- 1Department of Computational Physiology, Simula Research Laboratory, Oslo, Norway
- 2NORMENT, Division of Mental Health and Addiction, Oslo University Hospital & Institute of Clinical Medicine, University of Oslo, Oslo, Norway
- 3Department of Neurology, Oslo University Hospital, Oslo, Norway
- 4Department of Neurosciences, University of California, San Diego, La Jolla, CA, United States
- 5Department of Radiology, University of California, San Diego, La Jolla, CA, United States
- 6Martinos Center for Biomedical Imaging, Massachusetts General Hospital, Harvard Medical School, Charlestown, MA, United States
- 7Department of Medical Genetics, Oslo University Hospital, Oslo, Norway
- 8NORMENT, Department of Clinical Science, University of Bergen, Bergen, Norway
- 9Department of Psychology, University of Oslo, Oslo, Norway
- 10Faculty of Medicine and Health Technology, Tampere University, Tampere, Finland
- 11Department of Genetic Epidemiology in Psychiatry, Central Institute of Mental Health, Medical Faculty Mannheim, University of Heidelberg, Mannheim, Germany
- 12Cognitive Neuroscience Department, Donders Institute for Brain, Cognition and Behaviour, Radboud University Medical Centre, Nijmegen, Netherlands
- 13Department of Psychiatry (UPK), University of Basel, Basel, Switzerland
- 14Prof. Dr. Alex. Obregia Clinical Hospital of Psychiatry, Bucharest, Romania
- 15Victor Babes National Institute of Pathology, Bucharest, Romania
- 16Faculty of Medicine, Titu Maiorescu University, Bucharest, Romania
- 17Faculty of Science and Technology, Norwegian University of Life Sciences, Ås, Norway
- 18Department of Physics, University of Oslo, Oslo, Norway
- 19Department of Informatics, University of Oslo, Oslo, Norway
- 20Centre for Computer Science and Informatics Research, University of Hertfordshire, Hatfield, United Kingdom
- 21Institute of Software Engineering and Theoretical Computer Science, Technische Universität zu Berlin, Berlin, Germany
- 22Department of Biosciences, University of Oslo, Oslo, Norway
The brain is the most complex of human organs, and the pathophysiology underlying abnormal brain function in psychiatric disorders is largely unknown. Despite the rapid development of diagnostic tools and treatments in most areas of medicine, our understanding of mental disorders and their treatment has made limited progress during the last decades. While recent advances in genetics and neuroscience have a large potential, the complexity and multidimensionality of the brain processes hinder the discovery of disease mechanisms that would link genetic findings to clinical symptoms and behavior. This applies also to schizophrenia, for which genome-wide association studies have identified a large number of genetic risk loci, spanning hundreds of genes with diverse functionalities. Importantly, the multitude of the associated variants and their prevalence in the healthy population limit the potential of a reductionist functional genetics approach as a stand-alone solution to discover the disease pathology. In this review, we outline the key concepts of a “biophysical psychiatry,” an approach that employs large-scale mechanistic, biophysics-founded computational modelling to increase transdisciplinary understanding of the pathophysiology and strive toward robust predictions. We discuss recent scientific advances that allow a synthesis of previously disparate fields of psychiatry, neurophysiology, functional genomics, and computational modelling to tackle open questions regarding the pathophysiology of heritable mental disorders. We argue that the complexity of the increasing amount of genetic data exceeds the capabilities of classical experimental assays and requires computational approaches. Biophysical psychiatry, based on modelling diseased brain networks using existing and future knowledge of basic genetic, biochemical, and functional properties on a single neuron to a microcircuit level, may allow a leap forward in deriving interpretable biomarkers and move the field toward novel treatment options.
Background: Disparate Progresses in Different Fields of Medicine
Mental illnesses place a large emotional, health, and financial burden on patients, their families, and the society (1). Mental disorders account for about one third of all years lived with disability worldwide (2) with rising prevalence (3), and there is overwhelming evidence of a large mortality gap between individuals with mental illness and the general population (4, 5). At the same time, there have been limited improvements in treatment of affected individuals over the past decades, and there are no diagnostic or prognostic biomarkers for these disorders. Crucially, most mental illnesses are not caused by a single condition but arise from a complex interplay between several internal and environmental factors, and this makes drug development challenging (6). As a result, most major pharmaceutical companies have left this research field due to lack of future potential in standard research approaches (7). These shortcomings have been highlighted by the European Union and the World Health Organization, which have called for additional research to address this problem (www.who.int/mental_health/mhgap/en/).
In other fields of biomedicine, significant progress has been made during the last decades. There has been a continuous reduction in the mortality rate of heart disease since the 1970s, and the mortality rate for several types of cancer has also started to decline (8, 9). A key development in recent years was the completion of the Human Genome Project in 2003 (10), which marked a beginning of a new era (11, 12). Important subfields of biology, such as structural and functional genomics, systems biology, and statistical genetics, have emerged to figure out the implications of the genetic content on living cells and how the genes play together to cause cellular and tissue-level phenotypes as well as human diseases (11).
In parallel, in the wake of efficient computer technology and advanced software, the use of computational models for describing neuronal function has become an important tool for understanding the behavior of single neurons and neuronal networks (cf. 13, 14). Detailed and compartment-specific models of neurons describe the transmembrane currents of different ion-channel families and their voltage-dependent gating, and they are typically based on real (three-dimensionally reconstructed) neuron morphologies, although reduced compartmental models can also be used for computational efficiency or generalizability (15). Moreover, the modelled neurons can be coupled with each other using descriptions of synaptic currents to create biophysical models of large-scale neuronal circuits (16). To standardize the methodology in computational neuroscience and to facilitate reproduction of simulation results, efficient simulation software has been developed, such as NEURON, which is a widely used simulator of single- and multi-compartment neuron models and model networks that flexibly allows the user to define the ion channels and their distribution along the neurites (17). These advances allow analyses of how alterations in a specific ion-channel family can affect the functions of the neural circuit at local and global scales and can thus foster many types of computational studies of heritable (or by other means of genetic origin, i.e., through de novo mutations) mental disorders (18–20).
At present, the role of specific signaling molecules and ion channels in mental disorders is poorly understood, and most of the specific hypotheses regarding, for example, molecular genetic factors and psychiatric disease will have to be considered tentative and preliminary. However, we are now getting to the situation where the computational modelling approaches will be sufficiently developed so that candidate hypotheses can be tested against experiments. Specifically, simulations of brain networks based on biophysically detailed neuron models are now becoming feasible, and molecular effects on the behavior of neurons and networks, and eventually also systems, can be systematically explored with mathematical modelling (14). This could be compared with the progress of weather forecasting technology. The knowledge about meteorological factors affecting the weather was defined already in the 1920s, but the computational power did not become available until the 1960s and 1970s, when weather forecasts, supported by improved parametrization of the underlying physical processes, started to become accurate (21). Today, we can accurately predict the weather for 1 week using advanced computational models that integrate meteorological and topographical data. While we do not yet have a single strong hypothesis of the underlying “microscopic principles” for mental disorders, the possibility to test, falsify, and refine present and future candidate hypotheses for these principles by comparing simulation results with physiological experiments could be a game-changer. Thus, building on genetic data, neuroscience research, biophysical insights, and large-scale computation, we might hope for a breakthrough in the understanding of the molecular and cellular mechanism behind mental disorders.
In the following, we review the relevant advances made in functional genomics, statistical genetics, and cellular neuroscience, and we suggest how these new data can be used in a computational neuroscience approach to understand mental disorders. Basing on the large increase in computation power and availability of quantitative data in molecular and cellular biology over the past years, we predict a wide range of possibilities for this “biophysical psychiatry” approach. Our main focus is on distorted functions of ion channels and disease phenotypes emerging from these alterations. Dysfunctional neuronal excitability represents the branch of mental disorders that is most ready for a prominent computational analysis, but we extend our discussion on other aspects of heritable mental diseases, which will be possible to simulate in the near future. We also discuss the most significant gaps of knowledge and obstacles for this approach.
The Power and Shortcomings of Genome-Wide Association Studies
Genome sequencing data are an ideal source of information for large-population studies for at least three reasons. First, the data obtained are independent of the state (e.g., fatigue, mood, and phase in daily routines) of the subject and, apart from cancerous tissue, generalizable to any cell type in the human body [although data challenging this view do exist (22, 23)]. Second, the data obtained are absolute (no scaling or other preprocessing is needed), although several sources of error exist [see, e.g., Ref. 24)]. Third, it is relatively inexpensive to both take the sample and perform the genome-wide screening, at least if we concentrate on the commonly used single-nucleotide polymorphism (SNP) arrays (25). Consequently, genome-wide association study (GWAS) sample sizes have grown to tens of thousands of subjects, increasing the statistical accuracy of the obtained results.
These advantages have made possible the application of GWAS to a lot of heritable traits and diseases, including mental disorders. Crucially, mental disorders are polygenic phenotypes, that is, no single gene determines the disorder outcome, but the risk of the disorder may depend on as much as hundreds of genetic loci. For example, for schizophrenia, an ever-increasing number of genetic loci are identified: 108 genetic loci were identified using a sample of 37,000 affected individuals and 113,000 controls (26), and the number of identified risk loci was yet larger (145) in the latest GWAS (27). Importantly, the new GWAS results typically replicate the majority of the previous GWAS findings, which is often not the case with the hypothesis-driven candidate gene methods that used to be the standard in the field (28). The large number of identified risk variants associated with mental disorders and their frequent prevalence in healthy population (most of the identified gene variants are common) place a challenge on the functional genomics approach typically applied to variants of heritable diseases. The identified 145 schizophrenia loci typically contain many risk SNPs with variable degrees of linkage disequilibrium with each other. Application of novel biostatistics tools will likely capture more of the heritability of mental disorders and thus further increase these numbers (29–31). Another related challenge is the functional diversity of the identified loci. In schizophrenia, the implicated genes contribute to the immune system (32), neuronal electrogenesis (33, 34), synaptic function and neurotransmission (35), and redox homeostasis (36, 37).
Thus, a systematic functional genomics approach to polygenic mental disorders would have to 1) consider many cellular phenomena that a variant may affect, each of which should be particularly designed to quantify a phenotype in the underlying genetic pathway, 2) test many genetic loci to capture the effects of all risk variants in the considered gene, and 3) perform the experiments with large sample sizes to detect small effects, as expected from common variants. Overcoming these three challenges is beyond the capabilities of the scientific community of today. And if it becomes possible, we would still be left with the question on how the interaction between different variants is involved in inducing disease phenotypes. Although this certainly does not altogether disqualify reductionist methods driven by genomic data, it points us toward alternative approaches that allow making and testing hypotheses on the disease mechanisms of polygenic mental disorders, ideally in a less costly but as standardized and reproducible manner as the functional genomics approach. In the following sections, we will discuss how well-suited biophysically detailed computational modelling is for this purpose.
The Computational Psychiatry Approach
Computational neuroscience builds upon describing of neurons, neuronal circuits, brain areas, or the whole brain by quantitative, computational models. On the single-neuron level, a computational model of a neuron is typically a set of equations that describes its properties based on a solid biophysical foundation—the current balance across the cell membrane and the conduction of ions across the media. Biophysically detailed whole-neuron modelling relies on two scientific breakthroughs in the last century [reviewed in Ref. (38)]. First, the work by Hodgkin and Huxley (39) to characterize and quantify the properties of action potential generation is still today a cornerstone of neuron modelling. Second, the work by Wilfrid Rall (40) to describe the signal propagation along neurites (the “cable theory”) forms a basis for multi-compartmental neuron modelling and the use of reconstructed neuron morphologies. The full potential of these two theoretical approaches has become (and is still becoming) possible through recent development of computer hardware and a base of knowledge about different ion channels and their functions. Indeed, Hodgkin and Huxley themselves could not reach their goal of characterizing the molecular basis of the action potential generation, but 20 years later, the discovery of ion channels established this basis [reviewed in Refs. (41, 42)].
During the 1980s and 1990s, a deeper characterization of the contributors to neuronal electrogenesis was obtained through the advances made in ion-channel blockers and electrophysiological and optical techniques, which allowed the construction of biophysically more detailed neuron models (43). Following the sequencing of the mammalian genomes, a focus is now on describing the ion-channel behavior in terms of their genetic composition. Online databases, such as Channelpedia (http://channelpedia.epfl.ch/) and ICGenealogy (https://icg.neurotheory.ox.ac.uk/) are examples of sources for such information (44, 45). Due to difficulties in both experimental design (46) and the model fitting (cf. 47, 48), this line of development is still in its infancy (45). Nevertheless, the existing biophysically detailed neuron models already classify different types of transmembrane currents based on their sensitivity to different blockers, and it is relatively well known which ion-channel subunits contribute to which of these currents (43). This lays a foundation for analyses of how different genes—and their different variants—affect the neuronal excitability on a cellular level.
Capturing Effects of Genetic Alterations on Channel Properties in Biophysically Detailed Models
Data from functional genomics have attracted modellers of neurons and other excitable cells ever since they became available (49). Clancy and Rudy (50) modelled the effects of long-QT syndrome risk variants on cardiac ventricular action potentials basing on data from electrophysiological measurements performed on cells with wild-type or mutated channels. Splawski et al. (51) measured the effects of a Timothy syndrome mutation on the inactivation of the L-type Ca2+ channels and modelled their effects on cardiomyocyte action potentials. Spampanato et al. (52) predicted altered firing thresholds and increased firing rates for epilepsy-associated mutations based on data from electrophysiological measurements. These are a few of numerous examples, but a notable trend is that most of them concern cardiac cells and heart diseases rather than neurons and brain disorders. This may partly be due to the fact that cardiac disease constitutes the largest cause of death (thus representing an ultimately important topic) and partly because the cardiac cell models are in general genetically better characterized than the neuron models and the link between cellular and tissue-level pathologies is better understood (cf. 53). Among the brain disorders, epilepsy is likely to be the one that has been most studied using computational modelling, due to the multitude of scales at which the disease symptoms and phenotypes can be both observed and modelled (54, 55)—for a review of computational modelling strategies in this field, see Soltesz and Staley (56) and Wendling et al. (57).
In the brain, there are a vast number of different neuron types (let alone glial and endothelial cells), each of which expresses a different set of ion channels and other proteins that change with the developmental stage of the cell and its involvement in neuronal network dynamics (e.g., long-term plasticity) (58, 59). Consequently, each neuron type may contribute in a unique and dynamic way to brain disorder phenotypes. Such a complexity, added to the challenges caused by the branching neurite geometry (60), is a likely cause for a slower development of highly detailed single-neuron models than is the progress made in biophysical models of cardiac cells [see, e.g., Ref. (61)]. Nevertheless, certain neuronal cell types are already relatively well characterized in terms of biophysically detailed models, offering a platform for modelling functional genomics data. Two such neuron types are the Purkinje cells in the cerebellum (62, 63) (cf. 64) and the layer V pyramidal cells in the neocortex (65). Building upon a long line of Purkinje cell models [reviewed in Ref. (66)], a recent model (67) described the kinetics of 15 gene-based types of ion channels and their (manually fitted) distributions along the neuron morphology. The ion-channel descriptions in models of layer V pyramidal cells are not as well characterized down to genetic level, but the more recent models [following the principles in an early, biophysically detailed model by (65)] included nine to 13 different ion channels (68–70). In particular, these models included a simple description of the intracellular Ca2+ dynamics, differentiated between low- and high-voltage-activated Ca2+ currents, and described the kinetics of the Ca2+-activated K+ channels underlying the medium or slow afterhyperpolarization. These models can thus be used to analyze the contributions of both ion channel- and Ca2+ transporter-encoding genes to hyperpolarizing and depolarizing transmembrane currents—and thus to neuron firing.
Unlike simpler models such as integrate-and-fire models or the basic two-channel Hodgkin–Huxley model (39), the biophysically detailed models enable analysis of many aspects of neuron excitability and the contributions of different genes and gene variants to these properties. Masoli et al. (67) validated the model against data from genetic studies that knocked out certain ion channel-encoding genes and observed effects on spontaneous and stimulated firing behaviors, which argues for the usability of the model in predicting effects of mutations in the underlying genes.
Modelling of Non-Ion Channel-Related Genetic Variants and Other Disease-Related Alterations
Rather than simple genetic channelopathies, heritable mental disorders are typically hypothesized to be complex diseases affected by genetic variants in a variety of gene ontologies as well as by environmental factors. To this end, many types of genetic perturbations, other than variants of neuronally expressed voltage-gated ion channel-encoding genes as outlined above, can be flexibly included in computational studies of heritable mental disorders. The premise is that neuronal signalling is the common pathway for all brain biology generating thoughts, emotions, and behavior, and therefore, dysfunctional or lacking neuron firing in one form or another is a common abnormality in all mental diseases. A typical solution for modelling mental diseases other than channelopathies is to use less biophysically detailed modelling approaches, where a disease-related condition observed at a cellular or network level (instead of at the level of proteins) is implemented, and the effects of the condition on the network dynamics or circuit functions are predicted. To name a few examples, in the modelling study of Vattikuti and Chow (71), the autism spectrum disorder-associated phenotypes of saccade hypometria and dysmetria were shown to result from a distorted excitation/inhibition balance, which is one of the leading hypotheses for network-level aberrations in autism spectrum disorder. In a similar fashion, NMDA receptor hypofunction—a widely hypothesized cellular mechanism in schizophrenia [reviewed in Ref. (72)]—was shown to lead to a schizophrenia-associated phenotype, namely, distortions in gamma band oscillations, in a series of modelling studies [reviewed in Ref. (73)]. Comparable computational network studies have used as a starting point a decreased intensity of GABAergic neurotransmission, a schizophrenia anomaly widely suggested by post-mortem studies (74, 75), and analyzed its effects on network dynamics in light of schizophrenia-associated phenotypes (76–79) or hypothesized modes of psychotic circuit activity (80). Similar strategies have been employed in examining the effects of hypodopaminergic modulation of prefrontal cortex, one of the more traditional hypotheses for schizophrenia mechanisms (81), on working memory capacity and precision (82). Typically, these studies employ simpler point-neuron models such as integrate-and-fire models, and the alteration of a model parameter used for representing the disease cannot be directly mapped down to gene level. However, new genetic data and understanding of genetic interactions could be used to revise these descriptions, which would lead to a better understanding of the heritable component of these disorders.
Another new promising subfield of computational psychiatry is the biochemically detailed modelling of brain disorders. For example, computational modelling has clarified the effects of Alzheimer’s disease-related genetic variants on β-amyloid plaque formation and tau-protein phosphorylation, which are among the main hypothesized causes for Alzheimer’s disease [see Ref. (83) for a review]. In Sasidharakurup et al. (84), intracellular signaling pathways in control vs. Parkinson’s disease cases were simulated, and the mechanisms leading to cell death were analyzed. Biochemically detailed modelling of immune system pathways may also constitute an important branch of future computational modelling work in the pathology of schizophrenia and autism, both of which have recently been associated with alterations in inflammatory pathways [reviewed in Refs. (85) and (86)]. A challenge in this type of models is the lack of cellular-level data with which to validate the model parameters describing the biochemical reactions as well as a lack of frameworks in which the model predictions in the cellular and subcellular regimes can be linked to system-level behavior. Nevertheless, such models may be a valuable aid in integrating the effects of environmental factors, including stress, with genetic factors to create a clearer picture of the pathogenesis of heritable mental disorders.
Biophysical Psychiatry: Biophysically Detailed Modelling of Heritable Mental Disorder Mechanisms
We propose to combine neuroscience modelling with psychiatric genomic data in an approach we have termed “biophysical psychiatry.” This is a particular type of neuroscience modelling that integrates data on risk genes and variants thereof to study the pathology of mental disorders. We propose that the development of biophysically detailed models is a suitable method for creating hypotheses on the mechanisms of heritable mental disorders. These can be tested and validated in experiments, both preclinically on a (translational) functional level and clinically, and then further refined. We illustrate the overall concept in Figure 1.
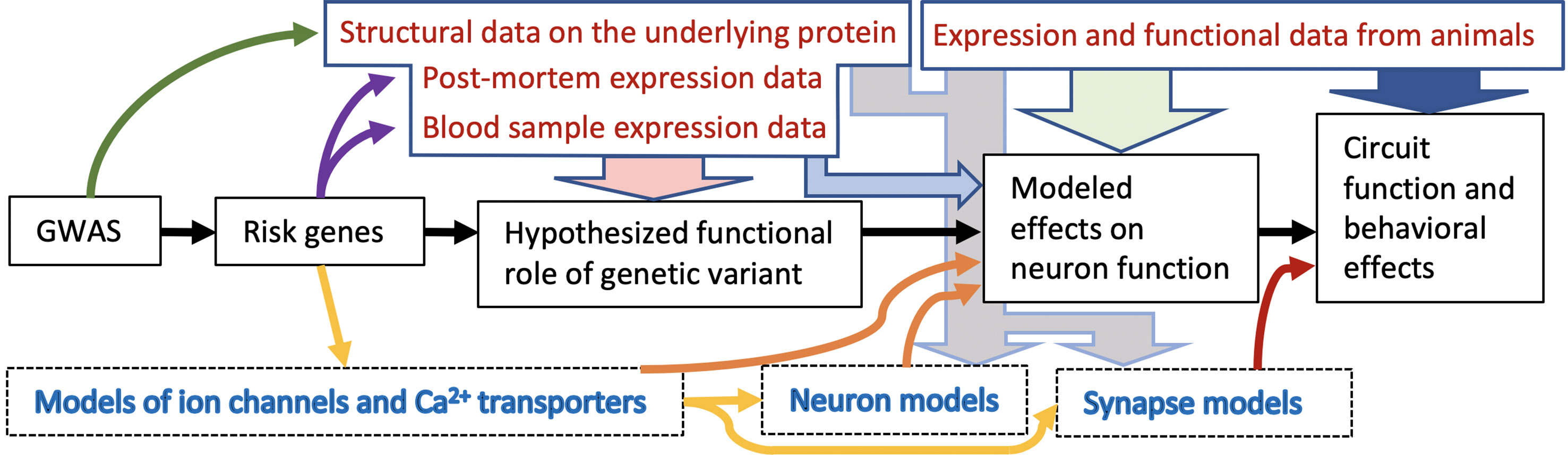
Figure 1 Schematic illustration of the biophysical psychiatry approach for studying heritable mental disorders. This approach typically consists of five main stages ranging from initial GWAS to prediction of the effects of genetic variants on circuit functions (black arrows). First, the results from GWASs are used to identify risk variants and risk genes. Data from patients and structural genomics can be used to constrain the range of possibilities how these variants affect the protein functions: Is there evidence of altered expression of these proteins in the patients and in what tissue (purple arrows)? Are the identified SNPs likely to affect the protein structure (dark green arrow)? This type of information is important for formulating an initial hypothesis on the functional role of the variant (light red arrow), and importantly, together with data from functional genomics, it can be used for computational models of neurons or ion channels as well as other proteins affecting the function of the neuronal circuit (yellow arrows). The effects of the risk gene variants on neuron function can be predicted with the models (orange arrows). When these effects are implemented in a network model that describes both neuronal activity and synaptic communication between the neurons, the impact of the variants on circuit and/or behavioral functions can be predicted (dark red arrow). In case the genetic background of the disease is not known or it is not possible to directly link the genetic variant effects to all desired aspects of the model behavior, cellular-level data from patients or animal model studies can be used as a shortcut and make the model express the dichotomy suggested by the experiments (light grey arrows). Lastly, data from animal studies can be used to validate the model: Is the studied neuron type really behind the modelled phenotype (light green arrow)? Are the genes in which the modelled variants are located expressed in the particular neuron type (light blue arrow)? Are there behavioral observations in animal model studies that support the conclusions (dark blue arrow)? These are but few questions to consider in a modelling approach bridging the gap between genes and behavior.
In our previous work, we applied a polygenic modelling framework similar to that of Figure 1 to study the effects of small effect-size variants of schizophrenia-associated genes on layer V pyramidal cell excitability and integration of inputs (20, 87). Another study measured the effects of schizophrenia-associated de novo mutations in CACNA1I gene on the surface expression of the encoded protein and the corresponding Ca2+ current and simulated their effects on neuron firing in the thalamic reticular nucleus (88). In a modelling study of major depressive disorder, Ramirez-Mahaluf et al. (89) showed that a slowed-down reuptake of glutamate [an alteration supported by post-mortem experimental data from major depressive disorder patients (90)], caused hyperexcitability in the ventral anterior cingulate cortex and suggested an impairment of switching from emotional to cognitive processing. A study combining experimental data and computational modelling showed that a reduction of SK currents, which was experimentally shown to follow a loss-of-function mutation of SCN1A in an epilepsy mouse model, led to prolonged seizure-like bursts of reticular thalamic cells (91). Importantly, when analyzing the effects of the mutations on network dynamics, the biophysically detailed models, unlike integrate-and-fire models and rate-based models, enable the separation between voltage-gated ion channel-mediated and synaptic scaling-mediated effects. For example, we recently showed that neither synaptic scaling [such as that provided by homeostatic plasticity (92)] nor an artificial manipulation of the passive membrane properties can imitate the predicted effects of our variants of voltage-gated ion channel- and Ca2+ transporter-encoding genes on delta power (93). Although such results could not be obtained using simple integrate-and-fire models, these simpler models are nevertheless invaluable in analysis of large-scale network activity. Previously, innovative approaches combining the scalability of the simpler models and the biophysical detail of the more complex models have been applied to the forward modelling of local field potentials (94) and analysis of neuronal input–output relationships (95) and could be employed in studying the genetic effects as well.
Top-Down and Bottom-Up Modelling Approaches
The approaches above can be considered bottom-up approaches as their foundation lies on models and data in the cellular and genetic levels, and they make predictions for higher-level phenotypes. Nevertheless, important insights can also be obtained from top-down approaches, where the models are fitted to higher-level data, such as electroencephalography (EEG) or functional magnetic resonance imaging (fMRI) data, and can make predictions for the cellular of sub-cellular level phenotypes. Typically, bottom-up models are founded in basic laws of physics, while top-down models are more phenomenological by nature. As an example of a top-down approach, in dynamic causal modelling (DCM), a neural mass model is constructed based on EEG or fMRI data and an underlying network model (96, 97). Given measurements of both mental disorder patients and healthy controls, this type of modelling can reveal important circuit pathologies underlying the mental disease. In one study based on DCM and EEG data from patients with psychosis and healthy controls performing an oddball task, a decrease in frontal inhibitory connections in patients with psychosis was predicted (98). Another DCM study predicted an impaired thalamocortical connection in schizophrenia basing on fMRI data collected from psychotic patients and healthy controls performing a verbal fluency task (99). However, the use of DCM for fMRI data has been questioned due to challenges in the underlying biophysical model and statistical inversion (100). Many other biophysically less detailed modelling approaches exist as well, as reviewed in Montague et al. (101) and Wang and Krystal (102). These approaches are especially useful in studying the pathology of non-heritable mental disorders, where the cause of the disorder may be dysfunction or dysconnectivity in a single brain region—however, the use of models that are more detailed, all the way to the genetic level, can be argued for when studying the cause of heritable mental disorders.
Limitations of Biophysical Models of Psychiatric Disease—and Opportunities
There are several challenges in the biophysical psychiatry outlined above. First, the risk variants are typically identified by tag SNPs, which represent variations within a region of DNA instead of a single causative SNP (103). This may distort the analysis of the effects of the variant, as the causal SNP may reside within or outside the protein-coding region of a gene or may regulate many distinct genes. Second, as mentioned earlier, the computational models made for ionic currents are usually not unique to ion-channel subunits encoded by a single gene, and even if they were, there are many parallel genetic pathways that affect the channel kinetics and maximal conductance. While the ion-conducting pore in voltage-gated Ca2+ and Na+ channels is composed of a single α subunit with repeating motifs, the pore in the K+ channels is composed of four subunits that may (homomeric) or may not (heteromeric) be identical, and the composition typically impacts the conductance, voltage dependence, or kinetics of the channel (104). Furthermore, the expression of voltage-gated K+ as well as Ca2+ and Na+ channels on the membrane and their functional properties is affected by the auxiliary subunits (e.g., β subunits) (105). This presents a particular challenge for modelling of polygenic diseases, such as schizophrenia, where there are identified gene variants in various subunit-encoding genes even of the same ion-channel family (34). Third, the neuron models are typically built and validated using data from a specific brain region of a particular animal species (typically, rat or mouse), and thus, predictions made for human disease phenotypes based on such models unavoidably represent a generalization of a kind (106). Fourth, since the models are typically built using single-cell data only, their predictions are primarily applicable to cellular phenotypes only, and prediction of brain-level phenotypes requires careful adjustment of the network model according to the underlying brain microcircuits (107). In particular, the heterogeneity of macroscopic brain signals, such as EEG, in both spatial and temporal domains makes it difficult to link cellular phenotypes to clinical observations (108). This presents a challenge for psychotic disorders, where the symptoms and phenotypes are complex and the cellular and network mechanisms underlying them are largely unknown (109).
Nevertheless, there are ongoing large-scale projects and recent technological advances that aid in overcoming the abovementioned obstacles. First, ongoing international projects such as ENCODE as well as new data from deep sequencing (110) offer new detailed insights on the genetic risks of diseases. This can help in identifying risk-conferring SNPs and discovering the correlation structure between the SNPs, both locally and across the genome, and thus complements the GWAS data from large consortia. Second, quantitative data on ion-channel behavior and the contributions of different subunits and other proteins are rapidly growing, thanks to both online databases (such as Channelpedia and ICGenealogy) and new insights from molecular dynamics simulation approaches (111). Third, new large-scale projects such as the Allen Brain Atlas (https://www.brain-map.org/) and Human Protein Atlas (https://www.proteinatlas.org/) offer unique, standardized data sets on the expression of genes in both mice and men. In addition to brain area-wise information on gene expression and connectivity in the mouse brain, the Allen Brain Atlas includes a detailed data set on expression of tens of thousands of genes in different layers of the mouse primary visual cortex (http://casestudies.brain-map.org/celltax). This allows modellers of effects of particular gene variants on cortical phenotypes to check whether the underlying gene and related genes are expressed in the cell type they are interested in or not, which helps in formulating and refining the research hypotheses. Moreover, neuroinformatics databases such as ModelDB (https://senselab.med.yale.edu/modeldb/) help the modelers by offering curated neuron models from a variety of brain areas. Fourth, there are ongoing and recently completed large-scale projects that extend our knowledge on structure and function of brain circuits, in terms of both quantitative, computational models and qualitative or conceptual models. The NIH BRAIN initiative is a mega-scale program aiming at this goal (112). The Human Brain Project (https://www.humanbrainproject.eu/) has a comparable goal in developing an infrastructure that allows multi-scale modelling of large brain networks (113). Supporting this goal, the preceding EPFL-led project, the Blue Brain project (lending its name from the IBM’s Blue Gene supercomputer project) already offers large amounts of data that are directly usable in models (114). Improved quantification and understanding of the structure and dynamics of the neural circuits are essential for the accuracy of forward models of macroscopic brain signals and thus vital to the translation of findings from the models into clinical knowledge.
The projects named above support the development of new neuron models that are more accurate than before, in terms of both single-cell structure and dynamics (which ion channels are expressed and where in the neuron) and network interactions (how the neuron activity is affected by inputs from other cells). This is an important milestone on the way toward better predictive power for maps from gene to network level (107)—yet one has to keep in mind that detailed biophysics in one domain, such as voltage-gated ion channels, does not mean that the model is biophysically detailed in all aspects (cf. 19, 102). In particular, there is much to improve in how the actions of neuromodulators can be taken into account in the neuron models (115). Emphasis should also be placed on validation of the generated neuron models, which has recently been made easier by new, standardized tools (116).
In addition to these challenges, there is a lack of understanding of contributions of intracellular signalling mechanisms (117) as well as glial cell functions (118) and adult neurogenesis (119) to brain functions in health and disease. The schizophrenia-associated genes encode many types of phosphatases and kinases involved in neurotransmission and long-term plasticity (35)—variants of these genes can thus have crucial effects on neuronal circuit functions. Moreover, some of the schizophrenia-associated ion channel-encoding genes are also expressed in astrocytes (120). Biophysical models of glial functions, particularly models for Ca2+ excitability, exist, but the field is immature, and models are required to be better validated against electrophysiological and imaging data [for a review of models and their characteristics, see Ref. (121)]. The complexity of the intracellular signalling pathways also manifests in compensatory mechanisms that may counteract the effects of the modelled variants on both genetic [see, e.g., Ref. (122)] and cellular and network levels (123). Better integration across different scales and expanded quantitative descriptions of the biochemical machinery have been proposed as a path toward improved predictive power of the biophysically and biochemically detailed models (124, 125).
Parallel Approaches in Computational Cardiac Science
As mentioned earlier, computational approaches have proved successful in reconstructing and explaining the mechanisms of several genetic conditions in cardiac electrophysiology. These successes have generally required detailed characterization of the functional outcomes of these mutations via classical electrophysiological methods, but similar approaches should be possible in sufficiently well-characterized neuropsychological conditions. The core requirement is a set of disease biomarkers that can be reproducibly observed in patients and a computational framework for replicating changes in those biomarkers due to testable genetic changes in the underlying neural machinery. Both of these characteristics have been developed for many congenital diseases of cardiac electrophysiology. This has been achieved in part by specifically seeking systematic understanding of diseases with clear distinguishing phenotypes, and in part through incrementally extending fundamental knowledge of cardiac bioelectricity to allow well-defined hypothesis generation and testing (126). While, as mentioned above, mental illnesses pose a greater set of challenges, there is no clear reason for these principles not to be similarly applied to the brain.
Independently of genetic diseases, current approaches to cardiac drug screening provide another example of the mature role that computation and modelling play in the development of cardiac pharmacology. At the simplest level, computational approaches have been adopted to define optimal drug characteristics for specific cardiac conditions—for example, to reduce the unintended impacts of atrial fibrillation treatments (127). Most recently and considerably, a U.S. Food and Drug Administration-initiated consortium named the Comprehensive In Vitro Proarrhythmia Assay initiative (CiPA; https://cipaproject.org/) has been created to develop a multidisciplinary platform for better discriminating compounds with likely cardiac toxicity from those that are inert or beneficial (128, 129). This is a critical societal development because cardiac toxicity is the single largest cause of advanced stage drug failure across all disease indications. As a testament to the importance of modelling in cardiac pharmacology, CiPA has been chosen as an aim to replace small mammal experimental testing with model-based computational translation of in vitro electrophysiology to predict drug outcomes in humans.
Finally, with respect to multiscale aspects, computational modelling of cardiac electrical pathology has advanced to the stage that patient-specific and heart-scale models are now being used to guide clinical intervention. The use of these models to predict the sites of ablation for infarct-associated arrhythmia has recently been approved for a full prospective clinical investigation by Johns Hopkins University School of Medicine. This substantial development has followed a series of compelling retrospective studies, and most recently a mixed retrospective/prospective approach (130). In silico studies of cellular and subcellular disease phenotypes can also be conducted on brain and heart cell models in parallel, as done in Mäki-Marttunen et al. (87).
Experimental Validation of Biophysical Models
Taken together, biophysically detailed modelling is in many ways a promising method for creating hypotheses on the mechanisms of mental disorders with a genetic component. The obtained hypotheses need to be tested experimentally, but these experiments are difficult partly for the same reasons that make the modelling of disease phenotypes difficult: the gaps between the scales of phenomena (genes to behavior) and the translation of findings from laboratory animals to humans. One method shows a particular potential in this regard: induced pluripotent stem cell (iPSC)-based cellular models (131). The development of iPSCs provides a rare opportunity for studying neuronal excitability and identifying clinically useful biomarkers in schizophrenia based on electrophysiology (132, 133). Although human iPSC-based approaches do not provide the full complexity of the central nervous system, the cellular phenotypes are likely to lie closer to the genetic and molecular disease mechanisms than phenotypes observed at the tissue or organism level (134). Therefore, cellular and small network phenotypes, achievable in iPSC-derived neuronal two- and three-dimensional cell cultures, may offer a more direct readout of the pathophysiological processes as an intermediate step toward understanding pathophysiology at the brain level.
As the cells in the iPSC approach are derived from patients, they include the complete genetic background (and possibly epigenetic modifications) of an individual in addition to the risk alleles. On the one hand, this may make the analysis of the results more difficult, but on the other hand, it can lead to discovery of new genetic modifiers (135). Moreover, iPSC-derived neurons from patients can be transplanted into developing or mature rodent brains to provide a (non-human) brain environment into which the human neurons can structurally and functionally integrate. This procedure is costly at the moment but shows promise in preclinical research on psychotic disorders (135–139).
Finally, since mental disorders appear to be exclusively human disorders, the hypotheses concerning the disease mechanisms should also be tested directly in human patients. Invasive recordings are very seldom an option, but data from non-invasive recordings, such as EEG, magnetoencephalography (MEG), and magnetic resonance imaging (MRI), could be used for testing certain model predictions. New, automated approaches may help in model validation against clinical observations—also against EEG data (140). To obtain a better translation of simulated neuronal dynamics to clinical measurements, new computational approaches allow forward modelling of EEG and MEG data based on the description of the transmembrane currents across the neuron morphology (141). As for MRI, the link between neuronal activity and measurable MRI signals involves more unknowns and is thus more speculative, but forward models exist for this approach too (142). The use of these models is constrained by the sparse temporal resolution that is typical to MRI signals (143). On the other hand, the number of studies showing differences in structural and functional MRI data between healthy controls and people with mental disorder is steadily increasing [see, e.g., a special issue on the topic (144), and newer discoveries (145–147)]. This type of data could potentially be used to validate mechanistic multiscale models that predict differences in brain-area interactions or even development of anatomical connections between healthy controls and patients.
Having a forward model from single-cell activity (or even subcellular dynamics) to brain measurables is important, because many of the endophenotypes of mental disorders (especially those of schizophrenia) are in fact electrophysiological measures of brain activity under certain stimulus protocols (148). As an example, the mismatch negativity (MMN) is the brain’s response to an outlier among a series of stimuli, such as a sound with a slightly different pitch or duration among other identical sounds. MMN is weakened in schizophrenia patients, and it is “automatic” in the sense that it is evoked in protocols that do not require cognitive effort (149). MMN has also recently been acknowledged as a key biomarker for better understanding the pathophysiology of schizophrenia (150).
Another prominent EEG-based biomarker of schizophrenia is altered delta power, which is one of the more robust schizophrenia-associated brain-oscillation phenotypes (151). To this end, computational modellers of neuronal networks have a long history of reproducing neural oscillations of different frequencies and analyzing the cellular and network mechanisms underlying them (152). Figure 2 shows findings from our recent study where the forward EEG modelling approach was used to show that alterations in schizophrenia-associated voltage-gated ion channel and Ca2+ transporter-encoding genes can cause increased delta power (93). Discovering the mechanisms through which genetic risk leads to these widely examined quantitative biomarkers is an important milestone on the path to understanding the complex, largely qualitative symptoms and pathogenesis of mental disorders. In addition to EEG protocols based on resting state or sensory stimuli, magnetic and electrical brain stimulation could also be used for testing hypotheses on altered brain activity in mental disorders [these approaches are reviewed in Ref. (102)].
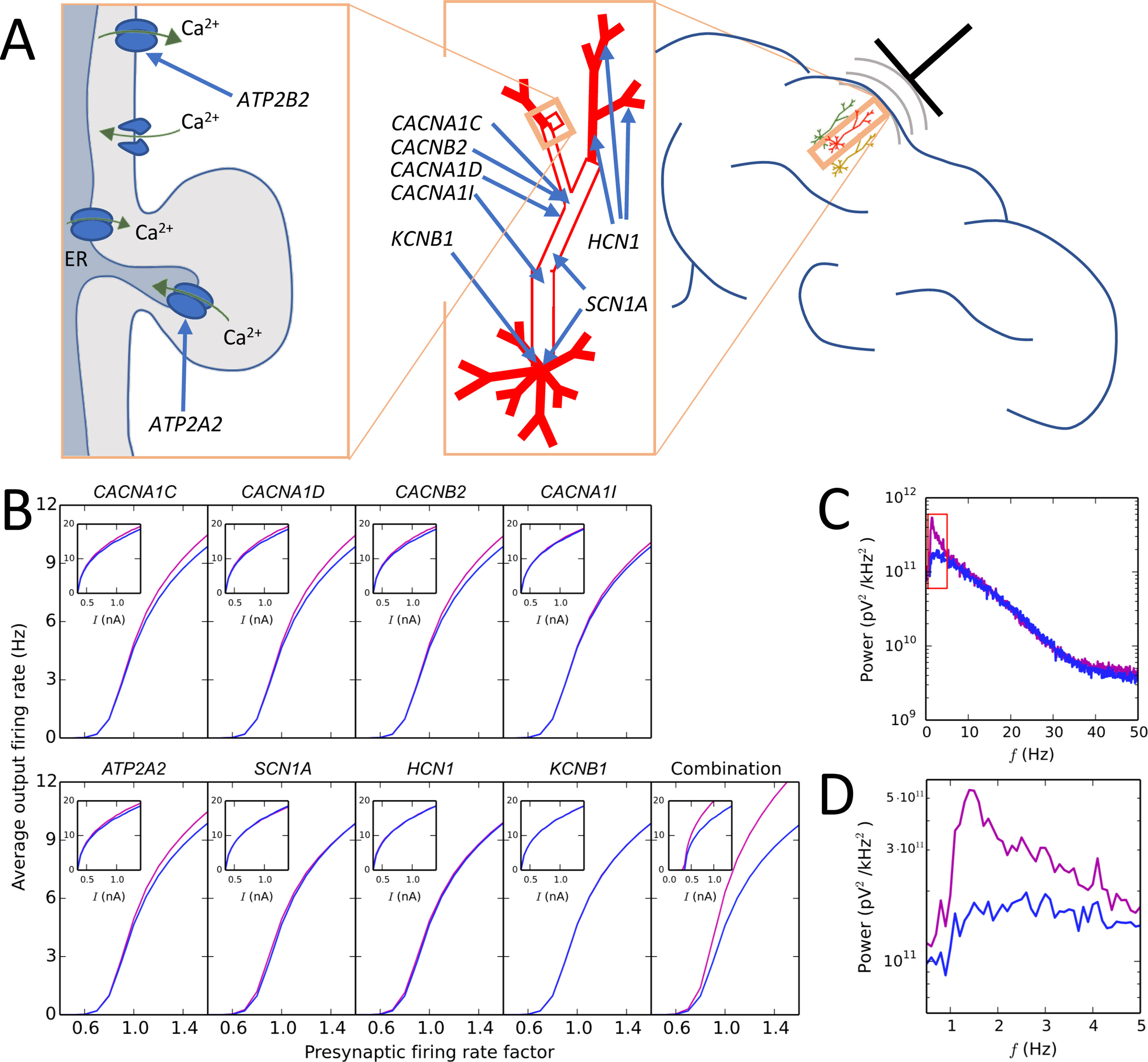
Figure 2 Illustration of a modelling approach predicting that a combination of small-effect variants in different schizophrenia-associated ion channel- and Ca2+ transporter-encoding genes causes large effects on the delta band in the EEG power spectrum. (A) Schematic illustration of the subcellular domain (left) of a layer V pyramidal cell (middle) and the localization of the neuron with respect to a recording electrode (right). Genes ATP2A2 and ATP2B2 contribute to the Ca2+ dynamics of the neuron: ATP2A2 encodes a subunit of the sarco/endoplasmic reticulum Ca2+-ATPase (SERCA) that pumps cytosolic Ca2+ into the endoplasmic reticulum (ER), and ATP2B2 encodes a subunit of the plasma membrane Ca2+ ATPase (PMCA) that expels cytosolic Ca2+ to the extracellular medium. Genes CACNA1C, CACNA1D, and CACNB2 encode subunits of high-voltage activated Ca2+ channels, and CACNA1I encodes a low-voltage activated Ca2+ channel: these channels are densely expressed in the “hot zone” of Ca2+ channels at the apical dendrite, but they are present in the soma as well (68). SCN1A encodes a subunit of the fast voltage-gated Na+ channel that is densely expressed in the soma and mildly expressed in the apical dendrite (68). Finally, KCNB1 encodes a subunit of a voltage-gated K+ channel that is only present in the soma, and HCN1 encodes a subunit of a non-specific cationic channel whose density grows toward the end of the apical dendrite (68). (B) Effects of model variants of schizophrenia-associated ion channel- and Ca2+ transporter-encoding genes on firing rates of layer V pyramidal neurons as part of a network (main curves) and in isolation (insets). In the network experiments, interconnected networks of 150 neurons were simulated for 11 s so that the rate of presynaptic inputs (AMPA, NMDA, and GABA receptor-mediated currents) was controlled by factor (shown on x-axis), which influenced the average firing rate of the neurons in the network (shown on y-axis). In the single-cell experiments, a prolonged somatic square-pulse current (amplitude shown on x-axis) was given at the soma, affecting the firing frequency (shown on y-axis). The blue curves show the control network/neuron, while the purple curves show the network/neuron with the model variant. The fifth subpanel in the bottom row shows the effects of combination of the eight variants. For network simulations, reduced-morphology representations (48) of the layer V pyramidal cells were used to avoid excessive simulation load. Adapted from Mäki-Marttunen et al. (93). (C) Power spectrum of the EEG signal predicted from the activity of the network corresponding to presynaptic rate factor 1.0 in control and variant-combination conditions of panel (B). To obtain this signal, transmembrane currents were registered at each compartment of each cell, and these currents were used to calculate the dipole moment time series of the cell population. From the dipole moments, the EEG signal was estimated using a theoretical model of Næss et al. (153), implemented in the LFPy Python package (141). Consistent with the predictions of panel (B), the EEG power is increased for the combination of model variants compared with the control network, especially in the delta (0.5–5 Hz) range. Adapted from Mäki-Marttunen et al. (93). (D) Zoomed-in view on the red rectangle of panel (B).
Relevance for Biomarkers and Drug Development
In addition to shedding light on the disease mechanisms of mental disorders, biophysically detailed neuron modelling is a suitable tool to assist drug development for diseases where ion-channel functions are impaired (cf.154, 155). However, until a clearer picture of the pathology of a mental disorder is formed, the use of biophysical modelling as a means of treatment design may remain a long-term ambition. Especially in the case of schizophrenia, there are currently many more open questions concerning all levels of brain function than answers to these questions: How do the schizophrenia-associated gene variants (27) affect neuronal excitability in different brain areas? Are the observed changes in connectivity a response to this altered excitability, or do they represent an independent disease phenotype? How can we relate changes in neuron excitability and neuronal connectivity to negative and positive symptoms of the disease? What is the cell-type specific contribution to the pathophysiology? If the impaired ion-channel function could be restored by drugs, should it be done before the onset of the disease or can it be done afterwards? From the modelling perspective, the involvement of the immune system in schizophrenia represents another great unknown, as there are few modelling efforts integrating the neuronal functions with altered immune system functions. Nevertheless, in combination with animal models of schizophrenia, computational models could be employed to test specific hypotheses on drug effects on cellular and network-level functions, and the data obtained could be used to translate them into hypotheses on human brain functions.
We believe that in the near future, biophysically detailed modelling can become a fruitful tool in systematic analysis of the endophenotypes and biomarkers of the mental disorders. Endophenotypes have been suggested as a gate toward understanding the pathology of diseases where both the genetic origin and disease symptoms are complex (156). This is a case in point for schizophrenia. There are many widely adopted schizophrenia-associated endophenotypes and biomarkers that can be quantified using behavioral tests or electrophysiology. Due to the possibility of reference to animal models, these endophenotypes and biomarkers are better characterized than the symptoms of schizophrenia, which are almost exclusively human symptoms. The genetic foundation of these endophenotypes and biomarkers is still largely unknown. However, unlike the symptoms of the mental disorders, the physiology and neuronal origin of these phenotypes are relatively well mapped. Biophysically detailed modelling could become an invaluable tool for deeper characterization of the effects of disease-associated variants on the disease endophenotypes and biomarkers, which would shed light on the pathology of the heritable mental disorder as a whole.
Conclusions
Biophysically detailed modelling of brain functions and the contributions of disease-associated genetic variants therein is a significant challenge for this century. Eventually, this “biophysical psychiatry” approach may become a powerful approach to generate testable hypotheses for the pathophysiology of complex, heritable mental disorders such as schizophrenia. International consortia that collect and analyze patient data as well as other large-scale efforts that map mammalian brain structure and function are key sources of data for this line of research, but new innovative experimental techniques and modelling approaches are required as well in order to gain maximally informative insights into mental disorder pathology.
Author Contributions
TM-M, AE, GE, and OA wrote the manuscript. TK, TE, AD, SD, LW, M-LL, MR, DS, SB, ME-B, FB, GH, EH, SN, TN, TM, CM, MF, and AD provided analytical support.
Funding
Funding for this work was received through NIH grant 5 R01 EB000790-10, the European Union Horizon 2020 Research and Innovation Programme under Grant Agreement No. 785907 [Human Brain Project (HBP) SGA2]; ERA-NET NEURON project “SYNSCHIZ” (Research Council of Norway, grant number 283798; Bundesministerium für Bildung und Forschung, grant number 01EW1810; Swiss National Science Foundation, grant number 32NE30 179602; Academy of Finland, grant number 318879; NWO & Hersenstichting, grant number 013-17-003 4538; UEFISCDI, grant number 6/2018); Research Council of Norway (216699, 223273, 226971, 248778, 249711, 248828, and 250128); South East Norway Health Authority (2016-082,2017-112); KG Jebsen Stiftelsen, and a research grant from Mrs. Throne-Holst.
Conflicts of Interest Statement
The authors declare that the research was conducted in the absence of any commercial or financial relationships that could be construed as a potential conflict of interest.
References
1. Rössler W, Salize HJ, van Os J, Riecher-Rössler A. Size of burden of schizophrenia and psychotic disorders. Eur Neuropsychopharmacol (2005) 15(4):399–409. doi: 10.1016/j.euroneuro.2005.04.009
3. Charlson FJ, Ferrari AJ, Santomauro DF, Diminic S, Stockings E, Scott JG, et al. Global epidemiology and burden of schizophrenia: findings from the Global Burden of Disease Study 2016. Schizophr Bull (2018) 44(6):1195–203. doi: 10.1093/schbul/sby058
4. Walker ER, McGee RE, Druss BG. Mortality in mental disorders and global disease burden implications: a systematic review and meta-analysis. JAMA psychiatry (2015) 72(4):334–41. doi: 10.1001/jamapsychiatry.2014.2502
5. Vos T, Barber RM, Bell B, Bertozzi-Villa A, Biryukov S, Bolliger I, et al. Global, regional, and national incidence, prevalence, and years lived with disability for 301 acute and chronic diseases and injuries in 188 countries, 1990–2013: a systematic analysis for the Global Burden of Disease Study 2013. Lancet (2015) 386(9995):743–800.
6. Sawa A, Snyder SH. Schizophrenia: diverse approaches to a complex disease. Science (2002) 296(5568):692–5. doi: 10.1126/science.1070532
7. Miller G (2010). Is pharma running out of brainy ideas? American Association for the Advancement of Science (United States). doi: 10.1126/science.329.5991.502
8. Jemal A, Murray T, Ward E, Samuels A, Tiwari RC, Ghafoor A, et al. Cancer statistics, 2005. CA. a cancer J for clinicians (2005) 55(1):10–30. doi: 10.3322/canjclin.55.1.10
9. Hashim D, Boffetta P, La Vecchia C, Rota M, Bertuccio P, Malvezzi M, et al. The global decrease in cancer mortality: trends and disparities. Annals of Oncology (2016) 27(5):926–33. doi: 10.1093/annonc/mdw027
10. Collins FS, Morgan M, Patrinos A. The Human Genome Project: lessons from large-scale biology. Science (2003b) 300(5617):286–90. doi: 10.1126/science.1084564
11. Collins FS, Green ED, Guttmacher AE, Guyer MS. A vision for the future of genomics research. Nature (2003a) 422(6934):835. doi: 10.1038/nature01626
12. Ginsburg GS, Willard HF. Essentials of genomic and personalized medicine. Academic Press, USA. (2009). doi: 10.1016/B978-0-12-374934-5.00001-5
13. Kandel ER, Markram H, Matthews PM, Yuste R, Koch C. Neuroscience thinks big (and collaboratively). Nat Rev Neurosci (2013) 14(9):659. doi: 10.1038/nrn3578
14. Einevoll GT, Destexhe A, Diesmann M, Grün S, Jirsa V, de Kamps M, et al. The scientific case for brain simulations. Neuron (2019) 102(4):735–44. doi: 10.1016/j.neuron.2019.03.027
15. Herz AV, Gollisch T, Machens CK, Jaeger D. Modeling single-neuron dynamics and computations: a balance of detail and abstraction. Science (2006) 314(5796):80–5. doi: 10.1126/science.1127240
16. Brette R, Rudolph M, Carnevale T, Hines M, Beeman D, Bower JM, et al. Simulation of networks of spiking neurons: a review of tools and strategies. J Comput Neurosci (2007) 23(3):349–98. doi: 10.1007/s10827-007-0038-6
17. Hines ML, Carnevale NT. The NEURON simulation environment. Neural Comput (1997) 9(6):1179–209. doi: 10.1162/neco.1997.9.6.1179
18. Bhattacharya BS, Chowdhury FN. Validating neuro-computational models of neurological and psychiatric disorders Vol. 14. Springer, Switzerland. (2015). doi: 10.1007/978-3-319-20037-8
19. Huys QJ, Maia TV, Frank MJ. Computational psychiatry as a bridge from neuroscience to clinical applications. Nat Neurosci (2016) 19(3):404. doi: 10.1038/nn.4238
20. Mäki-Marttunen T, Halnes G, Devor A, Witoelar A, Bettella F, Djurovic S, et al. Functional effects of schizophrenia-linked genetic variants on intrinsic single-neuron excitability: a modeling study. Biol Psychiatry Cogn Neurosci and Neuroim (2016) 1(1):49–59. doi: 10.1016/j.bpsc.2015.09.002
21. Bauer P, Thorpe A, Brunet G. The quiet revolution of numerical weather prediction. Nature (2015) 525(7567):47. doi: 10.1038/nature14956
22. Gottlieb B, Chalifour LE, Mitmaker B, Sheiner N, Obrand D, Abraham C, et al. BAK1 gene variation and abdominal aortic aneurysms. Hum Mutat (2009) 30(7):1043–7. doi: 10.1002/humu.21046
23. Sharma A, Ansari AH, Kumari R, Pandey R, Rehman R, Mehani B, et al. Human brain harbors single nucleotide somatic variations in functionally relevant genes possibly mediated by oxidative stress. F1000Research (2016) 5:2520. doi: 10.12688/f1000research.9495.1
24. Mastretta-Yanes A, Arrigo N, Alvarez N, Jorgensen TH, Piñero D, Emerson B. Restriction site-associated DNA sequencing, genotyping error estimation and de novo assembly optimization for population genetic inference. Mol Ecol Resour (2015) 15(1):28–41. doi: 10.1111/1755-0998.12291
25. Visscher PM, Wray NR, Zhang Q, Sklar P, McCarthy MI, Brown MA, et al. 10 years of GWAS discovery: biology, function, and translation. Am J Hum Genet (2017) 101(1):5–22. doi: 10.1016/j.ajhg.2017.06.005
26. Stephan R, Benjamin MN, Aiden C, James TRW, Kai-How F, Peter AH, et al. Biological insights from 108 schizophrenia-associated genetic loci. Nature (2014) 511(7510):421–7 doi: 10.1038/nature13595
27. Pardinas AF, Holmans P, Pocklington AJ, Escott-Price V, Ripke S, Carrera N, et al. Common schizophrenia alleles are enriched in mutation-intolerant genes and in regions under strong background selection. Nat Genet (2018) 50(3):381. doi: 10.1038/s41588-018-0059-2
28. Henriksen MG, Nordgaard J, Jansson LB. Genetics of schizophrenia: overview of methods, findings and limitations. Front Hum Neurosci (2017) 11:322. doi: 10.3389/fnhum.2017.00322
29. Wang Y, Thompson WK, Schork AJ, Holland D, Chen C-H, Bettella F, et al. Leveraging genomic annotations and pleiotropic enrichment for improved replication rates in schizophrenia GWAS. PLoS Genet (2016) 12(1):e1005803. doi: 10.1371/journal.pgen.1005803
30. Holland D, Wang Y, Thompson WK, Schork A, Chen C-H, Lo M-T, et al. Estimating effect sizes and expected replication probabilities from GWAS summary statistics. Front Genet (2016) 7:15. doi: 10.3389/fgene.2016.00015
31. Frei O, Holland D, Smeland OB, Shadrin AA, Fan CC, Witoelar A, et al. Bivariate Gaussian mixture model of GWAS (BGMG) quantifies polygenic overlap between complex traits beyond genetic correlation. bioRxiv (2017) 240275. doi: 10.1101/240275
32. Michel M, Schmidt MJ, Mirnics K. Immune system gene dysregulation in autism and schizophrenia. Dev Neurobiol (2012) 72(10):1277–87. doi: 10.1002/dneu.22044
33. Pers TH, Timshe lP, Ripke S, Lent S, Sullivan PF, O’donovan MC, et al. Comprehensive analysis of schizophrenia-associated loci highlights ion channel pathways and biologically plausible candidate causal genes. Hum Mol Genet (2016) 25(6):1247–54. doi: 10.1093/hmg/ddw007
34. Li W, Fan CC, Mäki-Marttunen T, Thompson WK, Schork AJ, Bettella F, et al. A molecule-based genetic association approach implicates a range of voltage-gated calcium channels associated with schizophrenia. Am J Med Genet Part B: NeuroPsychiatry Genet (2018) 177(4):454–67. doi: 10.1002/ajmg.b.32634
35. Devor A, Andreassen O, Wang Y, Mäki-Marttunen T, Smeland O, Fan C, et al. Genetic evidence for role of integration of fast and slow neurotransmission in schizophrenia. Mol Psychiatry (2017) 22(6):792. doi: 10.1038/mp.2017.33
36. Steullet P, Cabungcal J, Monin A, Dwir D, O’Donnell P, Cuenod M, et al. Redoxdysregulation, neuroinflammation, and NMDA receptor hypofunction: a “central hub”in schizophrenia pathophysiology? Schizophr Res (2016) 176(1):41–51. doi: 10.1016/j.schres.2014.06.021
37. Hardingham GE, Do KQ. Linking early-life NMDAR hypofunction and oxidative stress in schizophrenia pathogenesis. Nat Rev Neurosci (2016) 17(2):125. doi: 10.1038/nrn.2015.19
38. De Schutter E. Why are computational neuroscience and systems biology so separate? PloS Comput Biol (2008) 4(5):e1000078. doi: 10.1371/journal.pcbi.1000078
39. Hodgkin AL, Huxley AF. A quantitative description of membrane current and its application to conduction and excitation in nerve. J Physiol (Lond) (1952) 117(4):500–44. doi: 10.1113/jphysiol.1952.sp004764
40. Rall W. Branching dendritic trees and motoneuron membrane resistivity. Exp Neurol (1959) 1(5):491–527. doi: 10.1016/0014-4886(59)90046-9
41. Destexhe A, Huguenard JR. Modeling voltage-dependent channels. In: E, De Schutter, editor. Computational modeling methods for neuroscientists. The MIT Press, USA. (2007).
42. Sterratt D, Graham B, Gillies A, Willshaw D. Principles of computational modelling in neuroscience. Cambridge University Press, UK. (2011).
43. Catterall WA, Raman IM, Robinson HP, Sejnowski TJ, Paulsen O. The Hodgkin–Huxley heritage: from channels to circuits. J Neurosci (2012) 32(41):14064–73. doi: 10.1523/JNEUROSCI.3403-12.2012
44. Ranjan R, Logette E, Petitprez S, Marani M, Mirjia H, Muller EB, et al. Automated biophysical characterization of the complete rat Kv-ion channel family. Society for Neuroscience Meeting SfN2014, Washington, DC, USA, November 15-19, (2014).
45. Podlaski WF, Seeholzer A, Groschner LN, Miesenb¨ock G, Ranjan R, Vogels TP. Mapping the function of neuronal ion channels in model and experiment. Elife (2017) 6:e22152. doi: 10.7554/eLife.22152
46. Keren N, Bar-Yehuda D, Korngreen A. Experimentally guided modelling of dendritic excitability in rat neocortical pyramidal neurones. J Physiology (Lond) (2009) 587(7):1413–37. doi: 10.1113/jphysiol.2008.167130
47. Almog M, Korngreen A. Is realistic neuronal modelling realistic? J Neurophysiol (2016) 116(5):2180–209. doi: 10.1152/jn.00360.2016
48. Mäki-Marttunen T, Halnes G, Devor A, Metzner C, Dale AM, Andreassen OA, et al. A stepwise neuron model fitting procedure designed for recordings with high spatial resolution: application to layer 5 pyramidal cells. J Neurosci Methods (2018a) 273:264–83. doi: 10.1016/j.jneumeth.2017.10.007
49. Noble D. The rise of computational biology. Nature Rev Mol Cell Biol (2002) 3(6):459. doi: 10.1038/nrm810
50. Clancy CE, Rudy Y. Linking a genetic defect to its cellular phenotype in a cardiac arrhythmia. Nature (1999) 400(6744):566. doi: 10.1038/23034
51. Splawski I, Timothy KW, Sharpe LM, Decher N, Kumar P, Bloise R, et al. Cav1.2 calcium channel dysfunction causes a multisystem disorder including arrhythmia and autism. Cellular (2004) 119(1):19–31. doi: 10.1016/j.cell.2004.09.011
52. Spampanato J, AradiI, Soltesz I, Goldin AL. Increased neuronal firing in computer simulations of sodium channel mutations that cause generalized epilepsy with febrile seizures plus. J Neurophysiol (2004) 91(5):2040–50. doi: 10.1152/jn.00982.2003
53. Fenton FH, Cherry EM. Models of cardiac cell. Scholarpedia (2008) 3(8):1868. doi: 10.4249/scholarpedia.1868
54. Holt AB, Netoff TI. Computational modeling of epilepsy for an experimental neurologist. Exp Neurol (2013) 244:75–86. doi: 10.1016/j.expneurol.2012.05.003
55. Kuhlmann L, Grayden DB, Wendling F, Schiff SJ. The role of multiple-scale modelling of epilepsy in seizure forecasting. J Clin Neurophysiol (2015) 32(3):220. doi: 10.1097/WNP.0000000000000149
57. Wendling F, Bartolomei F, Modolo J. Neocortical/thalamic in silico models of seizures and epilepsy. In: Models of seizures and epilepsy., Elsevier, USA. (2017). p. 233–46. doi: 10.1016/B978-0-12-804066-9.00016-X
58. Tsodyks M. Computational neuroscience grand challenges—a humble attempt at future forecast. Front Neurosci (2008) 2:21. doi: 10.3389/neuro.01.021.2008
59. Ecker JR, Geschwind DH, Kriegstein AR, Ngai J, Osten P, Polioudakis D, et al. The BRAIN Initiative Cell Census Consortium: lessons learned toward generating a comprehensive brain cell atlas. Neuron (2017) 96(3):542–57. doi: 10.1016/j.neuron.2017.10.007
60. Connors BW, Regehr WG. Neuronal firing: does function follow form? Curr Biol (1996) 6(12):1560–2. doi: 10.1016/S0960-9822(02)70771-9
61. Pugsley MK, Curtis MJ, Hayes ES. Biophysics and molecular biology of cardiac ion channels for the safety pharmacologist. In: Principles of safety pharmacology. Springer, Germany. (2015). p. 149–203. doi: 10.1007/978-3-662-46943-9_7
62. De Schutter E, Bower JM. Simulated responses of cerebellar Purkinje cells are independent of the dendritic location of granule cell synaptic inputs. Proc Natl Acad Sci USA (1994) 91(11):4736–40. doi: 10.1073/pnas.91.11.4736
63. Zang Y, Dieudonné S, De Schutter E. Voltage- and branch-specific climbing fiber responses in Purkinje cells. Cell reports (2018) 24(6):1536–49. doi: 10.1016/j.celrep.2018.07.011
64. Gruol D, Manto M, Haines D. Ca2+ signaling in cerebellar Purkinje neurons. Cerebellum (2012) 11(3):605–8. doi: 10.1007/s12311-012-0404-4
65. Mainen ZF, Sejnowski TJ. Influence of dendritic structure on firing pattern in model neocortical neurons. Nature (1996) 382(6589):363–6. doi: 10.1038/382363a0
66. Bower JM. The 40-year history of modeling active dendrites in cerebellar Purkinje cells: emergence of the first single cell “community model”. Front Comput Neurosci (2015) 9:129. doi: 10.3389/fncom.2015.00129
67. Masoli S, Solinas S, D’Angelo E. Action potential processing in a detailed Purkinje cell model reveals a critical role for axonal compartmentalization. Front Cell Neurosci (2015) 9:47. doi: 10.3389/fncel.2015.00047
68. Hay E, Hill S, Schürmann F, Markram H, Segev I. Models of neocortical layer 5b pyramidal cells capturing a wide range of dendritic and perisomatic active properties. PloS Comput Biol (2011) 7:e1002107. doi: 10.1371/journal.pcbi.1002107
69. Almog M, Korngreen A. A quantitative description of dendritic conductances and its application to dendritic excitation in layer 5 pyramidal neurons. J Neurosci (2014) 34(1):182–96. doi: 10.1523/JNEUROSCI.2896-13.2014
70. Papoutsi A, Sidiropoulou K, Cutsuridis V, Poirazi P. Induction and modulation of persistent activity in a layer V PFC microcircuit model. Front Neural circuits (2013) 7:161. doi: 10.3389/fncir.2013.00161
71. Vattikuti S, Chow CC. A computational model for cerebral cortical dysfunction in autism spectrum disorders. Biol Psychiatry (2010) 67(7):672–8. doi: 10.1016/j.biopsych.2009.09.008
72. Gao W-J, Snyder MA. NMDA hypofunction as a convergence point for progression and symptoms of schizophrenia. Front Cell Neurosci (2013) 7:31. doi: 10.3389/fncel.2013.00031
73. Jadi MP, Behrens MM, Sejnowski TJ. Abnormal gamma oscillations in N-methyl-d-aspartate receptor hypofunction models of schizophrenia. Biol Psychiatry (2016) 79(9):716–26. doi: 10.1016/j.biopsych.2015.07.005
74. Gonzalez-Burgos G, Lewis DA. GABA neurons and the mechanisms of network oscillations: implications for understanding cortical dysfunction in schizophrenia. Schizophr Bull (2008) 34(5):944–61. doi: 10.1093/schbul/sbn070
75. Stan A, Lewis DA. Altered cortical GABA neurotransmission in schizophrenia: insights into novel therapeutic strategies. Current Pharm Biotechnol (2012) 13(8):1557–62. doi: 10.2174/138920112800784925
76. Vierling-Claassen D, Siekmeier P, Stufflebeam S, Kopell N. Modeling GABA alterations in schizophrenia: a link between impaired inhibition and altered gamma and beta range auditory entrainment. J Neurophysiol (2008) 99(5):2656–71. doi: 10.1152/jn.00870.2007
77. Spencer KM. The functional consequences of cortical circuit abnormalities on gamma oscillations in schizophrenia: insights from computational modeling. Front Hum Neurosci (2009) 3:33. doi: 10.3389/neuro.09.033.2009
78. Metzner C, Schweikard A, Zurowski B. Multifactorial modelling of impairment of evoked gamma range oscillation sine schizophrenia. Front Comput Neurosci (2016) 10:89. doi: 10.3389/fncom.2016.00089
79. John YJ, Zikopoulos B, Bullock D, Barbas H. Visual attention deficits in schizophrenia can arise from inhibitory dysfunction in thalamus or cortex. Comput Psychiatry (2018) 2:223–57. doi: 10.1162/cpsy_a_00023
80. Tanaka S. Dysfunctional GABAergic inhibition in the prefrontal cortex leading to “psychotic”hyperactivation. BMC neuroscience (2008) 9(1):41. doi: 10.1186/1471-2202-9-41
81. Howes OD, Kapur S. The dopamine hypothesis of schizophrenia: version III—the final common pathway. Schizophr Bull (2009) 35(3):549–62. doi: 10.1093/schbul/sbp006
82. Okimura T, Tanaka S, Maeda T, Kato M, Mimura M. Simulation of the capacity and precision of working memory in the hypodopaminergic state: relevance to schizophrenia. Neuroscience (2015) 295:80–9. doi: 10.1016/j.neuroscience.2015.03.039
83. Hassan M, Abbas Q, Seo S-Y, Shahzadi S, Al Ashwal H, Zaki N, et al. Computational modeling and biomarker studies of pharmacological treatment of Alzheimer’s disease. Mol Med Rep (2018) 18(1):639–55. doi: 10.3892/mmr.2018.9044
84. Sasidharakurup H, Dash P, Vijayan A, Nair B, Diwakar S. 2017 Computational modelling of apoptosis in parkinson's disease using biochemical systems theory. International Conference on Advances in Computing, Communications and Informatics ICACCI. IEEE (2017). p. 1229–35. https://ieeexplore.ieee.org/document/8125891
85. Estes ML, McAllister AK. Immune mediators in the brain and peripheral tissues in autism spectrum disorder. Nat Rev Neurosci (2015) 16(8):469. doi: 10.1038/nrn3978
86. Pouget JG. The emerging immunogenetic architecture of schizophrenia. Schizophr bull (2018) 44(5):993– 1004. doi: 10.1093/schbul/sby038
87. Mäki-Marttunen T, Lines GT, Edwards AG, Tveito A, Dale AM, Einevoll GT, et al. Pleiotropic effects of schizophrenia-associated genetic variants in neuron firing and cardiac pacemaking revealed by computational modeling. Translational Psychiatry (2017) 7:5. doi: 10.1038/s41398-017-0007-4
88. Andrade A, Hope J, Allen A, Yorgan V, Lipscombe D, Pan J. A rare schizophrenia risk variant of CACNA1I disrupts CaV3. 3 channel activity. Sci Rep (2016) 6:34233. doi: 10.1038/srep34233
89. Ramirez-Mahaluf JP, Roxin A, Mayberg HS, Compte A. Acomputational model of major depression: the role of glutamate dysfunction on cingulo-frontal network dynamics. Cereb Cortex (2015) 27(1):660–79. doi: 10.1093/cercor/bhv249
90. Choudary P, Molnar M, Evans S, Tomita H, Li J, Vawter M, et al. Altered cortical glutamatergic and GABAergic signal transmission with glial involvement in depression. Proc Natl Acad Sci USA (2005) 102(43):15653–8. doi: 10.1073/pnas.0507901102
91. Ritter-Makinson S, Clemente-Perez A, Higashikubo B, Cho FS, Holden SS, Bennett E, et al. Augmented reticular thalamic bursting and seizures in Scn1a-Dravet syndrome. Cell reports (2019) 26(1):54–64. doi: 10.1016/j.celrep.2018.12.018
92. Turrigiano GG, Nelson SB. Homeostatic plasticity in the developing nervous system. Nat Rev Neurosci (2004) 5(2):97–107. doi: 10.1038/nrn1327
93. Mäki-Marttunen T, Krull F, Bettella F, Hagen E, Næss S, Ness TV, et al. Alterations in schizophrenia-associated genes can lead to increased power in delta oscillations. Cerebral Cortex (2018b) bhy291. 29:875-91. doi: 10.1093/cercor/bhy291
94. Hagen E, Dahmen D, Stavrinou ML, Lindén H, Tetzlaff T, Van Albada S, et al. Hybrid scheme for modeling local field potentials from point-neuron networks. Cereb Cortex (2016) 26(12): 1–36. doi: 10.1186/1471-2202-16-S1-P67
95. Ladenbauer J, Augustin M, Obermayer K. How adaptation currents change threshold, gain, and variability of neuronal spiking. J Neurophysiol (2013) 111(5):939–53. doi: 10.1152/jn.00586.2013
96. Friston KJ, Harrison L, Penny W. Dynamic causal modelling. Neuroimage (2003) 19(4):1273–302. doi: 10.1016/S1053-8119(03)00202-7
97. Bastos AM, Usrey WM, Adams RA, Mangun GR, Fries P, Friston KJ. Canonical microcircuits for predictive coding. Neuron (2012) 76(4):695–711. doi: 10.1016/j.neuron.2012.10.038
98. Díez Á, Ranlund S, Pinotsis D, Calafato S, Shaikh M, Hall M-H, et al. Abnormal frontoparietal synaptic gain mediating the P 300 in patients with psychotic disorder and their unaffected relatives. Hum Brain Mapp (2017) 38(6):3262–76. doi: 10.1002/hbm.23588
99. Dauvermann MR, Whalley HC, Romaniuk L, Valton V, Owens DG, Johnstone EC, et al. The application of nonlinear dynamic causal modelling for fMRI in subjects at high genetic risk of schizophrenia. Neuroimage (2013) 73:16–29. doi: 10.1016/j.neuroimage.2013.01.063
100. Daunizeau J, David O, Stephan KE. Dynamic causal modelling: a critical review of the biophysical and statistical foundations. Neuroimage (2011) 58(2):312–22. doi: 10.1016/j.neuroimage.2009.11.062
101. Montague PR, Dolan RJ, Friston KJ, Dayan P. Computational psychiatry. Trends in cognitive Sciences (2012) 16(1):72–80. doi: 10.1016/j.tics.2011.11.018
102. Wang X-J, Krystal JH. Computational psychiatry. Neuron (2014) 84(3):638–54. doi: 10.1016/j.neuron.2014.10.018
103. Bush WS, Moore JH. Genome-wide association studies. PloS Comput Biol (2012) 8(12):e1002822. doi: 10.1371/journal.pcbi.1002822
104. Kerschensteiner D, Monje F, Stocker M. Structural determinants of the regulation of the voltage-gated potassium channel Kv2. 1 by the modulatory α-subunit Kv9.3. J Biol Chem. (2003) 278(20):18154-61. doi: 10.1074/jbc.M213117200
105. Gurnett CA, Campbell KP. Transmembrane auxiliary subunits of voltage-dependent ion channels. J Biol Chem (1996) 271(45):27975–8. doi: 10.1074/jbc.271.45.27975
106. Ellenbroek B, Youn J. Rodent models in neuroscience research: is it a rat race? Dis models mechanisms (2016) 9(10):1079–87. doi: 10.1242/dmm.026120
107. D’Angelo E, Solinas S, Garrido J, Casellato C, Pedrocchi A, Mapelli J, et al. Realistic modeling of neurons and networks: towards brain simulation. Funct Neurol (2013) 28(3):153. doi: 10.11138/FNeur/2013.28.3.153
108. Baillet S. Forward and inverse problems of MEG/EEG. In: Jaeger D, Jung R, editors. Encyclopedia of computational neuroscience. Springer, USA. (2015). p. 1226–33. doi: 10.1007/978-1-4614-6675-8_529
109. Siekmeier PJ. Computational modelling of psychiatric illnesses via well-defined neurophysiological and neurocognitive biomarkers. Neurosci Biobehav Rev (2015) 57:365–80. doi: 10.1016/j.neubiorev.2015.09.014
110. Telenti A, Pierce LC, Biggs WH, di Iulio J, Wong EH, Fabani MM, et al. Deep sequencing of 10,000 human genomes. Proc Natl Acad Sci USA (2016) 113(42):11901–6. doi: 10.1073/pnas.1613365113
111. Miranda WE, Ngo VA, Perissinotti LL, Noskov SY. Computational membrane biophysics: from ion channels interactions with drugs to cellular function. Biochim Biophys Acta (BBA)-Proteins and Proteomics. (2017) 1865(11 Pt B):1643-53. doi: 10.1016/j.bbapap.2017.08.008
112. Insel TR, Landis SC, Collins FS. The NIH brain initiative. Science (2013) 340(6133):687–8. doi: 10.1126/science.1239276
113. Amunts K, Ebell C, Muller J, Telefont M, Knoll A, Lippert T. The human brain project: creating a European research infrastructure to decode the human brain. Neuron (2016) 92(3):574–81. doi: 10.1016/j.neuron.2016.10.046
114. Markram H, Muller E, Ramaswamy S, Reimann MW, Abdellah M, Sanchez CA, et al. Reconstruction and simulation of neocortical microcircuitry. Cell (2015) 163(2):456–92. doi: 10.1016/j.cell.2015.09.029
115. Avery MC, Krichmar JL. Neuromodulatory systems and their interactions: a review of models, theories, and experiments. Front Neural Circuits (2017) 11:108. doi: 10.3389/fncir.2017.00108
116. Sarma GP, Jacobs TW, Watts MD, Ghayoomie SV, Larson SD, Gerkin RC. Unit testing, model validation, and biological simulation. F1000Research (2016) 5. doi: 10.12688/f1000research.9315.1
117. Lindskog M, Halnes G, Oliveira RF, Hellgren Kotaleski J, Blackwell KT. Biochemical networks in psychiatric disease. In: Systems biology in psychiatric research: from high-throughput data to mathematical modeling. Wiley Online Library, Germany. (2010). p. 301–20. doi: 10.1002/9783527630271.ch14
118. Verkhratsky A, Parpura V. Neurological and psychiatric disorders as a neuroglial failure. Period Biol (2014) 116(2):115–24.
119. Inta D, Lang U, Borgwardt S, Meyer-Lindenberg A, Gass P, (2016). Adult neurogenesis in the human striatum: possible implications for psychiatric disorders. Nature Publishing Group (United Kingdom?) doi: 10.1038/mp.2016.8
120. Cheli VT, Santiago González DA, Smith J, Spreuer V, Murphy GG, Paez PM. L-type voltage-operated calcium channels contribute to astrocyte activation in vitro. Glia (2016) 64(8):1396–415. doi: 10.1002/glia.23013
121. Manninen T, Havela R, Linne M-L. Computational models for calcium-mediated astrocyte functions. Front Comput Neurosci (2018) 12:14. doi: 10.3389/fncom.2018.00014
122. Garieri M, Delaneau O, Santoni F, Fish RJ, Mull D, Carninci P, et al. The effect of genetic variation on promoter usage and enhancer activity. Nat Commun (2017) 8(1):1358. doi: 10.1038/s41467-017-01467-7
123. Turrigiano G. Homeostatic synaptic plasticity: local and global mechanisms for stabilizing neuronal function. Cold Spring Harbor Perspect Biol (2012) 4(1):a005736. doi: 10.1101/cshperspect.a005736
124. Kotaleski JH, Blackwell KT. Modelling the molecular mechanisms of synaptic plasticity using systems biology approaches. Nat Rev Neurosci (2010) 11(4):239. doi: 10.1038/nrn2807
125. Bhalla US. Multiscale modelling and synaptic plasticity. In: Progress in molecular biology and translational science, vol. 123. Elsevier, USA. (2014). p. 351–86. doi: 10.1016/B978-0-12-397897-4.00012-7
126. Vagos MR, van Herck IG, Sundnes J, Arevalo HJ, Edwards AG, Koivumäki JT. Computational modelling of electrophysiology and pharmacotherapy of atrial fibrillation: recent advances and future challenges. Front Physiol (2018) 9:1221. doi: 10.3389/fphys.2018.01221
127. Ni H, Whittaker DG, Wang W, Giles WR, Narayan SM, Zhang H. Synergistic anti-arrhythmic effects in human atria with combined use of sodium blockers and acacetin. Front Physiol (2017) 8:946. doi: 10.3389/fphys.2017.00946
128. Gintant G, Fermini B, Stockbridge N, Strauss D. The evolving roles of human iPSC-derived cardiomyocytes in drug safety and discovery. Cell Stem Cell (2017) 21(1):14–7. doi: 10.1016/j.stem.2017.06.005
129. Vicente J, Zusterzeel R, Johannesen L, Mason J, Sager P, Patel V, et al. Mechanistic model-informed proarrhythmic risk assessment of drugs: review of the “CiPA” initiative and design of a prospective clinical validation study. Clin Pharmacol Ther (2018) 103(1):54–66. doi: 10.1002/cpt.896
130. Prakosa A, Arevalo HJ, Deng D, Boyle PM, Nikolov PP, Ashikaga H, et al. Personalized virtual-heart technology for guiding the ablation of infarct-related ventricular tachycardia. Nature Biomed Eng (2018) 2(10):732. doi: 10.1038/s41551-018-0282-2
131. Takahashi K, Yamanaka S. Induction of pluripotent stem cells from mouse embryonic and adult fibroblast cultures by defined factors. Cell (2006) 126(4):663–76. doi: 10.1016/j.cell.2006.07.024
132. Brennand K, Simone A, Tran N, Gage F. Modeling psychiatric disorders at the cellular and network levels. Mol Psychiatry (2012) 17(12):1239. doi: 10.1038/mp.2012.20
133. Hoffman GE, Hartley BJ, Flaherty E, Ladran I, Gochman P, Ruderfer DM, Stahl EA, et al. Transcriptional signatures of schizophrenia in hiPSC-derived NPCs and neurons are concordant with post-mortem adult brains. Nat Commun (2017) 8(1):2225. doi: 10.1038/s41467-017-02330-5
134. Habela CW, Song H, Ming G-l. Modeling synaptogenesis in schizophrenia and autism using human iPSC derived neurons. Mol Cell Neurosci (2016) 73:52–62. doi: 10.1016/j.mcn.2015.12.002
135. Falk A, Heine VM, Harwood AJ, Sullivan PF, Peitz M, Brüstle O, et al. Modeling psychiatric disorders: from genomic findings to cellular phenotypes. Mol Psychiatry (2016) 21(9):1167. doi: 10.1038/mp.2016.89
136. Espuny-Camacho I, Michelsen KA, Gall D, Linaro D, Hasche A, Bonnefont J, et al. Pyramidal neurons derived from human pluripotent stem cells integrate efficiently into mouse brain circuits in vivo. Neuron (2013) 77(3):440–56. doi: 10.1016/j.neuron.2012.12.011
137. Hockemeyer D, Jaenisch R. Induced pluripotent stem cells meet genome editing. Cell stem cell (2016) 18(5):573–86. doi: 10.1016/j.stem.2016.04.013
138. Windrem MS, Osipovitch M, Liu Z, Bates J, Chandler-Militello D, Zou L, et al. Human iPSC glial mouse chimeras reveal glial contributions to schizophrenia. Cell Stem Cell (2017) 21(2):195–208. doi: 10.1016/j.stem.2017.06.012
139. Mansour AA, Gonçalves JT, Bloyd CW, Li H, Fernandes S, Quang D, et al. An in vivo model of functional and vascularized human brain organoids. Nat Biotechnol (2018) 36(5):432. doi: 10.1038/nbt.4127
140. Metzner C, Mäki-Marttunen T, Zurowski B, Steuber V. Modules for automated validation and comparison of models of neurophysiological and neurocognitive biomarkers of psychiatric disorders: ASSRUnit—a case study. Comput Psychiatry (2018) 2:74–91. doi: 10.1162/cpsy_a_00015
141. Hagen E, Næss S, Ness TV, Einevoll GT. Multimodal modeling of neural network activity: computing LFP, ECoG, EEG and MEG signals with LFPy2.0 . Front Neuroinform (accepted) (2018). doi: 10.3389/fninf.2018.00092
142. Buxton RB. Dynamic models of BOLD contrast. Neuroimage (2012) 62(2):953–61. doi: 10.1016/j.neuroimage.2012.01.012
143. Logothetis NK. What we can do and what we cannot do with fMRI. Nature (2008) 453(7197):869. doi: 10.1038/nature06976
144. Fornito A, Harrison B. Brain connectivity and mental illness. Front Psychiatry (2012) 3:72. doi: 10.3389/fpsyt.2012.00072
145. Schmaal L, Hibar D, Sämann P, Hall G, Baune B, Jahanshad N, et al. Cortical abnormalities in adults and adolescents with major depression based on brain scans from 20 cohorts worldwide in the ENIGMA Major Depressive Disorder Working Group. Mol Psychiatry (2017) 22(6):900. doi: 10.1038/mp.2016.60
146. Hibar D, Westlye LT, Doan NT, Jahanshad N, Cheung J, Ching CR, et al. Cortical abnormalities in bipolar disorder: an MRI analysis of 6503 individuals from the ENIGMA Bipolar Disorder Working Group. Mol Psychiatry (2018) 23(4):932. doi: 10.1038/mp.2017.73
147. Van Erp TG, Walton E, Hibar DP, Schmaal L, Jiang W, Glahn DC, et al. Cortical brain abnormalities in 4474 individuals with schizophrenia and 5098 control subjects via the Enhancing Neuro Imaging Genetics Through Meta Analysis (ENIGMA) Consortium. Biol psychiatry (2018) 84(9):644–54. doi: 10.1016/j.biopsych.2018.04.023
148. Owens E, Bachman P, Glahn DC, Bearden CE. Electrophysiological end ophenotypes for schizophrenia. Harv Rev Psychiatry (2016) 24(2):129. doi: 10.1097/HRP.0000000000000110
149. Jahshan C, Cadenhead KS, Rissling AJ, Kirihara K, Braff DL, Light GA. Automatic sensory information processing abnormalities across the illness course of schizophrenia. Psychol Med (2012) 42(1):85–97. doi: 10.1017/S0033291711001061
150. Light GA, Näätänen R. Mismatch negativity is a breakthrough biomarker for understanding and treating psychotic disorders. Proc Natl Acad Sci USA (2013) 110(38):15175–6. doi: 10.1073/pnas.1313287110
151. Hunt MJ, Kopell NJ, Traub RD, Whittington MA. Aberrant network activity in schizophrenia. Trends Neurosci (2017) 40(6):371-38. doi: 10.1016/j.tins.2017.04.003
152. Ermentrout GB, Chow CC. Modeling neural oscillations. Physiol Behav (2002) 77(4-5):629–33. doi: 10.1016/S0031-9384(02)00898-3
153. Næss S, Chintaluri HC, Ness TV, Dale AM, Einevoll GT, Wöjcik DK. Corrected four-sphere head model for EEG signals. Front Hum Neurosci (2017) 11:490. doi: 10.3389/fnhum.2017.00490
154. Peter J, Siekmeier, David P. vanMaanen Development of antipsychotic medications with novel mechanisms of action based on computational modelling of hippocampal neuropathology. PLoS One (2013) 8(3):e58607. doi: 10.1371/journal.pone.0058607
155. McCulloch AD. Systems biophysics: multiscale biophysical modelling of organ systems. Biophys J (2016) 110(5):1023–7. doi: 10.1016/j.bpj.2016.02.007
Keywords: genome-wide association study, computational modelling, ion channels, schizophrenia, psychotic disorders
Citation: Mäki-Marttunen T, Kaufmann T, Elvsåshagen T, Devor A, Djurovic S, Westlye LT, Linne M-L, Rietschel M, Schubert D, Borgwardt S, Efrim-Budisteanu M, Bettella F, Halnes G, Hagen E, Næss S, Ness TV, Moberget T, Metzner C, Edwards AG, Fyhn M, Dale AM, Einevoll GT and Andreassen OA (2019) Biophysical Psychiatry—How Computational Neuroscience Can Help to Understand the Complex Mechanisms of Mental Disorders. Front. Psychiatry 10:534. doi: 10.3389/fpsyt.2019.00534
Received: 12 December 2018; Accepted: 10 July 2019;
Published: 06 August 2019.
Edited by:
Cynthia H. Y. Fu, University of East London, United KingdomReviewed by:
Tao Liu, Beihang University, ChinaHugo Geerts, In Silico Biosciences, United States
Kyriaki Sidiropoulou, University of Crete, Greece
Copyright © 2019 Mäki-Marttunen, Kaufmann, Elvsåshagen, Devor, Djurovic, Westlye, Linne, Rietschel, Schubert, Borgwardt, Efrim-Budisteanu, Bettella, Halnes, Hagen, Næss, Ness, Moberget, Metzner, Edwards, Fyhn, Dale, Einevoll and Andreassen. This is an open-access article distributed under the terms of the Creative Commons Attribution License (CC BY). The use, distribution or reproduction in other forums is permitted, provided the original author(s) and the copyright owner(s) are credited and that the original publication in this journal is cited, in accordance with accepted academic practice. No use, distribution or reproduction is permitted which does not comply with these terms.
*Correspondence: Tuomo Mäki-Marttunen, dHVvbW9Ac2ltdWxhLm5v