- 1Mood and Anxiety Disorders Research Program, Department of Psychiatry, University of Illinois at Chicago, Chicago, IL, United States
- 2Department of Psychology, University of Illinois at Chicago, Chicago, IL, United States
- 3Mental Health Service, Jesse Brown VA Medical Center, Chicago, IL, United States
Sufficient sleep plays an important role in neurocognitive function, yet, problematic sleep is ubiquitous in the general population. It is also frequently predictive of, and concurrent with, internalizing psychopathologies (IPs) such as anxiety and depression suggesting sleep quality is dimensional and transdiagnostic. Along with problematic sleep, IPs are characterized by negative affectivity, therefore, prominent neurobiological models of internalizing conditions involve the amygdala, a region central to emotion. In resting-state studies (independent of sleep considerations), abnormalities in amygdala-frontal functional connectivity are commonly observed suggesting emotion dysregulation may contribute to clinically-relevant phenotypes. In a separate line of research, studies of sleep deprivation, and insomnia disorder suggest sleep loss may alter amygdala-frontal connectivity. Taken together, findings point to shared neurobiology between sleep and emotion systems, however, the impact of sleep quality on the amygdala circuit in anxiety or depression is unclear. Therefore, we evaluated variance in naturalistic sleep quality on amygdala-based circuity in individuals with and without psychiatric illness. Resting-state fMRI data was collected in 87 un-medicated, treatment-seeking adults diagnosed with a primary anxiety disorder (n = 68) or primary depressive disorder (n = 19) in addition to healthy individuals (n = 40). Regression analysis was conducted with bilateral anatomical amygdala as seed regions and self-reported sleep quality was indexed with a validated self-report measure, the Pittsburgh Sleep Quality Index (PSQI). Post-hoc analysis was performed to evaluate whether diagnostic status (primary anxiety, primary depression, healthy) significantly explained functional connectivity results. Whole-brain regression analysis, controlling for anxiety and depression symptoms, revealed worse sleep quality (i.e., higher PSQI total scores) predicted increased left amygdala-subgenual anterior cingulate functional connectivity and reduced connectivity with posterior cerebellar lobe and superior temporal gyrus. For right amygdala, increased coupling with postcentral gyrus corresponded with worse sleep. Post-hoc analysis did not detect a significant relationship between diagnostic status and whole-brain findings. Results expand on previous studies and indicate variance in sleep quality tracks brain pathways involved in cognitive-emotion functions implicated in the neurobiology of IPs that may extend to individuals at risk for clinical anxiety or depression. Altogether, the clinical relevance of identifying phenotypes to improve our understanding of psychopathology may be improved by incorporating sleep quality.
Introduction
Sufficient sleep is critical for optimal brain function (1, 2) yet problematic sleep such as difficulty falling asleep, staying asleep, waking up too early, and other symptoms of insomnia is prevalent in the general population [e.g., about 30% of adults; (3, 4)] and has a negative impact on mood, cognitive functions, and health placing individuals at increased risk for mortality [e.g., (5, 6)]. Problematic sleep indicative of insomnia is marked by hyper-arousal [e.g., elevated autonomic and physiological activity; (7–9)] and poor sleep is a risk factor in the development and maintenance of mood disorders (10–12). Moreover, common internalizing psychopathologies (IPs) such as major depression, generalized anxiety disorder, social anxiety disorder, and panic disorder (13, 14) are highly comorbid with problematic sleep. For these anxiety disorders, 60–90% report sleep disturbances and for major depression, estimates are 50–83% (15–19). Notably, these IPs are also frequently comorbid with each other. For example, most individuals with major depression will have experienced a concurrent anxiety disorder [e.g., 59%; (20)] and up to 90% of individuals with an anxiety disorder will have had comorbid depression (21) indicating certain shared neural substrates across IPs.
Given strong links between poor sleep and anxiety and depression, variance in sleep quality would be expected to interact with neural pathways implicated in IPs. Yet, the impact of naturalistic sleep in the brain pathophysiology of IPs has been a neglected area of study potentially due to the general view that problematic sleep is a “nuisance” variable (e.g., secondary to primary dysfunction). However, this view is changing given evidence of shared neurobiology between sleep and emotion systems suggesting abnormalities in brain circuits may reflect dysfunction that converge on bidirectional sleep-emotion mechanisms (2, 22, 23).
A hallmark of IPs is negative affectivity (24) and influential neurobiological models of IPs involve the amygdala, a central structure in the neural circuitry of emotion (25, 26). Independent of sleep considerations, individuals with anxiety or depression frequently exhibit excessive amygdala reactivity to salient, negative stimuli compared to healthy participants (27, 28), and though less well studied, problematic sleep is also associated with an amplified amygdala response to relevant, negative stimuli (29) further supporting close ties between IPs and sleep loss. The amygdala has extensive connections in a distributed cortical–subcortical network (30–33), therefore, examining sleep quality in an amygdala-based circuit may advance the identification of phenotypes that cut across conventional diagnostic boundaries (34, 35).
Resting-state functional connectivity (rsFC) is an effective, efficient method for evaluating the relationship of large-scale intrinsic spontaneous brain activity between regions thought to reflect direct and indirect anatomical connections (36–39). Highlighting the widespread functional cortical-subcortical interactions with amygdala, Roy et al. (40) demonstrated spontaneous amygdala activity in healthy individuals is positively predicted by regions involved in evaluative functions, emotion processing, and cognitive-emotion interactions [e.g., anterior cingulate cortex, medial prefrontal cortex, insula, striatum, thalamus; (31, 32, 41)], and negatively coupled with regions associated with internally-directed cognition and effortful emotion regulation [e.g., middle frontal gyrus, posterior cingulate gyrus, precuneus; (42–45)]. In case-controlled rsFC studies with amygdala as the seed region, data show aberrant (hypo- or hyper-connectivity) in cortical and subcortical areas in insomnia, depression, and anxiety. For example, individuals with insomnia disorder (without concurrent psychiatric illness) exhibit altered rsFC between amygdala and the inferior frontal gyrus, subcortical structures (e.g., caudate, thalamus), temporal, parietal, and occipital regions relative to healthy controls (46). Also, limited research comparing insomnia disorder to an IP reported relatively more amygdala-rostral anterior cingulate cortex (ACC) rsFC in primary insomnia compared to generalized anxiety disorder (47). Though findings suggest a compensatory response in insomnia, both groups exhibited less amygdala-rostral ACC coupling than good sleepers signifying diminished top-down control of emotion regulation in these disorders (47). Similar dysfunction has been observed in IPs.
Broadly, anomalous amygdala-related rsFC in circuits that encompass emotion, cognition, emotion regulation, and sensorimotor processes are observed in depression and anxiety disorders. Although results across studies are inconsistent as to the pattern of aberrant connectivity and specific regions (48–55), reduced amygdala-frontal connectivity involving the medial prefrontal cortex (PFC) or ACC has been frequently reported. For example, compared to healthy participants, socially anxious individuals exhibit decreased amygdala-rostral ACC rsFC (55); though see (48); high trait (vs. low trait) anxious individuals, reduced amygdala-ventral medial (VMPFC) rsFC (51); adolescents with generalized anxiety disorder, decreased amygdala-dorsolateral PFC rsFC (52); adolescents with major depression, reduced amygdala-medial PFC rsFC (53); though see (49); and clinically anxious youth, decreased amygdala-VMPFC rsFC (50). While methodological differences across studies (e.g., participant sample including comorbidity, analytic approach) preclude direct comparison between studies, findings generally point to reduced amygdala-frontal rsFC in IPs. However, the extent to which problematic sleep in IPs modulates intrinsic connectivity in the amygdala circuit is unclear.
Therefore, the primary objective in the current study was to expand on the literature by examining the relationship between sleep quality and amygdala-based rsFC in individuals with and without clinical anxiety or depression. Since insufficient sleep is ubiquitous (3, 4), healthy individuals were expected to vary in sleep quality as well. We hypothesized individual differences in subjective sleep would converge on an amygdala-frontal circuit and that less rsFC in this circuit would be predicted by worse sleep across participants.
Methods
Participants
As part of a larger study, 87 treatment-seeking adults diagnosed with a primary anxiety disorder (n = 68) or primary depressive disorder (n = 19) participated. Healthy controls (HC; n = 40) completed the same tasks at the same time points as patients. All 127 participants completed a consent form approved by the local Institutional Review Board at the University of Illinois at Chicago. All participants met with a master's-level clinician or PhD/MD assessor, who performed the Structured Clinical Interview for DSM-5 (56) and other clinician-administered measures. Comorbidity was permitted, therefore, the Hamilton Depression Rating Scale [“HAM-D”; (57)] and Hamilton Anxiety Rating Scale [“HAM-A”; (58)] assessed symptom severity across disorders. All measures, including the Pittsburgh Sleep Quality Index (PSQI) (described below), were collected within a week of the fMRI scan and before receiving pharmacotherapy or psychotherapy. All participants tested negative on a urine toxicology screen before the scan.
All participants in this study were free from psychotropic medication and free from major medical and neurological illness as confirmed by a Board Certified physician. Exclusion criteria for healthy participants included a current or past Axis I disorder. Exclusion criteria for all participants were less than 18 or more than 65 years of age, contraindications to magnetic resonance imaging (e.g., pregnancy, ferrous objects), current substance dependence (within 6 months of the study), history of other major psychiatric illness (e.g., bipolar disorder, psychotic disorders), or current cognitive dysfunction (e.g., traumatic brain injury, pervasive developmental disorder). All participants were compensated for their time and all procedures complied with the Helsinki Declaration.
Subjective Measure of Sleep Quality
The PSQI is a 19-item self-report questionnaire that assesses sleep disturbance over the past month (59). The total score comprises 7 component scores: subjective sleep quality, sleep latency, sleep duration, habitual sleep efficiency, sleep disturbances, use of sleeping medication, and daytime dysfunction. Scores range from 0 to 21 and higher scores represent worse sleep (59).
Resting-State fMRI
Padding with foam cushions was used to reduce head movement. The participants were instructed to fixate on a crosshair centrally displayed on the blank gray screen, relax, and let their mind wander without falling asleep for 8 min.
fMRI Data Acquisition and Preprocessing
Scanning was conducted on a 3 Tesla GE Discovery System (General Electric Healthcare; Waukesha, WI) with an 8-channel head coil. Functional data were acquired using gradient-echo echo planar imaging (EPI) sequence with the following parameters: TR = 2 s, TE = minFull [~25 ms], flip angle = 90°, FOV = 22 × 22 cm2, acquisition matrix 64 × 64, 3-mm slice thickness, 44 axial slices, 180 volumes per run. For anatomical localization, a high-resolution, T1-weighted volumetric anatomical scan was acquired.
Connectivity analyses were performed using the Functional Connectivity (CONN) toolbox, which employs procedures from the Statistical Parametric Mapping software (SPM8; Wellcome Trust Centre for Neuroimaging, London, UK). Four initial volumes from each resting-state run were discarded to allow for T1 equilibration effects. Images were realigned to correct for motion, corrected for errors in slice timing, spatially transformed to standard MNI space using the functional template provided with SPM8, resampled to 2-mm voxels, and smoothed with an 8-mm FWHM Gaussian kernel prior to statistical analysis. All participants had no movement greater than 2-mm translation or 2 degrees rotation across the run. Effects of nuisance variables (global, white matter and CSF signals and movement parameters) were reduced following the CompCor strategy (60); data were band-pass filtered to 0.01–0.09 Hz.
In the seed-based analyses, temporal correlations of the resting-state BOLD signal time series were examined between the left and right amygdala regions anatomically derived from the Automated Anatomical Labeling (AAL) toolbox (61) and the rest of the brain. During second-level processing, PSQI total scores were regressed as a covariate of interest. To control for symptoms, HAM-A and HAM-D total scores were entered as a covariate of no interest. Specifically, due to the high concordance between these measures (r = 0.81, p < 0.001), the summation of HAM-A and HAM-D was used to control for symptom severity.
Following recent guidelines in response to concerns about false positives resulting from lenient significance thresholds (62, 63), whole-brain activity was considered significant if it exceeded adjustment for multiple comparisons across the entire brain (e.g., a whole-brain gray matter mask [volume = 1,459,304 mm3]) as determined via simulation using the 3dClustSim utility (10,000 iterations; updated and “bug-free” on December 2015; [https://afni.nimh.nih.gov/pub/dist/doc/program_help/3dClustSim.html]; 64). Significance at α < 0.05 and a voxel threshold of p < 0.001 yielded a minimum cluster size of 26 voxels (volume = 208 mm3) for the regression analysis.
For significant whole-brain findings, parameter estimates of peak activation (β weights, arbitrary units [a.u.]) were extracted from spherical (10-mm diameter) regions of interest (ROI) from each participant and submitted to Pearson's correlations and scatter plots in the Statistical Package for the Social Sciences (SPSS, Version 22) to illustrate the magnitude and direction of significant effects established at the whole-brain level. To evaluate whether findings were driven by a particular diagnostic group, significant regression results were submitted to post-hoc linear regression analysis in SPSS (enter method) where the neural activity extracted with the spherical ROI was the dependent variable and diagnostic status (dummy coded for primary anxiety, primary depression, and healthy control) was the independent variable (Model 1). To evaluate whether any component(s) of the PSQI significantly predicted whole-brain findings, the same regression analysis was performed (enter method) though the independent variable consisted of the seven PSQI components (Model 2).
Results
Participants
Eighty-eight of the 127 participants were female (69.3%). The average age in years was 25.6 ± 7.5 and the average education level in years was 15.8 ± 2.8. PSQI total scores ranged from 0 to 20 and the average was 6.6 ± 4.3. A PSQI total score > 5 denotes problematic sleep (sensitivity 89.6%, specificity 86.5%) (59), therefore, a portion of participants had substantial sleep difficulties. Regarding race/ethnicity, 60.6% self-identified as Caucasian, 21.3% as Asian or Pacific Islander, 9.4% as African American, 6.4% as other or unknown, and 18.9% as Hispanic or Latino. See Table 1 for demographic characteristics and Table 2 for all concurrent disorders.
Whole-Brain Regression: Left Amygdala
Regression results, controlling for symptom severity, revealed higher PSQI total scores corresponded with increased connectivity to the right subgenual ACC [(8, 14, −12) z = 3.54, k = 28, volume = 224 mm3; p < 0.05 corrected] (Figure 1). In contrast, a negative connectivity pattern was detected for right posterior cerebellar lobe [(10, −70, −42) z = 3.75, k = 89, volume = 712 mm3; p < 0.05 corrected] (Figure 2) and left superior temporal gyrus [(−38, −26, 4) z = 4.04, k = 74, volume = 592 mm3; p < 0.05 corrected] (Figure 3).
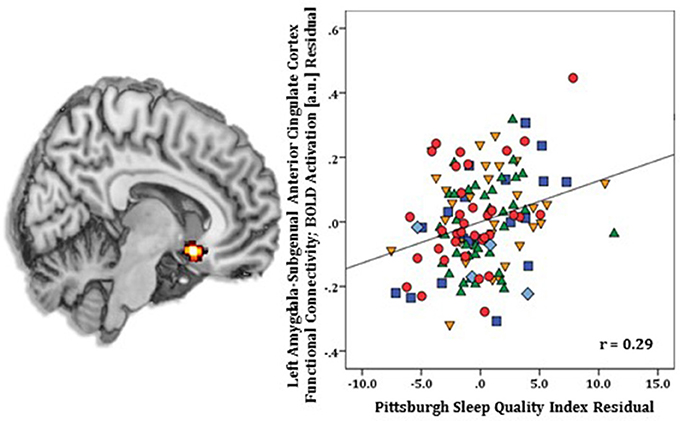
Figure 1. Whole-brain analysis of covariance with sleep quality, indexed with the Pittsburgh Sleep Quality Index (PSQI), showing amygdala-subgenual anterior cingulate cortex parameter estimates of functional connectivity, controlling for symptom severity, on a statistical t-map at p < 0.005 (Left). Scatter plot of the regression analyses depicting extracted parameter estimates of functional connectivity between amygdala and subgenual anterior cingulate cortex, controlling for symptom severity, showing greater connectivity is associated with higher PSQI total scores (Right). Green triangle, healthy participants; dark blue square, depression; orange inverse triangle, generalized anxiety disorder; light blue diamond, panic disorder; red circle, social anxiety disorder.
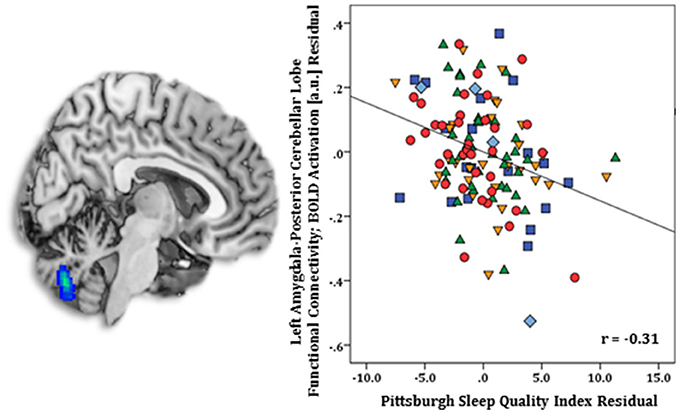
Figure 2. Whole-brain analysis of covariance with sleep quality, assessed with the Pittsburgh Sleep Quality Index (PSQI), showing amygdala-posterior cerebellar lobe parameter estimates of functional connectivity, controlling for symptom severity, on a statistical t-map at p < 0.005 (Left). Scatter plot of the regression analyses depicting extracted parameter estimates of amygdala-posterior cerebellar lobe functional connectivity, controlling for symptom severity, illustrating greater connectivity corresponds with lower PSQI total scores (Right). Green triangle, healthy participants; dark blue square, depression; orange inverse triangle, generalized anxiety disorder; light blue diamond, panic disorder; red circle, social anxiety disorder.
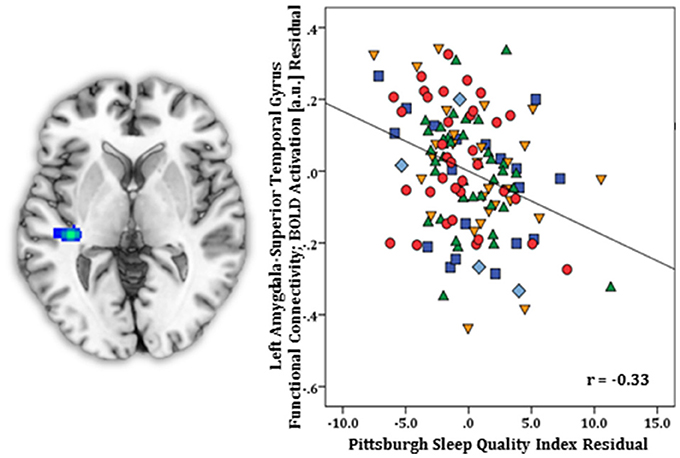
Figure 3. Whole-brain analysis of covariance with sleep quality, examined with the Pittsburgh Sleep Quality Index (PSQI), revealing amygdala-superior temporal gyrus parameter estimates of functional connectivity, controlling for symptom severity, displayed on a statistical t-map at p < 0.005 (Left). Scatter plot of the regression analyses depicting extracted parameter estimates of amygdala-superior temporal gyrus functional connectivity, controlling for symptom severity, demonstrating greater connectivity is linked with lower PSQI total scores (Right). Green triangle, healthy participants; dark blue square, depression; orange inverse triangle, generalized anxiety disorder; light blue diamond, panic disorder; red circle, social anxiety disorder.
In SPSS, post-hoc regression analysis showed diagnostic status did not predict connectivity results (lowest p = 0.16) (Model 1). Also, PSQI component scores were not significantly related to amygdala-subgenual ACC (p = 0.09) or amygdala-cerebellar (p = 0.10) functional connectivity (Model 2). However, amygdala-superior temporal gyrus coupling was predicted by the one-item overall sleep quality component [R2 = 0.16, F(7, 119) = 3.20, p < 0.004] (B = −0.051, t = −1.98, p < 0.05) but no other components (all p's > 0.05).
Whole-Brain Regression: Right Amygdala
Regression results, controlling for symptom severity, showed increased functional connectivity to the right postcentral gyrus [(24, −34, 70) z = 3.64, k = 29, volume = 232 mm3; p < 0.05 corrected] (Figure 4) was predicted by higher PSQI total scores. No negative connectivity patterns were detected.
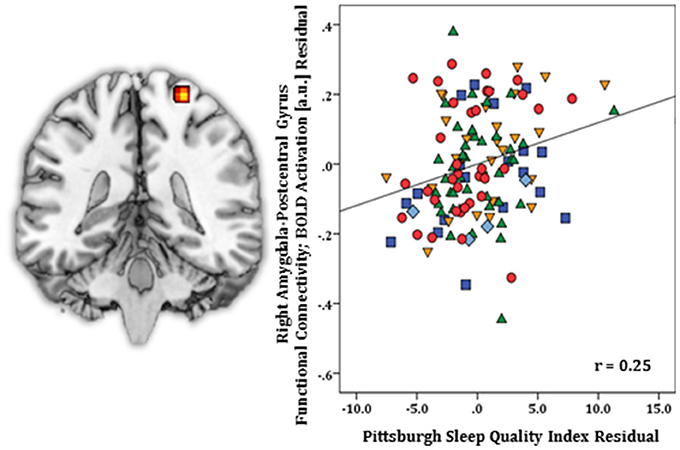
Figure 4. Whole-brain analysis of covariance with sleep quality, indexed with the Pittsburgh Sleep Quality Index (PSQI), showing amygdala-postcentral gyrus parameter estimates of functional connectivity, controlling for symptom severity, on a statistical t-map at p < 0.005 (Left). Scatter plot of the regression analyses depicting extracted parameter estimates of functional connectivity between amygdala and postcentral gyrus, controlling for symptom severity, showing greater connectivity is associated with higher PSQI total scores (Right). Green triangle, healthy participants; dark blue square, depression; orange inverse triangle, generalized anxiety disorder; light blue diamond, panic disorder; red circle, social anxiety disorder.
In SPSS, post-hoc regression analysis revealed diagnostic status did not predict amygdala-postcentral gyrus connectivity p = 0.41 (Model 1), and no particular PSQI component score(s) predicted significant functional connectivity (p = 0.35) (Model 2).
Discussion
The present study used a seed-to-whole brain analysis to examine individual differences in subjective sleep quality, indexed with the PSQI (59), on rsFC for bilateral amygdala in individuals with and without an anxiety or depressive disorder. Controlling for symptom severity, left amygdala revealed worse sleep (i.e., higher PSQI total scores) corresponded with increased FC to subgenual anterior cingulate cortex (sgACC) but less FC to posterior cerebellar lobe and superior temporal gyrus. Regarding right amygdala, poorer sleep was linked with increased FC to postcentral gyrus. Post-hoc regression analysis did not suggest significant FC results related to diagnostic status, and in general, post-hoc regression analysis indicated FC findings were explained by the summation of PSQI component scores. The exception was left amygdala-superior temporal gyrus coupling, which was predicted by the overall sleep quality component, a one-item scale (very good to very bad) but no other components (e.g., sleep latency, sleep duration, habitual sleep efficiency).
Our hypothesis was partially supported as whole-brain analysis revealed sleep quality modulated amygdala-frontal FC, however, the expected pattern of connectivity extrapolated from case-controlled studies of IPs was not supported. That is, more not less coupling between amygdala and sgACC was predicted by worse sleep when controlling for anxiety and depression symptoms. Findings are generally in line with a sleep deprivation resting-state study that reported increased FC between centromedial amygdala and rostral ACC in healthy individuals relative to rested wakefulness (65) and evidence of increased amygdala-rostral ACC FC in insomnia disorder when compared to generalized anxiety disorder (47). Notably, primate and effective connectivity studies indicate the amygdala has direct connections to sgACC (66, 67), a region implicated in the modulation of emotional behavior (68) and known to be dysfunctional in IPs [e.g., depression; (69)]. Taken together, poor sleep-related increased amygdala-ACC connectivity may reflect a compensatory response or hyperarousal (i.e., aberrant synchronization between linked regions).
Findings expand on a meta-analytic study involving anxiety and mood disorders and at-risk populations (e.g., history of childhood adversity, elevated trait anxiety, behavioral inhibition) that demonstrated aberrant (increased or reduced) FC was isolated to amygdala and ventral portions of the ACC (i.e., pregenual ACC, subgenual ACC) (70). Repeated reports of anomalous FC in amygdala-ACC indicates the sub-circuit plays a role in the general emotional dysregulation observed in the IP continuum. While speculative, our results suggest an aspect of amygdala-sgACC heterogeneity in FC may be explained in part by variance in sleep quality.
Worse sleep quality also corresponded with reduced amygdala-posterior cerebellar lobe connectivity. The cerebellum has extensive anatomical and functional connections with limbic (e.g., amygdala) and prefrontal regions (71, 72), and the posterior cerebellar lobe is involved in emotional control and executive function (e.g., planning, set-shifting, working memory) (73). Although it is unclear whether the region has a direct inhibitory effect on amygdala response or whether the amygdala has a modulatory effect on cerebellar activity, the diminished connectivity associated with higher PSQI total scores suggest worse sleep reduced coupling in a sub-circuit involved in higher-order processes (72). Analogous to these results, less intrinsic coupling between amygdala and superior temporal gyrus was also predicted by poorer sleep quality. There is evidence of a direct connection between amygdala and superior temporal gyrus (66), which is central to auditory processing, language function, and social behavior (74–77). Results indicate more sleep loss may have attenuated amygdala-superior temporal co-processing of external stimuli (e.g., noise related to scanner) or behaviors (e.g., self-monitoring) at rest.
For right amygdala, whole-brain regression results, controlling for symptom severity, revealed worse sleep quality corresponded with increased FC to postcentral gyrus, a sensorimotor region (78). In individuals with primary insomnia, increased resting-state FC between right amygdala and postcentral gyrus relative to healthy controls has been reported (46), therefore, results may reflect the heighted physiological arousal and/or cognitive arousal (e.g., intrusive thoughts) associated with problematic sleep (79–83).
When exploring PSQI components, the only item that significantly predicted significant FC was the sleep quality question, which has a similar inference to the PSQI total score. Altogether, more circumscribed components such as sleep latency, sleep duration, habitual sleep efficiency, or daytime dysfunction did not relate to whole-brain regression findings. Thus, whole-brain results were largely driven by individual differences in global sleep quality as opposed to a particular aspect of self-perceived sleep.
Overall, the evaluation of naturalistic sleep quality on resting-state amygdala connectivity is a novel area of study, therefore, it is important to consider that data from sleep deprivation studies involving healthy participants and case-controlled studies of insomnia disorder may not extend to our cohort who vary considerably in sleep quality and on average, experience less severe or less acute sleep loss. For example, sleep deprivation in laboratory settings alters functional connectivity patterns (84) and weakens the modular functional organization of resting-state networks, including the salience network which encompasses the amygdala (85, 86) resulting in a more random network (87). Even though moderate levels of insufficient sleep, when chronic, impairs neurobehavioral functions (88), it is unclear if more modest sleep loss yields similar effects on network properties. Alternatively, in a departure from the modular model, a contemporary formulation of emotion asserts multiple overlapping networks and coordinated activity in large-scale cortical-subcortical systems underlie emotional states (89). The inference is the positive and negative FC predicted by sleep quality in our study modulates a broad interconnected system of which the amygdala circuit is an important hub rather than impacting discrete core amygdala functions (e.g., generation of affect) (89). Thus, further study is needed to understand the intersection of sleep on intrinsic networks in the IP spectrum.
This study is not without important limitations. First, there was an unequal distribution of patients and healthy participants. Moreover, among patients, more individuals had a primary anxiety disorder than major depressive disorder. Therefore, even though diagnostic status did not appear to significantly contribute to whole-brain findings, the majority of participants were anxious. Second, the patient cohort was highly comorbid, therefore, we cannot disambiguate the contribution of anxiety and depression symptoms on findings. Third, the cross-sectional, correlational nature of this study precludes an ability to make causal inferences. Fourth, we did not screen for sleep disorders, therefore, it is not known whether a portion of participants, including healthy individuals, experienced clinical sleep dysfunction. Fifth, there was no direct manipulation of sleep therefore, results rely on indirect estimates of sleep quality. Sixth, the PSQI, not unlike other self-report measures, is subject to inaccuracy; results may not generalize to objective measures of sleep (e.g., actigraphy).
In conclusion, this is the first study we are aware of to show self-perceived sleep quality modulates amygdala rsFC in individuals with and without clinical anxiety or depression. Several lines of research signify mechanisms of emotion dysregulation and problematic sleep are transdiagnostic and inter-related to varying degrees. Our findings indicate individual differences in sleep quality tracks brain pathways involved in cognitive-emotion functions commonly implicated in the neurobiology of IPs. Therefore, the clinical relevance of identifying transdiagnostic phenotypes may be improved by incorporating sleep measures.
Author Contributions
HK contributed to the study design, interpretation of results, and writing of manuscript. BH analyzed the data. KLP contributed to the interpretation of results and writing of manuscript.
Conflict of Interest Statement
The authors declare that the research was conducted in the absence of any commercial or financial relationships that could be construed as a potential conflict of interest.
Acknowledgments
This work was supported by NIMH K23MH093679 (HK), Brain & Behavior Research Foundation (formerly NARSAD) Award (HK) and in part by NIMH R01MH101497 (KLP) and the Center for Clinical and Translational Research (CCTS) UL1RR029879.
References
1. Baglioni C, Nanovska S, Regen W, Spiegelhalder K, Feige B, Nissen C. Sleep and mental disorders: a meta-analysis of polysomnographic research. Psychol Bull. (2016) 142:969–90. doi: 10.1037/bul0000053
2. Harvey AG, Murray G, Chandler RA, Soehner A. Sleep disturbance as transdiagnostic: consideration of neurobiological mechanisms. Clin Psychol Rev. (2011) 31:225–35. doi: 10.1016/j.cpr.2010.04.003
3. Liu Y, Wheaton AG, Chapman DP, Cunningham TJ, Lu H, Croft JB. Prevalence of healthy sleep duration among adults — United States, 2014. MMWR Morb Mortal Weekly Rep. (2016) 65:137–41. doi: 10.15585/mmwr.mm6506a1
4. Roth T. Insomnia: definition, prevalence, etiology, and consequences. J Clin Sleep Med. (2007) 3:S7–S10.
5. Gallicchio L, Kalesan B. Sleep duration and mortality: a systematic review and meta-analysis. J Sleep Res. (2009) 18:148–58. doi: 10.1111/j.1365-2869.2008.00732.x
6. Grandner MA, Chakravorty S, Perlis ML, Oliver L, Gurubhagavatula I. Habitual sleep duration associated with self-reported and objectively determined cardiometabolic risk factors. Sleep Med. (2014) 15:42–50. doi: 10.1016/j.sleep.2013.09.012
7. Harvey A. A cognitive model of insomnia. Behav Res Ther. (2002) 40:869–93. doi: 10.1016/S0005-7967(01)00061-4
8. Kay D, Buysse D. Hyperarousal and beyond: new insights to the pathophysiology of insomnia disorder through functional neuroimaging studies. Brain Sci. (2017) 7:23. doi: 10.3390/brainsci7030023
9. Riemann D, Spiegelhalder K, Feige B, Voderholzer U, Berger M, Perlis M. The hyperarousal model of insomnia: a review of the concept and its evidence. Sleep Med Rev. (2010) 14:19–31. doi: 10.1016/j.smrv.2009.04.002
10. Ford D, Kamerow D. Epidemiologic study of sleep disturbances and psychiatric disorders. An opportunity for prevention? JAMA Psychiatry (1989) 262:1479–84. doi: 10.1001/jama.262.11.1479
11. Gillin JC. Are sleep disturbances risk factors for anxiety, depressive and addictive disorders? Acta Psychiatr Scand Suppl. (1998) 393:39–43. doi: 10.1111/j.1600-0447.1998.tb05965.x
12. Gregory AM, Caspi A, Eley TC, Moffitt TE, Oconnor TG, Poulton R. Prospective longitudinal associations between persistent sleep problems in childhood and anxiety and depression disorders in adulthood. J Abnorm Child Psychol. (2005) 33:157–63. doi: 10.1007/s10802-005-1824-0
13. Hicks BM, DiRago AC, Iacono WG, McGue M. Gene-environment interplay in internalizing disorders: consistent findings across six environmental risk factors. J Child Psychol Psychiatry (2009) 50:1309–17. doi: 10.1111/j.1469-7610.2009.02100.x
14. Sunderland M, Batterham P, Carragher N, Calear A, Slade T. Developing and validating a computerized adaptive test to measure broad and specific factors of internalizing in a community sample. Assessment (2017) 1:107319111770781. doi: 10.1177/1073191117707817
15. Nutt D, Wilson S, Paterson L. Sleep disorders as core symptoms of depression. Dialogues Clin Neurosci. (2008) 10:329–36.
16. Sunderajan P, Gaynes BN, Wisniewski SR, Miyahara S, Fava M, Akingbala F. Insomnia in patients with depression: a STAR*D report. CNS Spectr. (2010) 15:394–404. doi: 10.1017/S1092852900029266
17. Uhde TW, Cortese BM, Vedeniapin A. Anxiety and sleep problems: emerging concepts and theoretical treatment implications. Curr Psychiatry Rep. (2009) 11:269–76. doi: 10.1007/s11920-009-0039-4
18. van Mill JG, Hoogendijk WJG, Vogelzangs N, van Dyck R, Penninx BWJH. Insomnia and sleep duration in a large cohort of patients with major depressive disorder and anxiety disorders. J Clin Psychiatry (2010) 71:239–46. doi: 10.4088/JCP.09m05218gry
19. Raffray T, Bond TLY, Pelissolo A. Correlates of insomnia in patients with social phobia: role of depression and anxiety. Psychiatry Res. (2011) 189:315–7. doi: 10.1016/j.psychres.2011.03.004
20. Kessler RC, Berglund P, Demler O, Jin R, Koretz D, Merikangas KR. National Comorbidity Survey Replication. The epidemiology of major depressive disorder: results from the National Comorbidity Survey Replication (NCS-R). JAMA (2003) 289:3095–105. doi: 10.1001/jama.289.23.3095
21. Gorman JM. Comorbid depression and anxiety spectrum disorders. Depress Anxiety (1996) 4:160–8. doi: 10.1002/(SICI)1520-6394(19964:4<160::AID-DA2>3.0.CO;2-J
22. Cox RC, Olatunji BO. A systematic review of sleep disturbance in anxiety and related disorders. J Anxiety Disord. (2016) 37:104–29. doi: 10.1016/j.janxdis.2015.12.001
23. Gruber R, Cassoff J. The interplay between sleep and emotion regulation: conceptual framework empirical evidence and future directions. Curr Psychiatry Rep. (2014) 16:500. doi: 10.1007/s11920-014-0500-x
24. Goldberg DP, Krueger RF, Andrews G, Hobbs MJ. Emotional disorders: cluster 4 of the proposed meta-structure for DSM-V and ICD-11. Psychol Med. (2009) 39:2043–59. doi: 10.1017/S0033291709990298
25. Davis M, Whalen PJ. The amygdala: vigilance and emotion. Mol Psychiatry (2001) 6:13–34. doi: 10.1038/sj.mp.4000812
26. Phelps EA, LeDoux JE. Contributions of the amygdala to emotion processing: from animal models to human behavior. Neuron (2005) 48:175–187. doi: 10.1016/j.neuron.2005.09.025
27. Etkin A, Wager TD. Functional neuroimaging of anxiety: a meta-analysis of emotional processing in PTSD, social anxiety disorder, and specific phobia. Am J Psychiatry (2007) 164:1476–88. doi: 10.1176/appi.ajp.2007.07030504
28. Hamilton JP, Etkin A, Furman DJ, Lemus MG, Johnson RF, Gotlib IH. Functional neuroimaging of major depressive disorder: a meta-analysis and new integration of base line activation and neural response data. Am J Psychiatry (2012) 169:693–703. doi: 10.1176/appi.ajp.2012.11071105
29. Baglioni C, Spiegelhalder K, Regen W, Feige B, Nissen C, Lombardo C. Insomnia disorder is associated with increased amygdala reactivity to insomnia-related stimuli. Sleep (2014) 37:1907–17. doi: 10.5665/sleep.4240
30. LeDoux JE. Emotion circuits in the brain. Annu Rev Neurosci. (2000) 23:155–84. doi: 10.1146/annurev.neuro.23.1.155
31. Phelps EA. Emotion and cognition: insights from studies of the human amygdala. Annu Rev Psychol. (2006) 57:27–53. doi: 10.1146/annurev.psych.56.091103.070234
32. Phillips ML, Drevets WC, Rauch SL, Lane R. Neurobiology of emotion perception I: the neural basis of normal emotion perception. Biol Psychiatry (2003) 54:504–14. doi: 10.1016/S0006-3223(03)00168-9
33. Young MP, Scannell JW, Burns GA, Blakemore C. Analysis of connectivity: neural systems in the cerebral cortex. Rev Neurosci. (1994) 5:227–50. doi: 10.1515/REVNEURO.1994.5.3.227
34. Insel T, Cuthbert B, Garvey M, Heinssen R, Pine DS, Quinn K. Research domain criteria (RDoC): toward a new classification framework for research on mental disorders. Am J Psychiatry (2010) 167:748–51. doi: 10.1176/appi.ajp.2010.09091379
35. Kozak MJ, Cuthbert BN. The NIMH research domain criteria initiative: background, issues, and pragmatics: NIMH research domain criteria initiative. Psychophysiology (2016) 53:286–97. doi: 10.1111/psyp.12518
36. Greicius MD, Supekar K, Menon V, Dougherty RF. Resting-state functional connectivity reflects structural connectivity in the default mode network. Cereb Cortex (2009) 19:72–8. doi: 10.1093/cercor/bhn059
37. Hagmann P, Cammoun L, Gigandet X, Meuli R, Honey CJ, Wedeen VJ, et al. Mapping the structural core of human cerebral cortex. PLoS Biol. (2008) 6:e159. doi: 10.1371/journal.pbio.0060159
38. van den Heuvel MP, Mandl RCW, Kahn RS, Hulshoff Pol HE. Functionally linked resting-state networks reflect the underlying structural connectivity architecture of the human brain. Hum Brain Mapp. (2009) 30:3127–41. doi: 10.1002/hbm.20737
39. Vincent JL, Patel GH, Fox MD, Snyder AZ, Baker JT, Van Essen DC. Intrinsic functional architecture in the anaesthetized monkey brain. Nature (2007) 447:83–6. doi: 10.1038/nature05758
40. Roy AK, Shehzad Z, Margulies DS, Kelly AMC, Uddin LQ, Gotimer K. Functional connectivity of the human amygdala using resting state fMRI. Neuroimage (2009) 45:614–26. doi: 10.1016/j.neuroimage.2008.11.030
41. Etkin A, Egner T, Kalisch R. Emotional processing in anterior cingulate and medial prefrontal cortex. Trends Cogn Sci. (2011) 15:85–93. doi: 10.1016/j.tics.2010.11.004
42. Buckner RL, Andrews-Hanna JR, Schacter DL. The brain's default network: anatomy, function, and relevance to disease. Anna NY Acad Sci. (2008) 1124:1–38. doi: 10.1196/annals.1440.011
43. Buhle JT, Silvers JA, Wager TD, Lopez R, Onyemekwu C, Kober H, et al. Cognitive reappraisal of emotion: a meta-analysis of human neuroimaging studies. Cereb Cortex (2014) 24:2981–90. doi: 10.1093/cercor/bht154
44. Messina I, Bianco S, Sambin M, Viviani R. Executive and semantic processes in reappraisal of negative stimuli: insights from a meta-analysis of neuroimaging studies. Front Psychol. (2015) 6:956. doi: 10.3389/fpsyg.2015.00956
45. Raichle ME, MacLeod AM, Snyder AZ, Powers WJ, Gusnard DA, Shulman GL. A default mode of brain function. Proc Natl Acad Sci USA (2001) 98:676–682.
46. Huang Z, Liang P, Jia X, Zhan S, Li N, Ding Y, et al. Abnormal amygdala connectivity in patients with primary insomnia: Evidence from resting state fMRI. European Journal of Radiology (2012) 81:1288–95. doi: 10.1016/j.ejrad.2011.03.029
47. Pace-Schott EF, Zimmerman JP, Bottary RM, Lee EG, Milad MR, Camprodon JA. Resting state functional connectivity in primary insomnia, generalized anxiety disorder and controls. Psychiatry Research: Neuroimaging (2017) 265:26–34. doi: 10.1016/j.pscychresns.2017.05.003
48. Anteraper SA, Triantafyllou C, Sawyer AT, Hofmann SG, Gabrieli JD, Whitfield-Gabrieli S. Hyper-connectivity of subcortical resting-state networks in social anxiety disorder. Brain Connect. (2014) 4:81–90. doi: 10.1089/brain.2013.0180
49. Cullen KR, Westlund MK, Klimes-Dougan B, Mueller BA, Houri A, Eberly LE. Abnormal amygdala resting-state functional connectivity in adolescent depression. JAMA Psychiatry (2014) 71:1138. doi: 10.1001/jamapsychiatry.2014.1087
50. Hamm LL, Jacobs RH, Johnson MW, Fitzgerald DA, Fitzgerald KD, Langenecker SA. Aberrant amygdala functional connectivity at rest in pediatric anxiety disorders. Biol Mood Anxiety Disord. (2014) 4:15. doi: 10.1186/s13587-014-0015-4
51. Kim MJ, Gee DG, Loucks RA, Davis FC, Whalen PJ. Anxiety dissociates dorsal and ventral medial prefrontal cortex functional connectivity with the amygdala at rest. Cereb Cortex (2011) 21:1667–73. doi: 10.1093/cercor/bhq237
52. Liu W, Yin D, Cheng W, Fan M, You M, Men W. Abnormal functional connectivity of the amygdala-based network in resting-state FMRI in adolescents with generalized anxiety disorder. Med Sci Monit. (2015) 21:459–67. doi: 10.12659/MSM.893373
53. Pannekoek JN, van der Werff SJA, Meens PHF, van den Bulk BG, Jolles DD, Veer IM. Aberrant resting-state functional connectivity in limbic and salience networks in treatment-naïve clinically depressed adolescents. J Child Psychol Psychiatry (2014) 55:1317–27. doi: 10.1111/jcpp.12266
54. Pannekoek JN, Veer IM, van Tol M-J, van der Werff SJA, Demenescu LR, Aleman A. Aberrant limbic and salience network resting-state functional connectivity in panic disorder without comorbidity. J Affect Disord. (2013) 145:29–35. doi: 10.1016/j.jad.2012.07.006
55. Prater KE, Hosanagar A, Klumpp H, Angstadt M, Phan KL. Aberrant amygdala–frontal cortex connectivity during perception of fearful faces and at rest in generalized social anxiety disorder. Depress Anxiety (2013) 30:234–41. doi: 10.1002/da.22014
56. American Psychiatric Association. Diagnostic and Statistical Manual of Mental Disorders, 5th Edition. Arlington, VA: American Psychiatric Publishing (2013).
57. Hamilton M. A rating scale for depression. J Neurol Neurosurg Psychiatr. (1960) 23:56–62. doi: 10.1136/jnnp.23.1.56
58. Hamilton M. The assessment of anxiety states by rating. Br J Med Psychol. (1959) 32:50–5. doi: 10.1111/j.2044-8341.1959.tb00467.x
59. Buysse DJ, Reynolds CF III, Monk TH, Berman SR, Kupfer DJ. The pittsburgh sleep quality index: a new instrument for psychiatric practice and research. Psychiatry Res. (1989) 28:193–213. doi: 10.1016/0165-1781(89)90047-4
60. Behzadi Y, Restom K, Liau J, Liu TT. A component based noise correction method (CompCor) for BOLD and perfusion based fMRI. Neuroimage (2007) 37:90–101. doi: 10.1016/j.neuroimage.2007.04.042
61. Tzourio-Mazoyer N, Landeau B, Papathanassiou D, Crivello F, Etard O, Delcroix N. Automated anatomical labeling of activations in SPM using a macroscopic anatomical parcellation of the MNI MRI single-subject brain. Neuroimage (2002) 15:273–89. doi: 10.1006/nimg.2001.0978
62. Eklund A, Nichols T, Knutsson H. Cluster failure: why fMRI inferences for spatial extent have inflated false-positive rates. Proc Natl Acad Sci USA. (2016) 113:7900–95. doi: 10.1073/pnas.1602413113
63. Woo C-W, Krishnan A, Wager TD. Cluster-extent based thresholding in fMRI analyses: pitfalls and recommendations. NeuroImage (2014) 91:412–9. doi: 10.1016/j.neuroimage.2013.12.058
64. Cox RW. AFNI: software for analysis and visualization of functional magnetic resonance neuroimages. Comput Biomed Res. (1996) 29:162–173. doi: 10.1006/cbmr.1996.0014
65. Shao Y, Lei Y, Wang L, Zhai T, Jin X, Ni W, et al. Altered resting-state amygdala functional connectivity after 36 Hours of total sleep deprivation. PLoS ONE (2014) 9:e112222. doi: 10.1371/journal.pone.0112222
66. Aggleton JP, Burton MJ, Passingham RE. Cortical and subcortical afferents to the amygdala of the rhesus monkey (Macaca mulatta). Brain Res. (1980) 190:347–68. doi: 10.1016/0006-8993(80)90279-6
67. Stein JL, Wiedholz LM, Bassett DS, Weinberger DR, Zink CF, Mattay VS, et al. A validated network of effective amygdala connectivity. Neuroimage (2007) 36:736–45. doi: 10.1016/j.neuroimage.2007.03.022
68. Drevets WC, Savitz J, Trimble M. The subgenual anterior cingulate cortex in mood disorders. CNS Spectr. (2008) 13:663–81. doi: 10.1017/S1092852900013754
69. Mayberg HS, Liotti M, Brannan SK, McGinnis S, Mahurin RK, Jerabek PA, et al. Reciprocal limbic-cortical function and negative mood: converging PET findings in depression and normal sadness. Am J Psychiatry (1999) 156:675–682.
70. Marusak HA, Thomason ME, Peters C, Zundel C, Elrahal F, Rabinak CA. You say “prefrontal cortex” and I say “anterior cingulate”: meta-analysis of spatial overlap in amygdala-to-prefrontal connectivity and internalizing symptomology. Transl Psychiatry (2016) 6:e944. doi: 10.1038/tp.2016.218
71. Heath RG, Dempesy CW, Fontana CJ, Myers WA. Cerebellar stimulation: effects on septal region, hippocampus, and amygdala of cats and rats. Biol Psychiatry (1978) 13:501–29.
72. Schmahmann JD. Disorders of the cerebellum: Ataxia, Dysmetria of thought, and the cerebellar cognitive affective syndrome. J Neuropsychiatry Clin Neurosci. (2004) 16:367–78. doi: 10.1176/jnp.16.3.367
73. Schmahmann JD, Caplan D. Cognition, emotion and the cerebellum. Brain (2006) 129:290–2. doi: 10.1093/brain/awh730
74. Adolphs R. Is the human amygdala specialized for processing social information? Anna NY Acad Sci. (2003) 985:326–40. doi: 10.1111/j.1749-6632.2003.tb07091.x
75. Gernsbacher MA, Kaschak MP. Neuroimaging studies of language production and comprehension. Annu Rev Psychol. (2003) 54:91–114. doi: 10.1146/annurev.psych.54.101601.145128
76. Martin RC. Language processing: functional organization and neuroanatomical basis. Annu Rev Psychol. (2003) 54:55–89. doi: 10.1146/annurev.psych.54.101601.145201
78. Taylor L, Jones L. Effects of lesions invading the postcentral gyrus on somatosensory thresholds on the face. Neuropsychologia (1997) 35:953–61. doi: 10.1016/S0028-3932(97)00023-7
79. Bonnet MH, Arand DL. Heart rate variability: sleep stage, time of night, and arousal influences. Electroencephalogr Clin Neurophysiol. (1997) 102:390–6. doi: 10.1016/S0921-884X(96)96070-1
80. Bonnet MH, Arand DL. 24-Hour metabolic rate in insomniacs and matched normal sleepers. Sleep (1995) 18:581–8. doi: 10.1093/sleep/18.7.581
81. Broman J-E, Hetta J. Perceived pre-sleep arousal in patients with persistent psychophysiologic and psychiatric insomnia. Nordic J Psychiatry (1994) 48:203–7. doi: 10.3109/08039489409081360
82. Lichstein KL, Rosenthal TL. Insomniacs' perceptions of cognitive versus somatic determinants of sleep disturbance. J Abnorm Psychol. (1980) 89:105–7. doi: 10.1037/0021-843X.89.1.105
83. Nicassio PM, Mendlowitz DR, Fussell JJ, Petras L. The phenomenology of the pre-sleep state: the development of the pre-sleep arousal scale. Behav Res Ther. (1985) 23:263–71. doi: 10.1016/0005-7967(85)90004-X
84. Liu H, Li H, Wang Y, Lei X. Enhanced brain small-worldness after sleep deprivation: a compensatory effect. J Sleep Res. (2014) 23:554–63. doi: 10.1111/jsr.12147
85. Menon V. Salience Network. In: Brain Mapping An Encyclopedic Reference Vol 2: Anatomy and Physiology, Systems ed A. W. Toga (Waltham, MA: Academic Press). p. 597–611.
86. Seeley WW, Menon V, Schatzberg AF, Keller J, Glover GH, Kenna H. Dissociable intrinsic connectivity networks for salience processing and executive control. J Neurosci. (2007) 27:2349–56. doi: 10.1523/JNEUROSCI.5587-06.2007
87. Simon EB, Maron-Katz A, Lahav N, Shamir R, Hendler T. Tired and misconnected: a breakdown of brain modularity following sleep deprivation: impaired modularity of the sleep deprived brain. Hum Brain Mapp. (2017) 38:3300–14. doi: 10.1002/hbm.23596
88. Van Dongen HP, Maislin G, Mullington JM, Dinges DF. The cumulative cost of additional wakefulness: dose-response effects on neurobehavioral functions and sleep physiology from chronic sleep restriction and total sleep deprivation. SLEEP (2003) 26:117–26. doi: 10.1093/sleep/26.2.117
Keywords: fMRI rest, anxiety, depression, sleep, insomnia, neuroimaging
Citation: Klumpp H, Hosseini B and Phan KL (2018) Self-Reported Sleep Quality Modulates Amygdala Resting-State Functional Connectivity in Anxiety and Depression. Front. Psychiatry 9:220. doi: 10.3389/fpsyt.2018.00220
Received: 08 November 2017; Accepted: 07 May 2018;
Published: 29 May 2018.
Edited by:
Amit Anand, Cleveland Clinic Lerner College of Medicine, United StatesReviewed by:
Casimiro Cabrera Abreu, Department of Psychiatry, Queens University, CanadaBeata Godlewska, University of Oxford, United Kingdom
Copyright © 2018 Klumpp, Hosseini and Phan. This is an open-access article distributed under the terms of the Creative Commons Attribution License (CC BY). The use, distribution or reproduction in other forums is permitted, provided the original author(s) and the copyright owner are credited and that the original publication in this journal is cited, in accordance with accepted academic practice. No use, distribution or reproduction is permitted which does not comply with these terms.
*Correspondence: Heide Klumpp, aGtsdW1wcEB1aWMuZWR1