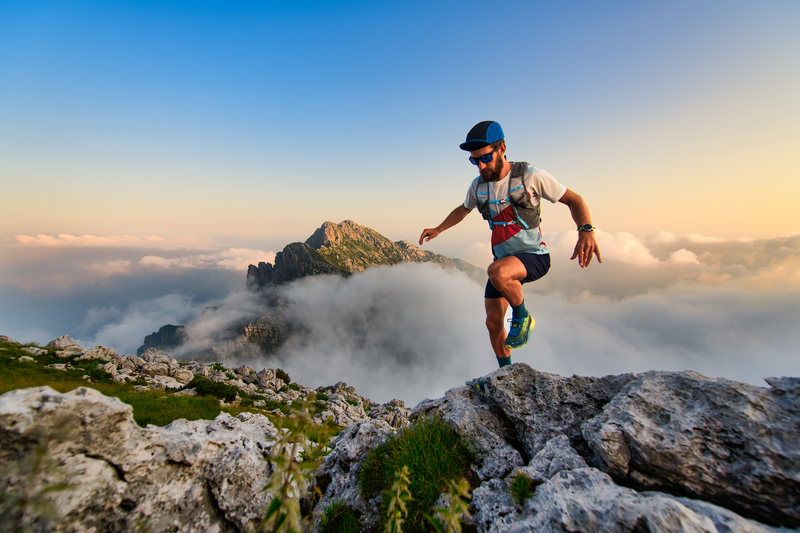
94% of researchers rate our articles as excellent or good
Learn more about the work of our research integrity team to safeguard the quality of each article we publish.
Find out more
ORIGINAL RESEARCH article
Front. Psychiatry , 05 September 2019
Sec. Addictive Disorders
Volume 10 - 2019 | https://doi.org/10.3389/fpsyt.2019.00624
This article is part of the Research Topic Brain and Cognition for Addiction Medicine: From Prevention to Recovery View all 32 articles
Conscious attempts to regulate alcohol and drug use are often undermined by automatic attention and arousal processes that are activated in the context of salient cues. Response to these cues involves body and brain signals that are linked via dynamic feedback loops, yet no studies have targeted the cardiovascular system as a potential conduit to alter automatic neural processes that maintain cue salience. This proof-of-concept study examined within-person changes in neural response to parallel but unique sets of visual alcohol-related cues at two points in time: prior to versus following a brief behavioral intervention. The active intervention was resonance breathing, a rhythmical breathing task paced at 0.1 Hz (6 breaths per minute) that helps normalize neurocardiac feedback. The control intervention was a low-demand cognitive task. Functional magnetic resonance imaging (fMRI) was used to assess changes in brain response to the cues presented before (A1) and after (A2) the intervention in 41 emerging adult men and women with varying drinking behaviors. The resonance breathing group exhibited significantly less activation to A2 cues compared with A1 cues in left inferior and superior lateral occipital cortices, right inferior lateral occipital cortex, bilateral occipital pole, and temporal occipital fusiform cortices. This group also showed significantly greater activation to A2 cues compared with A1 cues in medial prefrontal, anterior and posterior cingulate, and precuneus cortices, paracingulate, and lingual gyri. The control group showed no significant changes. Thus, following resonance breathing, activation in brain regions involved in visual processing of cues was reduced, while activation in brain areas implicated in behavioral control, internally directed cognition, and brain–body integration was increased. These findings provide preliminary evidence that manipulation of the cardiovascular system with resonance breathing alters neural activation in a manner theoretically consistent with a dampening of automatic sensory input and strengthening of higher-level cognitive processing.
Moment-to-moment changes in internal states (e.g., cognition, emotion, visceral processes, moods) and environments (e.g., cues, persons) influence decisions to use alcohol and other drugs (1). These dynamic, intra-individual change processes derive from the body’s ability to collect and relay information to the brain about the environment (afferent neural traffic), as well as from the brain’s ability to integrate this information and generate a behavioral response (efferent neural traffic). In other words, behavior is influenced by both body and brain signals that are linked via reflexive and predictive bidirectional feedback (2, 3).
In the case of the cardiovascular system, this feedback loop (Figure 1) has been extensively documented in terms of its neurophysiology and functional anatomy in rodent and primate models [e.g., (4, 5)]; parallel functional anatomy emerged in a meta-analysis of human neuroimaging studies (6). The loop maintains signaling between the brain and heart via the vagus and sympathetic nerves, baroreceptors located on the aortic arch, carotid artery, and other vessel walls, and a network of brain regions referred to as the central autonomic network (4). These bodies of literature reveal how the brain elicits cardiovascular signals that promote arousal (e.g., increasing heart rate and blood pressure) that, in turn, prepare the organism for goal-directed behavior to respond to in-the-moment demands. Through this loop, feedback from the heart and vasculature is integrated with other autonomic information and relayed to forebrain structures that mediate cognitive and emotional experience (7–9). Consideration of cardiovascular processes as embedded components of affect and cognition implies that these processes contribute to motivated human behavior, including behavioral flexibility toward alcohol and other drugs (10–12). This is important because several non-invasive, low-cost behavioral interventions that help normalize cardiovascular functioning have demonstrated efficacy across various mental and physical health conditions (13–20).
Figure 1 Schematic overview of the neurocardiac feedback loop. Efferent information (blue arrows) emanates from cortical, subcortical, and brain stem structures of the central-autonomic network and flows to the sinoatrial (SA) node of the heart via the sympathetic and parasympathetic branches of the autonomic nervous system. Afferent information (red arrows) from the heart and blood vessels is conveyed back to the brain via baroreceptors located mainly in the walls of the aortic and carotid arteries. Afferent signals enter the brain (shaded in green) via the nucleus tractus solitarius in the brain stem and are integrated with other sensory, cognitive, and affective information as it ascends to cortical regions, including the medial frontal, cingulate, and insular cortices.
Two compelling qualities of the neurocardiac feedback loop for intervention development are its plasticity and responsivity to relatively simple behavioral interventions. Afferent stream activation of the neurocardiac feedback loop can be accomplished by manipulating peripheral functions, such as respiration and muscle flexion (21–24). Breathing paced at 6 breaths per minute (0.1 Hz) is slower and more rhythmical than typical breathing (12–20 breaths per minute). It creates resonance within the cardiovascular system by synchronizing cardiac oscillations driven by respiratory sinus arrhythmia (i.e., the phenomena of heart rate acceleration with inhalation and deceleration with exhalation) with cardiac oscillations driven by the baroreflex, which links heart rate acceleration/deceleration to corresponding changes in the blood pressure (21, 25). As shown in Figure 2, breathing at this frequency lowers systolic blood pressure, increases variability in the time intervals between R-spikes of the electrocardiogram (ECG) (i.e., heart rate variability), generates large oscillations in pulse transit time (i.e., vascular tone variability), and increases the sensitivity of heart rate to changes in blood pressure (i.e., baroreflex gain) (12, 21). A recent meta-analysis found that clinical interventions involving paced breathing at a resonance frequency of the cardiovascular system resulted in large effect size reductions in anxiety and stress (26). Preliminary evidence also suggested paced breathing may reduce craving for appetitive substances (27).
Figure 2 Physiological data from one representative individual collected during a 5-min baseline task (normal breathing) and a 5-min resonance breathing task. Resonance breathing elicited instantaneous changes in respiration, heart rate, pulse transit time (i.e., vascular tone), systolic arterial pressure, and baroreflex sensitivity such that oscillations were magnified and more rhythmic across all measures. In addition, resonance breathing decreased systolic pressure, improved vascular tone, and increased the sensitivity of the neurocardiac feedback loop (i.e., baroreflex). Adapted from (12). Used with permission.
The brain structures of the central autonomic network that participate in cardiovascular signaling overlap considerably with those that process reward, emotion, and habit formation (28), including medial prefrontal, cingulate, and insular cortices, and amygdala. These structures also figure prominently in current translational models of putative addiction neurocircuitry (29–31), with the brain stem serving as the first point of neural integration of afferent autonomic and somatic signals from the body. Psychophysiological evidence suggests that the neurocardiac feedback loop may participate in substance use behaviors through its contribution to attention capture by stimulating cues, affective modulation, and relay of visceral reactivity to the brain [e.g., (32–36)], but little research has extended these findings to the neural structures that comprise the central autonomic network. Nonetheless, converging lines of evidence suggest that ineffective or maladaptive functioning of this feedback loop can set into motion a cascade of biological events that alter one’s ability to adaptively modulate affect, arousal, and stress response (2, 37, 38).
Neural cue reactivity studies, wherein brain activation is measured while participants are exposed to salient alcohol- or drug-related cues, have received significant attention in the neuroscience and psychology of addiction literatures (39, 40). Cue reactivity studies typically compare within-person differences in brain activation to alcohol or drug cues versus control cues (40). There is substantial evidence that elevated alcohol and drug cue neural reactivity is found in individuals with substance use disorders (40–42). Increased neural (43) and cardiac (44) cue reactivity also has been associated with increased drug craving (2, 37, 38). Experimental evidence suggests that heightened neural (45) and cardiovascular (46) reactivity to alcohol and drug cues is related to high risk substance use in non-clinical populations. Thus, altered reactivity to affective and appetitive stimuli appears to increase the likelihood that individuals will be susceptible to contextual influences on substance use, even following extended periods of abstinence (47, 48). This raises the question of whether behavioral interventions that enhance the efficiency of neurocardiac signaling might be used to alter neurocardiac activation to contextual challenges that promote substance use and relapse (49, 50).
This proof-of-concept study examined whether stimulating the afferent stream of the neurocardiac feedback loop with a 5-min course of resonance breathing can affect subsequent neural activation to visual alcohol cues. In contrast to cue reactivity paradigms that compare neural activation to alcohol versus control cues, this study examined within-person changes in neural response to alcohol cues at two points in time. We compared neural activation with unique sets of alcohol cues viewed prior to versus following the breathing task. Because this is the first study of its kind, there is no empirical literature to guide predictions about brain activation changes when participants are exposed to visual cues following resonance breathing. Based on the anatomy of the central autonomic network (4, 5) and drug cue salience networks (28, 40), we hypothesized that significant changes in activation may be observed in brainstem, medial prefrontal, cingulate, and insular cortices, as well as in the amygdala. We further allowed for the possibility of spreading activation, wherein structures within the central autonomic network that share additional network circuitry with regions outside the central autonomic network (e.g., the mesocorticolimbic circuit, ventral striatum) may exhibit activation changes as well. Significant changes in neural response were not anticipated in the group that viewed alcohol cues before and after completing a low-demand cognitive task.
Forty-nine men and women, ages 18 to 25 years, were recruited at a large, northeast U.S. university and in the surrounding community through advertisements targeting alcohol drinkers. Initial inclusion criteria for all participants assessed via self-report were fluency in English, right-handedness, near 20/20 vision (corrected), and alcohol consumption at least once per month. Exclusion criteria assessed via self-report included: MRI contraindications (e.g., permanent metal in the body, claustrophobia), abnormal hearing, any serious medical condition (e.g., epilepsy, diabetes), cardiovascular problems (e.g., hypertension, heart murmur), current learning disability or attention difficulties, loss of consciousness for longer than 30 min, and, for women, pregnancy. To reduce heterogeneity related to psychiatric comorbidities and poly-substance use, lifetime diagnosis of a bipolar disorder or psychosis (e.g., schizophrenia, schizoaffective disorder), past year psychiatric/psychological treatment, past year cannabis use exceeding four times per month in the past year, other past year illicit drug use more than twice per month, past or current substance use treatment (including Alcoholics Anonymous/Narcotics Anonymous), and substance use during pregnancy on the part of the biological mother also were exclusionary.
Half of the participants were recruited based on meeting the National Institute on Alcohol Abuse and Alcoholism (NIAAA) “low risk” drinking criteria [i.e., no more than 5 drinks per day for men (4 drinks per day for women), no more than 14 drinks per week for men (7 drinks per week for women)], as well as an additional criterion of not binge drinking more than once in the past 6 months. The other half met DSM-IV-TR criteria (51) for alcohol dependence. This proof-of-concept examination of resonance breathing as a neurally active intervention included all participants with the exception that data from eight participants were excluded due to excessive motion in the scanner. The final sample (n = 41) had a mean age of 21.4 (SD = 1.9) years and was racially and ethnically diverse (27% Asian, 27% black/African American, 29% white, 17% other/multiple race; 11% Latino/a); 46% of the participants identified as female.
Potential participants who gave verbal consent completed a telephone screening interview to determine initial eligibility. Eligible participants were asked to abstain from alcohol and drug use (except caffeine and nicotine) for 24 h prior to the experimental session. After screening, they were randomized into the active intervention (i.e., resonance breathing) or the control intervention (i.e., vanilla task), with drinking profiles being approximately equally distributed in both groups.
Upon arrival at the imaging center, participants provided written informed consent, supplied a breath sample to verify zero blood alcohol concentration, and completed a MRI safety screener and self-report questionnaires regarding alcohol use, mood state (Positive and Negative Affect Scale) (52), and stress (Perceived Stress Scale) (53). Basic physiological measures (e.g., temperature, blood pressure, weight) and a urine sample were collected; participants with a positive urine screen for cocaine, methamphetamine, opiates, and/or benzodiazepines (One Step Multi-Drug Screen Test Panel) were excluded. Participants with a positive urine screen for marijuana were asked additional follow-up questions about their drug use, and those with marijuana use exceeding four times per month were excluded. Women were screened for pregnancy using a standard urine dipstick. All participants were trained to use an MRI-compatible response box and to perform their assigned intervention task. Task training lasted approximately 2 min. Participants then were fitted with ECG sensors and a respiration belt and positioned in the scanner.
The overall paradigm (Figure 3A) involved four 5-min tasks: 1) viewing a set of nature picture cues, 2) viewing a set of alcohol picture cues (A1), 3) performing the intervention task, 4) viewing a second, distinct set of alcohol picture cues (A2); a 6-min resting state task was then performed. After each task, participants responded to the question, “How much are you currently craving alcohol right now?” using a track ball on a visual analogue scale (VAS, 43) anchored from “not at all” (0) to “extremely” (100). Stimulus cues were presented using E-Prime software (Psychology Software Tools Inc.). Images were projected onto a screen positioned at the rear of the scanner bore and viewed through a mirror attached to the head coil. A trigger pulse synchronized the start of each task with the E-Prime software. Total scan time was approximately 45 min.
Figure 3 Visual depiction of the study and cue task design. Panel (A) shows the complete study design. Participants first viewed a set of nature picture cues (data not shown). Participants then viewed a set of alcohol picture cues (A1), followed by a 5-min intervention task (active condition: resonance breathing; control condition: vanilla task). They then immediately viewed a second, distinct set of alcohol picture cues (A2). The study ended with a 6-min resting state task (data not shown). Panel (B) shows representative images from the alcohol cue tasks, both of which involved viewing 30 unique images that were presented for 6 s, with 4-s inter-stimulus intervals.
Data from the two alcohol cue tasks (A1, A2) were analyzed in the present study. Each task included 30 unique images that were presented for 6 s with 4-s inter-stimulus intervals (Figure 3B), a design driven by the larger study’s broader goal of characterizing the relationship between cardiovascular and neural reactivity. The alcohol cues were drawn from prior studies in our and others’ laboratories (34, 54, 55). Each participant’s self-reported preferred beverage (i.e., beer, wine, “straight” liquor, or mixed drinks) made up approximately 50% of the images to which they were exposed. Participants were instructed to pay attention to the images and to press a response box button when they saw an image that contained their preferred drink.
Between the A1 and A2 cue sets, participants in the active intervention (resonance breathing) synchronized their breathing with a visual pacer at the rate of 0.1 Hz (i.e., 6 breaths per minute). Compliance to the breathing task was verified via analysis of the respiratory signal. Time series respiratory frequency data were Fourier transformed, and the shape of the spectrum was visually inspected; all participants showed a respiratory peak at 0.1 Hz and spectral characteristics consistent with resonance breathing. Participants in the control intervention group completed a low-demand cognitive “vanilla” task wherein different colored rectangles were presented for 10 s each; they were instructed to silently count the number of blue rectangles (56).
After exiting the scanner, participants were compensated for their time. Those who met the criteria for alcohol dependence were given an informational brochure on alcohol use disorders and treatment options. This study was approved by the university’s institutional review board for the protection of human subjects involved in research.
Imaging data were collected using a 3T Siemens Trio scanner and 12-channel head coil. Standard localizer, anatomical, scout, and field map scans were collected. High-resolution anatomical images were acquired using a T1-weighted MPRAGE protocol with parameters: repetition time (TR) = 1,900 ms, echo time (TE) = 2.51 ms, matrix = 256 × 256 voxels, field-of-view (FOV) = 256 mm, voxel size = 1 × 1 × 1 mm, 176 1-mm sagittal slices (.5 mm gap). Functional blood oxygen level-dependent (BOLD) data were acquired using single-shot gradient echo-planar imaging (EPI) sequences with parameters: TR = 2,000 ms, TE = 25 ms, flip, angle = 90°, matrix = 64 × 64 voxels, FOV = 192 mm, voxel size = 3 × 3 × 3 mm, 35 contiguous 3-mm sagittal slices (1 mm gap). ECG and respiration data were collected using a MRI-compatible BIOPAC acquisition system (Biopac Systems, Goleta, CA) as part of the larger study.
FSL 5.0.9 software was used to conduct image preprocessing and data analysis (FMIRB’s Software Library, https://fsl.fmrib.ox.ac.uk). Non-brain tissue was removed from all anatomical and BOLD images using FSL’s Brain Extraction Tool (BET, 57) by estimating each image’s center of gravity and manually adjusting BET parameters as necessary until an optimal result was obtained. BOLD data were motion-corrected using FSL’s MCFLIRT (58), and the output was reviewed to identify participants with excessive motion during the resting-state scan. Excessive motion was defined conservatively as mean absolute and/or relative displacement greater than .5 mm. A paired t-test was performed to compare mean framewise displacement between the randomized intervention groups. No significant differences were observed in motion between the groups (p > 0.05). BOLD images were segmented into gray matter, white matter (WM), and cerebral spinal fluid (CSF) using FSL’s FAST (59). Probability maps of CSF and WM were derived, and time-series data for these signals were extracted from each participant. These nuisance parameters (i.e., WM, CSF) along with extended head motion parameters were used as covariates in the linear regression models implemented in FSL to decrease the effects of signals-of-no-interest. BOLD data were registered to standard space with a two-step process using FMRIB’s Linear Image Registration Tool (FLIRT) (60). The data were first registered to the T1-weighted anatomical image and then to MNI-152 standard space using 9 degrees-of-freedom and SINC interpolation. All data were visually inspected for gross errors in registration. A high pass temporal filter was set to 50 s, and spatial smoothing was set to a 6-mm full-width at half-maximum Gaussian kernel.
Analyses of the BOLD data from the A1 and A2 cue reactivity tasks were performed using a two-step process. Subject-level effects were calculated using first-level analyses in FSL’s FEAT, and group effects were determined using higher-level analyses. In the first-level analysis, each alcohol image event was modeled and convolved with a double-gamma hemodynamic response function (HRF), and the mean task activation for A1 and A2 was calculated for each participant. In the higher-level analysis stage, two sets of analyses were performed using Randomise, the non-parametric permutation-testing tool implemented in FSL (61). First, one-sample t-tests were conducted to characterize neural activation in each intervention group before (A1) and after (A2) the intervention. Next, to examine intervention effects on neural activation to visual stimuli, paired t-tests with two contrasts were conducted on each intervention group (i.e., resonance breathing, control) separately (61). For each contrast, 5,000 permutations were calculated. One contrast (A1 > A2) was designed to determine brain areas that demonstrated greater activation pre- compared to post-intervention task, and the second contrast (A2 > A1) was designed to determine brain areas that demonstrated greater activation post-intervention compared to pre-intervention task. Threshold-free cluster enhancement was employed (62), and activation was considered significant at p < 0.05 (corrected for multiple comparisons using FSL Randomise).
A repeated-measures mixed model was used to assess the effect of resonance breathing on VAS craving scores. Craving data for one participant was missing due to equipment failure; thus, data from 40 participants were available for analysis. A between-subjects factor of intervention group (resonance breathing, control) and a within-subjects factor of craving scores following A1 and A2, as well as their interaction, were modeled. To examine the relationship between VAS craving scores and brain regions that exhibited significant pre-intervention to post-intervention changes, regions-of-interest (ROIs) were defined by creating 6-mm spheres around the peak voxel of each significant cluster of activation for the A1 > A2 and A2 > A1 contrasts. Mean activation values of these ROIs were extracted for each participant from the subject-level A1 and A2 cope images. Pearson correlations were then used to test the associations between ROI activation and VAS craving scores at A1 and A2. Point biserial correlations were used to examine the relationship of binary drinking status (low-risk = 0, alcohol dependent = 1) to ROI activation at A2 in the resonance breathing group. These analyses were performed using SAS 9.4 software (SAS Institute, Cary, NC, USA).
Both intervention groups exhibited widespread neural activity in response to the visual alcohol cues, including in bilateral posterior parahippocampal gyri, temporal occipital fusiform cortices, lateral occipital cortices (inferior and superior divisions), postcentral gyri, and cerebellum at A1 and A2. The resonance breathing group (n = 22) additionally showed significant activation in bilateral inferior frontal gyri, left insula, left pallidum, left putamen, left amygdala, and left thalamus (A1, A2), and left precentral gyrus (A1). The control group (n = 19) additionally showed significant activation in the right thalamus (A1) and left precentral gyrus (A2). These results are shown in Figure 4.
Figure 4 Significant Neural Activation to Visual Alcohol Cue Sets. One-sample t-tests were used to identify areas of significant neural activation during alcohol cue set viewing. The neural responses of the active intervention (resonance breathing) group are shown in Panels (A) (A1 task, cues viewed prior to the intervention) and (B) (A2 task, cues viewed after the intervention). The neural responses of the control intervention (vanilla task) group are shown in Panels (C) (A1 task, cues viewed prior to the intervention) and (D) (A2 task, cues viewed after the intervention). Axial slices are shown in MNI standard space at z = −6 (first slice) and every fourth subsequent slice. Images are oriented using radiological convention. Areas of significant activation are shown in red.
Participants in the resonance breathing group demonstrated greater activation in response to alcohol cues pre-breathing compared with post-breathing (A1>A2) in left inferior and superior lateral occipital cortices and right inferior lateral occipital cortex, as well as bilateral occipital pole and temporal occipital fusiform cortices. They also demonstrated greater activation post-breathing compared with pre-breathing (A2 > A1) in voxels spanning precuneus cortex, posterior cingulate gyrus, and bilateral lingual gyri, as well as in medial prefrontal cortex (MPFC), paracingulate gyrus, and anterior cingulate cortex (ACC). These results are shown in Table 1 and Figure 5.
Table 1 Anatomical location at peak voxel coordinates in significant clusters of activation in resonance breathing group.
Figure 5 Significant Clusters of Activation in Resonance Breathing Group. Blue-cyan clusters represent regions with greater activation during A1 compared to A2 (A1 > A2), and red-yellow clusters represent regions with greater activation during A2 compared with A1 (A2 > A1). Voxels were thresholded at p < 0.05. Image is shown in MNI standard space at x = −4, y = −66, z = 6, and oriented using radiological convention.
The control group analysis yielded no significant activation in either the A1 > A2 or A2 > A1 contrasts, indicating that there were no statistically significant changes in brain activation in response to visual alcohol cues in the group that performed the control task.
Surveys administered prior to the neuroimaging session revealed that the sample as a whole had low-moderate perceived stress (mean ± standard deviation = 17 ± 6), and positive (mean ± standard deviation = 30.7±9.7) and negative (mean ± standard deviation = 13.9 ± 6.2) affect scores that were similar to those reported from the original general adult normative sample (52). There were no differences in affect or stress between the intervention groups nor between the drinking groups (all p > .05).
Craving was measured in the scanner after exposure to each cue block. The results of a repeated-measures (A1, A2) mixed model indicated that there was a significant main effect of group on craving, but no main effect of task (i.e., from pre- to post-intervention). Participants randomized to the resonance breathing intervention group reported lower levels of in-the-moment craving compared to those randomized to the control intervention group [F(76,1) = 5.76, p = 0.0188; least square mean ± standard error of resonance breathing group = 28.1 ± 4.5 and of control group = 43.7 ± 4.7]. The group–task interaction was not statistically significant, suggesting that changes in subjective reports of craving pre- to post-intervention did not significantly differ between the two groups.
No significant correlations were observed at A1 between VAS craving scores and the ten cluster activation scores in the full sample (r range, −0.24 to 0.09, all p > .05). In addition, there were no significant correlations at A2 between craving scores and the ten cluster activation scores within either group (resonance breathing group r range, −0.32 to 0.28, all p >.05; control group r range, −0.42 to 0.44, all p > .05). Lastly, there were no significant correlations at A2 between drinking status (AUD vs. low risk) and the ten-cluster activation scores in the resonance breathing group (r range, −0.27 to 0.28, all p > .05).
Evidence that visceral afferent signaling influences stimulus processing argues for intervention development aimed at manipulating cardiovascular signals to alter detection and neural processing of affective stimuli (63). The results of the present study provide the first proof-of-concept evidence that a brief behavioral intervention of resonance breathing can significantly alter drinkers’ neural activation to visual alcohol cues. The observed changes in brain activity included both decreases and increases in the activation of distinct brain regions.
In the group that performed resonance breathing between the visual cue tasks, but not in the control group, there was reduced activation in occipital regions from the first set of alcohol cues to the second, different set of alcohol cues. This pattern of results suggests that the breathing intervention prompted a subsequent decrease in visual cortex activation when individuals were confronted with alcohol-related visual stimuli. The specificity of these changes to alcohol-related content is unclear as this proof-of-concept study did not include a cue set of non-alcohol–related images presented before and after the intervention. Indeed, visual cortex activation to many types of images, including faces, is modulated by their emotional and social significance (64–66). Multiple lines of evidence also support the involvement of the visual cortex in appetitive cue processing. Several meta-analyses found that drug users consistently showed increased activation in occipital regions in response to drug-related cues compared to controls, even when non-visual drug-related stimuli were presented (41, 67–69). Increased visual cortex activation has been observed in individuals with behavioral addictions, such as pathological gambling, as well (70–72). Thus, although the literature suggests that the role of the visual cortex in alcohol and drug cue reactivity is not specific, decreased activation in the lateral occipital cortices following resonance breathing would be consistent with decreased perception, representation, and recognition of the images (73) and/or may potentially reflect less attention being directed toward the cues by the amygdala (66) or higher cortical areas (74).
In parallel with reduced visual processing of the cues, we observed increased activation in bilateral medial prefrontal, anterior and posterior cingulate, and precuneus cortices during the second alcohol cue task, only in the resonance breathing group. The ACC and MPFC, as regions of the central autonomic network, bi-directionally influence, and are influenced by, afferent cardiovascular signaling. Resonance breathing increases cardiovascular input to the brain via activation of brainstem nuclei that share connectivity with the ACC and MPFC (4) and are thought to give rise to the visceral experience of emotion (75). Functionally, the ACC is a part of the mesocorticolimbic circuit, which is thought to be involved in conflict monitoring and the regulation of cognitive and emotional processing by integrating input and modulating processing in other regions (76, 77). The MPFC is considered to be part of a cognitive control system in the brain that promotes goal-directed behaviors (78) by using incoming information to predict the most adaptive response based on past experience (79).
Hypothetically, increased activation of MPFC and ACC in response to alcohol cues following the breathing intervention would be consistent with heightened internal monitoring of cognitive-emotional state and enhanced cognitive control. At the same time, some studies have identified these regions as sites of heightened reactivity to alcohol and other drug cues (42), and heightened reactivity in these regions has been related to post-treatment drinking and relapse, although the results in this area have not been consistent (80). Thus, it is unclear whether or under what circumstances these and other brain regions accentuate or restrain cue-elicited craving and substance use behaviors. Evidence for individual differences in brain areas most reactive to appetitive cues (42) and inconsistencies in replication add further complication to interpretation. More nuanced examination of intra-individual changes in neural activation across brain areas, and perhaps also across simultaneously operating psychological and physiological systems involved in motivated behavior, are needed.
The posterior cingulate cortex (PCC) and the precuneus showed increased activation to visual alcohol cues following the breathing intervention, but not the control task. Both of these regions are considered core nodes of the default mode network, a functional brain network involved in self-referential thought and mind-wandering (81) that shows preserved connectivity during cognitive load (82). The lingual gyrus, a brain region involved in visual encoding and higher-order analysis of complex visual stimuli (83), also showed increased activation only in the resonance breathing group. This gyrus has been implicated in spontaneous thought and often co-activates with the default mode network (84). Whether increased activation in these regions potentially plays a role in promoting self-regulation in response to alcohol or other affectively valenced cues is unknown, but warrants further investigation. One possibility is that following resonance breathing the brain reverts to its “baseline” resting state (85) for some amount of time despite activation by salient cues, rather than transitioning to a heightened state of arousal.
We did not observe acute changes in self-reported craving levels in the resonance breathing group following the second presentation of alcohol cues (absence of significant cue task by group interaction), nor were craving levels related to brain clusters of activation in response to cues at A1 or A2. Several factors likely contributed to these null findings. Randomization into resonance breathing and control groups in the present study did not result in equivalent mean craving rating scores; the resonance breathing group reported significantly lower craving levels throughout the study. Failures of randomization in small samples are common (86), and future studies may benefit from selecting participants with high levels of self-reported craving and/or matching on craving levels across intervention groups. It may also be that the brief 5-min duration of resonance breathing did not affect conscious self-estimates of craving in the present sample, or that resonance breathing works in a way that affects a different pathway, such as the operation of cue salience (50), rather than consciously experienced craving levels. The present data are limited in not speaking to these alternative speculations.
If replicated and extended, the current findings that a brief, 5-min bout of resonance breathing changed neural activation in brain areas implicated in affective and appetitive stimulus processing could have clinical implications for individuals who show elevated neural reactivity in response to appetitive cues (44). Resonance breathing is the active mechanism of heart rate variability biofeedback, an empirically supported behavioral intervention for disorders with core features of affective and emotional dysregulation (13, 14, 17, 26) including alcohol use disorders (44, 87, 88). Emerging evidence suggests that heart rate variability biofeedback and paced breathing interventions reduce self-reported craving for alcohol and other appetitive stimuli, such as food (27, 89). While standard heart rate variability biofeedback delivery protocols include five to ten 1-h sessions and home practice (90, 91), resonance breathing itself produces immediate physiological effects (see Figure 2). This proof-of-concept study was novel in examining whether resonance breathing also elicits immediate neural effects. The findings provide an initial step in validating resonance breathing as an in-the-moment behavioral tool that potentially could be used ad lib in the natural environment to alter neural activation, both before and during contexts of heightened risk for substance use. Accessible smart phone applications are available to self-administer resonance breathing and HRV biofeedback, suggesting promise for a scalable intervention tool if future research is successful in demonstrating that such effects are linked to reduced alcohol and drug use behaviors.
As a proof-of-concept study, these findings should be interpreted with caution and used for the generation of future hypotheses regarding the effects of resonance breathing on neural activation to alcohol-related visual stimuli, behavioral correlates of alcohol use such as in-the-moment craving, and actual use behaviors. Importantly, the changes observed in neural activation to the cues following the resonance breathing intervention should not be considered specific to alcohol-cue reactivity, as this study did not include a comparison condition of matched, non-alcohol cues presented before and after the intervention. This study also was limited in not being sufficiently powered to examine sensitively the relation of individual differences in alcohol use behaviors to changes in neural activation following resonance breathing. We note that the cue presentation paradigm of the present study was designed in line with the goal of better understanding afferent cardiovascular input to neural reactivity and thus was not typical of those used in many other fMRI studies of cue reactivity. A recent meta-analysis found that cue paradigm and type did not significantly influence neural response patterns associated with cue reactivity however (28), suggesting the fMRI assessment of neural activation is robust to multiple cue presentation approaches. Future studies should include larger samples to link current and chronic substance use behaviors to cue reactivity, and a design that counterbalances and compares neural response to alcohol-related and non-alcoholic beverage cues. Specificity may be addressed also by comparisons to non-alcohol or drug-related, yet positive or negative affectively valenced, visual cue sets.
In summary, this study presents preliminary evidence that individuals ranging in drinking behaviors from low-risk to alcohol-dependent may be less visually engaged by alcohol cues and initiate greater top-down cognitive processing of cues following resonance breathing. This is consistent with the broader literature on resonance breathing that shows it normalizes neurocardiac feedback and improves autonomic nervous system regulation (25). Moreover, it points to a potential neural foundation for the effects of resonance breathing and adds to the scientific premise for the use of heart rate variability biofeedback as an intervention for brain-based mental and physical health conditions. More highly powered studies are needed to replicate and extend these neural activation results. Critical next steps are to understand how the cardiovascular and neural changes elicited by resonance breathing are linked to changes in the subjective experience of craving and alcohol use behaviors.
This study was carried out in accordance with the recommendations of the National Institutes of Health guidelines for ethical treatment of human subjects with written informed consent from all subjects. All subjects gave written informed consent in accordance with the Declaration of Helsinki. The protocol was approved by the Rutgers University Arts and Sciences Institutional Review Board for the Protection of Human Subjects Involved in Research.
MB and JB designed the study. MB and LL wrote the first draft of the article. All authors contributed to writing sections of the manuscript. LL and SU collected all data. LL and SU post-processed the imaging data. LL, SU, and SG performed the data analyses. All authors read and approved the final manuscript.
This work was supported in part by R21AA022748 and K24AA021778 (MEB), K02AA025123 (JFB), and F31AA027147 (LML) from the US National Institutes of Health.
The authors declare that the research was conducted in the absence of any commercial or financial relationships that could be construed as a potential conflict of interest.
We acknowledge Dr. Evgeny Vaschillo, an originator of heart rate variability biofeedback and the study of cardiovascular resonance. We also thank the staff and students of the Cardiac Neuroscience Laboratory for their assistance in screening and testing participants.
1. Shiffman S, Stone AA, Hufford MR. Ecological momentary assessment. AnnRev Clin Psychol (2008) 4:1–32. doi: 10.1146/annurev.clinpsy.3.022806.091415
3. Gianaros PJ, Jennings JR. Host in the machine: a neurobiological perspective on psychological stress and cardiovascular disease. Am Psychol (2018) 73:1031–44. doi: 10.1037/amp0000232
4. Benarroch EE. The central autonomic network. In: Low PA, editor. Clinical Autonomic Disorders. 2nd ed Lippincott-Raven (1997). p. 17–23.
5. Goldstein DS. The autonomic nervous system in health and disease. New York: Marcel Dekker, Inc. (2001).
6. Beissner F, Meissner K, Bar KJ, Napadow V. The autonomic brain: an activation likelihood estimation meta-analysis for central processing of autonomic function. J Neurosci (2013) 33:10503–11. doi: 10.1523/JNEUROSCI.1103-13.2013
7. Lacey BC, Lacey JI. Some autonomic-central nervous system interrelationships. In: Blank P, editor. Physiological Correlations of Emotion. Academic Press (1970). p. 205–27. doi: 10.1016/B978-0-12-102850-3.50016-5
8. Iversen S, Iversen L, Saper CB. The autonomic nervous system and the hypothalamus. In: Kandel ER, Schwartz CE, Jessell TM, editors. Principle of Neural Science. McGraw-Hill (2000). p. 960–81.
9. Critchley HD. Neural mechanisms of autonomic, affective, and cognitive integration. J Compar Neurol (2005) 493:154–66. doi: 10.1002/cne.20749
10. Bates ME, Buckman JF. Emotional dysregulation in the moment: why some college students may not mature out of hazardous alcohol and drug use. In: White HR, Rabiner DL, editors. College drinking and drug use. The Guilford Press (2011). p. 83–101.
11. Bates ME, Buckman JF. Integrating body and brain systems in addiction neuroscience. In: Miller P, editor. Biological Research on Addiction: Comprehensive Addictive Behaviors and Disorders. Elsevier (2013). doi: 10.1016/B978-0-12-398335-0.00020-0
12. Buckman JF, Vaschillo EG, Fonoberova M, Mezic I, Bates ME. The translational value of psychophysiology methods and mechanisms: multilevel, dynamic, personalized. J Stud Alcohol Drugs (2018) 79:229–38. doi: 10.15288/jsad.2018.79.229
13. Hassett AL, Radvanski DC, Vaschillo E, Vaschillo B, Sigal LH, Karavidas MK, et al. A pilot study of the efficacy of heart rate variability (HRV) biofeedback in patients with fibromyalgia. Appl Psychophysiol Biofeedback (2007) 32:1–10. doi: 10.1007/s10484-006-9028-0
14. Karavidas MK, Lehrer PM, Vaschillo E, Vaschillo B, Marin H, Buyske S, et al. Preliminary results of an open label study of heart rate variability biofeedback for the treatment of major depression. Appl Psychophysiol Biofeedback (2007) 32:19–30. doi: 10.1007/s10484-006-9029-z
15. Windhorst U. Muscle proprioceptive feedback and spinal networks. Brain Res Bull (2007) 73:155–202. doi: 10.1016/j.brainresbull.2007.03.010
16. Reiner R. Integrating a portable biofeedback device into clinical practice for patients with anxiety disorders: results of a pilot study. Appl Psychophysiol Biofeedback (2008) 33:55–61. doi: 10.1007/s10484-007-9046-6
17. Siepmann M, Aykac V, Unterdorfer J, Petrowski K, Mueck-Weymann M. A pilot study on the effects of heart rate variability biofeedback in patients with depression and in healthy subjects. Appl Psychophysiol Biofeedback (2008) 33:195–201. doi: 10.1007/s10484-008-9064-z
18. Zucker TL, Samuelson KW, Muench F, Greenberg MA, Gevirtz RN. The effects of respiratory sinus arrhythmia biofeedback on heart rate variability and posttraumatic stress disorder symptoms: a pilot study. Appl Psychophysiol Biofeedback (2009) 34:135–43. doi: 10.1007/s10484-009-9085-2
19. Sutarto AP, Wahab MN, Zin NM. Resonant breathing biofeedback training for stress reduction among manufacturing operators. Int J Occup Saf Ergon (2012) 18:549–61. doi: 10.1080/10803548.2012.11076959
20. Eddie D, Kim C, Bates ME, Lehrer P, Deneke E. A pilot study of brief heart rate variability biofeedback to reduce craving in young adult men receiving inpatient treatment for substance use disorders. Appl Psychophysiol Biofeedback (2014) 39:181–92. doi: 10.1007/s10484-014-9251-z
21. Lehrer PM, Vaschillo E, Vaschillo B, Lu SE, Eckberg DL, Edelberg R, et al. Heart rate variability biofeedback increases baroreflex gain and peak expiratory flow. Psychosomatic Med (2003) 65:796–805. doi: 10.1097/01.PSY.0000089200.81962.19
22. Vaschillo E, Vaschillo B, Lehrer P. Heartbeat synchronizes with respiratory rhythm only under specific circumstances. Chest (2004) 126:1385–6. doi: 10.1016/S0012-3692(15)31329-5
23. Lehrer P, Vaschillo E, Trost Z, France CR. Effects of rhythmical muscle tension at 0.1Hz on cardiovascular resonance and the baroreflex. Biol Psychol (2009) 81:24–30. doi: 10.1016/j.biopsycho.2009.01.003
24. Vaschillo EG, Vaschillo B, Pandina RJ, Bates ME. Resonances in the cardiovascular system caused by rhythmical muscle tension. Psychophysiology (2010) 48:927–36. doi: 10.1111/j.1469-8986.2010.01156.x
25. Vaschillo EG, Vaschillo B, Buckman JF, Pandina RJ, Bates ME. The investigation and clinical significance of resonance in the heart rate and vascular tone baroreflexes. In: Fred A, Filipe J, Gamboa H, editors. Biomedical Engineering Systems and Technologies: Communications in Computer and Information Science. Springer (2011). p. 224–37. doi: 10.1007/978-3-642-18472-7_18
26. Goessl VC, Curtiss JE, Hofmann SG. The effect of heart rate variability biofeedback training on stress and anxiety: a meta-analysis. Psychol Med (2017) 47:2578–86. doi: 10.1017/S0033291717001003
27. Alayan N, Eller L, Bates ME, Carmody DP. Current evidence on heart rate variability biofeedback as a complementary anticraving intervention. J Altern Complement Med (2018) 24:1039–50. doi: 10.1089/acm.2018.0019
28. Noori HR, Cosa Linan A, Spanagel R. Largely overlapping neuronal substrates of reactivity to drug, gambling, food and sexual cues: a comprehensive meta-analysis. Eur Neuropsychopharmacol (2016) 26:1419–30. doi: 10.1016/j.euroneuro.2016.06.013
29. Kelley AE, Berridge KC. The neuroscience of natural rewards: relevance to addictive drugs. J Neurosci (2002) 22:3306–11. doi: 10.1523/JNEUROSCI.22-09-03306.2002
30. Naqvi NH, Bechara A. The hidden island of addiction: the insula. Trends Neurosci (2009) 32:56–67. doi: 10.1016/j.tins.2008.09.009
31. Koob GF, Volkow ND. Neurocircuitry of addiction. Neuropsychopharmacology (2010) 35:217–38. doi: 10.1038/npp.2009.110
32. Sinha R, Fuse T, Aubin LR, O’malley SS. Psychological stress, drug-related cues and cocaine craving. Psychopharmacology (Berl) (2000) 152:140–8. doi: 10.1007/s002130000499
33. Thayer JF, Lane RD. A model of neurovisceral integration in emotion regulation and dysregulation. J Affect Disord (2000) 61:201–16. doi: 10.1016/S0165-0327(00)00338-4
34. Vaschillo EG, Bates ME, Vaschillo B, Lehrer P, Udo T, Mun EY, et al. Heart rate variability response to alcohol, placebo, and emotional picture cue challenges: effects of 0.1-Hz stimulation. Psychophysiology (2008) 45:847–58. doi: 10.1111/j.1469-8986.2008.00673.x
35. Garland EL, Carter K, Ropes K, Howard MO. Thought suppression, impaired regulation of urges, and Addiction-Stroop predict affect-modulated cue-reactivity among alcohol dependent adults. Biol Psychol (2012a) 89:87–93. doi: 10.1016/j.biopsycho.2011.09.010
36. Garland EL, Franken IH, Sheetz JJ, Howard MO. Alcohol attentional bias is associated with autonomic indices of stress-primed alcohol cue-reactivity in alcohol-dependent patients. Exp Clin Psychopharmacol (2012b) 20:225–35. doi: 10.1037/a0027199
37. Porges SW, Doussard-Roosevelt JA, Maita AK. Vagal tone and the physiological regulation of emotion. Monogr Soc Res Child Dev (1994) 59(167–186):250–83. doi: 10.2307/1166144
38. Hugdahl K. Cognitive influences on human autonomic nervous system function. Curr Opin Neurobiol (1996) 6:252–8. doi: 10.1016/S0959-4388(96)80080-8
39. Kuhn S, Gallinat J. Common biology of craving across legal and illegal drugs—a quantitative meta-analysis of cue-reactivity brain response. Eur J Neurosci (2011) 33:1318–26. doi: 10.1111/j.1460-9568.2010.07590.x
40. Jasinska AJ, Stein EA, Kaiser J, Naumer MJ, Yalachkov Y. Factors modulating neural reactivity to drug cues in addiction: a survey of human neuroimaging studies. Neurosci Biobehav Rev (2014) 38:1–16. doi: 10.1016/j.neubiorev.2013.10.013
41. Schacht JP, Anton RF, Myrick H. Functional neuroimaging studies of alcohol cue reactivity: a quantitative meta-analysis and systematic review. Addict Biol (2013) 18:121–33. doi: 10.1111/j.1369-1600.2012.00464.x
42. Hanlon CA, Dowdle LT, Gibson NB, Li X, Hamilton S, Canterberry M, et al. Cortical substrates of cue-reactivity in multiple substance dependent populations: transdiagnostic relevance of the medial prefrontal cortex. Transl Psychiatry (2018) 8:186. doi: 10.1038/s41398-018-0220-9
43. Myrick H, Anton RF, Li X, Henderson S, Drobes D, Voronin K, et al. Differential brain activity in alcoholics and social drinkers to alcohol cues: relationship to craving. Neuropsychopharmacology (2004) 29:393–402. doi: 10.1038/sj.npp.1300295
44. Witteman J, Post H, Tarvainen M, De Bruijn A, Perna Ede S, Ramaekers JG, et al. Cue reactivity and its relation to craving and relapse in alcohol dependence: a combined laboratory and field study. Psychopharmacology (Berl) (2015) 232:3685–96. doi: 10.1007/s00213-015-4027-6
45. Dager AD, Anderson BM, Stevens MC, Pulido C, Rosen R, Jiantonio-Kelly RE, et al. Influence of alcohol use and family history of alcoholism on neural response to alcohol cues in college drinkers. Alcohol Clin Exp Res (2013) 37 Suppl 1:E161–171. doi: 10.1111/j.1530-0277.2012.01879.x
46. Mun EY, Von Eye A, Bates ME, Vaschillo EG. Finding groups using model-based cluster analysis: heterogeneous emotional self-regulatory processes and heavy alcohol use risk. Dev Psychol (2008) 44:481–95. doi: 10.1037/0012-1649.44.2.481
47. Tiffany ST. The role of cognitive factors in reactivity to drug cues. In: Drummond DC, Tiffany ST, editors. Addictive Behaviour: Cue Exposure Theory and Practice. Wiley (1995).
48. Robinson TE, Berridge KC. Review. The incentive sensitization theory of addiction: some current issues. Philos Trans R Soc London— B: Biol Sci (2008) 363:3137–46. doi: 10.1098/rstb.2008.0093
49. Marlatt GA. Section I. theoretical perspectives on relapse: taxonomy of high-risk situations for alcohol relapse: evolution and development of a cognitive-behavioral model. Addiction (1996) 91:S37–49. doi: 10.1111/j.1360-0443.1996.tb02326.x
50. Grusser SM, Wrase J, Klein S, Hermann D, Smolka MN, Ruf M, et al. Cue-induced activation of the striatum and medial prefrontal cortex is associated with subsequent relapse in abstinent alcoholics. Psychopharmacology (2004) 175:296–302. doi: 10.1007/s00213-004-1828-4
51. American Psychiatric Association. Diagnostic and statistical manual of mental disorders. Washington, DC: American Psychiatric Association (2000).
52. Crawford JR, Henry JD. The Positive and Negative Affect Schedule (PANAS): construct validity, measurement properties and normative data in a large non-clinical sample. Br J Clin Psychol (2004) 43:245–65. doi: 10.1348/0144665031752934
53. Cohen S, Karmarck T, Mermelstein R. A global measure of perceived stress. J Health Soc Behav (1983) 24:385–96. doi: 10.2307/2136404
54. Tapert SF, Cheung EH, Brown GG, Frank LR, Paulus MP, Schweinsburg AD, et al. Neural response to alcohol stimuli in adolescents with alcohol use disorder. Arch Gen Psychiatry (2003) 60:727–35. doi: 10.1001/archpsyc.60.7.727
55. Ray S, Hanson C, Hanson SJ, Bates ME. fMRI BOLD response in high-risk college students (Part 1): during exposure to alcohol, marijuana, polydrug and emotional picture cues. Alcohol Alcohol (2010) 45:437–43. doi: 10.1093/alcalc/agq042
56. Jennings JR, Kamarck T, Stewart C, Eddy M, Johnson P. Alternate cardiovascular baseline assessment techniques: vanilla or resting baseline. Psychophysiology (1992) 29, 742-50. doi: 10.1111/j.1469-8986.1992.tb02052.x
57. Smith SM. Fast robust automated brain extraction. Hum Brain Mapp (2002) 17:143–55. doi: 10.1002/hbm.10062
58. Jenkinson M, Bannister P, Brady M, Smith SA. Improved optimization for the robust and accurate linear registration and motion correction of brain images. Neuroimage (2002) 17:825–41. doi: 10.1006/nimg.2002.1132
59. Zhang Y, Brady MS, Smith S. Segmentation of brain MR images through a hidden Markov random field model and the expectation-maximization algorithm. IEEE Transact Med Imaging (2001) 20:45–57:S. doi: 10.1109/42.906424
60. Jenkinson M, Smith S. A global optimisation method for robust affine registration of brain images. Med Image Anal (2001) 5:143–56. doi: 10.1016/S1361-8415(01)00036-6
61. Winkler AM, Ridgway GR, Webster MA, Smith SM, Nichols TE. Permutation inference for the general linear model. Neuroimage (2014) 92:381–97. doi: 10.1016/j.neuroimage.2014.01.060
62. Smith SM, Nichols TE. Threshold-free cluster enhancement: addressing problems of smoothing, threshold dependence and localisation in cluster inference. Neuroimage (2009) 44:83–98. doi: 10.1016/j.neuroimage.2008.03.061
63. Critchley HD, Garfinkel SN. Interactions between visceral afferent signaling and stimulus processing. Front Neurosci (2015) 9:286. doi: 10.3389/fnins.2015.00286
64. Adolphs R. Neural systems for recognizing emotion. Curr Opin Neurobiol (2002) 12:169–77. doi: 10.1016/S0959-4388(02)00301-X
65. Schupp HT, Junghoefer M, Weike AI, Hamm AO. Emotional facilitation of sensory processing in the visual cortex. Psychol Sci (2003) 14:7–13. doi: 10.1111/1467-9280.01411
66. Vuilleumier P, Pourtois G. Distributed and interactive brain mechanisms during emotion face perception: evidence from functional neuroimaging. Neuropsychologia (2007) 45:174–94. doi: 10.1016/j.neuropsychologia.2006.06.003
67. Chase HW, Eickhoff SB, Laird AR, Hogarth L. The neural basis of drug stimulus processing and craving: an activation likelihood estimation meta-analysis. Biol Psychiatry (2011) 70:785–93. doi: 10.1016/j.biopsych.2011.05.025
68. Engelmann JM, Versace F, Robinson JD, Minnix JA, Lam CY, Cui Y, et al. Neural substrates of smoking cue reactivity: a meta-analysis of fMRI studies. Neuroimage (2012) 60:252–62. doi: 10.1016/j.neuroimage.2011.12.024
69. Hanlon CA, Dowdle LT, Naselaris T, Canterberry M, Cortese BM. Visual cortex activation to drug cues: a meta-analysis of functional neuroimaging papers in addiction and substance abuse literature. Drug Alcohol Depend (2014) 143:206–12. doi: 10.1016/j.drugalcdep.2014.07.028
70. Potenza MN, Steinberg MA, Skudlarski P, Fulbright RK, Lacadie CM, Wilber MK, et al. Gambling urges in pathological gambling: a functional magnetic resonance imaging study. Arch Gen Psychiatry (2003) 60:828–36. doi: 10.1001/archpsyc.60.8.828
71. Crockford DN, Goodyear B, Edwards J, Quickfall J, El-Guebaly N. Cue-induced brain activity in pathological gamblers. Biol Psychiatry (2005) 58:787–95. doi: 10.1016/j.biopsych.2005.04.037
72. Goudriaan AE, De Ruiter MB, Van Den Brink W, Oosterlaan J, Veltman DJ. Brain activation patterns associated with cue reactivity and craving in abstinent problem gamblers, heavy smokers and healthy controls: an fMRI study. Addict Biol (2010) 15:491–503. doi: 10.1111/j.1369-1600.2010.00242.x
73. Grill-Spector K, Kourtzi Z, Kanwisher N. The lateral occipital complex and its role in object recognition. Vision Res (2001) 41:1409–22. doi: 10.1016/S0042-6989(01)00073-6
74. Kanwisher N, Wojciulik E. Visual attention: insights from brain imaging. Nat Rev Neurosci (2000) 1:91–100. doi: 10.1038/35039043
75. Venkatraman A, Edlow BL, Immordino-Yang MH. The Brainstem in Emotion: A Review. Front Neuroanat (2017) 11:15. doi: 10.3389/fnana.2017.00015
76. Bush G, Luu P, Posner MI. Cognitive and emotional influences in anterior cingulate cortex. Trends Cogn Sci (2000) 4:215–22. doi: 10.1016/S1364-6613(00)01483-2
77. Botvinick MM, Cohen JD, Carter CS. Conflict monitoring and anterior cingulate cortex: an update. Trends Cogn Sci (2004) 8:539–46. doi: 10.1016/j.tics.2004.10.003
78. Ridderinkhof KR, Ullsperger M, Crone EA, Nieuwenhuis S. The role of the medial frontal cortex in cognitive control. Science (2004) 306:443–7. doi: 10.1126/science.1100301
79. Euston DR, Gruber AJ, Mcnaughton BL. The role of medial prefrontal cortex in memory and decision making. Neuron (2012) 76:1057–70. doi: 10.1016/j.neuron.2012.12.002
80. Courtney KE, Schacht JP, Hutchison K, Roche DJ, Ray LA. Neural substrates of cue reactivity: association with treatment outcomes and relapse. Addict Biol (2016) 21:3–22. doi: 10.1111/adb.12314
81. Buckner RL, Andrews-Hanna JR, Schacter DL. The brain’s default network: anatomy, function, and relevance to disease. Ann N Y Acad Sci (2008) 1124:1–38. doi: 10.1196/annals.1440.011
82. Fransson P, Marrelec G. The precuneus/posterior cingulate cortex plays a pivotal role in the default mode network: evidence from a partial correlation network analysis. Neuroimage (2008) 42:1178–84. doi: 10.1016/j.neuroimage.2008.05.059
83. Machielsen WC, Rombouts SA, Barkhof F, Scheltens P, Witter MP. FMRI of visual encoding: reproducibility of activation. Hum Brain Mapp (2000) 9:156–64. doi: 10.1002/(SICI)1097-0193(200003)9:3<156::AID-HBM4>3.0.CO;2-Q
84. Fox KC, Spreng RN, Ellamil M, Andrews-Hanna JR, Christoff K. The wandering brain: meta-analysis of functional neuroimaging studies of mind-wandering and related spontaneous thought processes. Neuroimage (2015) 111:611–21. doi: 10.1016/j.neuroimage.2015.02.039
85. Gusnard DA, Raichle ME, Raichle ME. Searching for a baseline: functional imaging and the resting human brain. Nat Rev Neurosci (2001) 2:685–94. doi: 10.1038/35094500
86. Kang M, Ragan BG, Park JH. Issues in outcomes research: an overview of randomization techniques for clinical trials. J Athl Train (2008) 43:215–21. doi: 10.4085/1062-6050-43.2.215
87. Penzlin AI, Siepmann T, Illigens BM, Weidner K, Siepmann M. Heart rate variability biofeedback in patients with alcohol dependence: a randomized controlled study. Neuropsychiatr Dis Treat (2015) 11:2619–27. doi: 10.2147/NDT.S84798
88. Penzlin AI, Barlinn K, Illigens BM, Weidner K, Siepmann M, Siepmann T. Effect of short-term heart rate variability biofeedback on long-term abstinence in alcohol dependent patients—a one-year follow-up. BMC Psychiatry (2017) 17:325. doi: 10.1186/s12888-017-1480-2
89. Eddie D, Conway FN, Alayan N, Buckman J, Bates ME. Assessing heart rate variability biofeedback as an adjunct to college recovery housing programs. J Subst Abuse Treat (2018) 92:70–6. doi: 10.1016/j.jsat.2018.06.014
90. Lehrer PM, Vaschillo E, Vaschillo B. Resonant frequency biofeedback training to increase cardiac variability: rationale and manual for training. Appl Psychophysiol Biofeedback (2000) 25:177–91. doi: 10.1023/A:1009554825745
Keywords: alcohol, biofeedback, cardiovascular, neural reactivity, functional magnetic resonance imaging, heart rate variability, respiration, resonance breathing
Citation: Bates ME, Lesnewich LM, Uhouse SG, Gohel S and Buckman JF (2019) Resonance-Paced Breathing Alters Neural Response to Visual Cues: Proof-of-Concept for a Neuroscience-Informed Adjunct to Addiction Treatments. Front. Psychiatry 10:624. doi: 10.3389/fpsyt.2019.00624
Received: 17 January 2019; Accepted: 05 August 2019;
Published: 05 September 2019.
Edited by:
Hamed Ekhtiari, Laureate Institute for Brain Research, United StatesReviewed by:
Kesong Hu, Lake Superior State University, United StatesCopyright © 2019 Bates, Lesnewich, Uhouse, Gohel and Buckman. This is an open-access article distributed under the terms of the Creative Commons Attribution License (CC BY). The use, distribution or reproduction in other forums is permitted, provided the original author(s) and the copyright owner(s) are credited and that the original publication in this journal is cited, in accordance with accepted academic practice. No use, distribution or reproduction is permitted which does not comply with these terms.
*Correspondence: Marsha E. Bates, bWViYXRlc0BzbWl0aGVycy5ydXRnZXJzLmVkdQ==
Disclaimer: All claims expressed in this article are solely those of the authors and do not necessarily represent those of their affiliated organizations, or those of the publisher, the editors and the reviewers. Any product that may be evaluated in this article or claim that may be made by its manufacturer is not guaranteed or endorsed by the publisher.
Research integrity at Frontiers
Learn more about the work of our research integrity team to safeguard the quality of each article we publish.