modification (AtBM), approach bias modification (ApBM) and inhibition training (IT)] and within the CBMs differentiated between experimental laboratory study (ELS) including non-clinical samples and randomized-controlled trials (RCT) including clinical samples. Effects are reported as follows, with color coding in parentheses: + significant effect (green); (+) trend (green); +/− mixed results (orange); − no effect of the intervention (red); (−) no effects in ITT analysis, but subgroup with bias change showed drinking reduction (red); * outcome assessed, but not statistically tested or no control group (white); (*) no clear effect of intervention, but pre-post reduction in all groups (including control group) (white); Ǿ not measured (gray). Effects are summarized using a conservative approach in the spirit of intention-to-treat analyses (whole group analyses) and only positive effects observed against a control group were seen as clear evidence. Proximal effects: up to 1 month; Distal effects: 1 month and more. AAT, Approach Avoidance Task; ApBM, Approach Bias Modification; AtBM, Attentional Bias Modification; AUD, Patients With Alcohol Use Disorders; CBM, Cognitive Bias Modification; ELS, Experimental Laboratory Study; GNG, Go/Nogo Task; HD, Heavy Drinkers; HFD, Harmful Drinkers; HZD, Hazardous Drinkers; IAT, Implicit Association Task; IT, Inhibition Training; RCT, Randomized-Controlled Trial; SD, Social Drinkers; SRC, Stimulus Response Compatibility Task; SST, Stop-Signal Task; TT, Taste Test; VPT, Visual Probe Task. Reading example: In those RCTs on ApBM, which measured both, bias change and drinking effects (26–28), one can see that the effects on bias change (column “bias change”) consistently point in the same (positive) direction as the effects on proximal and distal drinking outcomes. In the ELS studies on ApBM, not only is this pattern less clear, but one can also see that two-thirds of the studies failed to report a bias change in the first place.
Figure 3 Alignment of all studies according to description of the samples’ severity of drinking behavior and overview of effects on experimental tasks and proximal and distal drinking outcomes. Notes. Effects are reported as follows, with color coding in parentheses: + significant effect (green); (+) trend (green); +/− mixed results (orange); − no effect of the intervention (red); (−) no effects in ITT analysis, but subgroup with bias change showed drinking reduction (red); * outcome assessed, but not statistically tested or no control group (white); (*) no clear effect of intervention, but pre-post reduction in all groups (including control group) (white); Ǿ not measured (gray). Effects were summarized using a conservative approach in the spirit of intention-to-treat analyses (whole group analyses) and only positive effects observed against a control group were seen as clear evidence. Proximal effects: up to 1 month; Distal effects: 1 month and more. AAT, approach avoidance task; ApBM, Approach bias modification; AtBM, Attentional bias modification; AUD, patients with alcohol use disorders; CBM, cognitive bias modification; ELS, experimental laboratory study; GNG, Go/NoGo task; HD, heavy drinkers; HFD, harmful drinkers; HZD, hazardous drinkers; IAT, implicit association task; IT, inhibition training; RCT, randomized-controlled trial; SD, social drinkers; SRC, Stimulus response compatibility task; SST, stop-signal task; TT, taste test; VPT, visual probe task. Reading example: In the RCTs summarized in the lower part of the figure, one can see that the effects on bias change (column “bias change”) mostly point in the same direction as the effects on proximal and distal drinking outcomes. In the ELS studies, this pattern is inconsistent.
AtBM aims to alter AtB by training to direct attention away from alcohol-specific cues (40). The intervention is typically based on the visual probe task (VPT), during which alcohol-specific and control pictures appear simultaneously on a computer screen. When they disappear, a visual probe appears in the location of one of the previously shown pictures, and participants are required to react accordingly (35). During the VPT-AtBM intervention, the visual probe consistently replaces the control pictures, thus training participants to direct their attention to these pictures and away from the alcohol-related pictures. Such an AtBM intervention is then usually compared to a control condition, during which probes are divided equally between alcohol-specific and neutral stimuli. Certain other studies, however, feature different control conditions (e.g. 31, 35).
Some studies (33, 34, 41) based their intervention on a Stroop-like rationale (Stroop-AtBM) aiming to train participants to ignore the task-irrelevant aspect of stimuli (e.g. alcohol-relatedness), and to respond faster to another aspect (e.g. the color). Table 1A summarizes twelve studies investigating alcohol-specific computerized AtBM.
Research investigating the effects of VPT-AtBM has reported changes in VPT-AtB in the expected direction in young adult drinkers (38), heavy social drinkers (35), heavy drinkers (HD) (10, 32, 40), and AUD (31). Another study in AUD showed a trend (27), while two studies in AUD (29, 30), and one in binge drinkers (36) found no effects. It is also noteworthy that in Rinck et al. (27), neither baseline bias nor bias change did mediate drinking changes. In the seven studies investigating the generalization to other tasks, four reported no generalization (e.g. the Stroop (10), the flicker (10, 40), the stimulus response task (10), the implicit association task (30) or the visual search task [32)]. Meanwhile, one study found VPT-AtBM to inflict expected changes in both an implicit association task and an alcohol-specific Stroop task (38). Further, two studies reported an effect of AtBM on alcohol approach inclination as measured by an approach-avoidance test (27) or by eye-movements in a free viewing task (37). When employing Stroop-AtBM, which is inspired by, but not identical to a Stroop paradigm, effects on an alcohol-related Stroop task were reported for hazardous and harmful drinkers (34). This might be interpreted as evidence for generalization to a new—albeit similar—task. The sole study that investigated the persistence of AtBM effects over time reported that the effects of a Stroop-AtBM on an alcohol-related Stroop-task could still be found at 3-month follow-up (34).
Regarding the effect of VPT-AtBM on alcohol consumption in experimental lab studies, two studies reported less drinking during (39) or immediately after the intervention period (35), while three studies failed to observed such effects in non-clinical samples immediately (10, 36) or 2 weeks after the intervention (32). In a so-called preference test, which does not measure drinking behavior since participants simply choose between alcoholic and non-alcoholic beverages without actually drinking them, no differences were found either (40).
Two studies reported a decrease in alcohol consumption in non-clinical samples 1 week or 3 months after Stroop-AtBM, however these studies either lacked a control group (34) or the effect was observed in all groups (41). Taken together, experimental laboratory studies do not suggest that AtBM reliably changes drinking behavior. It has been argued that this might be attributable to a lack of motivation to change drinking behavior. However, the only experimental lab study which included non-clinical harmful drinkers motivated to cut down their drinking only partially aligns with this assumption: In this study investigating the effects of Stroop-AtBM on drinking behavior, effects were either marginal or not significant at post-intervention. Nevertheless, at later time points, there were significant effects on different measures of alcohol consumption at 3-month follow-up, with effects partially persisting even until 6 months after the intervention (33).
Generally, motivation to change is assumed to be higher in populations with more problematic drinking patterns. When considering clinical trials on AtBM in AUD, however, two of four studies reported that AtBM increases the time to first drink at 3-month follow-up (31) or reduces relapse rates at 1-year follow up (27), while two other studies failed to report such effects at 4-week (29) or 1-year follow-up (30). Interestingly, those studies, which reported evidence for a bias change (27, 31) were also those reporting drinking effects, while the studies, in which the bias could not be changed did not find any effects on drinking behavior (29, 30).
To the best of our knowledge, there are no studies investigating neurophysiological effects of AtBM.
Considering effects on experimental tasks, two-thirds of the studies showed that AtBM influences AtB when measured with a similar task. Support for generalization to other cognitive tasks is limited, with only one-third of the studies reporting such effects. It is possible that these tasks measure different and only slightly intercorrelated incentive-motivational aspects of alcohol-related cues that remain unaffected by the intervention.
Beneficial effects of AtBM on drinking behavior were only observed in the minority of experimental lab studies. In clinical RCTs, where the motivational prerequisites for the translation of bias to drinking behavior change might be given more often, the two studies observing a bias change also observed changes in drinking behavior, while the two studies that failed to change the bias reported no drinking changes. Note however, that the only study analyzing specifically whether bias change mediates changes in drinking behavior did not show such a mediation (27).
It has been suggested that drinking behavior change should only be expected if a bias change was achieved and if participants are motivated to change their drinking behavior (24). Considering that, one might argue that the reported “synchrony” in bias change and drinking change is in line with this expectation, but the failure to observe a mediation effect in 27 speaks against such a conclusion. Challenging this view more generally, Cristea et al. (66) have argued that it is questionable to select only the studies with successful bias change when it comes to the summary of effects on drinking behavior.
Regardless of this controversy, it remains to state that in clinical trials, the intended effects on bias change or drinking behavior, respectively, could not be reliably replicated. Factors influencing this variability must be identified, experimentally examined, and optimized if AtBM is to truly help clinical populations with AUD.
Alcohol approach/avoidance inclinations can be assessed with the Approach Avoidance Task (AAT), which has also been the basis for the development of an intervention targeting action tendencies (55). In this ApBM intervention, pictures of alcoholic and non-alcoholic beverages are presented. Depending on the format (landscape or portrait), participants must react either by avoiding the cue, i.e. pushing a joystick away from themselves, or approaching the cue, i.e. pulling a joystick toward them (55). In the AAT, which is used to assess action tendencies, alcohol-related pictures appear with equal probability in either landscape or portrait format. The differences in reaction time between the push and the pull trials reflect approach/avoidance biases. In the training version to modify AAT (AAT-ApBM), however, the picture type is linked to the picture format: Aiming to decrease alcohol approach tendencies, alcohol-related pictures appear in the format that required participants to avoid the stimulus. In non-clinical trials, opposite contingencies, i.e. pairing alcohol-related pictures with the approach reaction, potentially increasing ApB, are often used in control conditions. Because such a condition is less favorable in AUD patients, clinical studies have used control conditions using either equal probabilities for the alcohol-push and -pull-pairings (e.g. 28) or neutral images (e.g. 44). Table 1B summarizes 15 papers investigating alcohol-specific computerized ApBM.
ApBM was shown to modify alcohol avoidance and approach bias (AvB/ApB) in the expected direction in social drinkers (54), hazardous drinkers (HZD) (55), risky drinkers (51), and AUD (26–28). However, replication of these effects failed in six studies in social (53), heavy (48, 50, 52, 53), hazardous drinkers (49), and AUD (42, 46). Loijen et al. (43) reported an ApB reduction in AUD but did not test this effect against a control group, thereby limiting the statistical explanatory power.
Regarding generalization of effects to new tasks, two studies showed a generalization to the IAT (28, 55). Yet, three studies conversely found no effect on the IAT (49, 50, 53), neither did these same studies find an effect on ApB in the first place. Sharbanee et al. (54) reported generalization to another measure of ApB, but not to a measure of selective attention.
With respect to working mechanisms, there is some evidence that the effect of ApBM on ApB is moderated by strong baseline ApB (26), and that the training effect on drinking outcomes is mediated by change in ApB (26, 54, 55). However, this mediation effect could neither be replicated for baseline ApB nor for change in ApB in a clinical trial by Rinck et al. (27). In another clinical sample, neither change in ApB scores nor change in general IAT scores mediated the effect of ApBM on relapse rates (28), while alone stimulus-specific IAT scores did (45).
Experimental lab studies conducted in non-clinical samples supposedly not motivated to change drinking behavior reported no effects on drinking during a post-intervention taste test in social (54), heavy (52) or hazardous drinkers (55). However, additional analyses in two of these studies (54, 55) indicated that successfully trained participants, whose ApB/AvB scores changed in the intended direction, showed the expected alterations in drinking behavior. Additionally, one study did find HD to drink significantly less than controls during a taste test (50). Four studies with hazardous and heavy drinkers observed no effects of ApBM on alcohol consumption at 1-week, 1-month, or 3-month post-intervention (41, 48, 49, 51).
Contrary to these findings in experimental lab studies, the majority of clinical RCT trials did report effects of ApBM on drinking behavior. One study in AUD showed a 21.4% lower relapse rate in the ApBM compared to the control group at 2-week follow-up (marginally significant), but did not report differences regarding time to relapse, mean drinking days, and mean standard drinks per drinking day (44). Long-term effects of ApBM on drinking behavior were analyzed in three clinical studies with AUD samples, with clinically relevant reductions in relapse rates at 1-year follow-up of 13% (marginally significant) (28), 8.5% and 7.7% (26, 27, both significant). The pattern of results in ApBM thus aligns with the assumption that the translation of bias change to effects on drinking behavior works better in clinical samples, assumedly because patients are more motivated to reduce drinking. However, none of the clinical trials on ApBM actually measured their participants’ motivation to change.
fMRI studies showed ApBM to decrease amygdala activation during a cue-reactivity task, an effect also related to decreased subjective craving (46). Comparing brain activation during the AAT from pre- to post-intervention, ApBM reduced activation in the medial prefrontal cortex (mPFC), specifically in the dorsal anterior cingulate cortex (dACC) and middle frontal gyrus. The decrease in dACC was also positively correlated to ApB changes (47). By contrast, the only electrophysiological study examining the P3 peak amplitude during an oddball and cue reactivity task yielded no significant effects (49).
Analyses on experimental data is mixed: about half of the studies report an effect of ApBM on ApB while the other half does not. Thus, compared to earlier reviews (e.g. 24), this systematic review of all currently available data suggests a more cautious evaluation of the potential of ApBM to impact ApB, underlining the necessity to clarify under which circumstances ApBM can change the targeted bias. To date, it seems that bias change can be more reliably achieved in clinical populations (three out of four studies) than in non-clinical experimental lab studies (three out of eight studies). This might be due to clinical populations showing a stronger ApB at baseline, which has been shown to moderate ApBM effects (26, but see 27), while ApB baselines are rarely assessed. Remarkably, if other tasks were included in the study, those studies observing positive effects on ApB could also report generalization of effects. This pattern is consistent with mediation analyses and earlier reviews suggesting that ApBM achieves its beneficial effects via ApB changes (18, 26, 45, 54, 67), even if other studies did not find such mediation (27 and 28).
In clinical populations, ApBM reduced alcohol consumption and relapse rates in AUD (26–28, 44), the limitation being that some of these effects are marginally significant. Nevertheless, since they comprise remarkable effects on relapse rates, they are considered to be of clinical relevance. The majority of studies in non-clinical samples showed no effects on drinking behavior even after multiple sessions of ApBM (41, 42, 54, 55). This discrepancy between clinical and non-clinical samples is discussed in terms of motivational differences (17). It might, however, also be due to the difficulty in achieving a bias change in non-clinical populations in the first place. The proposed working mechanism suggests that a change in drinking behavior should only be expected if the according bias alteration could be achieved first. In line with this assumption, all the clinical trials investigating bias changes and reporting beneficial effects on drinking behavior could also show a bias change (see Figure 2). In the seven pre-clinical experimental laboratory studies investigating both bias change and drinking outcomes, four reported consistent (non-)effects (i.e. bias change and drinking behavior change, or no bias change and no drinking behavior change), with one study reporting a bias as well as a drinking change (54), and three showing neither a bias change nor a drinking change (48, 49, 52). One study (55) reported a bias but no drinking change in the whole group analysis, but reported a significant drinking reduction for those whose bias had been changed successfully by the intervention. In the two remaining studies, behavioral and cognitive effects diverge, with one reporting no bias change, albeit a reduction in drinking behavior (50) and one showing a bias but no drinking behavior change (51), the latter presumably explained by a lack of motivation to change.
Overall, we conclude that a) the evidence speaks for the proposed working mechanism of ApBM to work via changes in ApB and that b) this bias change as well as changes in drinking behavior could quite consistently be shown in clinical populations. However, c) effects regarding bias change as well as drinking behavior change are inconsistent in non-clinical experimental studies. Earlier reviews (e.g. 24) explained the lack of effects in drinking behavior in non-clinical populations with the lack of motivation to change. But this argument cannot be extended to explain the variation of effects regarding bias change, as this is supposed to be independent of motivation or even awareness. However, it is possible that variations in baseline ApB, which might differ between clinical and non-clinical samples, could explain this divergence.
Neurophysiological effects were reported using fMRI (4647) but not EEG (49), and indicate that ApBM induces changes in regions related to saliency (Amygdala) and motivational values of stimuli (mPFC).
Two tasks measuring different aspects of inhibitory control (68), the Go-NoGo-task (GNG, measuring action restraint) and the Stop signal task (SST, measuring action cancellation), served as a basis for the development of IT. To date, the majority of IT studies have based their training intervention on a modified GNG, in which alcohol-related stimuli are paired consistently with a stopping response. This alcohol-NoGo-intervention has either been contrasted with a control condition, in which alcohol-related stimuli are consistently paired with a Go response, or with control conditions utilizing only neutral stimuli in Go and NoGo trials. Alcohol-specific IT based on a modified SST was also developed, but to date tested in only one study (59): 59 used an SST-based alcohol-specific IT in which an auditory cancellation signal was consistently paired with alcohol-related stimuli. In the control conditions, cancellation was either paired with neutral cues or auditory cues were to be ignored.
The discussion about the potential working mechanisms and the conceptual framing of IT followed mainly two lines: One assumption is that IT improves inhibitory control over a patient’s reaction to alcohol-specific cues (69). Following this line, effects of IT would be expected in inhibitory control tasks. Another explanation, the stimulus devaluation hypothesis (70) suggests that IT is a means to decrease motivational properties of a stimulus. This hypothesis assumes that IT affects implicit semantic associations to alcohol-specific cues and has led to the definition of IT as a form of (implicit) memory bias alteration (24). In the section IT: Effects on Experimental Tasks, we will review whether existing data speaks in favor of one or the other hypothesis and whether the conceptual framing of IT as a form of memory bias modification is still justified. Table 1C summarizes ten studies investigating alcohol-specific computerized IT.
Considering the mechanism of action of IT, two main hypotheses have guided the selection of experimental tasks employed in these studies. The first and more straightforward hypothesis is that IT increases inhibitory control (69) and should thus affect participant’s performance on tasks such as the GNG or the SST. The second hypothesis, the stimulus devaluation hypothesis (70), states that consistently pairing a stimulus with a stopping response decreases the stimulus’ valence and motivational properties, an effect observable in tasks such as the implicit association task (IAT).
The effects of alcohol-specific IT on inhibitory control have been investigated in six studies: Regarding SST-based IT, one study reported a selective decrease in inhibition errors in alcohol-related stop trials when comparing the first to the last training block which might indicate an improvement of inhibitory control (59), while another study reported no effects on the SST (60). Regarding GNG-based IT, four studies reported no effects on inhibitory control as indicated by an SST (50, 58, 60, 61) or by reaction times during Go-trials on a GNG (50). Strickland et al. (64) reported a decrease in errors of commission (EOCs) during training, but could not compare this finding to a control group. Notably, however, there is no data on whether GNG-based IT alters the number of EOCs during a GNG performed after training, even though these are a typical parameter of inhibitory control (6).
The effects of alcohol IT on implicit associations have been examined in seven studies. Two studies reported that GNG-based IT decreased positive implicit associations to alcohol (57, 58), supporting the stimulus devaluation hypothesis. Yet, five subsequent studies were unable to replicate this effect for GNG-based IT (50, 56, 60, 61, 63) or SST-based IT (60). As the IAT effect originally motivated the conceptual framing of IT as a form of memory bias modification, this conceptualization seems questionable in light of this current summary.
No generalization was observed on automatic action tendencies (58) nor on a stimulus response compatibility task (61).
To date, effects on alcohol-specific IT on drinking behavior have so far only been investigated in non-clinical samples, and as such, have primarily included participants who lack the motivation to change their drinking behavior. Motivation to change is discussed to be a necessary prerequisite for any CBM to affect drinking behavior (24). Even if the sample in the Strickland et al. (64) study was required to fulfill the DSM-5 criteria for AUD, the participants were not seeking treatment. Moreover, less than 20% of the sample reported a desire to reduce drinking, which is why this study is classified as an experimental lab study rather than a clinical randomized controlled trial in this review. With respect to alcohol consumption measured in a taste test immediately after the intervention, four studies reported a decrease due to alcohol-specific IT (50, 56, 59, 62), one study reported a non-significant trend (57), and another observed no effect other than an unexpected increase in one of the experimental conditions (63). The sole effect in Kilwein et al. (61) was that it took the experimental group significantly longer to take the first sip of an alcoholic beverage. Across the 2 weeks of IT training, no reduction in alcohol consumption could be found in one study (64). When assessing alcohol consumption for 1 or 2 weeks post-intervention, three studies reported a decrease due to alcohol-specific IT (57, 58, 61). Interestingly, in two of these studies (57, 61) participants in the control condition also drank significantly more. One study (64) found a reduction of overall drinking days, but no effect on heavy drinking days. Three studies found no significant effects of IT on drinking behavior at 1-week follow-up (56, 59, 63), and in another study, the experimental group (Beer NoGo) targeted to reduce drinking even drank significantly more than the control group (Beer Go; 62). One study investigated the effects of IT in HD, who had reported to be motivated to reduce alcohol consumption, but found no significant effects on drinking across the 4-week study participation, nor at 2-, 4-, and 6-week follow-up (60). Lastly, Liu et al. (62) found a decrease of alcohol consumption at 4-week follow-up in all groups, including the control condition.
To date, the only study investigating neurophysiological effects of IT in HD (56) concentrated on frontal EEG asymmetry in the alpha frequency band as an indicator of approach motivation. Results showed an insignificant change in frontal EEG asymmetry, corresponding to decreased approach motivation.
Considering the effects on experimental tasks, the data currently contradicts earlier summaries (24, 67), and fails to support the notion that IT works via stimulus devaluation, at least not when assessed with the IAT (50, 56, 60, 61, 63). Thus, while earlier reviews conceptualized IT as a form of memory bias alteration (24), our current review shows that this conceptualization is contradicted by the available data and seems no longer justifiable. With respect to inhibitory performance, only one study (59) reported an immediate effect of IT on inhibitory performance, while five studies failed to report such effects when assessing inhibition with a SST (57, 58, 60), a flanker task (63), or with Go reaction times during a GNG (50). These results leave the question of how IT eventually works still unanswered. In the case of an inhibitory working mechanism, there are, however, two points to be considered: Firstly, there is a noticeable lack of data on a proximal experimental outcome of a GNG-IT, the analysis of errors of commission during a GNG. Secondly, most IT studies used Go-NoGo ratios of 50/50, making inhibition less strenuous, thus possibly minimizing effects. The only study with a higher ratio (63; ratio of 75/25) assessed inhibitory effects with a flanker task but did not include an experimental assessment conceptually closer to the intervention.
Studies describing effects of IT on drinking behavior have only been conducted in non-clinical samples. Summarizing all existing data, one might suggest cautiously that IT has an effect on drinking behavior during or immediately after the intervention, with three (50, 56, 59) of seven studies reporting such effects and one reporting marginal effects (57). Longer effects on drinking behavior were observed in less than 50% of the studies (see Figure 2). Importantly, all of the aforementioned studies were experimental lab studies, thus the majority of their participants might have lacked the motivation to change their drinking behavior, which is discussed to hamper the translation of CBM effects to drinking behavior (24). However, the sole study recruiting participants with a self-reported motivation to change drinking showed no effects of IT on drinking behavior (60).
Although the number of IT studies has nearly doubled since recent meta-analyses (20, 21), one still must acknowledge a considerable variability in size and stability of IT effects on drinking behavior. Furthermore, there is still a lack of clinical studies in patients with AUD and of data from longer follow-up periods, both critical to clinically relevant conclusions. Extending the investigation of IT to clinical samples is also vital because one might tentatively speculate that a pattern similar to ApBM with more promising effects in clinical populations could emerge. A meta-analysis (21) summarizing studies on IT together with other CBMs reported stable effects on bias change but also questioned CBMs effects on drinking behavior. Importantly, our summary of the now larger number of studies on IT does not align with this conclusion: If any, we see an effect of IT on short-term drinking behavior, but not on bias change. This divergent pattern of effects in IT compared to other CBMs might be attributable to a suboptimal assessment of bias in IT: While in AtBM and ApBM studies, most bias assessments used in the studies strongly resembled the intervention to modify the according bias (e.g. VPT to assess attentional bias, VPT-AtBM to modify the bias), this could not be seen in IT as consistently.
We set out to compare the effects of CBM with respect to severity of drinking behavior of the investigated sample. As reported, ApBM has shown more consistent beneficial results in clinical samples, while for AtBM, this pattern is less consistent with two positive and two negative trials. Meanwhile, there are no clinical studies on IT in treatment-seeking patients motivated to change drinking In a first-time attempt to analyze the relation between drinking severity and CBM effect over all three interventions, studies using the alcohol use disorder identification test (AUDIT) were aligned according to the mean AUDIT value (Figure 4). In a further step, all studies were aligned by sample description with less severe samples at the top and AUD patients at the bottom (Figure 3). By doing so, we took a conservative approach, concentrating on those effects reported for the whole group in the spirit of intention-to-treat analyses, while ignoring effects found only in re-analyses focused on a certain subgroup. Furthermore, we considered only the effects that were shown against a control group (i.e. interactions) as clear evidence of a CBM effect. We infer the following from this alignment: There seems to be no continuous improvement of CBM effectiveness with increasing severity, meaning that outcome patterns do not improve with severity within the non-clinical experimental lab studies. Rather, there seems to be a categorical effect with better outcomes in randomized controlled trials with clinical samples compared to experimental lab studies with non-clinical samples. Regarding drinking outcomes, this might be due to the higher motivation to change expected in clinical samples. One must keep in mind however, that this pattern may be due largely to the rather consistent effects that one of the CBM interventions, ApBM, has shown in clinical samples (see Figure 3), thus generalization to all CBMs is questionable. Interestingly, the pattern of results within the clinical randomized controlled trials is in line with the proposed working mechanism, which suggests that a change in drinking behavior is driven by the modification of the according bias: Figure 2 shows that in those RCTs with bias change, drinking behavior changed as well, while those RCTs without bias change showed no drinking behavior changes. Unfortunately, however, this conclusion is supported by only one successful mediation analysis (26), but challenged by two clinical randomized controlled trials that failed to show such mediation effects (27, 28).
Figure 4 Alignment of studies according to AUDIT means and overview of proximal and distal drinking outcomes. Notes. Columns show if study yielded significant effect, indicating that the intervention reduced drinking behavior or relapse rates. Effects are reported as follows + significant effect; (+) trend; +/− mixed results (if different measures were reported); − no effect of the intervention; (−) no effects in ITT analysis, but subgroup with bias change showed drinking reduction; * outcome assessed, but not statistically tested or no control group; (*) no clear effect of intervention, but pre-post reduction in all groups (including control group); Ǿ not measured. Effects were summarized using a conservative approach in the spirit of intention-to-treat analyses (whole group analyses) and only positive effects observed against a control group were seen as clear evidence. Proximal effects: up to 1 month; Distal effects: 1 month and more. Top row: WHO terminology to categorize drinking behavior according to scored AUDIT values: non-problematic: AUDIT 0–7; hazardous: AUDIT 8–15; harmful: AUDIT 16–19; probability of dependence: AUDIT > 20. If not stated in the original research paper, pooled means and standard deviations of AUDIT values were calculated. RR, Relapse rate; TT, Taste test, WHO, World Health Organization.
What also becomes evident in Figure 4 is the considerable variation in the use of terminology in sample descriptions: Indeed, the thorough inspection of the literature unveiled a deficiency in standardized definitions for alcohol-related drinking types. When aligning papers along the AUDIT mean values of the investigated samples, it becomes evident that sample descriptions (e.g. HD) are not uniformly used. To our knowledge, no papers have systematically addressed either the problem of drinking type definitions, the variability in terminology and/or the resulting limitations regarding reviews and meta-analyses. One handicap is that some of the terms used for sample description lack a clear, commonly accepted definition (e.g. heavy social drinkers). Furthermore, the alignment with the AUDIT values also shows that the term used may occasionally be misleading or fail to apply to the whole sample, as can be seen from the AUDIT means and standard deviations. For instance, while a sample is called “heavy social drinkers”, this may include AUDIT values indicating hazardous to even alcohol-dependent participants, whereas a sample called “regular drinkers” may include AUDIT values of hazardous drinkers values of hazardous drinkers (as defined e.g. by the World Health Organisation, WHO). In short, only referring to the terminology used in a given publication might imply one drinking type, while the AUDIT scores might point to an additional drinking type. Comparability of studies and clarity of findings would benefit from the use of unified terminology, as has for example been proposed by the WHO (71; see also top of Figure 4 for WHO terminology: non-problematic use, hazardous use, harmful use, and probability of dependence).
Generally, there is still limited research elucidating computerized CBM’s effects. The abundance of cross-sectional studies reporting altered cognitive processing in HD/AUD, which led to the development of computerized interventions, is contrasted by a limited number of longitudinal studies that investigate computerized interventions’ effectiveness, let alone working mechanisms or neuronal effects. We reviewed 40 papers on alcohol-specific computerized CBM interventions. In doing so, we set out to analyze all studies available to date on three alcohol-specific CBMs, namely AtBM, ApBM, and IT, and to summarize this data regarding effects on drinking behavior, experimental tasks, and neurophysiological effects. In contrast to earlier analyses of several (72, 73) or one CBM (18–20,), we summarized data for each CBM separately, subsequently comparing them to one another. Our cautious conclusion after that comparison is as follows: ApBM has shown the most consistent effects in clinical samples, while evidence on AtBM is more inconsistent, and data on IT is still lacking important information, such as the effectiveness in clinical samples and the effects on inhibitory control itself.
Several limitations when comparing CBM studies and in terms of this review should be addressed. Firstly, despite having carefully searched several databases and articles, we cannot fully rule out having missed a paper that should have been included in this review. Secondly, because the non-uniform use of terminology regarding the subjects’ drinking behavior complicates conclusions with respect to severity, we strongly encourage future studies to lean on the WHO’s definitions depending on the achieved AUDIT score across the sample (71). Furthermore, the studies in this review primarily included student samples. Only few non-clinical studies investigated representative and socio-economically mingled samples, and less than one-third of the available studies were conducted in clinical populations, in which these interventions should ultimately be implemented. Beside the fact that students generally represent a rather young and cognitively strong sample, which may impact results, students may also lack the motivation to change their drinking behavior. Motivation to change, which is discussed as a prerequisite or mediator for a successful intervention (e.g. 17, 24), is assessed in some studies only (32, 34, 41, 49), and even more seldom included in the analyses (see Supplementary Table 1). It is highly probable that on average, patients show a higher motivation to change their drinking behavior when compared to non-clinical samples. But even among patient samples, motivation to change is not a fully stable and omnipresent phenomenon. Thus, future research would profit from the assessment of motivation to change in the participants. Also, baseline levels of the biases which are to be targeted by the intervention may vary among individuals. Unfortunately, those levels are rarely reported, even if they have been shown to moderate an intervention’s effectiveness (26, but see 27) and were considered relevant in a recent review on AtBM (75).
Further, regarding methodological variation, the number of sessions ranged between one and 14 in the articles reviewed, and within certain studies, participants completed different numbers of interventions. Earlier research suggests that it is unlikely for one session to have a therapeutic effect (21). For ApBM, it has been demonstrated that the optimal number of training sessions shows strong interindividual variance, with six sessions being the mean (76). This suggests that most of the studies reviewed here may not have reached the optimal effect with the intervention. In addition, the web-based vs. laboratory setting may have led to differences in study compliance. An alternative explanation for the discrepant results regarding AtBM might be attributable to the fact that the three studies testing multiple sessions in HD conducted the training sessions at home (i.e. over the web), which might have reduced the participants compliance, attention, and/or motivation (24, 74). Also, most papers did not tailor the pictures presented according to participants’ drinks of choice. Since a subjects’ preferred drink has a higher incentive value than other types of beverage, the use of individually meaningful stimulus material may play a pivotal role and influence the effects of CBM interventions. Lastly, the lack of neurophysiological assessments (see Table 1) investigating neuronal processes underlying bias change and effects on drinking outcomes is regrettable and hampers any firm conclusions in this realm. As all three of the CBMs can at least in part be linked to basic and clinical neuroscientific concepts, the scientific anchoring of these interventions would profit from expanded knowledge regarding their neuroscientific effects.
Overall, it seems advisable for future research to concentrate on clinical populations, include the assessment of motivation and baseline bias levels, analyze mediation, and neurophysiological effects and use longer follow-up periods to augment the understanding of computerized interventions’ effects.
HB conducted the literature search in January and May 2019. HB and MS equally contributed to tables, figures, and writing the initial manuscript. HB, MS, and FM elaborated conclusions. HB, MS, RT, LS, and FM contributed to revising the manuscript and finalizing it. HB and FM assessed each study for quality criteria. Whenever unclear, MS was asked for her opinion.
This research was supported by a grant from the Swiss National Science Foundation (grant-nr: 105319_159286) to MS, LS and FM.
The authors declare that the research was conducted in the absence of any commercial or financial relationships that could be construed as a potential conflict of interest.
We would like to express our deepest gratitude to Stephan Furger for his assistance with the tables and figures, to Sarah Diezig for her support with Tables 1A–C, and to Sarah Batschelet for copyediting.
The Supplementary Material for this article can be found online at: https://www.frontiersin.org/articles/10.3389/fpsyt.2019.00871/full#supplementary-material
1. Rehm J. The risks associated with alcohol use and alcoholism. Alcohol Res Health (2011) 34(2):135–43.
2. Anton RF, O’Malley SS, Ciraulo DA, Cisler RA, Couper D, Donovan DM, et al. Combined pharmacotherapies and behavioral interventions for alcohol dependence: the COMBINE study: a randomized controlled trial. JAMA (2006) 295(17):2003–17. doi: 10.1001/archpsyc.65.2.135
3. Wiers RW, Becker D, Holland RW, Moggi F, Lejuez CW. Addictions. A Social Psychological Perspective. In: Kopetz CE, Lejuez CWeditors. Cognitive motivational processes underlying addiction treatment. Routledge (2016). p. 201–36.
4. Franken IH, Rosso M, Van Honk J. Selective memory for alcohol cues in alcoholics and its relation to craving. Cogn Ther Res (2003) 27(4):481–8. doi: 10.1023/A:1025480615623
5. Field M, Cox WM. Attentional bias in addictive behaviors: a review of its development, causes, and consequences. Drug Alcohol Depend (2008) 97(1):1–20. doi: 10.1016/j.drugalcdep.2008.03.030
6. Smith JL, Mattick RP, Jamadar SD, Iredale JM. Deficits in behavioural inhibition in substance abuse and addiction: a meta-analysis. Drug Alcohol Depend (2014) 145:1–33. doi: 10.1016/j.drugalcdep.2014.08.009
7. Field M, Wiers RW, Christiansen P, Fillmore MT, Verster JC. Acute alcohol effects on inhibitory control and implicit cognition: implications for loss of control over drinking. Alcohol Clin Exp Res (2010) 34(8):1346–52. doi: 10.1111/j.1530-0277.2010.01218.x
8. Stein M, Fey W, Koenig T, Oehy J, Moggi F. context-specific inhibition is related to craving in alcohol use disorders: a dangerous imbalance. Alcohol Clin Exp Res (2018) 42(1):69–80. doi: 10.1111/acer.13532
9. Volkow ND, Baler R. Addiction science: uncovering neurobiological complexity. Neuropharmacology (2014) 76:235–49. doi: 10.1016/j.neuropharm.2013.05.007
10. Field M, Duka T, Eastwood B, Child R, Santarcangelo M, Gayton M. Experimental manipulation of attentional biases in heavy drinkers: do the effects generalise? Psychopharmacol (Berl) (2007) 192(4):593–608. doi: 10.1007/s00213-007-0760-9
11. Palfai TP, Ostafin BD. Alcohol-related motivational tendencies in hazardous drinkers: assessing implicit response tendencies using the modified-IAT. Behav Res Ther (2003) 41(10):1149–62. doi: 10.1016/S0005-7967(03)00018-4
12. Field M, Marhe R, Franken IHA. The clinical relevance of attentional bias in substance use disorders. CNS Spectrums (2014) 19(3):225–30. doi: 10.1017/S1092852913000321
13. Cox WM, Fadardi JS, Pothos EM. The addiction-stroop test: theoretical considerations and procedural recommendations. Psychol Bull (2006) 132(3):443. doi: 10.1037/0033-2909.132.3.443
14. Watson P, De Wit S, Hommel B, Wiers RW. Motivational mechanisms and outcome expectancies underlying the approach bias toward addictive substances. Front Psychol (2012) 3:440. doi: 10.3389/fpsyg.2012.00440
15. Jones A, Christiansen P, Nederkoorn C, Houben K, Field M. Fluctuating disinhibition: implications for the understanding and treatment of alcohol and other substance use disorders. Front Psychiatry (2013) 4:140. doi: 10.3389/fpsyt.2013.00140
16. Lindenmeyer J. Is there a case in neuropsychological relapse prevention in alcoholic patients? Suchttherapie (2010) 11(04):166–72.
17. Gladwin TE, Wiers CE, Wiers RW. Interventions aimed at automatic processes in addiction: considering necessary conditions for efficacy. Curr Opin Behav Sci (2017) 13:19–24. doi: 10.1016/j.cobeha.2016.08.001
18. Kakoschke N, Kemps E, Tiggemann M. Approach bias modification training and consumption: A review of the literature. Addict Behav (2017) 64:21–8. doi: 10.1016/j.addbeh.2016.08.007
19. Christiansen P, Schoenmakers TM, Field M. Less than meets the eye: reappraising the clinical relevance of attentional bias in addiction. Addict Behav (2015) 44:43–50. doi: 10.1016/j.addbeh.2014.10.005
20. Allom V, Mullan B, Hagger M. Does inhibitory control training improve health behaviour? A meta-analysis. Health Psychol Rev (2016) 10(2):168–86. doi: 10.1080/17437199.2015.1051078
21. Cristea IA, Kok RN, Cuijpers P. The effectiveness of cognitive bias modification interventions for substance addictions: a meta-analysis. PloS One (2016) 11(9):e0162226. doi: 10.1371/journal.pone.0162226
22. Stock A-K. Barking up the Wrong Tree: Why and How We May Need to Revise Alcohol Addiction Therapy. Front Psychol (2017) 8:884.
23. Jones A, Di Lemma LC, Robinson E, Christiansen P, Nolan S, Tudur-Smith C, et al. Inhibitory control training for appetitive behaviour change: A meta-analytic investigation of mechanisms of action and moderators of effectiveness. Appetite (2016) 97:16–28. doi: 10.1016/j.appet.2015.11.013
24. Wiers RW, Boffo M, Field M. What’s in a Trial? On the importance of distinguishing between experimental lab studies and randomized controlled trials: the case of cognitive bias modification and alcohol use Disorders. J Stud Alcohol Drugs (2018) 79(3):333–43. doi: 10.15288/jsad.2018.79.333
25. DiClemente CC, Bellino LE, Neavins TM. Motivation for change and alcoholism treatment. Alcohol Res Health (1999) 23(2):87–92.
26. Eberl C, Wiers RW, Pawelczack S, Rinck M, Becker ES, Lindenmeyer J. Approach bias modification in alcohol dependence: do clinical effects replicate and for whom does it work best? Dev Cognit Neurosci (2013) 4:38–51. doi: 10.1016/j.dcn.2012.11.002
27. Rinck M, Wiers RW, Becker ES, Lindenmeyer J. Relapse prevention in abstinent alcoholics by cognitive bias modification: clinical effects of combining approach bias modification and attention bias modification. J Consult Clin Psychol (2018) 86(12):1005–16. doi: 10.1037/ccp0000321
28. Wiers RW, Eberl C, Rinck M, Becker ES, Lindenmeyer J. Retraining automatic action tendencies changes alcoholic patients’ approach bias for alcohol and improves treatment outcome. Psychol Sci (2011) 22(4):490–7. doi: 10.1177/0956797611400615
29. Clerkin EM, Magee JC, Wells TT, Beard C, Barnett NP. Randomized controlled trial of attention bias modification in a racially diverse, socially anxious, alcohol dependent sample. Behav Res Ther (2016) 87:58–69. doi: 10.1016/j.brat.2016.08.010
30. den Uyl TE, Gladwin TE, Lindenmeyer J, Wiers RW. A clinical trial with combined transcranial direct current stimulation and attentional bias modification in alcohol-dependent patients. Alcohol Clin Exp Res (2018) 42(10):1961–9. doi: 10.1111/acer.13841
31. Schoenmakers TM, de Bruin M, Lux IF, Goertz AG, Van Kerkhof DH, Wiers RW. Clinical effectiveness of attentional bias modification training in abstinent alcoholic patients. Drug Alcohol Depend (2010) 109(1-3):30–6. doi: 10.1016/j.drugalcdep.2009.11.022
32. Boendermaker WJ, Sanchez Maceiras S, Boffo M, Wiers RW. Attentional bias modification with serious game elements: evaluating the shots game. JMIR Serious Games (2016) 4(2):e20. doi: 10.2196/games.6464
33. Cox WM, Fadardi JS, Hosier SG, Pothos EM. Differential effects and temporal course of attentional and motivational training on excessive drinking. Exp Clin Psychopharmacol (2015) 23(6):445–54. doi: 10.1037/pha0000038
34. Fadardi JS, Cox WM. Reversing the sequence: reducing alcohol consumption by overcoming alcohol attentional bias. Drug Alcohol Depend (2009) 101(3):137–45. doi: 10.1016/j.drugalcdep.2008.11.015
35. Field M, Eastwood B. Experimental manipulation of attentional bias increases the motivation to drink alcohol. Psychopharmacol (Berl) (2005) 183(3):350–7. doi: 10.1007/s00213-005-0202-5
36. Langbridge JE, Jones RD, Canales JJ. A neurophysiological and behavioral assessment of interventions targeting attention bias and sense of control in binge drinking. Front Hum Neurosci (2018) 12:538. doi: 10.3389/fnhum.2018.00538
37. Lee S, Lee J-H. The effect of automatic attentional bias modification on alcohol ambivalence. Addictive Behav (2015), 46: 58–64. doi: 10.1016/j.addbeh.2015.03.01025800362
38. Luehring-Jones P, Louis C, Dennis-Tiwary TA, Erblich J. A single session of attentional bias modification reduces alcohol craving and implicit measures of alcohol bias in young adult drinkers. Alcohol Clin Exp Res (2017) 41(12): 2207–16. doi: 10.1111/acer.13520
39. McGeary JE, Meadows SP, Amir N, Gibb BE. Computer-delivered, home-based, attentional retraining reduces drinking behavior in heavy drinkers. Psychol Addict Behav (2014) 28(2):559–62. doi: 10.1037/a0036086
40. Schoenmakers T, Wiers RW, Jones BT, Bruce G, Jansen AT. Attentional re-training decreases attentional bias in heavy drinkers without generalization. Addiction (2007) 102(3):399–405. doi: 10.1111/j.1360-0443.2006.01718.x
41. Wiers RW, Houben K, Fadardi JS, van Beek P, Rhemtulla M, Cox WM. Alcohol cognitive bias modification training for problem drinkers over the web. Addict Behav (2015c) 40:21–6. doi: 10.1016/j.addbeh.2014.08.010
42. den Uyl TE, Gladwin TE, Rinck M, Lindenmeyer J, Wiers RW. A clinical trial with combined transcranial direct current stimulation and alcohol approach bias retraining. Addict Biol (2017) 22(6):1632–40. doi: 10.1111/adb.12463
43. Loijen A, Rinck M, Walvoort SJW, Kessels RPC, Becker ES, Egger JIM. Modification of automatic alcohol-approach tendencies in alcohol-dependent patients with mild or major neurocognitive disorder. Alcohol Clin Exp Res (2018) 42(1):153–61. doi: 10.1111/acer.13529
44. Manning V, Staiger PK, Hall K, Garfield JB, Flaks G, Leung D, et al. Cognitive bias modification training during inpatient alcohol detoxification reduces early relapse: a randomized controlled trial. Alcohol Clin Exp Res (2016) 40(9):2011–9. doi: 10.1111/acer.13163
45. Gladwin TE, Rinck M, Eberl C, Becker ES, Lindenmeyer J, Wiers RW. Mediation of cognitive bias modification for alcohol addiction via stimulus-specific alcohol avoidance association. Alcohol Clin Exp Res (2015) 39(1):101–7. doi: 10.1111/acer.12602
46. Wiers CE, Stelzel C, Gladwin TE, Park SQ, Pawelczack S, Gawron CK, et al. Effects of cognitive bias modification training on neural alcohol cue reactivity in alcohol dependence. Am J Psychiatry (2015b) 172(4):335–43. doi: 10.1176/appi.ajp.2014.13111495
47. Wiers CE, Ludwig VU, Gladwin TE, Park SQ, Heinz A, Wiers RW, et al. Effects of cognitive bias modification training on neural signatures of alcohol approach tendencies in male alcohol-dependent patients. Addict Biol (2015a) 20(5):990–9. doi: 10.1111/adb.12221
48. Claus ED, Klimaj SD, Chavez R, Martinez AD, Clark VPA randomized trial of combined tDCS over right inferior frontal cortex and cognitive bias modification: Null effects on drinking and alcohol approach bias. Alcohol Clin Exp Res (2019) 43(7):1591–9. doi: 10.1111/acer.14111
49. den Uyl TE, Gladwin TE, Wiers RW. Electrophysiological and behavioral effects of combined transcranial direct current stimulation and alcohol approach bias retraining in hazardous drinkers. Alcohol Clin Exp Res (2016) 40(10):2124–33. doi: 10.1111/acer.13171
50. Di Lemma LCG, Field M. Cue avoidance training and inhibitory control training for the reduction of alcohol consumption: a comparison of effectiveness and investigation of their mechanisms of action. Psychopharmacol (Berl) (2017) 234(16):2489–98. doi: 10.1007/s00213-017-4639-0
51. Hahn AM, Simons RM, Simons JS, Wiers RW, Welker LE. Can cognitive bias modification simultaneously target two behaviors? Approach bias retraining for alcohol and condom use. Clin Psychol Sci (2019). 7(5):1078–93. doi: 10.1177/2167702619834570
52. Leeman RF, Nogueira C, Wiers RW, Cousijn J, Serafini K, DeMartini KS, et al. A test of multisession automatic action tendency retraining to reduce alcohol consumption among young adults in the context of a human laboratory paradigm. Alcoholism-Clin Exp Res (2018) 42(4):803–14. doi: 10.1111/acer.13613
53. Lindgren KP, Wiers RW, Teachman BA, Gasser ML, Westgate EC, Cousijn J, et al. Attempted training of alcohol approach and drinking identity associations in us undergraduate drinkers: null results from two studies. PloS One (2015) 10(8):e0134642. doi: 10.1371/journal.pone.0134642
54. Sharbanee JM, Hu L, Stritzke WGK, Wiers RW, Rinck M, MacLeod C. The effect of approach/avoidance training on alcohol consumption is mediated by change in alcohol action tendency. PloS One (2014) 9(1):e85855. doi: 10.1371/journal.pone.0085855
55. Wiers RW, Rinck M, Kordts R, Houben K, Strack F. Retraining automatic action-tendencies to approach alcohol in hazardous drinkers. Addiction (2010) 105(2):279–87. doi: 10.1111/j.1360-0443.2009.02775
56. Bowley CFC, Hegarty B, Johnstone S, Smith J, Kelly P, Rushby J. The effects of inhibitory control training on alcohol consumption, implicit alcohol-related cognitions and brain electrical activity. Int J Psychophysiol (2013) 89(3):342–8. doi: 10.1016/j.ijpsycho.2013.04.011
57. Houben KNC, Wiers RW, Jansen A. Resisting temptation: decreasing alcohol-related affect and drinking behavior by training response inhibition. Drug Alcohol Depend (2011) 116(1-3):132–6. doi: 10.1016/j.drugalcdep.2010.12.011
58. Houben KHRC, Nederkoorn C, Jansen A. Beer a no-go: learning to stop responding to alcohol cues reduces alcohol intake via reduced affective associations rather than increased response inhibition. Addiction (2012) 107(7):1280–7. doi: 10.1111/j.1360-0443.2012.03827.x
59. Jones A, Field M. The effects of cue-specific inhibition training on alcohol consumption in heavy social drinkers. Exp Clin Psychopharmacol (2013) 21(1):8. doi: 10.1037/a0030683
60. Jones A, McGrath E, Robinson E, Houben K, Nederkoorn C, Field M. A randomized controlled trial of inhibitory control training for the reduction of alcohol consumption in problem drinkers. J Consulting Clin Psychol (2018) 86(12):991–1004. doi: 10.1037/ccp0000312
61. Kilwein TM, Bernhardt KA, Stryker ML, Looby A. Decreased alcohol consumption after pairing alcohol-related cues with an inhibitory response. J Subst Use (2018) 23(2): 154–61. doi: 10.1080/14659891.2017.1378736
62. Liu Q, Hu L, Smith JL, Mewton LR. Can inhibitory training produce reductions in drinking? Evaluating the influence of the control condition. J Stud Alcohol Drugs (2019) 80(1):96–101. doi: 10.15288/jsad.2019.80.96
63. Smith JL, Dash NJ, Johnstone SJ, Houben K, Field M. Current forms of inhibitory training produce no greater reduction in drinking than simple assessment: A preliminary study. Drug Alcohol Depend (2017) 173:47–58. doi: 10.1016/j.drugalcdep.2016.12.018
64. Strickland JC, Hill JC, Stoops WW, Rush CR. Feasibility, acceptability, and initial efficacy of delivering alcohol use cognitive interventions via crowdsourcing. Alcoholism-Clin Exp Res (2019) 43(5):888–99. doi: 10.1111/acer.13987
65. Higgins JP, Altman DG, Gøtzsche PC, Jüni P, Moher D, Oxman AD, et al. The Cochrane Collaboration’s tool for assessing risk of bias in randomised trials. BMJ (2011) 343:d5928. doi: 10.1136/bmj.d5928
66. Cristea IA, Kok RN, Cuijpers P. Persistent Double Standards in Evaluating the Effectiveness of Cognitive Bias Modification: A Commentary on Wiers (2018). J Stud Alcohol Drugs (2018) 79(3):344–5. doi: 10.15288/jsad.2018.79.344
67. Verdejo-Garcia A. Cognitive training for substance use disorders: neuroscientific mechanisms. Neurosci Biobehav Rev (2016) 68:270–81. doi: 10.1016/j.neubiorev.2016.05.018
68. Schachar R, Logan GD, Robaey P, Chen S, Ickowicz A, Barr C. Restraint and cancellation: multiple inhibition deficits in attention deficit hyperactivity disorder. J Abnormal Child Psychol (2007) 35(2):229–38. doi: 10.1007/s10802-006-9075-2
69. Verbruggen F, Logan GD. Automatic and controlled response inhibition: associative learning in the go/no-go and stop-signal paradigms. J Exp Psychol: Gen (2008) 137(4):649. doi: 10.1037/a0013170
70. Veling H, Holland RW, van Knippenberg A. When approach motivation and behavioral inhibition collide: behavior regulation through stimulus devaluation. J Exp Soc Psychol (2008) 44(4):1013–9. doi: 10.1016/j.jesp.2008.03.004
71. Babor TF, Higgins-Biddle J, Saunders J, Monteiro M. (2001). The alcohol use disorders identification test (AUDIT). Guidelines for use in primary care.
72. Gladwin TE, Wiers CE, Wiers RW. Cognitive neuroscience of cognitive retraining for addiction medicine: from mediating mechanisms to questions of efficacy. Prog Brain Res (2016) 224:323–44. doi: 10.1016/bs.pbr.2015.07.021
73. Copersino ML. Cognitive Mechanisms and Therapeutic Targets of Addiction. Curr Opin Behav Sci (2017) 13:91–8. doi: 10.1016/j.cobeha.2016.11.005
74. Mogoaşe C, David D, Koster EH. Clinical efficacy of attentional bias modification procedures: an updated meta-analysis. J Clin Psychol (2014) 70(12):1133–57. doi: 10.1002/jclp.22081
75. Heitmann J, Bennik EC, van Hemel-Ruiter ME, de Jong PJ. The effectiveness of attentional bias modification for substance use disorder symptoms in adults: a systematic review. Systematic Rev (2018) 7(1):160. doi: 10.1186/s13643-018-0822-6
Keywords: alcohol use disorder, cognitive bias modification, approach bias, attentional bias, inhibition, alcohol-specific computerized intervention
Citation: Batschelet HM, Stein M, Tschuemperlin RM, Soravia LM and Moggi F (2020) Alcohol-Specific Computerized Interventions to Alter Cognitive Biases: A Systematic Review of Effects on Experimental Tasks, Drinking Behavior, and Neuronal Activation. Front. Psychiatry 10:871. doi: 10.3389/fpsyt.2019.00871
Received: 27 August 2019; Accepted: 05 November 2019;
Published: 09 January 2020.
Edited by:
Marc N. Potenza, Yale University, United StatesReviewed by:
Victoria Manning, Monash University, AustraliaCopyright © 2020 Batschelet, Stein, Tschuemperlin, Soravia and Moggi. This is an open-access article distributed under the terms of the Creative Commons Attribution License (CC BY). The use, distribution or reproduction in other forums is permitted, provided the original author(s) and the copyright owner(s) are credited and that the original publication in this journal is cited, in accordance with accepted academic practice. No use, distribution or reproduction is permitted which does not comply with these terms.
*Correspondence: Maria Stein, bWFyaWEuc3RlaW5AdXBkLnVuaWJlLmNo
†These authors share first authorship
Disclaimer: All claims expressed in this article are solely those of the authors and do not necessarily represent those of their affiliated organizations, or those of the publisher, the editors and the reviewers. Any product that may be evaluated in this article or claim that may be made by its manufacturer is not guaranteed or endorsed by the publisher.
Research integrity at Frontiers
Learn more about the work of our research integrity team to safeguard the quality of each article we publish.