- 1Information Science Institute, Computer Science Centre, University of Geneva, Geneva, Switzerland
- 2Experimental Clinical Psychology, Department of Psychology, University of Würzburg, Würzburg, Germany
- 3Neuroscience of Emotion and Affective Dynamics Lab, Swiss Center for Affective Sciences, Department of Psychology, University of Geneva, Geneva, Switzerland
- 4Department of Clinical Psychology, Behavioural Science Institute, Radboud University Nijmegen, Nijmegen, Netherlands
The present paper explores the benefits and the capabilities of various emerging state-of-the-art interactive 3D and Internet of Things technologies and investigates how these technologies can be exploited to develop a more effective technology supported exposure therapy solution for social anxiety disorder. “DJINNI” is a conceptual design of an in vivo augmented reality (AR) exposure therapy mobile support system that exploits several capturing technologies and integrates the patient’s state and situation by vision-based, audio-based, and physiology-based analysis as well as by indoor/outdoor localization techniques. DJINNI also comprises an innovative virtual reality exposure therapy system that is adaptive and customizable to the demands of the in vivo experience and therapeutic progress. DJINNI follows a gamification approach where rewards and achievements are utilized to motivate the patient to progress in her/his treatment. The current paper reviews the state of the art of technologies needed for such a solution and recommends how these technologies could be integrated in the development of an individually tailored and yet feasible and effective AR/virtual reality-based exposure therapy. Finally, the paper outlines how DJINNI could be part of classical cognitive behavioral treatment and how to validate such a setup.
Introduction
In the well-known movie Amélie (1), the eponymous heroine has trouble managing social situations. When a friend of hers is publicly embarrassed by his boss, being called a vegetable, she intends to help, but does not know how to react. However, her wishful thinking of having someone whispering the perfect response to her at the right time magically comes true, and she sovereignly masters the situation by replying: “You’ll never be a vegetable. Even artichokes have hearts!.” While the dream of being helped out unobtrusively when caught off-guard or feeling insecure in social situation is usually not fulfilled and may even seem naïve, the aim of this paper is to explore the technical possibilities in achieving just this. By using the metaphor of a “DJINNI,” an Arabian mythological spirit that can be summoned to help it’s “Master” and has the ability to hide itself to others, we convey our core idea behind a set of technological solutions for helping individuals fearing social situations.
The last two decades saw the emergence of virtual reality (VR) as a therapeutic tool for treatment of various mental disorders (2). Especially in the field of anxiety disorders, the immersive experience that VR offers has made it a useful tool for exposure therapy, the gold standard in the treatment of these conditions. In fact, in situations where the confrontation with the feared object or situation is expensive (e.g., fear of flying) or difficult to provide (e.g., high buildings in rural areas when treating fear of heights; fear of open spaces; audiences for fear of speaking in public, etc.), virtual reality exposure therapy (VRET) has become a tangible solution (3). Most challenging in that respect, however, is social anxiety disorder (SAD). The nature of social situations that SAD patients fear is heterogeneous. Simulating various in vivo situations in VR where patients can experience possible negative evaluation by others is quite difficult to achieve technologically. A lot of efforts and resources would be required to build virtual environments that would simulate different situations experienced by the patient. In fact, the heterogeneous nature of situations experienced by SAD patients is quite difficult to achieve also for traditional approaches of exposure therapy. While the presence of the therapist in traditional exposure therapy for first guidance or modeling purposes is usual in the treatment of other anxiety disorders (e.g., specific phobia or agoraphobia), it seems rather odd to accompany a patient to speeches they have to give, or to parties, or dates. As a mental disorder, SAD is desperately in need of novel technological therapeutic solutions that can overcome the current limitations. The aim of the present manuscript is to explore the potential of new emerging, alternative technologies, that have advanced a lot in the last years [e.g., augmented reality (AR) glasses and various Internet of Things (IoT) devices such as smartwatches, sporting sensors, etc.], as support tools for exposure therapy. With “DJINNI,” we propose a technological solution integrating VR, AR, and IoT technologies to deliver a complete support solution for patients suffering from SAD as well as for their therapists.
SAD as a Mental Disorder
Social anxiety disorder (or social phobia) is a mental disorder accumulating in frequency (4, 5) with lifetime prevalence rates of 7–13% in Europe (6), and it belongs to the most prevalent mental disorders after depression and substance abuse (7, 8). It is a debilitating condition characterized by marked fear and embarrassment in social and performance situations and when under scrutiny by others (9). SAD typically has a chronic course if untreated (6), and becomes increasingly associated with comorbid mental problems such as depression, or alcohol abuse. In the Netherlands, mental health services for SAD cost 11,952€ per capita. Even the costs for sub-threshold SAD sum up to 4,687€, substantially more than for healthy individuals (10, 11). But, most importantly, it severely disrupts social and occupational functioning (6, 12) of individuals suffering from SAD.
Cognitive models of SAD (13, 14) suggest that socially anxious individuals (SAs) tend to interpret their own performance in social contexts and ambiguous social information surrounding them, in a threat-confirming way (15). In addition, they are believed to quickly attend to negative social stimuli, predominantly faces (16). Both of these cognitive biases are believed to fuel SAs’ subjective experiences of anxiety and reconfirm the fearful convictions they enter a situation with thereby maintaining the disorder (12, 13). Interestingly, measurable relationships with observable behavior (14, 17–20) or physiology (21, 22) have been indecisive. This is intriguing as cumulative evidence suggests that SAs are experienced as somewhat odd in interactions—although no systematic behavioral patterns have been identified (23, 24). With SAs being experts in looking for signs of devaluation, they will most likely sense negative signals validating their fear of negative evaluation (24). Consequently, along with attentional biases, and interpretational biases, measurement of psychophysiological indices and assessment as well as retraining of “true” social behavior in real social contexts should become part of therapeutic approaches in the future.
In sum, while evidence supports the notion that social anxiety is related to a negative interpretive and attentional biases, and elevated subjective anxiety levels (15), deviations in behavioral and physiological indices have been difficult to substantiate. One reason may be that fear is, according to Lang’s model of emotion (25, 26), reflected in three independent but interrelated systems: verbal report, fear related behavior, and patterns of somatic activation. He stresses that these systems are not necessarily synchronized. In fact, it is plausible to assume that, e.g., continuous reporting (on one’s thoughts, behavior, or physiology) will disrupt their natural patterns. Additionally, human (anxious) behavior is often guided by reflexive, automatic behavioral responses inaccessible to introspection (27, 28). When social anxiety is considered, self-report could be compromised by SAs’ proneness to social desirability effects (29), or again cognitive biases (15). Furthermore, SAs’ self-reported deficits in, e.g., social skills appear to be quite accurate in one context (social interaction) but not in another (public speaking) (30).
Another reason for these inconsistencies may lie in the fact that social anxiety is quite heterogeneous. Its specific form knows many facets such as fear of trembling, blushing, or sweating, and speaking, writing, eating/drinking, and urinating in public restrooms. Often, research participants are screened on general aspects of social anxiety, which they all share. However, stimuli or social scenarios might not be specific enough to tap into their particular fear.
Although effective treatment regimens exist, the very same heterogeneity is thought responsible for the smaller treatment outcomes and higher relapse rates when compared to exposure therapy in other anxiety disorders: the effect sizes of SAD treatments (1.16) lag behind those of the other anxiety disorders (>1.74) (31, 32) and of the 60% that remit due to treatment 40% relapse (33). In addition, cognitive behavior therapy (CBT), the gold standard in anxiety treatment, focuses on distorted cognitions and exposure to frequently avoided social situations, but readily ignores shortcomings in subtle interpersonal behavior.
Finally, replicable scientific investigation of observable social behavior in real life is hampered by the necessity to incorporate numbers of confederates. Their (non-)verbal reactions, even after extensive training, are neither completely controllable nor predictable. Physiological assessment with mobile hardware makes “normal” interaction awkward. In addition, a reliable behavioral observation demands at least two observers/film angles capable of registering, e.g., frequency and length of eye contact or physical distance between interlocutors, and a team of observers/evaluators blind to the conditions (of the participant). High-resolution measurement is impossible in real social environments, even under laboratory conditions.
With regard to exposure treatment, SAD is one of the few anxiety disorders where the therapist is usually absent during the in vivo exposure sessions. He or she can only rely on the subjective record of the patient’s description of the situation. They cannot assess the anxiety levels or behaviors “in vivo” to evaluate if the session was successful. In addition, gradual exposure is difficult to accomplish and the therapist cannot serve either as model or as helping hand, should an exposure task seem too difficult.
Current State-of-the-Art Solutions
Virtual Reality Exposure Therapy
To overcome the abovementioned difficulties in creating anxiety evoking, highly controllable, and replicable situations in real life, immersive virtual reality (IVR) technology and VRET has gained considerable attention in research and assessment of anxiety disorders over the last decennia (34). As analog to in vivo exposure, VRET to threat-evoking stimuli has proven to be an equally effective way for provoking (reflexive) threat responses in close-to-real situations and initiating habituation as prerequisite of treatment (35).
However, the difficulty to assess and address the complex pattern of SAD symptoms in VRET is reflected in a relative scarcity of studies in the field. Two meta-analyses by Opriş et al. (36) and a more recent one by Kampmann and colleagues (37) explored the efficacy of technology-assisted interventions for SAD since 1985. They list only a handful of high-quality studies on VRET in SAD. These studies yield generally positive results. In a study by Klinger and colleagues (38), patients participated in virtual conversations in a meeting room and at a dinner table, were scrutinized by, and needed to assert themselves against virtual agents. This treatment was found to be similarly effective as group CBT. Wallach and colleagues (39) found effects comparable to CBT in a public speaking task in a VR scenario while at the same time achieving smaller dropout rates. Similarly, Anderson and colleagues (40) also report significant improvement in a public speaking task in VR and no difference between virtual and in vivo exposure therapy. Moreover, they found the effect to be stable throughout a period of 1 year after treatment. Finally, Kampmann et al. (37) found VRET treatment effects by exposing patients to virtual speech situations, small-talk with strangers, job interviews, dining in a restaurant, having a blind date, or returning bought products to a shop. Most interestingly in this study, the therapist could adjust the number, gender, and gestures of avatars, the friendliness, and to a certain degree content of the semistructures dialogs depending on the patients’ needs, anxiety, and treatment progress. Yet, treatment evaluation was heavily based on subjective self-report questionnaires and improvements in speech performance as the only behavioral measure.
While the studies conducted so far document a promising future for VR applications in SAD treatment, they fall short on exploiting its full potential. Besides often being unpretentious in its audiovisual quality, they put, except of the Kampmann’s study, a strong emphasis on the fear of public speaking, largely neglecting the complex structure of other contexts in which SAD symptoms occur. More importantly, they fail to properly address the dissimilarities that exist between SAD patients with regard to attentional and behavioral indices. Therefore, it is advised to establish a VR treatment program that covers the complex pattern of SAD symptoms while allowing for patient-specific adaptations.
The Potential of AR As a Complementary or an Alternative Tool to VR
Although numerous virtual scenarios exist for treatment purposes, they are generally not individually tailored and very few utilize behavioral measures available through VR technology such as amount of mimicry (41), interpersonal distance and movement speed (42), or gaze direction (43), nor combine them with physiological measures (44). In addition, AR devices would allow therapists to get a first-hand impression of their patient’s behavior in a social situation in order to individually tailor the VRET scenarios. AR could also incorporate physiological data from, e.g., smartwatches to analyze indices of anxiety in a situation, hint on friendly faces in a crowd via emotion recognition, and provide helpful sentences to the patient (invisible for others) should he/she be stuck in a conversation.
Taken together, utilizing IVR or AR means a great step forward in investigating the interplay between self-report, actual behavior, and physiology in social anxiety as well as their response to different aspects of the treatment or their predictive value for treatment outcome. While circumventing the methodological difficulties of assessment in real life, IVR allows investigating differences in behavior such as gaze direction, gaze duration, distance from, and movement speed toward audience/interlocutor, psychophysiology, and voice properties between high and low SAs, in a high ecologically valid setting. In addition, e.g., wearable AR glasses could be used to assess actual social behavior of SADs in real social contexts. But they could also provide cues for positive social interaction via emotion recognition and help out in conversations or provide soothing comments, should the heartbeat registered by peripheral devices indicate anxiety. In sum, such an integrative approach will yield the potentially most reliable source of assessment for all possible indices of social anxiety, will fill the gaps in our comprehension of their correlations and of SAD, and will lead to better treatment in the future.
Application of Wearable AR Glasses in Mental Health
Similar to VR, AR has also been employed in mental disorders for the last two decades, however, in much smaller scale due to AR’s complexity and limitations (45). With the more recent emergence of modern AR wearable glasses, such as Google Glass™, and the maturation of this technology, AR started becoming a serious potential tool for treatment of various mental disorders. AR has been employed as assistive technology for social interaction by assisting users to identify/remember people and acquire more information about these people (46, 47). Swan and colleagues (48) proposed a system for brain training using wearable AR glasses. AR has also been used in treatment of small animal phobia (49, 50) with some promising results. McNaney and colleagues investigate the employment of Google Glass™ wearable glasses as an assistive everyday device for people suffering from Parkinson’s disease (51). Wearable AR glasses are recently also being utilized as tool for supporting and teaching children suffering from autism spectrum disorder in recognizing emotions and social signals in Stanford’s Autism Glass project (52, 53) and as commercial products of the start-up company Brain Power (54).
Although AR has been used in exposure therapy, to date, no serious attempts have been published to use AR in SAD treatment, though its potential use has been speculated (45). However, early attempts at simulating patients in other fields of health sciences using wearable AR glasses can be taken as a demonstration of its feasibility (55).
Proposed Solution
Enhancing CBT with VRET and Augmented Reality Exposure Therapy (ARET)
To address the challenges and limitations of contemporary SAD exposure therapy approaches as described above, DJINNI is proposed as a software and hardware solution that integrates AR and VR and various sensing technologies to support SAD patient’s CBT treatment. In a nutshell, DJINNI would offer (1) an ARET that would compensate for the absence of the therapists during the in vivo exposure experiences and would automatically interpret various events occurring during these experiences and guide and support the patient; and (2) a VRET that would simulate exposure experiences in a safe 3D environment while incorporating the interaction and behavioral data collected from the ARET experiences. The goals and the scenarios of both ARET and VRET will be influenced by the CBT. The experiences and progress statistics collected by both ARET and VRET can influence the course of the whole SAD treatment (Figure 1).
Augmented Reality Exposure Therapy
In the ARET situation, DJINNI will be experienced as an intelligent assistant that guides and supports the patient during in vivo real-life exposure experiences. As illustrated in Figure 2, DJINNI will be experienced by the patient through her/his wearable AR glass as a system that “understands” the patient’s environment, interprets the state of the people in the environment (location, activity, conversational state, emotions, etc.), interprets the social context, assesses the state of the patient/user (location, activity, conversational state, emotions, etc.), and provides support and advices in the in vivo exposure experience by providing cues, advices, and soothing comments. As illustrated in Figure 2, the system’s interaction with the patient follows a gamification approach (56) where progress is measured in scores/rewards and the patient is encouraged to progress in her/his exposure treatment. The gamification approach will be partly based on previous work where techniques for visualization of rewards and progress were employed to motivate eating disorder patients (57–59).
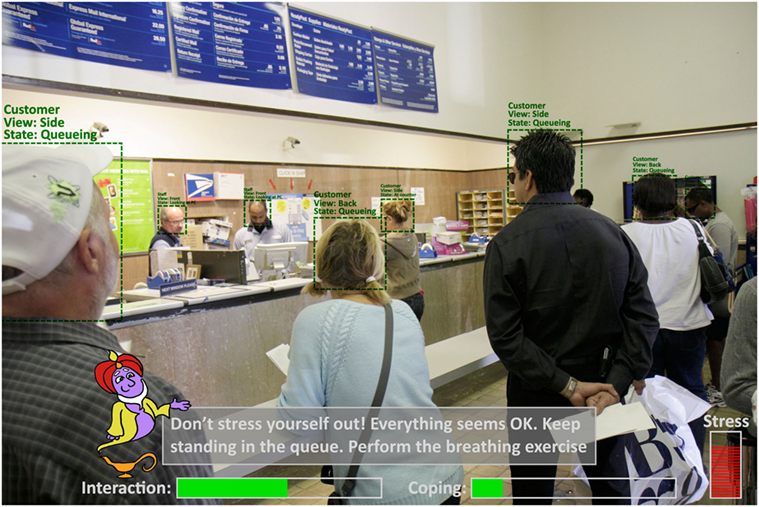
Figure 2. Proposed user interface of DJINNI in in vivo augmented reality exposure therapy situation. (Use of Djinni by courtesy of Gesa Kappen©; use of post-office photo by courtesy of RosaIreneBetancourt 6/Alamy Stock Photo).
Let us say we have a patient suffering from anxiety of being in crowded places with high probability of scrutiny by others. For the in vivo exposure therapy, the patient will be equipped with wearable AR glasses, a smartphone, and a wristband with physiological sensors and will be sent to, for example, a post office. For such system to function properly in an unpredictable environment, it needs to be able to reliably detect and interpret various events occurring in the environment (e.g., spatial information; people in the environment, their roles, and their current actions/behaviors; the patient, her/his actions, and her/his physiological state, etc.). With the help of the sensors, the patient is connected to and/or sensors installed in the environment, and based on the predefined information entered by the therapist about the environment, the system should be able to capture and detect various events reliably if we acknowledge some constrains and limitations as will be discussed later.
In practice, the system will understand that the persons behind the counter or person with specific shirt color and name tags are post office employees. The other persons are clients also waiting in the queue. Upon entering the post office, the system should monitor the physiological state of the patient and how she/he behaves in the environment. When hesitating, the system can display some instructional or motivational messages on the AR glasses on what to do (e.g., DJINNI could say: “You are in the post office. Please stand in the queue and wait for your turn”). When getting stressed the system can attempt to provide messages to calm down the patient (e.g., “Don’t panic. Everything is ok. Please keep standing in the queue”). When detecting the facial expressions and the gaze of others in the environment the system would be able to have a better understanding of the situation (e.g., “The employee just greeted you and smiles at you. Try to smile back”). The system will also be able to detect if anxiety has caused the patient toward performing inappropriate behaviors such as jumping the queue, anxiously looking people right in the eyes, or especially avoiding eye contact (e.g., “Relax. No reason to panic. Don’t stare at her/Try to look into her eyes once a while,” or “Call a friend on the phone to feel better”).
Virtual Reality Exposure Therapy
The DJINNI VRET system will complement the ARET system by allowing the patient to re-experience similar exposure events in the safe 3D VR environment. The novelty in DJINNI’s VRET system in comparison with traditional VRET solutions lies in its incorporation of data collected by the ARET system, and, by doing so, it is possible to automatically generate and adapt VR elements to simulate the events similarly to how they occurred in the in vivo ARET experience. Thus, it gives the patient a platform to, first, re-experience (replay) previous experiences recorded by the ARET system. Consequently, they can learn to objectively analyze/evaluate others’ and one’s own behavior to prevent negative rumination/post-event processing. Finally, they eventually learn how to deal with these experiences in the safe environment of VR as the ARET feeds right into the VRET platform. Similar to the ARET system, the VRET system will also follow a gamification approach to motivate the patient during her/his therapy by feeding back the actual achievements but also the progress that is already accomplished.
Considering the “post-office scenario” as described in Section “Augmented Reality Exposure Therapy,” DJINNI’s VRET system would utilize the data related to the events and the people in the post office environment to automatically adapt the VRET virtual world by simulating similar density of crowd in the post office, specific events that occurred (various people starting looking at the patient, someone in the queue smiled at the patient, etc.), the timing and duration of these events, etc.
Technical Specifications of DJINNI
The DJINNI solution consists of two separate systems that complement each other and exchange data between each other to improve the treatment. As illustrated in Figure 3, DJINNI’s ARET system will rely on several intelligent software components that together determine the functioning and behavior of the system.
In general, DJINNI’s ARET platform consists of the following:
• Wearable AR glasses for displaying DJINNI’s messages to the user and capturing the events in the environment through the built-in camera.
• Smart phone for giving the patient a familiar and safe interface to interact with the system.
• The components environment events tracking, patient behavior interpretation, and people behavior analyze data acquired by the various perception components, the predefined environment information, and workflows and interpret the occurring event, the patient’s current behavior, or the behavior of other people in the environment, respectively. The perception components would detect what they perceive (e.g., woman facing patient is smiling), while the interpretation components would “understand” what it means in the current situation (e.g., the post office employee just greeted the patient with a smile).
• The workflow engine represents the core component of the ARET system and generates the supportive and guiding behaviors of the system by executing the predefined workflows during the exposure experience.
• Progress tracking component tracks the progress of the patient and communicates it with the therapist based on predefined objectives set by the therapist. This component is also responsible for the gamification-based reinforcement/motivation.
• Event tracking and logging logs all the events that occur during an exposure experience, which can be used to generate the VRET scenarios.
• Vision-based analysis represents all the components responsible for analyzing the video captured by the camera of the wearable AR glasses.
• Audio-based analysis represents all the components responsible for analyzing the audio and detected speech and environmental sounds.
• Physiology-based analysis represents the components that measure the patient’s physiological signals through wristband and other sensors.
• Indoor and outdoor localization represent components for determining the geographical location of the patient. Implementation of indoor localization may require equipping popular exposure therapy venues in the city (e.g., postal office, supermarket) with localization sensors that help the system in determining the exact location of the patient in an indoor environment.
• Scenario workflows are predefined workflows/programs defining, in a detailed way, how the system should behave during each situation in a specific exposure scenario/environment. Scenario workflows should be considered as complex but general definitions created for all the patients, while individualized customization are simple adaptations to these general workflows considering specific patient’s needs.
As is the case with the ARET system, the VRET system as illustrated in Figure 4 will also rely on several intelligent software components that together determine the functioning and behavior of the system. However, all the components that analyze and interpret the in vivo situation events are excluded as they are rather simulated instead of captured in the VR environment.
Next to the components already described above for the ARET systems, the VRET system includes the following components:
• Environment simulation component simulates the environment and the events in the environment based on predefined data as well as on data collected by the ARET system.
• People/agents simulation component simulates the behavior of the people in the scenario based on the predefined workflows as well as the by ARET captured data.
Workflows and Interpretations
DJINNI’s scenario workflows will define step by step what actions the system should take at each possible step/situation of a specific exposure experience. Based on previous work on interactive virtual environments (60), the workflows will be implemented in artificial intelligence condition–action rules defining what actions to take given certain derived or predefined data. Table 1 illustrates the types of data that will be derived by the system or predefined by the therapist/programmer that will serve as conditions for determining the system’s actions.
Technological Feasibility
The development of the DJINNI solution would require the integration of various technologies of which some are mature and others are experimental. The following sub-sections will review these technologies and determine their current level of development and will provide recommendations on how these technologies should be integrated and utilized in the DJINNI solution.
Wearable AR Glasses
Although, the idea of having wearable AR glasses as a communication interface for guiding and supporting the patient is innovative, it can also be seen as pitfall. The currently disconnected Google Glass™ wearable glasses (61) have been for a long time seen as the standard in wearable AR glasses. However, due to their cyborg style design, they would not be suitable for patients suffering from SAD to wear them as they would attract undesirable attention. However, the latest years also saw the development of various new wearable AR glasses that may attract less undesirable attention than Google Glass™. Currently available wearable AR glasses such as Laforge Shima™ (62) and Vuzix VidWear™ B3000 (63) will enable DJINNI to exploits the advantages of AR glasses without the negative impact risked if the technology is visible. Furthermore, the emerging waveguide optical technologies [e.g., Trulife Optics™ (64) and Dispelix™ (65)], which is also being used in Laforge and Vuzix, will enable many wearable AR glasses manufacturers to produce more fashionable and less attention-attracting glasses in the near future.
Consequence for DJINNI
For the first prototype of DJINNI, it is the intention to employ Laforge Shima™ or Vuzix VidWear™ B3000 AR glasses.
Audio- and Vision-Based Perception and Interpretation Technologies
Although our perception capabilities as humans is quite advanced and we are able to easily distinguish between the different objects in our human vision and to understand the characteristics and affordances of these objects, yet, for a computer system nowadays, it is impossible to reliably detect and recognize all the objects in its view, let alone to derive their characteristics and affordances. However, for each type of object, specific algorithms have been developed and some algorithms started to reach a level of sufficient maturity (66). Using machine learning techniques, computers are enabled to emulate human cognitive abilities such as sound detection, speech recognition, image recognition, emotion recognition, and behavior analysis.
Speech Recognition
Although speech recognition has significant success in research using techniques such as hidden Markov models (67), practical experiments have shown that currently available speech technologies are still not reliable enough for natural human–computer interaction as most algorithms are still too sensitive to noise and accents (68). In addition, it appears that the most reliable speech recognition stems from the English language (60).
Consequence for DJINNI
Because of its unreliableness, speech recognition technologies will only be used in DJINNI VRET system and only if really needed. Use of speech recognition in public places will be avoided.
Object Recognition/Tracking
Object recognition/tracking has seen some progress in the past decades due to innovations in learning algorithms, image processing techniques, and feature extraction (69, 70). However, most object recognition and tracking algorithms only work well with objects that are near the camera view or objects that are large enough (represented by a large number of pixels in the image).
Consequence for DJINNI
As the camera is part of the wearable glasses, object recognition/tracking algorithms will only be able to detect objects that are in the view of the camera and close to the patient. Small objects and objects far away will not be detected by the system. Therefore, use of object recognition and tracking should be limited in the scenario workflows to large or clearly visible objects and only at certain states of the workflows (e.g., for detecting the post office counter).
Facial Expression Recognition
Facial expression recognition research has been ongoing for some time and has reached a convincing level of maturity. For instance, the facial action coding system, originally designed to allow human users to objectively describe facial states (71), can now be automatically processed and classified into prototypical emotional expressions using computer algorithms (72). Various research and commercial software prototypes have been designed and have reached a sufficient level of recognition.
Consequence for DJINNI
When speaking about emotional expressions, one has to be aware that there is no common definition of what emotions are (73) and workflows should be limited to utilizing prototypical Ekmanian emotions—the basic emotions anger, fear, sadness, disgust, surprise, and happiness, which are believed to be the most universal/stable across cultures and contexts (74). In addition, emotion detection should only occur at states of the workflows where emotions are expected to be detected and relevant. Limiting the scenario will increase the reliability. Furthermore, the system should also be able to differentiate whether someone is talking to the patient or not.
Sound Detection
Sound detection algorithms have reached a very convincing level of maturity in the last years. Reliable commercial and open-source products that have been released can reliably distinguish between a large variety of sounds [e.g., ROAR™ (75) and Audio Analytics™ Ltd. (76)].
Consequence for DJINNI
One of the reliable sound detection products can be used to detect environmental sounds that are relevant to identify certain social environments.
VR Technologies
The last years saw a rapid emergence of high-quality and affordable commercial VR head-mounted devices [e.g., HTC Vive™ (77), Oculus Rift™ (78), Sony PlayStation VR™ (79), Samsung Gear VR™ (80), and Microsoft HoloLens™ (81)].
Consequence for DJINNI
All the existing products have reached sufficient quality and can be directly deployed for DJINNI VRET system.
Physiological and Affective State Interpretation Technologies
Over the last years, various fitness wristband and chest straps have been developed and employed to track one’s fitness performance by integrating peripheral sensors for tracking of motions and heart rate [Mio LINK™ (82), Fitbit Charge HR™ (83), and Garmin Soft Strap Premium HR Monitor™ (84)]. Information derived from these affordable fitness trackers can be used to extract some information about the patient physiological state. The more professional wristband Empatica E4™ (85) has also been gaining prominence the last years in research due to its reliability and the number of sensors that it embeds. Detected sensor signals and signals changes can be directly incorporated into the workflows and can lead to system actions. Furthermore, research has also shown that heart rate variability and galvanic skin response can also be used as a good indicator of stress (86, 87). However, interpretation of psychological signals is also prune to errors due to noise and to the other causes of the same physiological changes.
Consequence for DJINNI
As indicated with other components, it is possible to reach a certain level of reliability if limited to certain scenario. When a change in physiological signals is preceded by events than can cause stress, there is a high chance that the patients feel stressed. By considering these indicators, DJINNI can reach a good level of reliability.
Indoor/Outdoor Localization
Localization can refer to either indoor or outdoor localization, and it refers to locating an object or oneself in a building or outside. Various technologies for outdoor localization already exist. However, it is mainly GPS, sometimes complemented by WIFI router-based localization, that has been the most reliable until now. Indoor localization is enabled by various technologies (e.g., iBeacons, RFID, sound, infrared, ultra-wideband, etc.) (88). However, the most accurate solutions for indoor localization are the ones that combine various technologies rather than using only one (89, 90). Several mature commercial systems exist that also employ various technologies [e.g., IndoorAtlas™ (91) and AccuWare™ (92)].
Consequence for DJINNI
For DJINNI, it is also intended to combine various technologies for indoor localization and create a hybrid system based on previous work (93). However, indoor localization is not the most crucial component of the DJINNI solution. Although the example of the post office may require some indoor localization, many other exposure scenarios do not (e.g., public speaking anxiety, dating anxiety, etc.). For several scenarios where indoor localization is needed, local health institutes can install localization devices in some public buildings if these buildings can be used for exposure therapy (e.g., iBeacons).
Validation Study
As shown above, VR and AR are certainly the keys to improve actual exposure therapies. To validate DJINNI and its ARET and VRET components, the following validation study needs to be conducted. The study aims to evaluate the impact that new technology has on therapeutic progress in a population with, e.g., SAD. The proposed design consists of three consecutive steps.
Diagnostic Phase and Anxiety Evaluation
Naturally, the first step consists of thorough diagnostics of patients, by trained psychologist/therapists, by means of (structured) clinical interviews [e.g., Mini-International Neuropsychiatric Interview (94) or Structured Clinical Interview for DSM-5 (95)] and trait questionnaires [e.g., Social Phobia Scale (96, 97) or Fear of Negative Evaluation Scale (98)]. After the diagnostics, the therapist should repeatedly assess subjective reports of state anxiety in anxiety evoking situations by traditional scales [e.g., State Anxiety Inventory (99), Subjective Units of Discomfort Scale (100), or visual analog scale (101)] and/or its physiological equivalents (e.g., heart rate, skin conductance). These parameters at baseline should be assessed again in the same (formerly) anxiety evoking situations at the end of the treatment (after 12 weeks) and at follow-up (3 months after treatment) to eventually analyze treatment effects.
ARET/VRET/Traditional Exposure Therapies
Assuming that traditional CBT as well as VRET are effective treatments of SAD (37, 102), the experimental design aims to evaluate the added value of adaptive VRET and ARET. Accordingly, six groups of patients, with a principle diagnosis of SAD would be necessary to combine all possible exposure treatments (see Table 2) with ARET.
Based on this semi-experimental design and short-term and long-term changes in subjective experienced anxiety, behavioral and physiological indices can be used to evaluate the added value of (adaptive) VR and AR in exposure therapy. Furthermore, it will also help to assess the importance of technology-based individual tailoring/customization of treatment and evaluate the role of positive or negative feedback on the patient’s behavior.
Conclusion and Discussion
The present article presented a conceptual design of DJINNI, a system that combines AR and VR to provide more effective exposure therapy solutions for patients suffering from SAD. Due to the meager effect sizes of traditional exposure therapies, heterogeneity of the patients’ difficulties in social interaction and the difficulty of personalization in current state-of-the-art VRET, an approach that exploits the benefits of wearable AR glasses is desperately needed.
Yet, the use of AR technology as well as various experimental technologies for environment analysis should not lead to the development of an unreliable system that will not be beneficial or even suitable for use by SAD patients in the end. However, by taking the limitations of various technologies carefully into consideration and incorporating various contextual information regarding the place, situation, and phases of the interaction when developing the solution, it would be possible to achieve a reliable levels of accuracy with the proposed technologies. A thorough evaluation of various technologies is needed before integration into analytical and therapeutic workflows. After doing so, the paper has proposed technologies that have reached a sufficient level of reliability to be considered mature enough for building an effective ARET system.
Another innovation of DJINNI is the adaptive nature of its VRET system. By incorporating data collected from in vivo exposure experience or even everyday social encounters, it is possible to improve and personalize VRET scenarios. Data collected during anxiety evoking events, as well as the behavior of the people in the patients’ environment, can be used to inform the behavior of the virtual environment and the people in it. It is expected that this solution can be more effective than traditional VRET solutions as it is very personalized: It simulates events that have been recently experienced by the patient in in vivo situations, and, yet, can take place in a “safe” therapy setting.
The final deployment of DJINNI would require the development of detailed workflows that define how the system should behave in ARET and VRET situations. This may require considerable work as each possible situation that may occur and possible actions to take by the system need to be defined in the workflows. However, by distinguishing between the general workflows and the individualized customizations, personalizing a system for each patient should not be too time consuming.
Ideally, by providing advanced, high-end technical support solutions, DJINNI could substantially improve the efficacy of CBT (for SAD) and thereby ameliorate the individuals’ suffering and societal burden considerably.
Author Contributions
All authors listed have made substantial, direct, and intellectual contribution to the work and approved it for publication.
Conflict of Interest Statement
The authors declare that the research was conducted in the absence of any commercial or financial relationships that could be construed as a potential conflict of interest.
Acknowledgment
We would like to give special thanks to Mrs. Gesa Kappen for providing us with her lovely Djinni-drawing on such short notice.
Funding
This work was partly supported by the European research projects Miraculous Life (Grant No. 616421), Vizier (Grant No. AAL-2015-2-145), GrowMeUp (Grant No. 643647), and GEO-SAFE (Grant No. 691161).
References
1. Deschamps J-M, Ossard C, Jeunet J-P. Le fabuleux destin d’Amélie Poulain. France: UGC-Fox Distribution (2001).
2. Rizzo AA, Schultheis M, Kerns KA, Mateer C. Analysis of assets for virtual reality applications in neuropsychology. Neuropsychol Rehabil (2004) 14:207–39. doi: 10.1080/09602010343000183
3. Meyerbröker K, Emmelkamp PM. Virtual reality exposure therapy in anxiety disorders: a systematic review of process-and-outcome studies. Depress Anxiety (2010) 27:933–44. doi:10.1002/da.20734
4. Furmark T. Social phobia: overview of community surveys. Acta Psychiatr Scand (2002) 105:84–93. doi:10.1034/j.1600-0447.2002.1r103.x
5. Rapee RM, Spence SH. The etiology of social phobia: empirical evidence and an initial model. Clin Psychol Rev (2004) 24:737–67. doi:10.1016/j.cpr.2004.06.004
6. Fehm L, Pelissolo A, Furmark T, Wittchen H-U. Size and burden of social phobia in Europe. Eur Neuropsychopharmacol (2005) 15:453–62. doi:10.1016/j.euroneuro.2005.04.002
7. Fehm L, Wittchen HU. Comorbidity in social anxiety disorder. In: Bandelow B, Stein DJ, editors. Social Anxiety Disorder. New York: Dekker (2004). p. 49–63.
8. Kessler RC, Berglund P, Demler O, Jin R, Merikangas KR, Walters EE. Lifetime prevalence and age-of-onset distributions of DSM-IV disorders in the national comorbidity survey replication. Arch Gen Psychiatry (2005) 62:593–602. doi:10.1001/archpsyc.62.6.593
9. American Psychiatric Association. Diagnostic and Statistical Manual of Mental Disorders. 5th ed. Washington, DC: American Psychiatric Association (2013).
10. Acarturk C, Smit F, de Graaf R, van Straten A, ten Have M, Cuijpers P. Economic costs of social phobia: a population-based study. J Affect Disord (2009) 115:421–9. doi:10.1016/j.jad.2008.10.008
11. Heimberg RG, Stein MB, Hiripi E, Kessler RC. Trends in the prevalence of social phobia in the United States: a synthetic cohort analysis of changes over four decades. Eur Psychiatry (2000) 15(1):29–37. doi:10.1016/S0924-9338(00)00213-3
12. Stein MB. An epidemiologic perspective on social anxiety disorder. J Clin Psychiatry (2006) 67(Suppl 12):3–8.
13. Clark DM, Wells A. A cognitive model of social phobia. In: Heimberg R, Liebowitz M, Hope D, Schneier F, editors. Social Phobia: Diagnosis, Assessment and Treatment. New York: Guilford Press (1995). p. 69–112.
14. Rapee RM, Heimberg RG. A cognitive-behavioral model of anxiety in social phobia. Behav Res Ther (1997) 35:741–56. doi:10.1016/S0005-7967(97)00022-3
15. Huppert JD, Pasupuleti RV, Foa EB, Mathews A. Interpretation biases in social anxiety: response generation, response selection, and self-appraisals. Behav Res Ther (2007) 45:1505–15. doi:10.1016/j.brat.2007.01.006
16. Staugaard SR. Threatening faces and social anxiety: a literature review. Clin Psychol Rev (2010) 30:669–90. doi:10.1016/j.cpr.2010.05.001
17. Baker SR, Edelmann RJ. Is social phobia related to lack of social skills? Duration of skill-related behaviours and ratings of behavioural adequacy. Br J Clin Psychol (2002) 41:243–57. doi:10.1348/014466502760379118
18. Hofmann SG. Cognitive factors that maintain social anxiety disorder: a comprehensive model and its treatment implications. Cogn Behav Ther (2007) 36(4):193–209. doi:10.1080/16506070701421313
19. Moscovitch DA, Hofmann SG. When ambiguity hurts: social standards moderate self-appraisals in generalized social phobia. Behav Res Ther (2007) 45:1039–52. doi:10.1016/j.brat.2006.07.008
20. Rapee RM, Abbott MJ. Mental representation of observable attributes in people with social phobia. J Behav Ther Exp Psychiatry (2006) 37:113–26. doi:10.1016/j.jbtep.2005.01.001
21. Gramer M, Sprintschnik E. Social anxiety and cardiovascular responses to an evaluative speaking task: the role of stressor anticipation. Pers Individ Dif (2008) 44:371–81. doi:10.1016/j.paid.2007.08.016
22. Mauss I, Wilhelm F, Gross J. Is there less to social anxiety than meets the eye? Emotion experience, expression, and bodily responding. Cogn Emot (2004) 18:631–42. doi:10.1080/02699930341000112
23. Schneider BW, Turk CL. Examining the controversy surrounding social skills in social anxiety disorder: the state of the literature. In: Weeks JW, editor. The Wiley Blackwell Handbook of Social Anxiety Disorder. Chichester, UK: John Wiley & Sons, Ltd (2014). p. 366–87. doi:10.1002/9781118653920.ch17
24. Lange W-G, Rinck M, Becker ES. Behavioral deviations: surface features of social anxiety and what they reveal. In: Weeks JW, editor. The Wiley Blackwell Handbook of Social Anxiety Disorder. Chichester, UK: John Wiley & Sons, Ltd (2014). p. 344–65. doi:10.1002/9781118653920.ch16
25. Lang PJ. A bio-informational theory of emotional imagery. Psychophysiology (1979) 16:495–512. doi:10.1111/j.1469-8986.1979.tb01511.x
26. Lang PJ. The cognitive psychophysiology of emotion: fear and anxiety. In: Maser JD, Tuma AH, editors. Anxiety and the Anxiety Disorders. Hillsdale, NJ: Lawrence Erlbaum Associates (1985). p. 131–70.
27. De Houwer J. What are implicit measures and why are we using them? In: Wiers RW, Stacy AW, editors. Handbook of Implicit Cognition and Addiction. Thousand Oaks, CA: SAGE (2006). p. 11–28. doi:10.4135/9781412976237.n2
28. Strack F, Deutsch R. Reflective and impulsive determinants of social behavior. Pers Soc Psychol Rev (2004) 8:220–47. doi:10.1207/s15327957pspr0803_1
29. Frisch MB. Social-desirability responding in the assessment of social skill and anxiety. Psychol Rep (1988) 63:763–6.
30. Voncken MJ, Bögels SM. Social performance deficits in social anxiety disorder: reality during conversation and biased perception during speech. J Anxiety Disord (2008) 22:1384–92. doi:10.1016/j.janxdis.2008.02.001
31. Bijl RV, Van Zessen G, Ravelli A. Psychiatric morbidity among adults in the Netherlands: the NEMESIS-study. II. Prevalence of psychiatric disorders. Netherlands Mental Health Survey and incidence study. Ned Tijdschr Geneeskd (1997) 141(50):2453–60.
32. Simon NM, Otto MW, Korbly NB, Peters PM, Nicolaou DC, Pollack MH. Quality of life in social anxiety disorder compared with panic disorder and the general population. Psychiatr Serv (2002) 53:714–8. doi:10.1176/appi.ps.53.6.714
33. Fehm L, Beesdo K, Jacobi F, Fiedler A. Social anxiety disorder above and below the diagnostic threshold: prevalence, comorbidity and impairment in the general population. Soc Psychiatry Psychiatr Epidemiol (2008) 43:257–65. doi:10.1007/s00127-007-0299-4
34. Bush J. Viability of virtual reality exposure therapy as a treatment alternative. Comput Human Behav (2008) 24:1032–40. doi:10.1016/j.chb.2007.03.006
35. Powers MB, Emmelkamp PM. Virtual reality exposure therapy for anxiety disorders: a meta-analysis. J Anxiety Disord (2008) 22:561–9. doi:10.1016/j.janxdis.2007.04.006
36. Opriş D, Pintea S, García-Palacios A, Botella C, Szamosközi Ş, David D. Virtual reality exposure therapy in anxiety disorders: a quantitative meta-analysis. Depress Anxiety (2012) 29:85–93. doi:10.1002/da.20910
37. Kampmann IL, Emmelkamp PM, Morina N. Meta-analysis of technology-assisted interventions for social anxiety disorder. J Anxiety Disord (2016) 42:71–84. doi:10.1016/j.janxdis.2016.06.007
38. Klinger E, Bouchard S, Légeron P, Roy S, Lauer F, Chemin I, et al. Virtual reality therapy versus cognitive behavior therapy for social phobia: a preliminary controlled study. Cyberpsychol Behav (2005) 8:76–89. doi:10.1089/cpb.2005.8.76
39. Wallach HS, Safir MP, Bar-Zvi M. Virtual reality cognitive behavior therapy for public speaking anxiety: a randomized clinical trial. Behav Modif (2009) 33:314–38. doi:10.1177/0145445509331926
40. Anderson PL, Price M, Edwards SM, Obasaju MA, Schmertz SK, Zimand E, et al. Virtual reality exposure therapy for social anxiety disorder: a randomized controlled trial. J Consult Clin Psychol (2013) 81:751–60. doi:10.1037/a0033559
41. Vrijsen JN, Lange W-G, Becker ES, Rinck M. Socially anxious individuals lack unintentional mimicry. Behav Res Ther (2010) 48:561–4. doi:10.1016/j.brat.2010.02.004
42. Rinck M, Rörtgen T, Lange W-G, Dotsch R, Wigboldus DH, Becker ES. Social anxiety predicts avoidance behaviour in virtual encounters. Cogn Emot (2010) 24:1269–76. doi:10.1080/02699930903309268
43. Wieser MJ, Pauli P, Grosseibl M, Molzow I, Mühlberger A. Virtual social interactions in social anxiety-the impact of sex, gaze, and interpersonal distance. Cyberpsychol Behav Soc Netw (2010) 13:547–54. doi:10.1089/cyber.2009.0432
44. Wiederhold BK, Wiederhold MD. Virtual Reality Therapy for Anxiety Disorders: Advances in Evaluation and Treatment. 1st ed. Washington, DC: American Psychological Association (2005).
45. Baus O, Bouchard S. Moving from virtual reality exposure-based therapy to augmented reality exposure-based therapy: a review. Front Hum Neurosci (2014) 8:112. doi:10.3389/fnhum.2014.00112
46. Utsumi Y, Kato Y, Kunze K, Iwamura M, Kise K. Who are you? A wearable face recognition system to support human memory. Proceedings of the 4th Augmented Human International Conference. New York, NY: ACM Press (2013). p. 150–3.
47. Mandal B, Chia S-C, Li L, Chandrasekhar V, Tan C, Lim J-H. A wearable face recognition system on Google glass for assisting social interactions. In: Jawahar CV, Shan S, editors. Lecture Notes in Computer Science (Including Subseries Lecture Notes in Artificial Intelligence and Lecture Notes in Bioinformatics) Cham: Springer International Publishing (2014). p. 419–33. doi:10.1007/978-3-319-16634-6_31
48. Swan M, Kido T, Ruckenstein M. BRAINY–multi-modal brain training app for Google glass: cognitive enhancement, wearable computing, and the Internet-of-Things extend personal data analytics. Workshop on Personal Data Analytics in the Internet of Things 40th International Conference on Very Large Databases. Hangzhou, China (2014).
49. Botella C, Breton-López J, Quero S, Baños RM, García-Palacios A, Zaragoza I, et al. Treating cockroach phobia using a serious game on a mobile phone and augmented reality exposure: a single case study. Comput Human Behav (2011) 27:217–27. doi:10.1016/j.chb.2010.07.043
50. Michaliszyn D, Marchand A, Bouchard S, Martel MO, Poirier-Bisson J. A randomized, controlled clinical trial of in virtuo and in vivo exposure for spider phobia. Cyberpsychol Behav Soc Netw (2010) 13:689–95. doi:10.1089/cyber.2009.0277
51. McNaney R, Vines J, Roggen D, Balaam M, Zhang P, Poliakov I, et al. Exploring the acceptability of Google glass as an everyday assistive device for people with Parkinson’s. Proceedings of the 32nd Annual ACM Conference on Human Factors in Computing Systems. New York, NY: ACM Press (2014). p. 2551–4.
52. Voss C, Winograd T, Wall D, Washington P, Haber N, Kline A, et al. Superpower glass: delivering unobstructive real-time social cues in wearable systems. Proceedings of the 2016 ACM International Joint Conference on Pervasive and Ubiquitous Computing Adjunct. New York, NY: ACM Press (2016). p. 1218–26.
53. Washington P, Voss C, Haber N, Tanaka S, Daniels J, Feinstein C, et al. A wearable social interaction aid for children with autism. Proceedings of the 2016 CHI Conference Extended Abstracts on Human Factors in Computing Systems. New York, NY: ACM Press (2016). p. 2348–54.
54. Official Web Page of Brain Power LCC. (2016). Available from: http://www.brain-power.com/
55. Chaballout B, Molloy M, Vaughn J, Brisson Iii R, Shaw R. Feasibility of augmented reality in clinical simulations: using Google glass with manikins. JMIR Med Educ (2016) 2:e2. doi:10.2196/mededu.5159
56. Reeves B, Read JL. Total Engagement: How Games and Virtual Worlds Are Changing the Way People Work and Businesses Compete. Boston, MA: Harvard Business Review Press (2009).
57. Fernández-Aranda F, Jiménez-Murcia S, Santamaría JJ, Gunnard K, Soto A, Kalapanidas E, et al. Video games as a complementary therapy tool in mental disorders: Playmancer, a European multicentre study. J Ment Health (2012) 21:364–74. doi:10.3109/09638237.2012.664302
58. Fagundo AB, Santamaría JJ, Forcano L, Giner-Bartolomé C, Jiménez-Murcia S, Sánchez I, et al. Video game therapy for emotional regulation and impulsivity control in a series of treated cases with bulimia nervosa. Eur Eat Disord Rev (2013) 21:493–9. doi:10.1002/erv.2259
59. Fernandez-Aranda F, Jimenez-Murcia S, Santamaría JJ, Giner-Bartolomé C, Mestre-Bach G, Granero R, et al. The use of videogames as complementary therapeutic tool for cognitive behavioral therapy in bulimia nervosa patients. Cyberpsychol Behav Soc Netw (2015) 18:1–7. doi:10.1089/cyber.2015.0265
60. Tsiourti C, Ben-Moussa M, Quintas J, Loke B, Jochem I, Albuquerque Lopes J, et al. A virtual assistive companion for older adults: design implications for a real-world application. SAI Intelligent Systems Conference. London: IntelliSys (2016).
61. Official Web Page of Google Glass. (2016). Available from: https://www.google.com/glass/
62. Official Web Page of Laforge Shima. (2016). Available from: https://www.laforgeoptical.com/
63. Official Web Page of Vuzix VidWear B3000. (2016). Available from: https://www.vuzix.com/Products/Series-3000-Smart-Glasses
64. Official Web Page of Trulife Optics. (2016). Available from: https://www.trulifeoptics.com/
65. Official Web Page of Dispelix. (2016). Available from: http://www.dispelix.com/
66. Ben-Moussa M, Fanourakis MA, Tsiourti C. How learning capabilities can make care robots more engaging? RoMan 2014 Workshop on Interactive Robots for Aging and/or Impaired People. Edinburgh, UK (2014).
67. Jurafsky D, Martin JH. Speech and Language Processing: An Introduction to Natural Language Processing, Computational Linguistics, and Speech Recognition. Upper Saddle River, NJ: Pearson Prentice Hall (2009).
68. Tsiourti C, Joly E, Ben Moussa M, Wings-Kolgen C, Wac K. Virtual assistive companion for older adults: field study and design implications. 8th International Conference on Pervasive Computing Technologies for Healthcare (Pervasive Health). Oldenburg, Germany: ACM (2014). doi:10.4108/icst.pervasivehealth.2014.254943
69. Park IK, Singhal N, Lee MH, Cho S, Kim C. Design and performance evaluation of image processing algorithms on GPUs. IEEE Trans Parallel Distrib Syst (2011) 22:91–104. doi:10.1109/TPDS.2010.115
70. Sonka M, Hlavac V, Boyle R. Image Processing, Analysis, and Machine Vision. Australia: Cengage Learning (2014).
71. Ekman P, Friesen WV, Hager JC. Facial Action Coding System. Manual and Investigator’s Guide. Salt Lake City, UT: Research Nexus (2002).
72. Pantic M, Bartlett MS. Machine analysis of facial expressions. In: Delac K, Grgic M, editors. Face Recognition. InTech (2007). p. 377–416. doi:10.5772/4847
73. Scherer KR. What are emotions? And how can they be measured? Soc Sci Inf (2005) 44:695–729. doi:10.1177/0539018405058216
74. Ekman P. An argument for basic emotions. Cogn Emot (1992) 6:169–200. doi:10.1080/02699939208411068
75. Romano JM, Brindza JP, Kuchenbecker KJ. ROS open-source audio recognizer: ROAR environmental sound detection tools for robot programming. Auton Robots (2013) 34:207–15. doi:10.1007/s10514-013-9323-6
76. Mitchell CJ. Sound Identification Systems. (2015). Available from: https://www.google.com/patents/US20150106095
77. Official Web Page of HTC Vive. (2016). Available from: http://www.vive.com/
78. Official Web Page of Oculus Rift. (2017). Available from: https://www3.oculus.com/en-us/rift/
79. Official Web Page of Sony PlayStation VR. (2016). Available from: https://www.playstation.com/en-us/explore/playstation-vr/
80. Official Web Page of Samsung Gear VR. (2016). Available from: http://www.samsung.com/global/galaxy/gear-vr/
81. Official Web Page of Microsoft HoloLens. (2016). Available from: https://www.microsoft.com/microsoft-hololens/
82. Official Web Page of Mio LINK. (2016). Available from: http://www.mioglobal.com/en-us/Mio-Link-heart-rate-wristband/Product.aspx
83. Official Web Page of Fitbit Charge HR. (2016). Available from: https://www.fitbit.com/uk/chargehr
84. Official Web Page of Garmin Soft Strap Premium HR Monitor. (2016). Available from: https://buy.garmin.com/en-US/US/shop-by-accessories/fitness-sensors/soft-strap-premium-heart-rate-monitor/prod15490.html
85. Official Web Page of Empatica E4. (2016). Available from: https://www.empatica.com/e4-wristband
86. Hernandez J, McDuff D, Benavides X, Amores J, Maes P, Picard R. AutoEmotive: bringing empathy to the driving experience to manage stress. Proceedings of the 2014 Companion Publication on Designing Interactive Systems – DIS Companion. New York, NY: ACM Press (2014). p. 53–6.
87. Reynolds E. Nevermind: creating an entertaining biofeedback-enhanced game experience to train users in stress management. SIGGRAPH Posters; Anaheim, CA. New York, NY: ACM (2013). doi:10.1145/2503385.2503469
88. Mautz R. Indoor Positioning Technologies. Zurich: ETH Zurich (2012). doi:10.3929/ethz-a-007313554
89. Deak G, Curran K, Condell J. A survey of active and passive indoor localisation systems. Comput Commun (2012) 35:1939–54. doi:10.1016/j.comcom.2012.06.004
90. Xiao J, Zhou Z, Yi Y, Ni LM. A survey on wireless indoor localization from the device perspective. ACM Comput Surv (2016) 49:1–31. doi:10.1145/2933232
91. Official Web Page of IndoorAtlas. (2016). Available from: https://www.indooratlas.com/
92. Official Web Page of AccuWare. (2016). Available from: https://www.accuware.com/
93. Martinez C, Anagnostopoulos GG, Deriaz M. Smart position selection in mobile localisation. The Fourth International Conference on Communications, Computation, Networks and Technologies. Barcelona, Spain (2015).
94. Lecrubier Y, Sheehan D, Weiller E, Amorim P, Bonora I, Harnett Sheehan K, et al. The Mini International Neuropsychiatric Interview (MINI). A short diagnostic structured interview: reliability and validity according to the CIDI. Eur Psychiatry (1997) 12:224–31. doi:10.1016/S0924-9338(97)83296-8
95. First MB, Williams JBW, Karg RS, Spitzer RL. Structured Clinical Interview for DSM-5 Disorders, Clinician Version (SCID-5-CV). Arlington, VA: American Psychiatric Association (2015).
96. Liebowitz MR. Social phobia. Modern Problems of Pharmacopsychiatry (1987). p. 141–73. doi:10.1159/000414022
97. Fresco DM, Coles ME, Heimberg RG, Liebowitz MR, Hami S, Stein MB, et al. The Liebowitz Social Anxiety Scale: a comparison of the psychometric properties of self-report and clinician-administered formats. Psychol Med (2001) 31:1025–35. doi:10.1017/S0033291701004056
98. Leary MR. A brief version of the fear of negative evaluation scale. Pers Soc Psychol Bull (1983) 9:371–5. doi:10.1177/0146167283093007
99. Spielberger CD, Gorsuch RL, Lushene R, Vagg P, Jacobs G. Manual for the State-Trait Anxiety Inventory. Palo Alto, CA: Consulting Psychology Press (1983).
100. Kaplan DM, Smith T, Coons J. A validity study of the subjective unit of discomfort (SUD) score. Meas Eval Couns Dev (1995) 27:195–9.
101. Wewers ME, Lowe NK. A critical review of visual analogue scales in the measurement of clinical phenomena. Res Nurs Health (1990) 13:227–36. doi:10.1002/nur.4770130405
Keywords: social anxiety disorder, social phobia, VRET, exposure therapy, virtual reality therapy, augmented reality
Citation: Ben-Moussa M, Rubo M, Debracque C and Lange W-G (2017) DJINNI: A Novel Technology Supported Exposure Therapy Paradigm for SAD Combining Virtual Reality and Augmented Reality. Front. Psychiatry 8:26. doi: 10.3389/fpsyt.2017.00026
Received: 20 September 2016; Accepted: 01 February 2017;
Published: 28 April 2017
Edited by:
Anna Sort, University of Barcelona, SpainReviewed by:
Mª Angeles Gomez Martínez, Pontifical University of Salamanca, SpainBulent Coskun, Kocaeli University, Turkey
Copyright: © 2017 Ben-Moussa, Rubo, Debracque and Lange. This is an open-access article distributed under the terms of the Creative Commons Attribution License (CC BY). The use, distribution or reproduction in other forums is permitted, provided the original author(s) or licensor are credited and that the original publication in this journal is cited, in accordance with accepted academic practice. No use, distribution or reproduction is permitted which does not comply with these terms.
*Correspondence: Maher Ben-Moussa, maher.benmoussa@unige.ch